3.1 Automated contend production
The automation of the news creation process is perhaps the most important - and as a result the most controversial - of all the fields of application for algorithmic technology in journalism (Montal and Reich 2017; Schapals and Pmontaorlezza 2020). In the grand scheme of things, this particular field of application is considered a relatively recent development in the field of journalism (Ali and Hassoun, 2019; Graefe 2016) and it consists mainly of algorithms and automated software that are capable of creating news stories on their own (Diakopoulos 2019).
One of the most well known examples of early applications for automatic content production is that of "Quakebot", a program that was created on behalf of the Los Angeles Times in 2014. Its purpose was to closely monitor data from the US Geological Survey in an attempt to identify instances on seismic activity and proceed to write and publish simple reports on them (Otter 2017). Since then, automatic content production has taken major steps forward, to the point where some of the biggest contributors to the industry such as Forbes and The New York Times often rely on algorithmic production for their content, with the end result being almost impossible to distinguish from human writing (Clerwall 2014).
The basis for the innovations in automated content production is a technology called "Natural Language Generation" or NLG for short. Natural language generation is defined as "the automatic creation of text from digital structured data" (Caswell and Dörr 2018) and it is a technology that first made its appearance in the 1950s within the context of machine translation (Reiter 2010). NLG has seen exponential growth in the past few years and in light of these developments many industries begun to utilize it alongside artificial intelligence to further improve their products and services, with the news media industry being no exception to this rule (Diakopoulos 2019).
The adoption of these technologies by the journalistic profession brought with it a number of advantages, including a significant increase in productivity thanks to the publishing of stories without any human intervention (
Ali and Hassoun 2019) as well as the ability to allow journalists to redefine their core skill set (
Van Dalen 2012) and provide them with more creative freedom in their work (
Milosavljević and Vobič 2019), since computers were able to execute part of their responsibilities by taking over routine tasks (
Glahn 1970). Those advantages also seem to coincide with the increasingly high market demands for fast and accurate news stories, making algorithmic news production even more beneficial (
Clerwall 2014;
Diakopoulos 2019).
Thanks to the above, algorithmically generated news started to become a near necessity in the modern news production cycle (
Zangana 2017), which, in turn, has led to various forms of controversy from members of the news industry. The main discussion point between journalists and people that are employed in the news industry as a whole is the possibility that the automatization process might render human workers in the field obsolete (
Veglis and Maniou 2019). There have been many arguments recorded in related literature when it comes to this topic, and many workers have also voiced their opinion, suggesting that the increasingly dominant role of algorithms in the newsroom will pose a serious threat to the future of human journalists (
Kirley 2016). On the opposite end of the spectrum, a number of researchers seem to suggest that those fears are mostly unfounded, pointing out that artificial intelligence and algorithms are only going to enhance journalistic practice in the long run instead of replacing it (
Hansen et al. 2017).
Drawing a line between what might be a useful innovation and what might pose a threat to the industry due to the potential loss of jobs is certainly no easy task, and that is perhaps the reason behind this apparent split in the existing literature, with many researchers pointing out the benefits of automation, and others focusing on the potential danger it encompasses for the employees of the media industry. It is certain that automated content production plays a major role in the news production process nowadays, and it is commonly agreed by researchers that automation will hold a critical role in the future of news agencies (
Liu et al. 2017). As competition within the industry continues to rise, the only way to keep up with the ever-increasing demand for more news stories seems to be the utilization of automated content production technologies. The question remains, however, as to how the industry is going to adapt to these new conditions of automation, as the displacement of employees and an overall reduction of the workforce is indeed inevitable based on current projections (
Carlson 2015), as machines become more and more capable in substituting human workers in specific tasks.
There are a number of views shared by researchers and employees in the media industry that tend to challenge the arguments presented above, regarding the ability of algorithms to “free” journalists and allow them more time to pursue more investigative tasks (
Schapals and Porlezza 2020). These concerns mostly stem from the fact that computational technology is shaping journalism into a more streamline and sterile process, one that does not necessarily require human input in order to function, and they bring up some very valid points regarding the skill set that a modern journalist is expected to have in order to compete in this environment. Taking that into consideration, the fact that automation will make a number of jobs obsolete given enough time seems to be an inevitable outcome. While the way the industry intents to deal with this problem still remains to be seen, perhaps one potential solution to it lies in the adjustment of expectations and the redefining of the term “journalistic labor”. As
Carlson (
2015) puts it, “Automated journalism requires the transformation of journalistic labor to include such new positions as “meta-writer” or “metajournalist” to facilitate automated stories”. This point of view suggests that in order to achieve a fully symbiotic relationship between human workers and machines, a middle ground has to be reached, specifically one where media industry workers need to reevaluate their priorities and develop a skill set that supplements algorithmic news production, instead of attempting to compete head-on with it. In accordance to what
Van Dalen (
2012) has stated, this can be seen as an opportunity for workers to redefine their core skills and work in tandem with algorithms, as ultimately, these programs are fundamentally different from humans, since they lack traits such as creativity, flexibility and analytical thinking, which would mean that in order to achieve the best and overall most efficient result, both parties would need to work together and cooperate.
The fact that these programs lack traits such as creativity, flexibility and analytical thinking is an important factor that separates them from humans (
Van Dalen 2012); as such, these technologies do not present an immediate threat to the practitioners of the journalistic profession (
Ali and Hassoun 2019).
Despite how important automated content creation has been for the industry, it is apparent that algorithmic journalism is not limited just to the creation of automated news stories (
Jamil 2020). There are other important fields of application for these technological innovations that that have also impacted journalism in a major way, which will be examined below.
3.2 Data Mining
One of the most defining characteristics of the information age that we are currently undergoing is the so-called “data explosion”, which refers to the constant increase of widely available data on the internet, with some sources approximating that the digital universe roughly doubles in size every 18 months (
Zhu et al. 2009). Data, however, should not be mistaken for information (
Aljazairi 2016). Within this ever-increasing landscape of available resources, journalists are struggling more than ever to separate clutter from actually useful information (
Chen and Liu 2004), and this is where the need for procedures such as data mining starts to become apparent.
According to
Bramer (
2007), data mining is a central part of a broader process called “knowledge discovery” and it refers to the extraction of useful information from a larger subset of data (). There are many applications for this type of technology in journalism, with the most obvious one being the acquisition of specific information from large databases. The case of “Quakebot” that was mentioned above also constitutes a very good example of data mining, despite the fact that it is mostly known to be an instance of automated content production, since the program was able to single out and use information form a much larger dataset (which was all of the data provided by the US geological survey). Chatbots and other similar automated agents have been utilized extensively in these procedures (
Veglis and Kotenidis 2020).
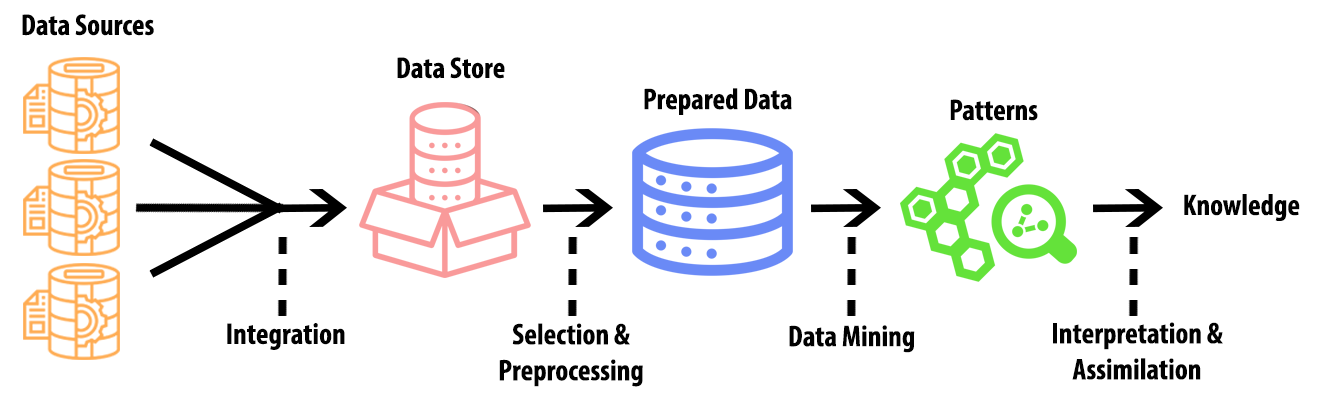
Figure 2. The knowledge discovery process according to
Bramer (
2007).
Other than this more obvious use case, however, the technology behind data mining can also be utilized for various other complex tasks related to journalism. For example, there are instances where datasets are too massive for humans to even comprehend, because of characteristics such as their volume (terabytes–petabytes) or their velocity (being created in real time), and this makes algorithmic data mining the only reasonable way to tackle these so-called “Big Data” (
Kitchin 2014). Journalists often find themselves working with these types of data sets as part of their job and data mining can help them uncover previously unseen connections between variables with high statistical significance, which in turn can allow them to test complex ideas and hypotheses (
Latar 2015).Data mining also has the ability to enable other fields of application found in algorithmic journalism since it can be used to discover new social trends and automatically target specific consumers who might find the content more relevant (
Latar 2015), as well as being used in conjunction with automated content production, as seen in the example presented earlier in the manuscript.
While procedures such as data mining have mostly been recognized as strictly beneficial to the journalistic cause, there is still a discussion to be made regarding their ethical side. As
Kennedy and Moss (
2015) point out, the undoubtable usefulness of algorithmic mining—specifically in online spaces with user interactivity such as social media—can occasionally be overshadowed by privacy considerations regarding user surveillance that could lead to social discrimination. Metadata analyzed in this way can sometimes be even more valuable than the content that is being shared. Of course, as is the case with any tool, the intent behind the usage of data mining software is equally as important as any practical concerns surrounding it and that is the reason that studies such as the one mentioned above propose the democratization of these procedures via the introduction of regulations and more meticulous public supervision.
In addition to the above, the question of accessibility that has been raised earlier in the manuscript also applies to these advanced tools. Similarly to algorithmic news production, the introduction of Big Data and the appropriate procedures required to analyze them has also impacted the news industry in a big way, not only in the productivity department, but also in the skills required to work in this new and rapidly changing environment (
Hammond 2017). In order to be able to understand the complex information hidden in large datasets, workers in the news industry should be able to utilize modern tools and special software that will allow them to take full advantage of Big Data in order to supplement their reporting and information-gathering procedures (
Veglis and Maniou 2018). This argument is closely related to the considerations that surround automated content production, in the senses that the evolving media landscape is going to require workers to acquire a much more specialized role in order to stay competitive in this increasingly automated work environment. Much of what has been said about automated programs replacing human workers in the case of content production can also be said here, although in the case of algorithmic data mining, there are some notable exceptions such as the analysis of Big Data itself. In these instances, software agents seem to only expand the capabilities of the modern journalist, without any risk of replacing actual workers, since Big Data and other similar concepts are by their very nature unable to be processed by humans and would otherwise be inaccessible without the help of algorithms (
Kitchin 2014).
3.3 News dissemination
In this day and age, the internet accounts for a very large portion of daily media consumption (
Gaskins and Jerit 2012) and as such the way the dissemination of news is handled proves to be exceedingly important (
Orellana-Rodriguez and Keane 2018). There are three main platforms through which the majority of internet users receivetheir news content from, namely: news aggregators, search engines and social media sites (
Foster 2012). These digital intermediaries all have something in common: they largely rely on algorithms and automated systems in order to appropriately distribute content to their users (
Cádima 2018).
As media companies started to shift their focus on online news and the implementation of more interactive features (
Deuze 2005), these automatic news dissemination technologies proved to be a major driving force for journalism since news organizations started to utilize them more and more (
Carlson 2018). The advantages that emerged in the field of journalism through the use of these innovations became apparent quite quickly. Specifically, news outlets were able to utilize algorithms in order to automatically and systematically disseminate news on social media and other similar platforms, by using software agents called “news bots”. These programs are capable of distributing news and information to a large audience, as well as interacting with users in various ways and ensuring high visibility for the content in question, thereby supplementing the news dissemination process and helping media agencies to reach as wide an audience as possible (
Lokot and Diakopoulos 2016).
Controversy has also been observed in this field of application, although perhaps not to the extent of automated content production. Specifically, concerns have arisen from researchers over the years regarding the role of algorithmic news distribution technology as a “gatekeeper” of news (
Nechushtai and Lewis 2019;
Cádima 2018), the accountability and the impartiality of these programs (
Diakopoulos 2015) as well as ethical considerations regarding algorithmic transparency (
Diakopoulos and Koliska 2017) and the role these agents play in the spread of fake news and misinformation (
Shao et al. 2017;
Shin and Valente 2020;
Fernandez and Alani 2018).All of the above constitutes well-funded criticism related news dissemination that has yet to be addressed in a meaningful way. When it comes to news gatekeeping in particular,
Cádima (
2018) brings up an important point regarding the intermediation issue. As digital intermediaries are estimated to be redirecting more than 70% of internet news traffic, it is difficult to ensure that news circulation will remain democratic going forward. This poses a lot of questions about the future of journalism that are related both to quality deterioration, as well as censorship issues that could potentially affect a very large subset of the population. Ensuring that communication channels remain open and not allowing any third parties to consistently prioritize certain voices over others will prove vital for the future of the journalistic profession. Ultimately, however, an agreed-upon standard for humans as news gatekeepers does not exist, and this fact makes it all the more challenging to assess the performance of algorithms in this regard (
Nechushtai and Lewis 2019).
3.4 Content Optimization
Personalized content for individual recipients is not a new idea in the media industry, as some researchers have suggested functioning models for it even before the turn of the 21st century (
Bharat et al. 1998;
Billsus and Pazzani 1999). Despite this, however, it was not until the past few years that developments in algorithmic technology allowed news providers to target specific audiences on a large scale and deliver customized news experiences for them, thanks to the internet’s ability to provide almost real-time recommendations and information from all over the world (
Li et al. 2011). These personalized news content services have proved to be very useful because they can save time for the end used by drastically reducing the amount of irrelevant information and provide content only for subjects that are of interest (
Jokela et al. 2001).
Content optimization for users usually works in a similar manner to search engines, which utilize automated ranking algorithms in order to return the most relevant results for a user’s search. Using a similar structure, personalized news content and online ads are served to specific users with the use of automated algorithms (
Agarwal et al. 2008). Content optimization with the help of algorithmic technology has also been observed in other parts of the news production process, as some organizations utilize algorithms for tasks such as A/B testing for article headlines in order to better gauge their effectiveness (
Lokot and Diakopoulos 2016). The prime use for this technology, however, has been the delivery of personalized news content through customized newsfeeds or automated agents such as chatbots. These automated bots in particular have proven to be very effective in engaging with audiences by providing more interactive and personalized instances of news and articles as opposed to the traditional methods of content consumption (
Jones and Jones 2019).
Even though this technology provides a user-friendly way of consuming more relevant content, there have been a number of concerns regarding its use that are worth addressing. First off, some privacy concerns have been brought to light by users over the years in regard to content optimization. Specifically, those concerns are related to the way these algorithmic solutions function, since most content optimization systems from media organizations and other companies alike rely on the collection of personal data in order to fulfill their duties (
Das et al. 2007). Furthermore, the personalization employed by these algorithms often remains unnoticed by the users (
Powers 2017), which further feeds into this issue. That is the reason many researchers, such as
Diakopoulos and Koliska (
2017) and
Graefe (
2016), have started to advocate for algorithmic transparency over the past years, since many users do not feel comfortable with the idea of “being watched” by automated programs without them being notified while they are browsing the internet, even if that action ultimately aims to benefit them with more streamlined recommendations.
The privacy concerns mentioned above are likely to grow in scale with each passing year as technology gradually envelops more and more aspects of daily life, and as such, it is important for algorithmic transparency to be established as one of the pillars upon which future innovations can be developed, in order to avoid further frictions. Despite their importance, however, these concerns are not the only ones that were brought to light when it comes to personalization algorithms. Another relevant issue in this field of application has to do with the content that is being distributed. Specifically, researchers have noted that the constant stream of personalized content has the potential to negatively affect the news ecosystem, since it has been known to reduce news diversity for recipients and consequently lead to partial information blindness (
Haim et al. 2018). This phenomenon became widely known with the term “filter bubbles”, with similar theoretical constructs such as “news echo chambers” describing constant user exposure to like-minded opinions (
Garrett 2009). These online environments that stand devoid of varied viewpoints constitute a serious criticism regarding news personalization, since they tend to reinforce the user’s opinion on specific matters, and usually offer no counterpoints, or even alternative viewpoints to the one they have chosen to adopt. Even though this phenomenon is not exclusive to these technologies, or even to the internet as it can be observed in other media as well, the nature of online personalized content delivery seems to be enhancing this particular problem. To put it in simpler terms, while algorithmic personalization caters to the needs of the user and creates a more enjoyable and customizable experience, it also simultaneously encompasses them in their own “bubble” and prevents them from challenging their beliefs. This criticism puts the model of personalized news delivery into question, as it can be the epicenter of some serious ramifications in the future that can range from the spread of misinformation to the potential fragmentation of the public opinion (
Graefe 2016).