Accurate recognition of radar modulation mode helps to better estimate radar echo parameters, thereby occupying an advantageous position in the radar electronic warfare (EW). The pure radar signal representation (PSR) is disentangled from the noise signal representation (NSR) through a feature disentangler and used to learn a radar signal modulation recognizer under low-SNR environments. Signal noise mutual information loss is proposed to enlarge the gap between the PSR and the NSR.
1. Introduction
Accurate identification of the radar signal intrapulse modulation helps to estimate the function of the radar transmitter and improve the accuracy of radar signal parameter estimation, which is critical in electronic intelligence systems, modern electronic support measure systems, and the radar early warning receiver
[1][2][3][4][5]. Nevertheless, the currently widely used pulse compression technique greatly reduces the power spectral density of the radar signal, although it improves the range resolution of the pulse radar. Therefore, under the normal radar operating environments, the signal-to-noise ratio (SNR) of received radar signal is always significantly reduced, thereby seriously affecting the recognition accuracy of radar signal modulation type
[6][7]. How to accurately identify the modulation type of radar signals in a low-SNR environment is still an urgent problem to be solved
[6].
Traditional intrapulse modulation recognition (IPMR) methods consist of feature extraction and classifier
[8]. The accuracy of classic recognition techniques in low-SNR environments is mainly based on feature extraction algorithms, such as high-order cumulants (HOCs), cyclostationary spectrum, instantaneous frequency features, wavelet transformation features, and Wigner Ville distribution (WVD) features
[9][10]. Artificial feature extraction is an extremely skillful task that requires researchers with extensive experience. These approaches are difficult to generalize when recognizing new modulation formats. Recently, the works in
[4][6][10][11] have proposed to automatically learn discriminative feature representation based on deep convolutional neural networks (DCNNs) to identify radar signal modulation format. However, heavy noise negatively affects the feature learning process of the DCNN when the SNR is low, resulting in the failure to guarantee the high performance of IPMR. Therefore, antinoise embedding is crucial for deep radar IPMR models to automatically extract discriminative feature representation.
Some studies have proposed to denoise radar signals with low SNR before identifying modulation categories through deep convolutional networks
[3][12][13][14]. However, these models use denoising as a preprocessing process independent of the modulation recognition step, which is unsuitable for radar signal identification, since the useful signal is inevitably suppressed by noise filters. The residual noise of noncooperative radar signals adversely influences the feature learning of the DCNN when the SNR is below −6 dB
[14].
2. Conventional IPMR under Low SNR
Radar intrapulse modulated signals are difficult to detect and identify due to their extremely low peak power, high duty cycle, and other broad spectrum. Many studies on intrapulse feature extraction use signal statistics, such as cumulants (HOC), spectrum, and time–frequency features, as discriminant features to recognize the format of radar signals
[15][16]. In
[15][16][17], the composite cumulants such as phase jitter, phase offset, and frequency offset are used as extracted features to identify radar intrapulse modulations due to their robustness to noise and model mismatch. Ravi K. and Lunden J. used spectral analysis and instantaneous time-domain to classify digital modulation signals
[4][18].
Traditional radar modulation recognition methods can correctly recognize the radar modulation formats in normal SNR environments. However, the difficulty of extracting the characteristic parameters within the radar signal pulse also increases as these characteristic parameters become more diverse and fragile. Traditional recognition methods may have problems of low identification accuracy and computational complexity under ultralow-SNR conditions.
3. Deep-Learning-Based IPMR in Low-SNR Conditions
Unlike handcrafted feature extraction methods, deep-learning-based models have the capability to automatically capture discriminative feature representations to identify different radar modulation formats.
Artificial neural networks were used as a new method of modulation recognition in
[19] for the first time. Most deep learning-based IPMR approaches typically consist of two steps: denoising processing and modulation classification to improve system performance in low-SNR environments. The method proposed in
[20] involves designing an eight-layer CNN classifier to identify time-frequency images (TFIs), which is preprocessed by a series of 2D Wiener filters, bilinear interpolation and the Otsu method to remove background noise. Qu
[14] proposed the convolutional denoising autoencoder (CDAE) to effectively reduce the interference of low SNR on IPMR and improve the classification performance. In
[10], a deep autoencoder network for modulation classification was proposed. The network is trained with a non-negative constraint algorithm for constraining negative weights and inferring more meaningful hidden structures.
The above methods improve the recognition accuracy in low-SNR environments. However, disentangling the pure signal from the noise in deep feature space is a more straightforward solution when the background noise is extremely strong. The denoising and classification tasks can be synchronously completed in an end-to-end way through disentangled learning, and the two tasks can even supervise and promote each other.
4. Disentangled Learning
As a method of feature decomposition, disentangled learning aims to correctly reveal a set of independent factors that produce the current observation
[19], which has been demonstrated as effective in tasks of image translation and image classification
[21]. Interestingly, in
[22], Han et al. proposed a disentangled-learning-based network for exploring disentangled general representations in biosignal processing.
A disentangled framework is proposed here for not only noise reduction, but also to use disentangled learning to disentangle a low-SNR radar representation into pure PSRs and NSRs. This method has great potential in bridging the gap between radar signal denoising and classification by using denoising-guided disentangled network (DGDNet). It enhances the useful signal by correctly uncovering two independent feature representations in the modulated radar signals.
5. DGDNet
5.1. Structure of The Network
The DGDNet is divided into three parts, namely, the global feature extractor (backbone), the feature disentangler, and the modulation mode recognizer. The Inception_v4 similar backbone is directly used as the global feature extractor to pick up the integrated features, including the useful and noise signals in the TFIs. The featured disentangler includes a pure radar feature extractor and a noise feature extractor that are used to obtain the PSR and NSR, respectively. The cosine distance loss between the ideal images and reconstruction images is proposed to supervise the extraction processes of the PSR and NSR. The signal noise mutual information loss (SNMI) loss between the PSR and NSR is proposed to increase the independence between the pure radar feature extraction process and the noise feature extraction process. Discriminative features are automatically extracted by the modulation mode recognizer to perform the radar signal modulation format classification, as shown in Figure 1.
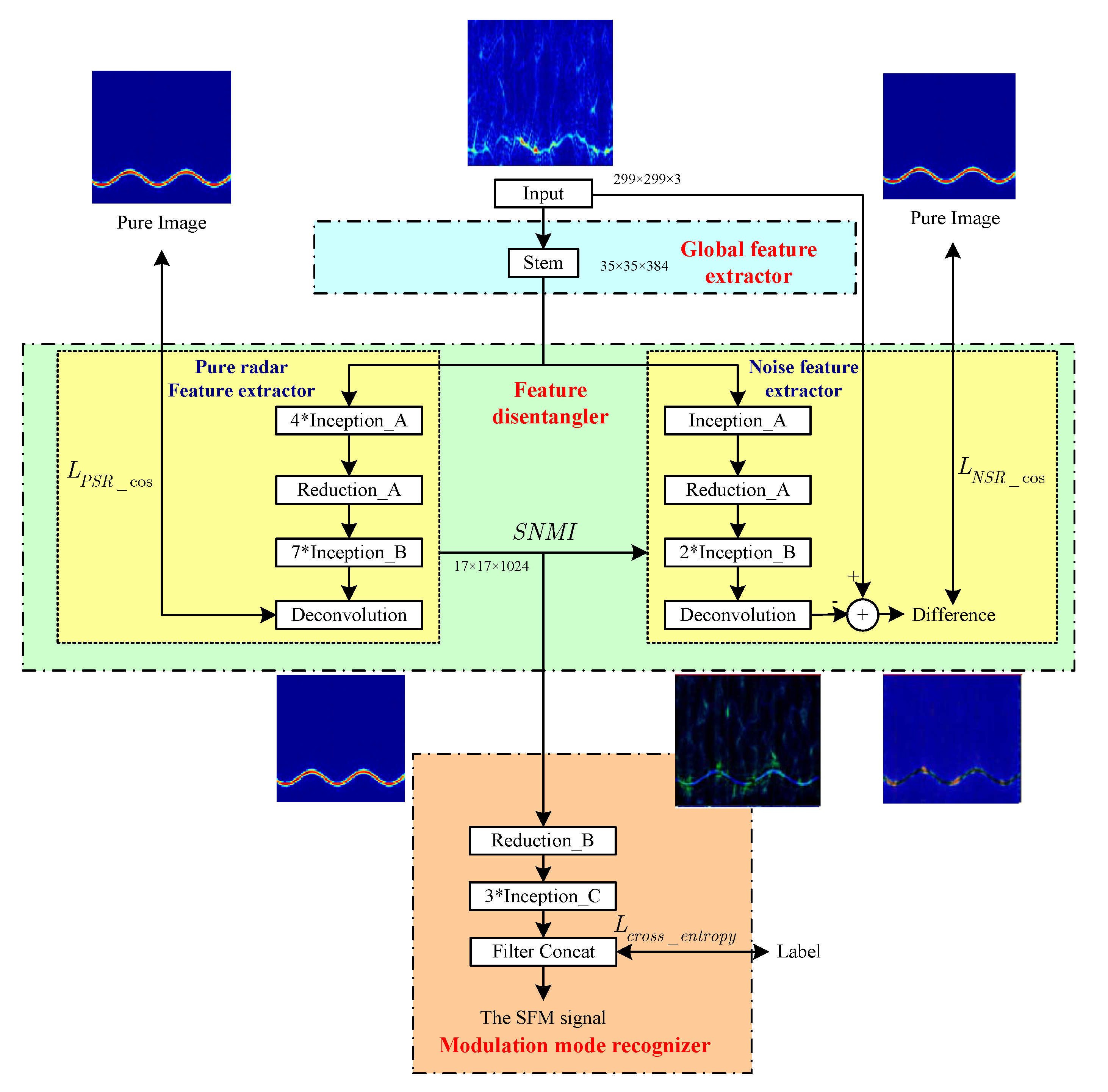
5.2. Global Feature Extractor
The global feature extractor is a stem module (Inception_v4 similar backbone) aimed to obtain the deep features of input TFIs. The network roughly consists of nine layers, where three filter cascade layers are found. Correspondingly, the two small branches in front of each concat layer are used to automatically extract discriminative features at different scales. The output simultaneously contains the useful information and the noise. Its structure is shown in Figure 2.
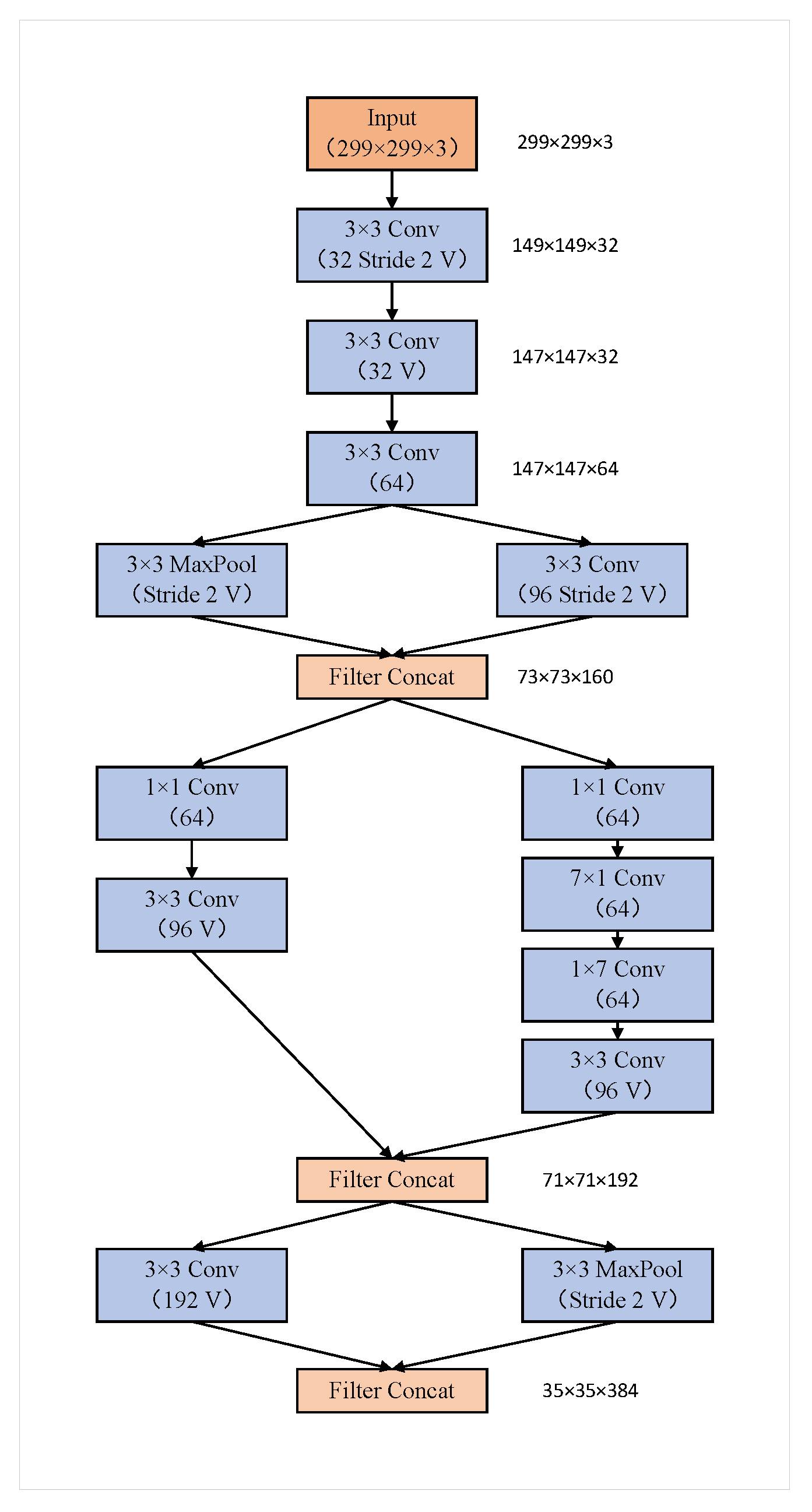
5.3. Feature Disentangler
The output of the global feature extractor is feature maps, including PSR and NSR, and the feature disentangler is devised to progressively disentangle the PSR from the NSR by using the pure radar feature extractor and noise feature extractor.
- Pure Radar Feature Extractor
The pure radar feature extractor includes four Inception_A modules, one Reduction_A module, seven Inception_B modules, and one deconvolution module. The Inception module is used to extract the useful signal features hidden in the TFIs. The reduction layer is applied to reduce the image size. The output of the pure radar feature extractor is the PSR, which can be used to classify different modulation formats. The PSR can be used to reconstruct the denoised TFIs through the deconvolution module.
Similar to the pure radar feature extractor, the noise signal extractor is based on the Inception structure. It contains one Inception_A module, one Reduction_A module, two Inception_B, and one Deconvolution module. The output of the noise feature extractor is the NSR, which can be used to reconstruct the noise images through the deconvolution module. Similar to the pure radar feature extraction process, the TFIs transformed from the radar signal under SNR of 16 dB can be used as the ideal denoising images. Therefore, the ideal noising images can be calculated as the difference between the input noisy TFIs and the ideal denoising images.
6. Conclusions
A novel network called DGDNet is proposed to recognize intrapulse modulation mode of radar signal. The noisy TFIs under low-SNR environments can be obtained through the class time-frequency distribution (CTFD). The DGDNet is used to simultaneously complete the denoising and recognition of noisy TFIs in an end-to-end method. Meanwhile, PSR and NSR can be automatically extracted from the feature disentangler to improve the radar signal modulation identification performance in a low-SNR environment.