Drones are small and low-cost unmanned aerial vehicles (UAVs). With the decrease in the cost and size of drones in recent years, their number has also increased exponentially. As such, the concerns regarding security aspects that are raised by their presence are also becoming more serious.
1. Introduction
Technical innovations continue to manifest at an ever-increasing speed, causing fast and drastic changes to modern society. These changes, driven by the possibilities offered by new technologies, affect citizens, governments, and all public and private industry sectors.
As a result, the development of small, low-cost unmanned aerial vehicles (UAVs), commonly known as drones, has resulted in an ever-increasing number of these devices being utilized in a variety of applications
[1]. UAVs have introduced new participants in aviation, quickly evolving beyond their military origin to become powerful business tools
[2][3].
Applications of UAVs range from recreation to commercial and military applications, including enjoyment, hobbies, games with drones, homemade entertainment videos, recreational movies
[4][5][6], low altitude flying base stations
[7], and the operation of UAVs for military purposes
[8][9][10][11][12][13].
2. The Necessity of Drone Detection and Defense Systems: Incidents and Regulations
The drone industry’s rapid rise has outpaced the rules for safe and secure drone operation, making them a symbol of illegal and destructive terror and crimes
[14].
Drones have gained attention as a threat to safety and security since their entrance into civilian technology, which has fueled the development of anti-drone (or counter-drone) technologies. Anti-drone systems are designed to protect against drone accidents or terrorism, but they will need to evolve in order to deal with future drone flight systems
[15].
UAVs have been used in a variety of military actions. Non-military UAVs have been accused of endangering airplanes, as well as persons and property on the ground. Due to the potential of an ingested drone to quickly damage an aircraft engine
[16], safety concerns have been raised. Multiple near-misses and verified collisions have occurred involving hobbyist UAV pilots operating when violating the aviation safety standards
[17].
3. Drone Detection and Defense Systems: Classification, Sensors, Countermeasures
3.1. Classification of Drone Detection and Defense Systems
Firstly, it is necessary to classify drone detection and defense systems (DDDSs) in order to understand their capabilities, as it is summarized in Table 1.
Table 1. Classification of DDDSs.
Category |
Definition |
Ground-based: fixed |
Systems designed for usage in fixed locations [18] |
Ground-based: mobile |
Systems designed to be installed on automobiles and operated while they are in motion [18] |
Hand-held |
Systems designed to be operated by a single person using their hands; the majority of these systems resemble rifles [19] |
UAV-based |
Systems designed to be mounted on unmanned aerial vehicles (UAVs) [19] |
UAV-swarm-based |
Systems designed to use multiple drones [20] |
3.2. Classification of Detection Sensors
All of the types of sensors that are currently used in DDDS present specific advantages and limitations and, as a direct consequence, such a system must incorporate more sensors of different types in order to achieve a higher detection rate
[18].
The different pros and cons for each category are summarized in Table 2.
Table 2. Pros and cons of sensors used in DDDSs.
Type |
Pros |
Cons |
References |
Acoustic |
-
Covers the spectrum of 20 Hz–20 kHz;
-
Acoustic signature library could be updated easily from flight to flight;
-
Lightweight and can be easily associated with other types of sensors.
|
|
[21][22][23][24][25][26][27][28][29][30][31][32][33][34][35][36][37][38] |
Imaging |
-
Covers all of the visible and IR spectrum (3 MHz–300 GHz);
-
IR cameras could operate in cloudy weather and in day or night;
-
Could be assisted by computer-vision technologies.
|
|
[39][40][41][42][43][44][45][46][47][48][49][50][51][52][53][54][55][56][57] |
Radar |
-
Bandwidth used: 3 MHz–300 GHz;
-
Could operate in all weather and day/night conditions;
-
Offers information regarding the velocity of the target;
-
Can recognize micro-Doppler signatures (MDS)
-
Offers high coverage;
-
Good accuracy;
-
Compact and high mobile, required for tactical applications;
-
High reliability.
|
-
Large radar cross-section is desired;
-
Difficult to differentiate UAVs from birds;
-
Limited performance for low altitudes and speeds (death cone);
-
Could interfere easily with small objects, especially birds;
-
LoS is required;
-
High cost.
|
[58][59][60][61][62][63][64][65][66][67][68][69][70][71][72][73][74][75][76][77][78][79][80][81][82][83][84][85][86][87] |
Radio Frequency (RF) |
-
Capturing the communication spectrum and signals UAV and operators;
-
Low complexity and easy to implement;
-
Could operate in all weather and day/night conditions;
-
Easier to improve due to modular implementation of receivers and digital signal processing units used in implementation;
-
Possibility to localize the pilot.
|
-
Knowledge regarding UAV communication specifications (e.g., frequency bands, modulations, etc.) is required;
-
Difficult to accurately determine AoA;
-
Difficult to use in urban areas due to fading and multipath phenomena;
-
Vulnerable to malicious or illegal modified RF that will exceed receiver capabilities.
|
[88][89][90][91][92][93][94][95][96][97][98] |
4. Drone Detection and Defense Systems Based on RF Methods
One of the most used methods for drone detection is the identification of the RF signals that are exchanged by the drones with another entity (ground station/operator). Moreover, the annihilation of the detected drones can also be obtained by RF methods, by means of transmitting strong enough jamming signals that can interrupt the communication between the drone and its operator.
Usually, drones operate on different frequencies, but most commercial drones operate in Industrial, Scientific, and Medical (ISM) frequency bands of 433 MHz and 2.4/5.8 GHz. The simple power detection in these bands will not work due to the presence of other legitimate users in the same geographical area. Therefore, most of the modern RF detection systems provide the detection and identification of the special and unique signals that are generated by the UAV or the data protocols implemented in a UAV.
There are two main functions that are necessary for the detection of the drones, as follows: The identification of the presence of the drones by scanning the frequency spectrum and localization of the drones. The annihilation function, which is necessary in order to allow the defense against the detected drones, can be performed by means of RF jamming, in order to interrupt the communication between the drones and their operators. Table 3 summarizes the main elements regarding the implementation of such systems.
Table 3. RF-based drone detection and defense systems.
References |
Implemented Functions |
Methods |
SDR Platform Used (Including Manufacturer, City and Country) |
[99] |
Identification Localization |
RF fingerprinting (SFS, WEE, PSE) AoA (MUSIC, RAP MUSIC) |
USRP-X310 (Ettus Research, Santa Clara, CA, USA) |
[100] |
Identification |
RF fingerprinting (DRNN) |
USRP-X310 (Ettus Research, Santa Clara, CA, USA) |
[101] |
Identification |
RF fingerprinting (CNN) |
USRP-X310 (Ettus Research, Santa Clara, CA, USA) |
[102] |
Identification |
RF fingerprinting (KNN) |
USRP-B210 (Ettus Research, Santa Clara, CA, USA) |
[103] |
Identification |
RF fingerprinting (KNN, XGBoost) |
- |
[104] |
Identification |
RF fingerprinting (Wi-Fi) |
- |
[105] |
Identification |
RF fingerprinting |
LimeSDR (Lime Microsystems, Guilford, UK)(customized) |
[106] |
Identification |
RF fingerprinting |
- |
[107] |
Localization |
Received-signal strength (RSS) |
USRP N210 (Ettus Research, Santa Clara, CA, USA) |
[108] |
Localization |
RSS |
AD-FMCOMMS5-EBZ Evaluation Board (Analog Devices, Wilmington, DC, USA) |
[109][110][111] |
Annihilation |
RF jamming |
BladeRF (Nuand, San Francisco, CA, USA) |
[112] |
Annihilation |
RF jamming |
Great Scott Gadgets HackRF One |
5. Challenges and Future Perspectives for Drone Detection and Defense Systems
One of the challenges that is faced when implementing a DDDS is the ability to identify and, in a further step, to annihilate not only one, but several different target drones. In recent years, many applications have used multiple drones
[113], therefore, such a feature becomes an important characteristic for a DDDS. Depending on the sensors that are used in the system, the possibility of detecting several target drones may or may not exist. A few examples of systems that include such a feature exist in the literature. In
[114][115], algorithms are developed in order to allow multi-UAV detection using video streams. In
[116], an RF-based deep learning (DL) algorithm is proposed for performing multiple drone detection. The possibility of a simultaneous annihilation of several drones is an even more challenging task. Electromagnetic pulses (EMP) have been proposed as a possible solution for defense against drone swarms
[117]. RF jamming performed using antenna arrays could also generate, by means of signal processing methods (beamforming), multiple beams that could be targeted towards multiple target drones.
Another challenge that a DDDS would have to face, especially if the area in which the system is installed is a residential area, and there are several households in the close neighborhood, is to avoid interference or damage to nearby equipment (in the case of RF jamming and EMP) and to respect the privacy of the nearby neighbors (in the case of imaging sensors). In the case of RF jamming, this could be solved if the antennas that are used or the beams, in the case of using a beamforming approach, are very directive and targeted directly towards the target drone(s).
6. DronEnd Detection and Defense System
The goal of the DronEnd ground defense system is to secure a certain area against the unauthorized presence of drones. In order to achieve this goal, the DronEnd system scans the RF spectrum in order to detect the presence of the drones in the supervised area, identifies the location of the drone by means of AoA algorithms, and annihilates the drone by using RF jamming methods. The block diagram of the implemented DronEnd ground defense system is presented in Figure 1.
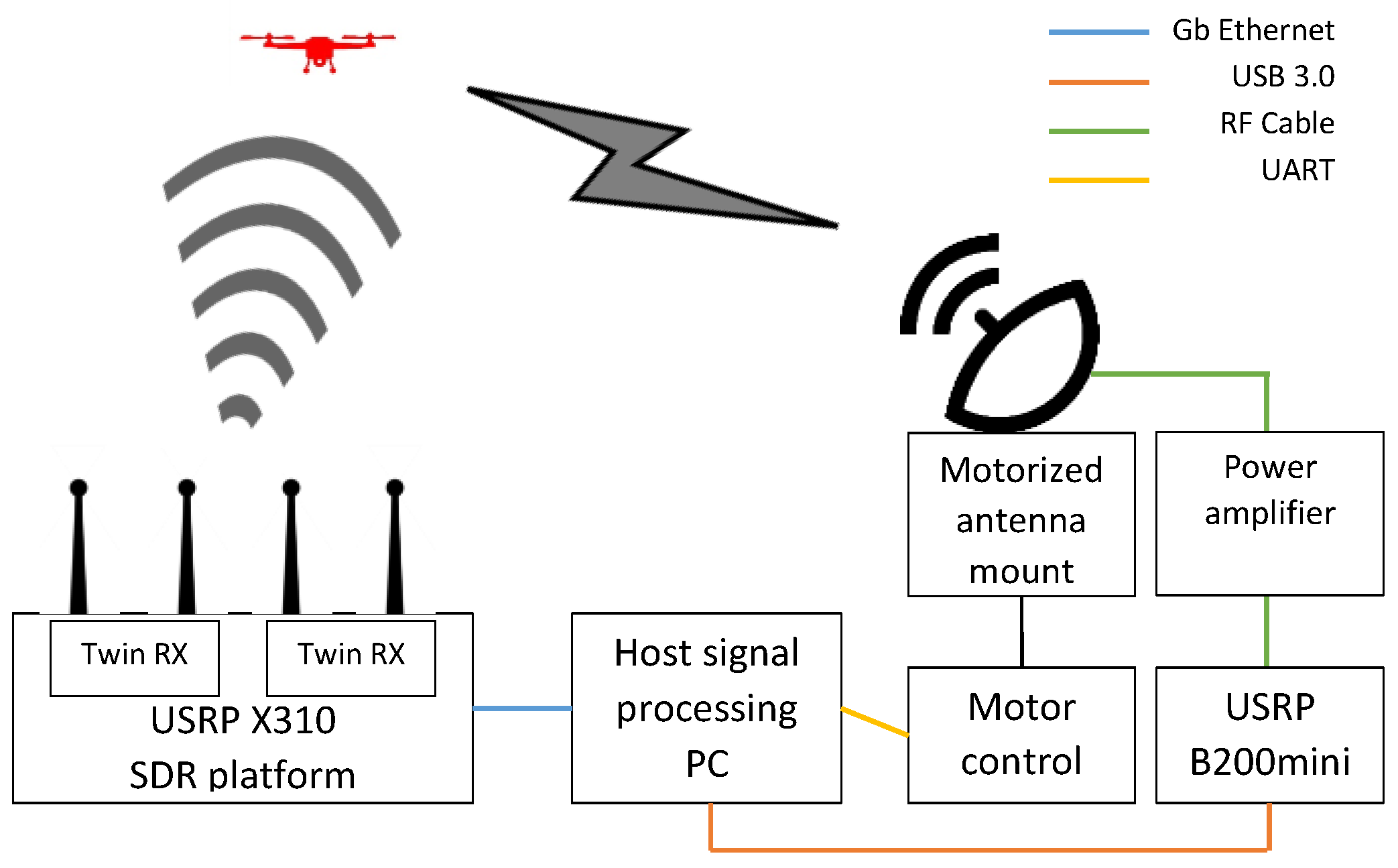
6.1. Detecting the Presence of the Drone Using Spectrum Sensing Algorithms
A first step required for detecting the presence of a drone in the case of RF-based drone defense systems is to monitor the radio spectrum through a spectrum sensing process in order to identify the signals that are transmitted by the drone. For the implementation of the spectrum sensing process in the DronEnd system, spectrum sensing algorithms based on the energy detection method have been used. Algorithms, such as 3EED
[118] and 3EED with an adaptive threshold
[119], that were previously developed, provide improved performance compared to the classical energy detection (CED)
[120] algorithm and were used to identify the presence of the drones in the monitored area. The above-mentioned algorithms were implemented on SDR platforms from the USRP family (USRP X310 (Ettus Research, Santa Clara, CA, USA)
[121] equipped with Twin-RX RF Daugterboards (Ettus Research, Santa Clara, CA, USA)
[122], 10–6000 MHz frequency range).
6.2. Localization of the Drone Using AoA Algorithms
Once the frequency that is used by the drone to communicate has been identified, a second necessary step is to obtain information about the position of the drone. This step was performed using AoA algorithms for detecting the angle of incidence of the detected RF signal. Such algorithms exploit the phase difference of the signals that are received from the drone using a multi-antenna system. The SDR platform that was used as the hardware for providing the RF receive front-end was the USRP X310
[121], on which two Twin-RX RF modules
[122] were mounted (covered frequency range of 10–6000 MHz, instantaneous bandwidth 80 MHz).
6.3. Annihilation of the Drone Using RF Jamming
A final step is to transmit a jamming signal to the identified target drone in order to disrupt the communication between the drone and its operator. As the jamming signal should only be transmitted in the direction of the target drone, in order to avoid interference with other equipment in the area, a directional antenna was used for the jamming operation.
6.4. Conclusion
To conclude, the main novel elements that are introduced by the DronEnd system, when compared to other drone detection and defense systems based on RF methods, can be summarized as follows:
-
Incorporates all of the three functions (identification, localization, and annihilation) that are necessary for a drone detection and defense system in an integrated and scalable platform, which can be reconfigured depending on the requirements of different use cases;
-
Includes an agile and accurate identification subsystem, based on improved spectrum sensing algorithms, which performs a real-time identification of the signals that are transmitted by the drone and, moreover, allows a dynamic tracking of the signal transmitted by the drone, even when the transmit frequency is changed;
-
Annihilates the detected drone by means of jamming, avoiding at the same time significant interference with nearby devices, as a directional antenna, targeted directly towards the target drone using a motorized antenna mount, is used.