1. Introduction
The focus is on the different steps that led to the use of hyperspectral imaging in the geosciences, the different fields involved in these developments, and their exploitation (Figure 1).
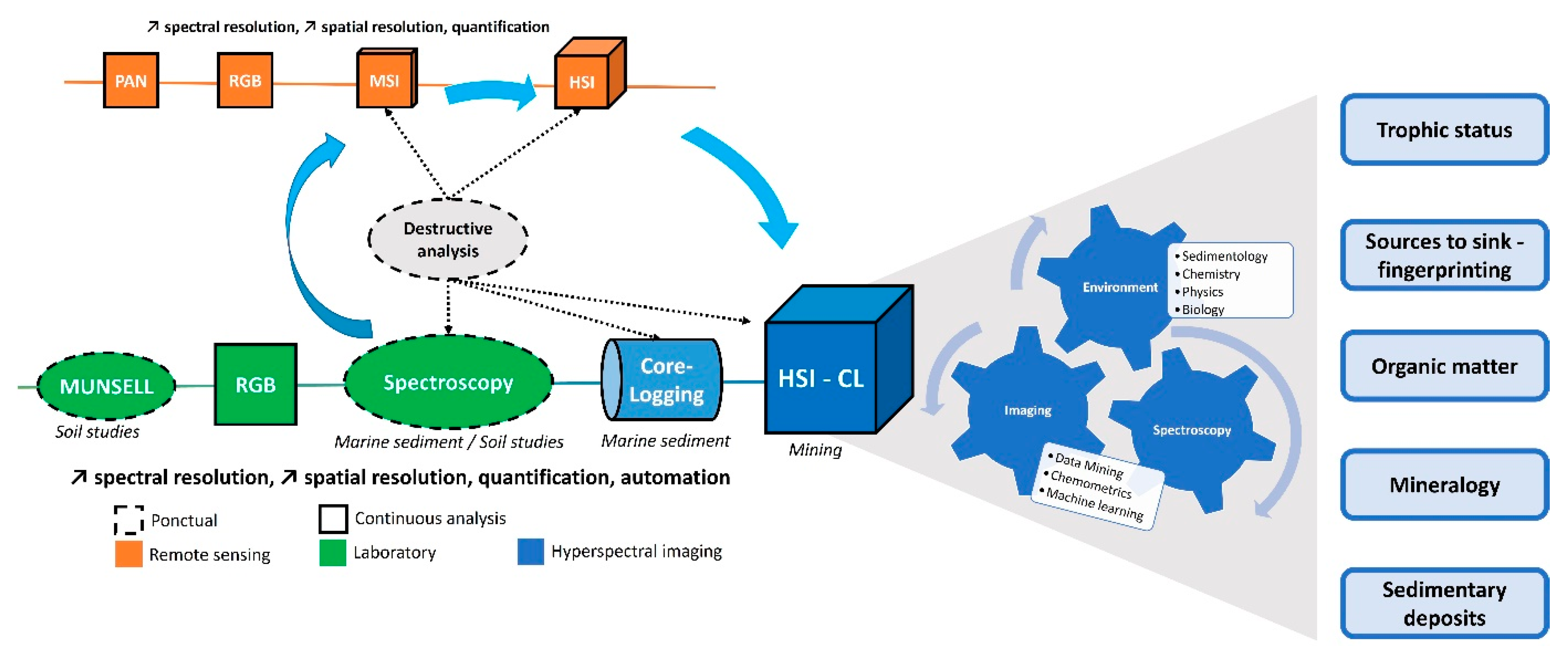
Figure 1. Schematic representation of the different techniques and domains involved in the development and exploitation of hyperspectral data for sediment core analysis (PAN: Panchromatic, MSI: MultiSpectral Image, HSI: HyperSpectral Image, CL: Core Logging) and some potential topics.
2. Munsell Lithology Description
Color and texture are two simple and important parameters to describe sediment facies. However, they are qualitative, and they lack rules to compare several sequences between them. This is why the soil community has adopted the work of Albert Henry Munsell on color classification
[1] to standardize the characterization of sample colors. This approach was then adapted for use onboard Ocean Drilling Program (ODP) coring ships such as the JOIDES Resolution and the Marion Dufresne
[2][3]. Then, it was democratized in various sedimentological studies.
The Munsell color chart is based on the vision with characterization of sediment color thanks to the definition of three parameters: hue (the color), value (its intensity), and chroma (its brightness). It is also the basis of some color spaces for digital object vision (Lab)
[4].
However, this method has limitations, as it is dependent on the experimenter’s vision and the lighting conditions, and the procedure can be time-consuming. It provides information on the sediment color at a given time to reveal development of structures, which change with the oxidation process as well as moisture content
[5].
3. RGB (Red, Green, Blue) Imaging
To overcome the subjective nature of visual observations, it is necessary to use reproducible and quantitative approaches. This is why the use of sensors recording colors has received great interest to archive the primary information of the sample (e.g., color and texture) before its destruction. The first imaging sensors are used to save the information in grayscale
[6][7][8][9] or X-ray
[10], but the interpretation of these levels is complex, as many mixtures of compounds may be involved. This is why RGB (red, green, blue) imaging is currently used because it provides information on three types of colors
[11][12].
Image sensors allow a resolution finer than the human eye and thus characterize finer variations. Moreover, the use of image processing can be applied to sediment cores to characterize different deposits, which allows us to complement the experimenter’s vision
[11][13][14][15][16][17]. These images also allow to observe temporal transformations on sample surfaces and to incorporate laboratory results so that better constraints of stratigraphic positions for the analyzed data points can be obtained. Even if their use is widespread, these images are rarely processed to extract the maximum amount of information (deposits, particle size distribution and s on), and these three channels as well as the grayscale levels do not allow a precise characterization of compounds within sediment.
4. Spectroscopic Analysis
RGB imaging, similar to the eyes, is sensitive to three wavelength zones (610 nm, 550 nm, and 466 nm), while the entire electromagnetic spectrum (
Figure 2) provides more information on the sample properties. The development of spectroscopic sensors such as spectrophotometry has allowed more accurate quantification of color through the different wavelengths they record (400–700 nm) directly. Moreover, their simplicity of use, speed, and cost have allowed their democratization. These sensors were used routinely during oceanic campaigns
[6][18][19][20][21] and then for the characterization of rocks
[22] before their use in the various disciplines of sedimentology
[23][24][25]. Spectrophotometric spectra are now commonly used to estimate the total reflectance L* to correlate cores, some minerals and organic pigments
[20][21][22][26][27][28][29]. The improvement of the detectors has allowed to extend the spectral range to cover visible and near-infrared wavelengths (400–2500 nm), which allows to obtain information on pigments and organic and mineral matter
[30][31][32].
Figure 2. Electromagnetic spectrum and names of the main corresponding spectroscopic and hyperspectral techniques (VNIR: Visible and Near-Infrared, SWIR: Short Wave-Infrared, MWIR: Mid-Wave-Infrared, LWIR: Long-Wave-Infrared)
Other sensors have also been developed to characterize other spectral ranges that allow to characterize more sedimentary properties (
Figure 2). XRF core logging is currently a very often used routine method, as it focuses on elemental analysis within the sample, which then provides an idea of the geochemistry and related sedimentation processes
[33][34][35]. Other spectral ranges often require sampling and therefore the destruction of the sediment core to extract compounds of interest with solvents or to obtain a sample in a different aspect (thin layer, pellet and so on). For instance, X-ray diffraction and Raman spectroscopy allow sample mineralogy
[36][37]. UV–visible fluorescence allows the characterization of fluorescent molecules such as organic compounds (PAHs, pigments, humic matter)
[38][39][40]. Mid-infrared spectroscopy allows organic and mineral materials
[41][42][43]. Some solutions in these areas are beginning to emerge for direct use on the sediment core. Each of these spectral ranges provides information that is different and complimentary to the other ranges, which is why sample analysis is very often performed with multiple sensors
[44][45].
However, even though these methods are nondestructive, fast, easy to use, and very informative, they are discrete and not very spatially resolved, which does not allow for accurate characterization of the past environment and climate at a fine temporal scale
[25][46][47].
5. Hyperspectral Imaging
The successive developments of these approaches have improved the relevance and robustness of sample color characterization.
This sensor made its debut in airborne remote sensing in the 1990s and then with satellites and laboratories in the geosciences in the 2000s. This is the result from the development of remote sensing sensors based on spectroscopy allowing an increase of the channel numbers: panchromatic (grayscale level, 1 channel), then RGB imaging (3 channels), then multispectral (<100 channels). HSI can acquire data over several hundred contiguous wavelengths. These advantages are why it is increasingly popular in many scientific domains.
The data acquired by an HSI sensor are called a data cube because of the two spatial dimensions and the spectral dimension (
Figure 3). The spectral dimension (z axis) exhibits the physical, chemical, and biological properties of the sample and the spatial dimensions (x and y axes) display their variations as a function of depth and time. The variations at any given time are therefore recorded through the lateral pixel as an individual slide. In short, HSI benefits from both imaging and spectroscopy domains to extract information of interest
[48].
Figure 3. Hyperspectral cube composed of two spatial dimensions (x along and y across the sample, which can also be viewed as time and cross-time dimensions) and one spectral dimension (z as wavelengths, wavenumbers, or energies). Each pixel contains a spectrum, and wavelength assemblage can create a composite image.
5.1. Hyperspectral Sensors
There are three ways to perform hyperspectral image acquisition. The first is the “whiskbroom scan”, which collects the spectrum one pixel at a time and has the highest spatial and spectral resolution but also the lowest speed. Then, there is a linear scan called “push-broom scanning”, which has a higher speed but a lower spectral resolution and suffers from a one-dimensional spatial limitation due to the detector (only a fixed number of pixels per line). The last is planar scanning, which has a high scanning speed but a low spectral resolution and suffers from a two-dimensional spatial limitation (only fixed numbers of pixels per row and column).
With high spatial resolution on the order of hundreds or fewer micrometers, high spectral resolution with hundreds or thousands of available wavelengths, and a consistent scan rate of minutes to hours point and line scanning are the most appropriate methods for the analysis of natural archives. Thus, the HSI can be obtained over most spectral ranges.
In the following, focus will be on push-broom sensors, frequently called core loggers, that are used on analysis benches to cover the ranges between the visible and mid-infrared wavelengths. Four main types of hyperspectral cameras from different manufacturers are available on the hyperspectral core-logging market. The visible and near-infrared (VNIR) camera covers the range of 400–1000 nm, allowing the detection of photosynthetic pigments (chlorophylls) and bacterial pigments (bacteriochlorophylls)
[49][50][51][52][53], iron oxides, and altered organic matter
[54]. The short wave-infrared (SWIR) sensor, which acquires a spectrum between 1000–1700 nm or 1000–2500 nm, allows organic compounds (aromatic, aliphatic)
[55][56][57] and types of deposits (clays, carbonates)
[58][59]. The mid-wave-infrared camera (MWIR) operating between 3000 nm and 5000 nm allows organic and mineral properties. Finally, the long wave infrared (LWIR), also called a thermal infrared (TIR), camera operating between 8000 nm and 12,000 nm allows the characterization of minerals
[60][61]. The choice between these different sensors is made according to the focus, as they provide different and complementary information, according to the constraints of these spectral domains and their characteristics (cost, speed, spatial and spectral resolution).
5.2. Acquisitions and Recommendations
Two main protocols have been published for HSI acquisition of sediment cores and they can be used for any other natural sample. The protocol of Butz et al.
[62] presents the main steps to obtain an HSI. Jacq et al.
[63] advanced from the first protocol and took a step further to thoroughly describe the different acquisition parameters and their influence on the image.
These two protocols help define the conditions necessary for the analysis of a sample. It must be as flat as possible to be within the depth of field of the camera (to have a sharp focus in an image) and not create microstructures and illumination differences due to the roughness of the surface
[64]. The positioning of the sample must be parallel to the camera. The depth of field is related to focal length, distance to the sample and pixel size. The latter is often minimized to obtain a precise measurement, which also leads to a limited depth of field, on the order of a few millimeters. This shows the importance of having the sample as flat as possible and well positioned to the camera. The sample must also have all the sediment structures visible to the naked eye, if possible, so it is necessary to clean its surface. To prevent specular reflection caused by moisture on the surface of the sample, it should be left at room temperature for a few minutes to stabilize its moisture content.
The different acquisition parameters (focus, integration time, frame rate, scanning speed) must be optimized according to the issues. They are related and must be defined together to obtain a relevant image with sharp focus and true dimensions.
Once all acquisition parameters are set, a sample can be imaged in three steps. First a black reference is imaged to estimate the instrumental noise (Sblack), then a white reference is imaged to calibrate the signal in percent reflectance (Swhite), and finally the sample (Sraw) is imaged. Normalization is performed for each spectrum (Sraw) by removing the instrumental noise from the black reference (Sblack) and the reflectance is calibrated in percent reflectance based on the white reference (Swhite). Thus, to have a well-parameterized exposure time to avoid saturating the image, Swhite is needed to conduct relevant normalization.
5.3. How to Process These Data?
To identify sedimentary processes recorded by hyperspectral data, four main steps are to be followed, fully or in part, as shown in Figure 4 [65] . (1) The first is to prepare the data by reducing the image to the spatial and spectral areas and to use pre-processing to reduce the noise present in the data. (2) The second allows to observe the first trends in the HSI, both in the spectral dimension with absorptions of some compounds and in the spatial dimensions with sedimentary deposits. (3) Data processing methods will allow to extract these first observations and to highlight other properties of interest with machine learning approaches. (4) The maps extracted by these methods can be spatially postprocessed to reduce the residual noise or to match the observations with other analyses. This last step can help to confirm the observations and to obtain an interpretation of the biophysical-chemical properties recorded by the hyperspectral sensors.
Figure 4. Diagram of the different steps that can be performed to (1) correct the initial HSI (prepro-cessing), (2) visualize the information contained in the image (exploration), (3) extract the information of interest (processing), and (4) apply a final correction (postprocessing) leading to a properly interpreted image.
5.4. What Sedimentary Properties Can Be Derived from It?
Five major themes aim to reveal the various sedimentary properties with the use of HSI and versatile methods. (1) The trophic status of lakes is extensively studied by spectroscopy, and HSI can take advantage of this work by leveraging specific pigment indices. (2) Source-to-sink studies can also be performed using spectral fingerprints (endmembers EMs) associated with sources in the lake catchment. (3) Organic matter is an important sediment property for paleoenvironmental reconstructions or the estimation of anthropogenic pollution and is generally characterized with regression/classification approaches or index definitions. (4) Sediment mineralogy is also an important property used to describe the sources of sediment supply within the watershed, and it can be characterized with indices or regression and classification methods. (5) Sedimentary structures and texture will have spectral and/or spatial fingerprints that can be recorded by HSI and characterized with classification methods. Another recent hyperspectral imaging review by Zander et al. focuses on the biogeochemical analysis of sediment cores and is complementary to the above [66].