1. Biosurveillance and One Health
The general components of a biosurveillance system are described by several publications which provided details on the process of data sources, data integration, analysis, and response
[1][2][3]. However, further detailed structures that provide a comprehensive illustration of the concepts of biosurveillance are few. Wagner
[1] illustrated the systematic and process-oriented nature of a biosurveillance system. Both Wagner
[1] and Kim and Tak
[3] provided illustrations of a biosurveillance process, which generally comprise collection and analyses of data from multiple sources on threats related to human, animal or plant health, with the goal of decision-making and response to the identified threat. However, the biosurveillance process illustrated by Wagner
[1] only described the different components in the process, but the illustration by Kim and Tak
[3] went further to describe multiple agencies and entities involved in the process.
The concept of One Health features prominently in the definition of biosurveillance. One Health refers to an integrated and unifying approach that aims to optimize and balance the health of people, animals, and the ecosystem in a sustainable way. The health of humans, animals, plants, and the environment are closely linked and interdependent. Hence, collaboration is required to develop holistic solutions and utilize the full spectrum of disease control to contribute to global health
[4]. Approximately 60% of pathogens causing human disease and 75% of emerging human pathogens originate directly or indirectly from animals. Furthermore, diseases in livestock pose a threat to food sustainability and the livelihoods of producers
[5].
Figure 1. Components of a biosurveillance system under a One Health concept, showing the complementary relationships between components and One Health Sectors.
2. Animal Health Surveillance
The processes and objectives of animal health surveillance are a fully aligned subset of biosurveillance. Both biosurveillance and animal health surveillance definitions describe data collection, analyses, and action. However, the scope of the data in animal health surveillance is limited to animal health-related data, but the scope of data in biosurveillance encompasses human, animal, plant, and environmental health.
Animal health surveillance is well described as a system where data are collected, analyzed and direct some form of action in response to the animal health event
[6]. For example, in the United States bovine tuberculosis surveillance program, inspection occurs at slaughterhouses to identify compatible lesions on bovine carcasses which can then be confirmed via laboratory tests. Infection-confirmed carcasses are traced back the herd of origin and affected herds are tested so that infected animals can be removed to eradicate bovine tuberculosis.
The four main surveillance objectives determine the type of data required to be collected. If a pathogen or agent is known to be present in the country, the first objective of surveillance can be to measure the frequency of disease to provide information for the design or evaluation of disease control measures. The second objective can be related to detect cases of infection or disease to facilitate disease eradication measures. If a pathogen is absent from a country, the third objective of surveillance can be to demonstrate freedom from disease to be used as evidence for movement and market access for animal products, and the fourth objective can be early detection of a disease incursion so that measures can be taken to contain and eradicate the disease before it spreads. These four surveillance objectives and related actions align with the analysis and action components of biosurveillance, respectively. For more detailed discussions on the design of animal health surveillance systems, readers are directed to existing literature
[6][7].
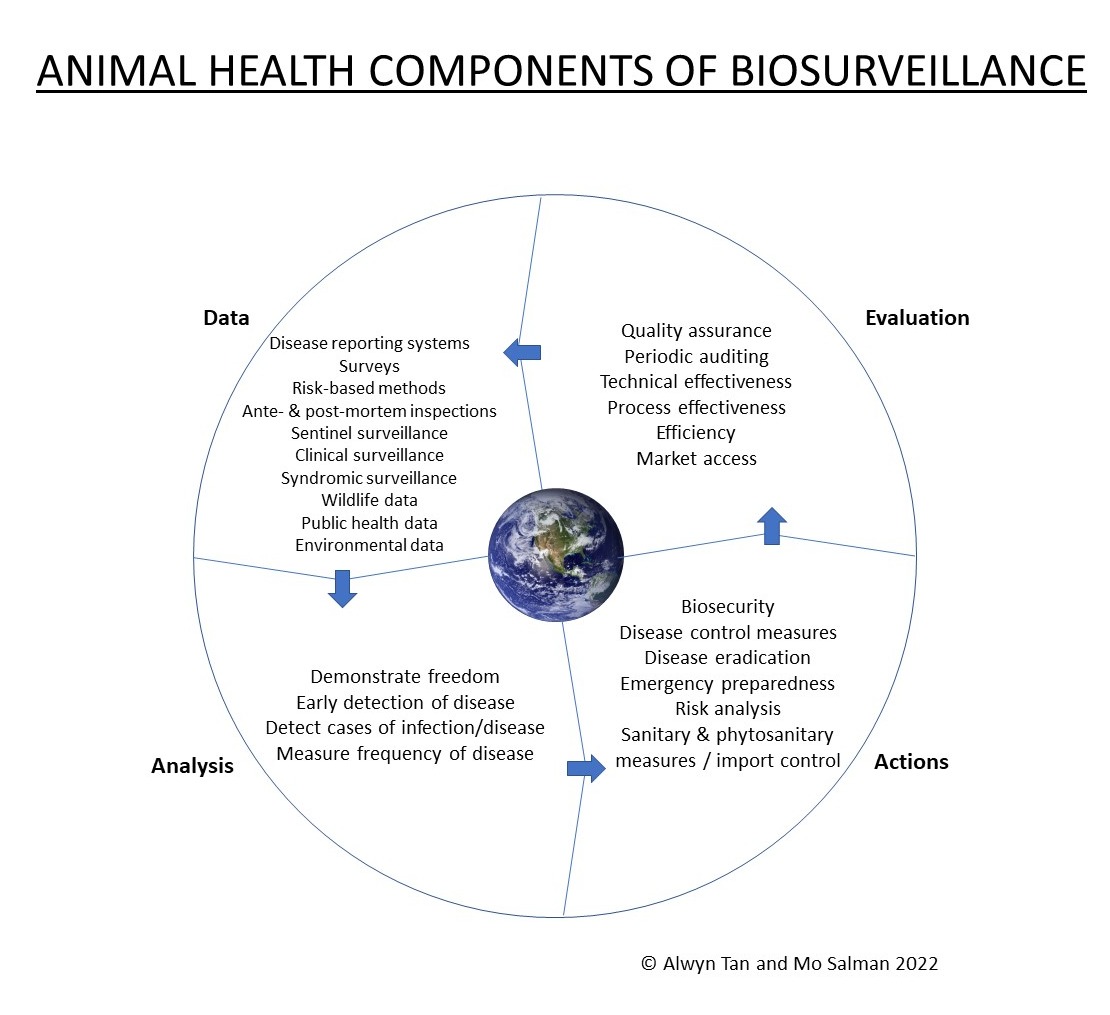
Figure 2. Animal health surveillance activities illustrated under a biosurveillance framework.
One Health interdependencies, important for the success of animal health surveillance within a biosurveillance framework, can be demonstrated in several examples of One Health collaboration on data collection, analysis, and actions. Firstly, antimicrobial resistance (AMR) poses a threat to the effective treatment of diseases in humans, animals and plants and requires a collaborative effort by all One Health sectors to contribute to the surveillance of AMR. The quadripartite One Health Joint Plan of Action
[8] and Strategic Framework for Collaboration on Antimicrobial Resistance
[9] aim to provide guidance and support for nations to implement actions across sectors to preserve antimicrobial efficacy and equitable access to antimicrobials. The guidance includes developing surveillance on AMR and antimicrobial use and developing best practices on the prudent use of antimicrobials. Secondly, the United States National Biodefense Strategy and Implementation Plan
[10] has the goal of countering biological threats, enhancing pandemic preparedness, and achieving global health security. The plan reflected on the limitations in biodefense capabilities with respect to the COVID-19 pandemic and emphasized the need for a One Health approach and multisectoral cooperation in the areas of communication, surveillance, preparedness, and response to biological incidents. Thirdly, although the COVID-19 pandemic is caused largely by human-to-human transmission, there has been an important One Health approach to surveillance for severe acute respiratory syndrome coronavirus 2 (SARS-CoV-2) infection in animals as animal reservoirs are a risk for emergence of new variants, zoonotic transmission to humans, and may affect the health and ecology of animal populations. During the early stages of the pandemic, research determined that multiple animal species, including cats, ferrets, hamsters, bats, non-human primates, and mink, were susceptible to SARS-CoV-2 and the WOAH recommended monitoring of infections in animals
[11][12]. More recently, a joint statement by the Food and Agricultural Organization of the United Nations (FAO), WOAH and World Health Organization (WHO) recommended that competent authorities prioritized the monitoring of SARS-CoV-2 infection in wildlife and prevent the formation of animal reservoirs
[13]. Fourthly, the Zero by 30 (global strategic plan to end human deaths from dog-mediated rabies by 2030) demonstrates a One Health collaboration to reduce human deaths from a zoonotic disease
[14]. While efforts are being made to increase the availability of vaccines and post-exposure prophylaxis to prevent infection and disease in humans, concurrent mass vaccination of dogs in rabies endemic countries is required to break the cycle of disease transmission. When successful, this One Health collaboration will improve the health of both humans and animals in the ecosystem. Fifthly, the United States Department of Health and Human Services established the Administration for Strategic Preparedness and Response to strengthen public health infrastructure and capabilities to coordinate a national response to disasters and emergencies
[15]. Despite the organization’s strong focus on medical resources, they have also included resources on the management of animals during disasters. This One Health approach of disaster management promotes the safety and recovery of families and their pets. Lastly, One Health collaboration was critical in detecting and controlling the largest Q fever outbreak affecting approximately 4000 people in the Netherlands between 2007–2010. Public health authorities, animal health authorities, and farmers worked together to identify goat farms as the major source of infection, leading to interventions such as improving hygiene practices on farms and vaccinating goats which eventually controlled the outbreak
[16].
3. Policy
The link between the science of biosurveillance and public policy is important to ensure alignment of national objectives, adequate attention, and available resources. Public policy is the sum of government activities, pursued directly or through agents, that have an influence on the lives of citizens
[17]. In general, public policies are made in response to issues that require attention, decisions are made by governments on behalf of the public to take action or not to take action, and policies are implemented by the government or other public or private stakeholders to address the issue
[18]. At any time, there are numerous agendas or issues competing for the attention of decision-makers and limited resources. This competition for attention means that the community involved in biosurveillance, that has direct benefits for public and animal health, must constantly demonstrate their importance to governments and citizens, and deliver positive impact on the lives of citizens.
Different countries may implement biosurveillance based on various policy priorities. For example, the United States National Strategy for Biosurveillance frames biosurveillance as a solution for national security
[19], whereas the Australian Department of Agriculture, Fisheries and Forestry frames biosurveillance as a means to protect and develop a very important agriculture industry
[20].
One example of policy driving other components of biosurveillance is demonstrated by the development and adoption of a geographical bovine spongiform encephalopathy (BSE) risk assessment method by the European Union in 1999
[21]. When BSE was confirmed as a zoonotic disease in 1996, countries established trade barriers to livestock and other animal-related products. Determining which countries to trade with was complicated by the uncertainty of the epidemiology of the disease. The main challenge with BSE was the long incubation period in cattle (average of 5 years) and difficulty in detecting the disease antemortem. Hence, it was difficult to determine the risk of BSE introduction from imports based on self-reporting of BSE cases by countries. With the objective of balancing trade with the need to protect human and animal health, the European Commission established the Scientific Steering Committee, which in turn established a Transmissible Spongiform Encephalopathies (TSE) ad hoc working group that comprised expert stakeholders from academia and government. The working group developed the geographical BSE risk assessment method that determined the likelihood of BSE infection presence in a country based on information on imports and the cattle farming system. Countries that participated in the risk assessment were classified on four levels of risk, ranging from highly unlikely to confirmed. A beneficial output of this risk assessment was that several countries that had not reported cases but were classified as BSE “cannot be excluded”, intensified their surveillance and eventually confirmed cases, allowing them to take necessary disease control measures. Additionally, conditions for import to reduce the risk of BSE could be applied to facilitate trade with countries that did not report cases but did not have negligible risk. The success in implementing this policy was evident in improved surveillance for BSE and reduction in the spread of BSE. This risk assessment was also the framework for development of the WOAH recommended international standard for BSE risk assessment in the Terrestrial Animal Health Code.
Hence, with an understanding of how biosurveillance and animal health surveillance fit within changing national agendas, the animal health community will better align their activities to contribute to the benefit of citizens and guide the framing of advocacy in areas that require attention and resources.
4. Stakeholders
The range of stakeholders that participate in biosurveillance is broad, ranging from government authorities, to businesses, to the general public, and these stakeholders may come from one or multiple sectors in the One Health community.
A large proportion of biosurveillance work is typically carried out by the government or state agencies and laboratories involved in collecting biological samples for disease testing. Other stakeholders play important roles in biosurveillance, such as private physicians and veterinarians who provide services to treat and manage the health of their human and animal patients. Since they are first to diagnose diseases in individuals, they are also responsible for reporting confirmed or suspected notifiable diseases to the relevant authorities. Most medical records are now stored on digital information systems, and there is wide potential for the use of health data for national biosurveillance, although there may be barriers to the aggregation of data across institutions. Wagner and Hogan
[22] described the potential use and system challenges accessing human healthcare records for biosurveillance. Similar challenges exist in the veterinary sector, but one recent breakthrough is the development of the Veterinary Companion Animal Surveillance System (VetCompass) that collects anonymized records from the database of participating veterinary clinics and hospitals in the United Kingdom
[23][24]. The data on VetCompass has been used for veterinary research and there is potential for it to be used for biosurveillance, such as a syndromic surveillance data stream.
In addition, stakeholders from different communities likely will have inherently different technical abilities, political interests, social and cultural values, and objectives. Hence it is important that the participants in biosurveillance need to be able to identify with the objectives and benefits of biosurveillance to ensure their continued participation and success of the system. Participatory epidemiology encourages the involvement of animal owners who have localized knowledge and experience on animal disease as well as social and cultural context
[25]. Participants should be engaged in the planning, design, and implementation of the system. For example, Bordier, et al.
[26] described a participatory approach to engaging stakeholders in designing One Health surveillance systems. The framework was utilized in the development of AMR and Salmonella surveillance programs in two countries, allowing diverse stakeholders to gain mutual understanding and expectations of the surveillance activities required. Additional examples of how participatory epidemiology was utilized to increase engagement of stakeholders in surveillance activities are presented in Alders, et al.
[25].
The Swine Health Information Center (SHIC) and the Swine Health Monitoring Project (SHMP) are examples of different stakeholders in the swine industry working together for a common goal
[27][28]. The SHMP started in 2011 as a collaboration between the United States pork industry, academic institutions, and government agencies, while the SHIC was created in 2015 to support the project. These stakeholders have come together to monitor the health of swine herds in the United States and respond to disease threats in a timely manner. This collaborative multi-stakeholder and multi-disciplinary approach to animal health surveillance brings together the expertise and resources of different stakeholders to address the disease challenges facing the swine industry. In another example, several networks such as the Extension Disaster Education Network (EDEN), National Plant Diagnostic Network (NPDN), and National Animal Health Laboratory Network (NAHLN) comprising government agencies, academic institutions, industry organizations, and diagnostic laboratories, exist to raise awareness and facilitate diagnoses of emerging infectious diseases in animals and plants. This collaboration enhances early detection and rapid response efforts to protect animal health, plant health, and the community
[29][30][31].
Stakeholder participation in biosurveillance can be strengthened through social science and behavioral methods Biosurveillance activities require the cooperation of stakeholders to participate in supporting resource allocation, providing data, and performing actions to control or reduce the risk of disease. Human behavior is not only influenced by scientific information, but depends on a myriad of social, cultural, and political factors.
Although COVID-19 vaccines have been scientifically proven to be effective at reducing COVID-19 infection, public confidence and acceptance of the vaccine was low in some segments of society. Evidence-based vaccination programs meant to protect citizens against COVID-19 disease were insufficient to overcome misinformation or distrust of some experts
[32]. According to the World Health Organization and United Nations Children’s Fund
[33], the behavioral and social drivers that affect vaccine uptake are what people think and feel about vaccines, social processes that drive or inhibit vaccination, individual motivation or hesitancy, and practical factors in seeking and receiving vaccination. Social and behavioral sciences are commonly used to study public health barriers and design interventions to improve health. Piltch-Loeb and DiClemente
[34] describe studying population characteristics of social class, culture, ethnicity, individual beliefs, attitudes and behaviors, and cultural and socio-political systems that can affect public health threats and solutions in the case of vaccine hesitancy.
In animal health surveillance, similar social and behavioral factors affect the success of biosurveillance goals. Firstly, a study of knowledge and attitudes of Australian livestock producers on biosecurity practices found that improving producers’ knowledge on biosecurity methods may increase their willingness to implement biosecurity practices, but the lack of communication from agricultural, veterinary or government organizations may be barriers to biosecurity practices
[35]. Secondly, disease reporting is an important data stream for early detection of disease in livestock, but farmers are believed to be underreporting diseases. Studies found that barriers to disease reporting by farmers include uncertainty about clinical signs of diseases, fear of social and economic consequences, negative beliefs on response measures, mistrust of animal health authorities, lack of incentives, and unawareness of reporting procedures
[36]. Hence, solutions to these behavioral barriers should adopt theories and methods from social sciences and behavioral methods to address the different identities, motivations and beliefs of stakeholders.
5. Data Streams
Data streams for biosurveillance can be classified as traditional or non-traditional sources, and are based on the objectives of biosurveillance
[37]. Velikina, et al.
[38] described potential traditional data streams to include notifiable disease reports by physicians and veterinarians, laboratory test results confirming disease, hospital records, livestock farming records, water supply testing results, and food and pharmaceutical industry records. Non-traditional and newer types of surveillance data include sales of over-the-counter medicines, chief complaint of patients seeking medical care, absenteeism rates at the workplace or schools, internet activity and remote sensors monitoring physiological conditions of patients, animals, or environmental conditions. These non-traditional sources may also be called pre-diagnostic information or syndromic data, as they do not directly measure cases of specific diseases and can contain an outbreak signal earlier than traditional data sources
[39]. Further types of data that may be utilized for biosurveillance include data associated with disease risk factors such as air and water quality measurements
[39].
Earlier methods of evaluating biosurveillance data were primarily based on ability of data to contribute to early detection of outbreaks, data availability, and cost of acquiring the data
[40]. However, biosurveillance has broader goals that include early warning of threats which precede any outbreak. Hence, disease reporting systems and laboratory test results, whilst being highly effective for the purpose of early disease detection or situational awareness, are not able to achieve the goal of early threat warning. To achieve the full spectrum of goals for biosurveillance, data streams from syndromic surveillance and environmental surveillance are required from across the One Health sectors
[37].
Margevicius, et al.
[37] described a framework for the evaluation of biosurveillance data streams based on the disease of interest, population, type of data (diagnostic, syndromic or environmental), categories (e.g., laboratory records, social media, sales) and whether they can achieve biosurveillance goals. The requirements to elevate a biosurveillance system from providing situational awareness to early warning is further explained by Velsko and Bates
[41] who described that in addition to traditional data sources such as disease reporting, syndromic surveillance data must be an automated component of a biosurveillance system.
One interesting environmental data stream for biosurveillance that is receiving renewed attention is the use of air samples to monitor for specific microorganisms and chemicals. The surveillance of air for biological threats is not new. For example, the BioWatch program by the United States Department of Homeland Security deploys air sampling devices in major cities to test for biological agents such as
Bacillus anthracis,
Yersinia pestis, and the smallpox virus
[42][43]. Air-sampling surveys of zoonotic poultry diseases have also been conducted, where exotic Newcastle disease and highly pathogenic avian influenza were detected in air samples collected at infected poultry facilities
[44][45]. However, there are gaps in the understanding of how bioaerosols are emitted, dispersed and deposited in the outdoor atmosphere, and ongoing research may provide more context for air surveillance to be used as a data stream in biosurveillance
[46][47][48][49]. For example, a study by Champion, et al.
[50] found that the disposal of foot and mouth disease (FMD) infected cattle carcasses by burning was unlikely a risk of airborne spread of FMD virus to other farms. However, a more recent environmental study by Moore, et al.
[51] found that wildland fires emitted high concentrations of microbes to the atmosphere, and the burning of dead vegetation contained more microbes than fresh vegetation.
6. Data Stream Integration, Processing, Analysis, and Access by Decision Makers
The collection of data for animal health surveillance is followed by prescribed and directed actions in response to exceeding a pre-determined threshold or the detection of an animal health event. Additionally, the numerous and varied data streams mentioned in the previous section must be transformed into information that can be converted into knowledge for decision makers. Dórea and Revie
[52] suggested that implementing data driven surveillance frameworks is a three-step process including data integration, data processing to generate information, and data analysis creating accessible outputs for decision-makers.
Data integration is challenging due to the volume and variability of data that are almost ubiquitously captured in non-standardized formats
[53]. Efforts at standardizing the data elements collected by syndromic surveillance systems, laboratory information management systems and national herd and animal identification systems have been almost universally unsuccessful
[54]. One notable success is the creation in the U.S. of a standard for electronic certificate of veterinary inspection data that is now transmitted between traceability systems facilitating more rapid and accurate disease tracing efforts
[55]. Without standardized data, extensive efforts to cleanse and transform data are required to facilitate analysis. Estberg, et al.
[56] reported on enhancing surveillance through improved processing and integration of data. Automation of omitting and flagging duplicate reports or reports with errors along with an integrated data pipeline that automatically combines results from disparate data streams into a single dataset have significantly impacted the accuracy of reporting on equine infectious anemia findings in the U.S. Leading edge software developments have revolutionized how data can be consumed, integrated, and used to inform decision-makers. With appropriate software, “organizations can clean, harmonize, and de-duplicate disparate data from across systems into a central, usable data layer for a single “source of truth” to improve care for population segments”
[57].
Leveraging biosurveillance streams for early threat detection requires innovative analytical and visualization methods. Syndromic surveillance research has focused on algorithms capable of detecting disease outbreak signals
[58]. Shewhart control charts, exponentially weighted moving averages (EWMA) control charts and Holt-Winters exponential smoothing have been investigated for their value in improving early detection of events from syndromic surveillance streams. A freely available R package, Vetsyn, includes the aforementioned algorithms and ability to perform retrospective analyses of syndromic surveillance data
[59]. This package aids epidemiologists and others responsible for implementing syndromic surveillance in leveraging data for early warnings. Odoi, et al.
[60] developed an automated early warning system that used the prospective space-time permutations scan statistic. The system was tested against data on equine abortions and found that abortion outbreaks could be detected one week earlier than through traditional surveillance systems.
The outputs from these analyses need to be presented to decision makers quickly and in visual formats that are easily accessible. Many government entities responsible for animal health surveillance have moved away from written analysis reports of surveillance activities to disease dashboards or other near real-time visualizations. An excellent example of the dashboard approach to result reporting is the United State Department of Agricultures (USDA) dashboard on detections of highly pathogenic avian influenza in wild birds
[61]. This specific USDA dashboard is available to the public but other dashboards are restricted to use by regulatory decision makers (e.g., African swine fever and classical swine fever surveillance for field operations).
7. Surveillance Evaluation
The ideal biosurveillance system is effective at achieving goals, efficient in the use of resources, and sustainable with time and emerging challenges. However, it is unlikely that such an ideal system exists based on the discussions in previous sections. Firstly, policymakers are making decisions and prioritizing between competing issues, with finite resources. Hence, biosurveillance programs may not be allocated the desired resources while remaining accountable to policymakers for the promised outcomes or goals. Secondly, there are many different stakeholders involved in providing data and performing actions in biosurveillance, and each stakeholder may bear different individual technical abilities, political interests, social and cultural values, which may affect the performance of a biosurveillance system. Thirdly, the myriad of traditional and non-traditional data streams in biosurveillance must not only contribute to achieving the biosurveillance goal, but also be available, practical, and not cost prohibitive.
Biosurveillance systems are vulnerable to external challenges. For example, the performance of a biosurveillance system that relies heavily on human resources may be severely impacted due external factors such as occurred in the “Great Resignation” in 2021
[62]. Also, components of biosurveillance that involve laboratory testing may be adversely affected if laboratory equipment supply chains are disrupted, which happened during the COVID-19 pandemic when laboratories faced shortages of equipment required to carry out diagnostic tests for COVID-19 itself and other disease surveillance
[63].
Biosurveillance evaluation can be used to overcome the sometimes unseen challenges of biosurveillance described above. Evaluation determines the merits of a surveillance system to achieve its goals, through a transparent, objective and evidence-based process, and provides recommendations on the system
[64].
The WHO has developed the Joint External Evaluation (JEE) tool to evaluate the public health capacities; and WOAH has developed the Performance of Veterinary Service (PVS) tool to evaluate the capacities of veterinary services respectively
[65][66]. These evaluation tools can be used internally (i.e., self-evaluation) or by an external independent expert evaluation team to evaluate the overall public health and veterinary service capacity of a country in accordance with international standards. Both the JEE and PVS include the evaluation of surveillance systems for diseases of importance to public and animal health and a higher capacity is recognized when countries perform regular evaluation on the performance of their surveillance systems.
Drewe, et al.
[67] however, found that there were no standardized and consistent methods of evaluating human and animal health surveillance systems and comprehensive evaluations were uncommon. The U.S. Centers for Disease Control and Prevention (CDC) Guidelines for Evaluating Public Health Surveillance Systems was often used to evaluate biosurveillance systems
[37][68][69]. However, there may be limitations when evaluation methods developed for public health surveillance are applied to animal health or biosurveillance. Firstly, the evaluation of acceptability of the surveillance system differs between human and animal health. Acceptability is defined as the willingness of a person or organization to participate in the surveillance system
[68][70]. In the public health surveillance context, acceptability focusses on the willingness of stakeholders to report data, whereas in the animal health surveillance context, it focusses on both the willingness of stakeholders to report data as well as their beliefs on actions that may be taken such as the movement restrictions, culling, and compensation of diseased animals. Secondly, the evaluation of resource efficiency in human health surveillance focusses on cost-effectiveness, but in animal health surveillance, there is the option for cost-benefit analysis. This option arises because saving a human life is universally accepted and there are no alternatives while the value of animals can be determined. Hence, a utilitarian approach is adopted when considering animal health as there are viable alternative benefits for society on the use of resources. For example, the cost and benefit to society can be compared between improving livestock production through animal health measures or improving transportation by building roads.
Recently, Peyre, et al.
[71] prescribed a comprehensive surveillance evaluation tool (EVA Survtool) that is applicable to both animal and human health surveillance, and, hence, biosurveillance. This tool allows the integrated evaluation of multiple attributes related to the effectiveness, efficiency, and sustainability of biosurveillance. In a case study, the EVA Survtool was used to evaluate a swine disease surveillance system in Vietnam
[71]. The study found that pig farmers had higher acceptability if selective culling was utilized instead of 100% culling of infected pigs. The timeliness of reporting by farmers and sensitivity of detecting diseased pigs increased when a higher compensation was paid for culled pigs. Although combining selective culling and high compensation was technically most effective at detecting and controlling pig disease, a cost-benefit analysis revealed that selective culling with a moderate compensation gave the highest benefit-cost ratio. This evaluation identified improvements to the surveillance system for pig diseases that would be acceptable technically and optimize the use of resources.