The Fourth Industrial Revolution drives industries from traditional manufacturing to the smart manufacturing approach. In this transformation, existing equipment, processes, or devices are retrofitted with some sensors and other cyber-physical systems (CPS), and adapted towards digital production, which is a blend of critical enabling technologies. In the current scenario of Industry 4.0, industries are shaping themselves towards the development of customized and cost-effective processes to satisfy customer needs with the aid of a digital twin framework, which enables the user to monitor, simulate, control, optimize, and identify defects and trends within, ongoing process, and reduces the chances of human prone errors.
1. Introduction
The technological advances, increases in global competitiveness, diversification of customer requirements, dynamic market trends, digitalization, and constantly increasing process complexities of the 21st century have significantly influenced traditional manufacturing industries
[1]. This divergence drives the traditional manufacturing industries towards smart manufacturing, which integrates the elements (robots, Big Data, cloud computing, Internet-of-Things (IoT), Industrial Internet-of-Things (IIoT), simulations, etc.) of the Industry 4.0 framework
[2]. This helps in developing processes or products with built-in capabilities, such as decision-making, performance optimization, reconfiguration, and adaptation within the stated framework
[3]. It is estimated that the smart manufacturing market will grow from USD 214.7 billion to USD 384.8 billion with a CAGR of 12.4% for the period of 2020–2025
[4]. Along with all the benefits of integrated elements and digitalization, maintenance is still a challenging issue in smart manufacturing.
Maintenance is one of the key factors that affect the industry economically and has gained special attention in the era of digitalization. With the use of advanced technologies, such as sensors, actuators, automation, and mechanization, maintenance has become the key issue in today’s industry
[5]. The overall cost of maintenance is estimated at approximately 15–40% of the total production cost
[6]. A lack of maintenance activities leads to sudden equipment failure, unplanned downtime, loss of productivity, and increases in production cost and time
[7]. Hence, a proper maintenance strategy needs to be deployed to address the issue. In general, there are three maintenance strategies, viz. preventive maintenance, reactive maintenance, and predictive maintenance
[8]. Preventive maintenance is analogous to scheduled maintenance or planned maintenance, in which the maintenance activities are scheduled or timed based on a process or product cycle
[9]. Too high a frequency of preventive maintenance leads to higher production costs, excessive equipment planned downtime, and loss of resources
[10]. However, reactive maintenance is a kind of failure-based maintenance, in which maintenance is performed after the failure of equipment or a component. It is also called “corrective maintenance” or “breakdown maintenance”
[11][12]. Reactive maintenance leads to sudden equipment failure, unplanned downtime, losses of productivity, and increases in costs of production
[13].
Among all the maintenance strategies, predictive maintenance is the most cost-effective maintenance strategy, which allows one to schedule maintenance when the equipment or machinery needs maintenance. It is condition-based, and its performance is based on the predictions made from the signals acquired from the machine
[14][15]. Predictive maintenance offers various benefits over traditional maintenance strategies such as earlier fault detection, reduced downtime, improved reliability, reduced cost, and improved performance
[16]. According to the U.S. Department of energy, predictive maintenance saves approximately 8–12% over preventive maintenance and up to 40% over reactive maintenance
[17]. The use of predictive maintenance increased from 47% to 51%, which reduced equipment failure from 61% to 57%, between 2017 and 2018
[18]. Therefore, maintenance has a direct influence on the industry’s economics. Additionally, in the present era of Industry 4.0, intelligent maintenance strategies with the help of digital twin (DT) can offer huge benefits over present maintenance approaches
[19].
Within the Industry 4.0 paradigm, the technological advancements in cyber-physical systems (CPS) and the steady improvements in the smart manufacturing frameworks spawn the concept of a “digital twin”
[20]. DT is the systematic integration between a physical entity and a virtual entity through different connections and the real-time data of the process
[21]. The advancement in enabling technologies, such as data analytics, IoT, IIoT, big data, cloud computing, sensors, augmented and virtual reality, simulation, artificial intelligence, etc., facilitates the seamless integration of DT into prominent fields
[22]. The DT environment allows real-time decision making, rapid reconfiguration, and optimization; it also facilitates improved reliability, better productivity, reduced risk, early fault detection, and lowered downtime
[23]. Due to the enormous advantages, DT can be used in different fields, such as aerospace, automotive, biomedical, healthcare, manufacturing, etc.
[24]. Therefore, the DT market is estimated to grow from USD 3.1 billion to USD 48.2 billion with a CAGR of 58% between 2020 and 2026
[25]. Hence, to study the different aspects of DT in the context of smart manufacturing, this paper provides a comprehensive bibliometric analysis of DT in the domain of smart manufacturing and studies the benefits, applications, and challenges in implementing a DT in real-time.
2. Case Study: Hybrid Predictive Maintenance of CNC Tool Using Digital Twin
As the CNC machine is the mother of all computerized machines, the machine tool is its most vital component, impacting the product quality, machining quality, time, and cost. Hence, performing timely maintenance is the most crucial task. The traditional maintenance method permits some human error, leading to significant equipment downtime, and increasing the cost. Therefore, the researcher here proposed a hybrid predictive maintenance method for the CNC machine tool using digital twin and verified the proposed methodology for use in estimating the remaining life of the cutting tool
[26]. In the hybrid approach, the model-based and data-driven methods are combined to derive more precise projections/forecasts.
To create the digital twin, a multi-space model of the CNC machine tool is constructed considering the degradation mechanism, which acts according to operating conditions and the material attributes. Through multi-physical simulation with the limit condition derived from the actual CNC machine tool, the condition inside can be configured, which resembles virtual detecting. The framework state-space model is an adaptation of the DT actual degradation model and is used to calculate framework states through hypothetical examination of the framework inside via re-enactment. Different sorts of sensors are introduced into the physical CNC machine tool and give information supporting the data-driven technique to estimate the RUL of the parts. In the data-driven strategy, precise data must go through a few stages, such as feature extraction, noise filtration, data pre-processing, and state acknowledgment; lastly, they become useful for forecasting. The RUL anticipated by the data-driven technique is utilized as the framework perception of the CNC machine tool. Hybrid algorithms combine the state space value, simulated value, and observational value, which are used to predict the reaming useful life and verify it, as depicted in Figure 1 below.
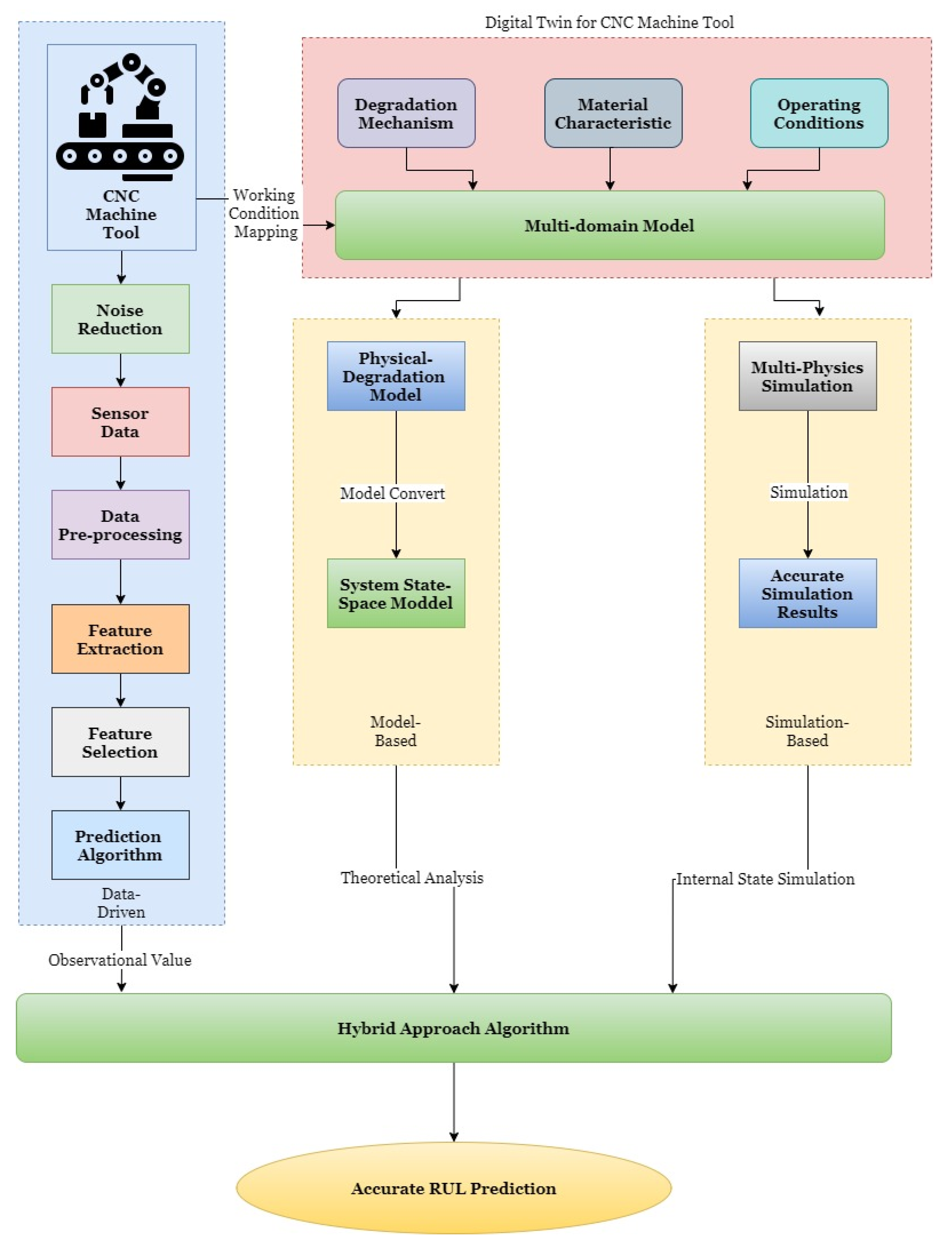
Figure 1. Framework for hybrid predictive maintenance approach for RUL estimation.
The proposed methodology was implemented for the prediction of the RUL of the CNC milling machine tool. The data-driven models were built using the run-time values derived from the accelerometer, dynamometer, and acoustic emission sensors. Simultaneously, the simulation model was developed using the data from the tool wear equation, and mathematical models for drive and working conditions gave data such as feed, spindle speed, depth of cut, and surrounding temperature. In the hybrid approach, data from both models are fused to train the particle filtering algorithm, which overcomes the limitations of each model in predicting the remaining useful life of the tool. Additionally, using the data from both the models, a digital twin for a tool is developed, and using communication protocols, the developed DT is updated continuously in the run-time environment.
Thus, the proposed hybrid predictive maintenance algorithm enables the better integration of system data and the precise prediction of the RUL of a milling tool through its digital twin.