1. Introduction
Over the last few decades, the impressive advances in the fields of computer science and imaging technologies have increased the machine/clinician synergy, bringing precision medicine into the current clinical practice [1]. In particular, the constant development of new devices and minimally invasive platforms together with the implementation of advanced imaging technologies has led to an epochal change within surgical disciplines. In fact, while the indications for minimally invasive surgical procedures are progressively extended towards more complex pathologies, traditional diagnostic disciplines such as radiology or endoscopy are developing an increasing portfolio of minimally invasive treatment options. In this context, the intraoperative use of imaging technologies which can augment the human sight are fundamental to increase the accuracy and precision of surgery. The ideal intraoperative imaging modality should be safe and user-friendly to smoothly fit within the operating workflow [2][3]. Additionally, it should provide reproducible and quantitative results without the need for an exogenous labelling agent [4], enriching the surgeon with additional useful information and assistance in the decision-making process.
In this view, hyperspectral imaging (HSI) displays most of the features of the ideal intraoperative imaging technology, as it can provide a qualitative and quantitative snapshot of the biological tissue’s chemical properties in a non-invasive, radiation-free, label-free, and user-friendly manner. HSI is included within the optical imaging domain, and it results from the combination of a digital camera with a spectrometer. The interaction of light with the target object generates specific signatures or fingerprints across the electromagnetic spectrum, which are detected with the spectrometric unit. The resulting dataset is a spatially and spectrally resolved three-dimensional set of information, called a hypercube (spatial coordinates: x, y; spectral coordinate: z). Given its ability to rapidly discriminate and quantify chemical composition over large areas, HSI has been successfully used within the fields of remote sensing [5], food quality control [6], vegetation and water resource control [7], forensic medicine [8], recycling industry [9], analysis/restoration of old paintings or manuscripts [10].
HSI can distinguish the biochemical composition of healthy and pathological biological tissue [11] in a non-invasive fashion, and for this reason, it has been increasingly used in the medical field [12][13]. Previously, other authors have written comprehensive overviews concerning the application of HSI in gastroenterology [14], surgery [15] or surgical clinical studies [16]. However, those previous works have been meant for biomedical engineers/scientists [14][15] and contain a high degree of theoretical and technical details, and they are rather difficult to digest for the average surgeon. Alternatively, overview articles have been written before 2018 [16], and starting from that period, several experimental and clinical works reporting interesting novel intraoperative applications using HSI have been published.
2. Theoretical Overview
This article is written for a clinical audience. As a result, the technical details regarding biophotonics, hardware engineering, data extraction and processing will be only concisely mentioned since they go beyond the purpose of this manuscript. For a more comprehensive technical and theoretical description of the principles behind HSI, we refer the interested reader to previous works [12][13][14][15].
Biophotonics, from the ancient Greek bios (βίος) meaning life, and fos (φῶς) meaning light, is the science studying the interactions between biological tissue and light. Once light enters living tissue, it induces several phenomena (mainly scattering, adsorption, reflection), which strictly depend on the chemical composition of the specific tissue. For this reason, each tissue type presents a characteristic optical pattern, named spectral signature or spectral fingerprint, which can be used to differentiate it from another tissue type. Similarly, the spectral signatures allow to discriminate pathological (e.g., cancerous, ischemic or burned tissue) from healthy tissue [11].
2.1. The Electromagnetic Spectrum and the Hypercube
The human eye only detects a narrow portion of the electromagnetic (EM) spectrum. In fact, human sight can only perceive light in the visible (VIS) range (400–780 nm) (Figure 1A). Light in the VIS range exhibits only minimal tissue penetration (1–2 mm), while in the near-infrared (NIR) range (780–2500 nm) it can pass up to several millimeters through tissue, hence having a higher diagnostic value than VIS [12].
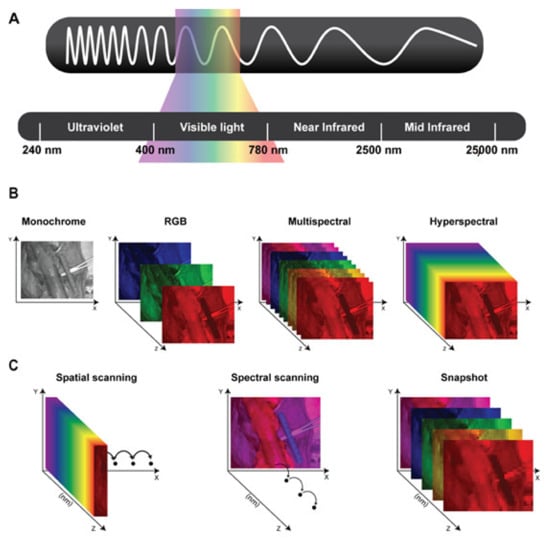
Figure 1. (A) Schematic representation of the electromagnetic spectrum’s wavelengths: Ultraviolet (240–400 nanometers), Visible light (400–780 nanometers), Near-Infrared (780–2500 nanometers, Mid- Infrared (2500–25000 nanometers). (B) Representation of the different datasets generated using: monochrome images; color or RGB (Red, Green, and Blue) images; multispectral and hyperspectral imaging. (C) Schematic representing the 3 different types of hyperspectral imaging devices. Spatial scanning: acquiring the spectral information as a whole and progressively scanning the spatial information. Spectral scanning: acquiring the spatial information as a whole and scanning the spectral one. Snapshot: simultaneously acquiring spectral and spatial information but providing a lower spatial and spectral resolution than previously mentioned hyperspectral imagers.
HSI is a hybrid imaging modality which combines digital photograph and spectrometry, generating a three-dimensional dataset, namely the hypercube. This is composed of a stack of two-dimensional images (spatial coordinates) across a wide and generally contiguous range of the EM spectrum (spectral coordinate), hence augmenting the human vision far beyond its natural capabilities (Figure 1B). HSI is a spatially resolved spectroscopy, allowing us to measure the spectral signature at every pixel. This imaging modality covers large contiguous portions of the EM spectrum, typically from UV to NIR, and possesses more spectral bands (up to several hundreds) than multispectral imaging (which has usually up to 12). As a result, HSI exhibits a much larger amount of information and consequently possesses a greater diagnostic potential than multispectral imaging (Figure 1B). Each pixel of the hypercube contains a spectral curve. For this reason, the hypercube yields a massive amount of information. Depending on the diagnostic purpose, different parts of the spectral signature might contain the relevant discriminative information. For this reason, data extraction and processing are fundamental and has complex steps, which are strictly dependent on the specific application. As a result, the assistance of advanced machine learning (ML) algorithms is required, and the great advances made with hyperspectral data extraction in the field of remote sensing [17], gradually using more sophisticated ML strategies, such as deep learning, are progressively used and adapted into medical HSI.
2.2. Types of Hyperspectral Imaging Hardware
Hyperspectral imagers are categorized into two main groups, according to the data acquisition mechanism, namely scanning devices (divided into spatial and spectral scanning) and snapshot devices (Figure 1C).
Spatial scanning devices acquire the whole spectral information and progressively scan the spatial information. They are either point scanning (wiskbroom), if they scan the spatial information pixel by pixel, or line scanning (pushbroom) if they scan the spatial information line by line. This kind of device typically shows a high spectral resolution, while the spatial resolution is limited by the number of lines or pixels of the image. However, the tradeoff between the spatial and spectral information is often acceptable. Consequently, they are widely used in the medical field [12]. However, the spatial scanning mechanisms are often bulky and require complex hardware, making their miniaturization [18] challenging [19][20]. Another main disadvantage of spatial scanning systems is that the acquisition is not made in real time and it is limited by the speed of the spatial scanning process that may take several seconds. As a result, the accuracy of spatial scanning devices is limited during the acquisition of moving targets, and they tend to produce motion artefacts due to breathing or heart beating, and most importantly, they provide static images.
Spectral scanning devices acquire the spatial information as a whole and they possess a tunable optical element, which allows to image the target using different wavelengths, depending on their spectral coverage. The optical element can be controlled either manually, or automatically as in the case of the Liquid Crystal Tunable Filter (LCTF) or the Acousto-Optic Tunable Filter (AOTF) devices. Considering that the hardware of those cameras is simpler than spatial scanning systems, they can be easily attached to existing optical devices, such as microscopes [21], laparoscopes [22], and flexible endoscopes [23]. However, they are limited by a considerably lower spectral resolution than spatial scanning devices. Of note, they are even more sensitive than spatial scanning cameras to motion artefacts since their spatial information is acquired repeatedly for each spectral wavelength. As a result, this kind of spectral imager also does not allow for real-time imaging.
Snapshot devices can quickly and simultaneously acquire spectral and spatial information. They allow for real-time imaging at the cost of spectral and spatial resolution, which are lower than spectral and spatial scanning devices. Ideally, imaging devices used during surgical procedures must display the spatial information with a high degree of accuracy. This is fundamental to localize the supplementary information onto the surgical field, and as such, it is an advanced imaging modality disclosed to the surgeon. Given their poor spatial resolution, snapshot cameras have been rarely used intraoperatively [14].
In most of the previously published experimental and human studies, the HSI systems used were custom-made. However, as comprehensively described elsewhere [15], there are only a few commercially available hyperspectral systems on the market (and many of the available spectral imagers are multispectral) and still fewer are designed or approved for clinical applications.
3. Hyperspectral Imaging as an Intraoperative Imaging Tool
Hyperspectral imaging is an emerging technology in medicine, particularly in the surgical field. For this reason, the number of studies involving HSI as an intraoperative guidance tool is limited. As previously mentioned, the existing reviews are thoroughly written, but they emphasize biomedical or technical aspects, which can be too intricate for surgeons and inevitably go beyond their needs. The rationale behind the current study is to provide surgeons with an overview of the possibilities and perspectives that HSI offers as an intraoperative imaging tool. The literature for our review was retrieved from PubMed and Google Scholar. Search terms included hyperspectral imaging (excluding multispectral), surgery, intraoperative. We included human and animal studies involving only large animal models, due to their straightforward translatability into clinical practice, in comparison to small animal studies. Ex vivo studies were included only if the HSI acquisition was performed in the operating room. Case reports or studies in a language other than English were excluded.
Intraoperative HSI has been mainly applied to two large areas, divided into subcategories (Table 1). The first is “tissue recognition” composed of (i) cancer recognition; (ii) anatomical structures recognition; and (iii) thermal ablation efficacy recognition. The second is “perfusion assessment” within: (i) colorectal surgery; (ii) upper gastrointestinal surgery; (iii) hepatopancreaticobiliary surgery; (iv) reconstructive surgery; (v) urology; and (vi) neurosurgery.
Table 1. Schematization of HSI applications split into surgical subspecialties.
Application Category |
Application/ Subcategory |
Target | Subject (n) | Device Type | Acquisition Time | Spatial Resolution | Spectral Range | Reference |
---|---|---|---|---|---|---|---|---|
Tissue recognition | Cancer recognition | brain tumor | human (22) | Spatial scanning (two cameras) | 40 + 80 s for both cameras | 1004 × 1787 pixels | 400 to 1700 nm | Fabelo H. et al. 2018 [24] |
brain tumor | human (16) | spatial scanning | ~1 min | 1004 × 1787 pixels | 400 to 1000 nm | Fabelo H. et al. 2019 [25] | ||
brain tumor | human (16) | spatial scanning | ND | 1004 × 1787 pixels | 400 to 1000 nm | Martinez I. et al. 2019 [26] | ||
colorectal cancer | human (54) | spatial scanning | ~6 s | 640 × 480 pixels | 500 to 1000 nm | Jansen-W., B et al. 2021 [27] | ||
colorectal cancer/esophageal cancer | human (22) | spatial scanning | ~6 s | 640 × 480 pixels | 500 to 1000 nm | Collins T. et al. 2021 [28] | ||
Anatomical structures recognition | biliary structure | pig (3) | spectral scanning | ~90 s | ND | 650 to 1100 nm | Zuzak, K.J et al. 2008 [29] | |
ureters, facial nerve | pig (3) | spectral scanning (two cameras) | ND | 1392 × 1040 pixels and 640× 12 pixels | 400–1100 nm and 850–1800 nm | Nouri, D et al. 2016 [30] | ||
artery, vein, bone, muscle, fat, connective tissue, parotid gland, and nerve | human (6) | spectral scanning | ND | 1920 × 1080 pixels | 380 to 1100 nm | Wisotzky, L. et al. 2018 [31] | ||
parathyroid, thyroid, and recurrent laryngeal nerve recognition | human (7) | spatial scanning | ~6 s | 640 × 480 pixels | 500 to 1000 nm | Barberio, M. et al. 2018 [32] | ||
parathyroid, thyroid, and recurrent laryngeal nerve recognition | human (9) | spatial scanning | ~6 s | 640 × 480 pixels | 500 to 1000 nm | Maktabi, M. et al. 2020 [33] | ||
artery, vein, nerve, muscle, fat, skin | pigs (8) | spatial scanning | ~6 s | 640 × 480 pixels | 500 to 1000 nm | Barberio, M. et al. 2021 [34] | ||
Thermal ablation efficacy recognition | thermal effect monitoring during hepatic laser ablation | pig (1) | spatial scanning | ~6 s | 640×480 pixels | 500 to 1000nm | De Landro, M et al. 2019 [35] | |
thermal effect monitoring during hepatic laser ablation | pig (1) | spatial scanning | ~6 s | 640 × 480 pixels | 50 to 1000 nm | De Landro, M et al. 2021 [36] | ||
Perfusion assessment | Colorectal surgery | small bowel perfusion | pig (1) | spatial scanning (two devices) | ND | 484 × 700 and 240 × 420 pixels | 400–1000 and 900-1700 nm | Akbari, H. et al. 2010 [37] |
small bowel perfusion | pig (6) | spatial scanning | ~6 s | 640 × 480 pixels | 500 to 1000 nm | Barberio, M. et al. 2019 [38] | ||
colonic perfusion | human (24) | spatial scanning | ~6 s | 640 × 480 pixels | 500 to 1000 nm | Jansen-W., B et al. 2019 [39] | ||
colonic perfusion | human (32) | spatial scanning | ~6 s | 640 × 480 pixels | 500 to 1000 nm | Jansen-W., B et al. 2021 [40] | ||
acute mesenteric ischemia | human (11) | spatial scanning | ~6 s | 640 × 480 pixels | 500 to 1000 nm | Mehdorn, M. et al. 2020 [41] | ||
Upper-gastrointestinal surgery | gastric conduit perfusion | pig (5) | spatial scanning | ~6 s | 640 × 480 pixels | 500 to 1000 nm | Barberio M. et al. 2020 [42] | |
gastric conduit perfusion | pig (17) | spatial scanning | ~6 s | 640 × 480 pixels | 500 to 1000 nm | Barberio M. et al. 2020 [43] | ||
gastric conduit perfusion | human (22) | spatial scanning | ~6 s | 640 × 480 pixels | 500 to 1000 nm | Köhler, H. et al. 2019 [44] | ||
perfusion of upper abdominal organs | human (20) | spatial scanning | ~6 s | 640 × 480 pixels | 500 to 1000 nm | Moulla, Y. et al. 2021 [45] | ||
Hepatopancreaticobiliary surgery | pancreatic perfusion | pig (6) | spatial scanning | ~6 s | 640 × 480 pixels | 500 to 1000 nm | Wakabayashi, T, et al. 2021 [46] | |
hepatic ischemia differentiation | pig (6) | spatial scanning | ~6 s | 640 × 480 pixels | 500 to 1000 nm | Felli, E. et al. 2020 [47] | ||
hepatic ischemia/reperfusion injury | pig (5) | Spatial scanning | ~6 s | 640 × 480 pixels | 500 to1000 nm | Felli, E. et al. 2021 [48] | ||
hepatic resection guidance | porcine (3) | spatial scanning | ~6 s | 640 × 480 pixels | 500 to 1000 nm | Urade, T. et al. 2021 [49] | ||
Reconstructive surgery | flap perfusion | human (22) | spatial scanning | ~6 s | 640 × 480 pixels | 500 to 1000 nm | Kohler, L.H. et al. 2021 [50] | |
perfusion of free and pedicled flap | human (30) | spatial scanning | ~6 s | 640 × 480 pixels | 500 to 1000 nm | Thiem, D.G et al. 2021 [51] | ||
Urology | renal perfusion | pig (7) | spectral scanning | <30 s | ND | 520 to 645 nm | Tracy, C.R. et al. 2010 [52] | |
renal perfusion (partial nephrectomies) | pig (14) | spectral scanning | <30 s | ND | 520 to 645 nm | Best, S.L. et al. 2011 [53] | ||
renal perfusion (partial nephrectomies) | human (21) | spectral scanning | <30 s | ND | 520 to 645 nm | Holzer, M.S. et al. 2011 [54] | ||
renal perfusion (partial nephrectomies) | human (26) | spectral scanning | <30 s | ND | 520 to 645 nm | Best, S.L. et al. 2013 [55] | ||
renal perfusion (partial nephrectomies) | human (37) | spectral scanning | <30 s | ND | 520 to 645 nm | Liu, Z.W. et al. 2013 [56] | ||
graft perfusion (kidney transplant) | human (17) | spatial scanning | ~6 s | 640 × 480 pixels | 500 to 1000 nm | Sucher, R. et al. 2020 [57] | ||
Neurosurgery | brain perfusion | human (4) | ND | 5–16 s | 640 × 480 data points (pixels) | 400 to 800 nm | Mori, M. et al. 2014 [58] |