Evapotranspiration (ET) is a process that includes evaporation from the surface, such as open water bodies, soil and vegetation, and transpiration as the water released mostly by the plant leaves transported from the root system. ET is a key factor in the hydrological cycle, since it describes the mechanism and energy needed to transport the liquid water stored in the soil-watershed-canopy system to the atmosphere, converted into water vapour.
2. Single-Source Energy Balance Models
2.1. Surface Energy Balance Index (SEBI) and Simplified-SEBI (S-SEBI) Models
Originally proposed by
[11][91], the physically-based assumption is that ET varies with the
Ts for a homogeneous surface. For a given surface albedo and boundary layer conditions, there exists a temperature gradient between the surface and the atmosphere (
Ts−Ta) at some crop height, that ranges from a maximum value for which ET is assumed to be zero, to a minimum value for which the potential ET (
ETp) is taken, as given by the Penman-Monteith equation
[7].
There exists a correlation between
Ts and the surface reflectance (albedo), where for low reflectance values
Ts remains constant with the progressive increase of such reflectance. This phenomenon is attributed to presence of body water or well-watered surfaces, where all the available energy is used for the evaporation. From certain reflectance threshold
Ts increases with the reflectance, inducing a decrease in the evaporation, in the so-called “evaporation controlled” range. From another higher reflectance threshold,
Ts decreases with the increase of the reflectance, because the
Rn decreases due to the high reflection of the energy received, a process known as “radiation controlled”.
Based on the previous assumptions, for a specific surface given
Ts,
[11][91] provided a value for
ET by means of an interpolation expression:

where
Tpbl is the average air potential temperature at higher elevation or at the top of the Planetary Boundary Layer (pbl) and
raH,min and
raHmax are the minimum and maximum aerodynamic resistance in the sensible heat flux H
[12][49].
A simplified version of the SEBI method (S-SEBI) was proposed by
[13][92] with the advantage that no additional meteorological data are needed if the hydrological conditions of the surface are extreme enough to observe a significant range of gradient temperatures for the corresponding reflectance spectra.
Therefore if for a given
αs value the two extremes of
Ts exist, that is for
Hmax (
Ts,max,
αs) and
LEmax (
Ts,min,
αs),
[13][92] expressed the
ET value as:

The method is called simplified if the wet and dry pixel conditions are observed for a specific albedo value in a satellite scene. But
[13][92] simplified even more Equation (19) by adjusting linearly the trends of maximum and minimum
Ts with the albedo, according the following equations:

where
amax and
bmax are the slope and offset coefficients for the linear regression of the maximum temperature values with the albedo and
amin and
bmin are the corresponding coefficients for the linear regression of the minimum temperatures, also with the albedo. These four parameters must be adjusted in each individual satellite scene. Including Equations,
ET expression from S-SEBI model can be defined then as:

Very few modifications have been proposed in the literature for the S-SEBI model. One of them is made by
[14][93], which in order to avoid using the LE and H fluxes, it is proposed using instead the evaporative fraction (EF), that can be extracted from the T
s-α scatterplot shape.
A recently
publis
hed study
[15][94] proposed an adaptation of EVapotranspiration Assessment from SPAce (EVASPA) tool
[16][95] to S-SEBI model. This model, denoted as E3S (for EVASPA S-SEBI Sahel), proposes two relevant changes. The first one is related to the handling of the algorithms determining the wet and dry edges, since in some regions like the Sahel with strong seasonal contrast only one edge may be identified. This led to the introduction of twelve additional seasonally-dependent algorithms to estimate the dry and wet points, using an ensemble member weighting. Secondly a cloud edge filtering method was implemented, to avoid that pixel outliers can distort the T
s scatterplot shape. The cloud edge filtering was developed in two levels: (1) the first level filter was based on the quality flag provided by the MODIS land surface temperature (LST) products. Thus, all pixels bordering clouds and associated with an LST error >1 K were removed; (2) a second level filter consisted in extending the first level filter to cloud-bordering pixels with LST below the first LST quartile in the entire image. This quantile level selection could be site and climate-specific.
2.2. Surface Energy Balance System (SEBS) Model
Proposed by
[17][46] the SEBS method is based, as it is S-SEBI, SEBAL and METRIC (to be described below), on the same main concept as SEBI. The difference of SEBS from the rest is that it deals with estimating the ET fluxes at large scales, therefore considering heterogeneous surfaces.
Therefore, the assumption of constant atmospheric conditions for the whole scene is not applicable. Moreover, spatial variation of roughness height for different surfaces is allowed.
The SEBS is an extended model that defines the surface roughness length in the heat fluxes, reformulating the EF at the limiting cases. To this end in the SEBS theory the dry and wet case limits are:
Dry limit:


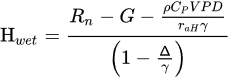
where
VPD is the vapor pressure deficit (difference between saturated and non-saturated vapor pressure), Δ is the slope of the curve relating temperature difference (
Ts-Ta) with
VPD, and
γ is the is the psychrometric constant.
As an intermediate step SEBS method defines a relative
EF (
EFr) as:
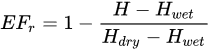
Some studies presented a modification of the SEBS inner structure in terms of: (i) momentum roughness length and zero plane displacement; (ii) an empirical relationship between land surface temperature and air temperature; and (iii) designing a new input interface of meteorological field at reference, with the objective of representing land surface and climatic changes of a specific region. These studies adapted SEBS to be applicable in different countries like Taiwan (SEBS-Taiwan,
[18][102]), China (SEBS-China,
[19][103]) or Iran (SEBS-Iran,
[20][104]). SEBS was also modified at local scale (SEBS-Urban,
[21][105]) after including the anthropogenic heat flux in the SEB equation to estimate the ET of Beijing city. This
study hypothesized that ET was equal to the water consumption in the study area.
An improvement of SEBS method was suggested by
[22][106] by correcting the excess resistance with a scale factor dependent on soil moisture (
SM), following:

where
a,
b and
c are coefficients of the sigmoid function and
SMrel is a relative soil moisture defined as:
SMmax and
SMmin values can be obtained using a time series analysis of the soil moisture data on annual or long-term basis
[22][106]. Wu et al.
[23][107] suggested the use of the Normal Differential Water Index (NDWI) instead of
SMrel in Equations. Yi et al.
[24][108] also recommended replacing
SMrel by the inverse of the modified perpendicular drought index (MPDI).
It is also worth mentioning the study of Chen et al.
[25][109], in which the excess resistance is internally modified to be robust for heat flux calculation for high and low canopies, since it has been proved its depends on environmental conditions, surface types, canopy structure and vegetation surface geometry.
kB−1 structure was adjusted from a one-foliage layer to a multi-foliage layer, which provides the possibility of including the impact of vertical variations in foliage leaf area density, foliage shelter factor, and foliage heat transfer coefficient.
2.3. Surface Energy Balance Algorithm for Land (SEBAL) Model
Proposed by
[26][120], SEBAL is an iterative and feedback-based numerical procedure that deduces the radiation, heat and evaporation fluxes. SEBAL estimates LE with the residual of Equations in a pixel by pixel satellite scene, just considering two anchor points (dry and wet points) for the full scene. At the dry point
LE = 0 and
H =
Rn-
G, and at the wet point
H = 0 and
LE can be considered either
Rn-
G or a reference ET
[7].
The main consideration of SEBAL is to establish a linear regression between
Ts and difference of
Ts with the air temperature at the crop height z (
δTa), as follows:

where
c1 and
c0 are the empirical coefficients derived from anchored conditions of wet
(δTa,wet = 0,
Ts,wet) and dry points (
Ts,dry), for which the temperature difference is expressed as:
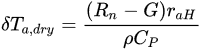
A first modification of SEBAL was proposed by
[27][121] after introducing Digital elevation model (DEM) data to account for impacts of slope aspect on incident solar radiation. But the main subject of modification of SEBAL model is related with the choice of endmembers or anchor points (i.e., “hot” and “wet” points in the satellite scene). A Modified version of SEBAL (M-SEBAL) was proposed by
[28][122], that based the election of the anchor (dry/wet) points on the type of surface, defined by the FVC. They adjusted Equations for every specific surface defined by FVC and subsequently estimated H and LE. Feng et al.
[29][123] derived geo-mathematically four reference dry and wet limits, instead of the classical option of two anchor points.
Another modification is addressed in
[30][124], where
resea
rcheuthors deal with the endmember pixel selection process, proposing an automated procedure by introducing an exhaustive search algorithm (ESA), in which all possible candidates are systematically considered and checked to determine if the solution is satisfied, based on two steps: (1) create a binary map of candidate pixels that are identified using a simple rule-based classifier, and (2) apply ESA to identify hot and cold pixels that meet the defined criteria. In this sense,
[31][125] created the automated SEBAL (ASEBAL) model that allows automatic selection of the endmember pixels according to criteria based on the variation in biophysical parameter values retrieved from satellite products.
It is also worth mentioning the study of
[32][126], where
resea
rcheuthors found more appropriate to combine the capabilities of Synthetic Aperture Radar (SAR) with optical remote sensing technique in the applications where soil moisture conditions to locate anchor pixels, since SAR is more suitable to detect soil moisture conditions.
Two interesting studies that suggested modifications of SEBAL ET model were related with changes in the SEB equation, by introducing new fluxes terms that must be accounted in certain situations. Mkhwanazi et al.
[33][127] proposed a modified version of SEBAL model (SEBAL-A), that is capable of accounting for advection flux term, estimated with a wind function which included a daily mean or extreme temperatures parametrization, which is incorporated it into the original SEBAL daily ET sub-model.
[34][128] also suggested enhancing the SEBAL model when it is applied in a heterogeneous urban environment (uSEBAL), after considering urban land surface parameters and including the urban anthropogenic heat flux in the SEB budget.
Finally, it is worth to note that recent open access software code versions of SEBAL are accessible. One of them is a new python version of SEBAL (pySEBAL), which incorporates an automated pixel selection procedure and is currently under development and testing at the IHE-Delft Institute
[35][129]. In addition, a new tool based on the SEBAL algorithm, modified with a simplified version of the Calibration using Inverse Modeling at Extreme Conditions process
[36][130] for the endmembers selection, was developed within the Google Earth Engine (geeSEBAL) environment
[37][131].
2.4. Mapping Evapotranspiration at High Resolution and with Internalized Calibration (METRIC) Model
METRIC was proposed by
[38][54], based almost completely on SEBAL method. The main differences with SEBAL are that
Rn and
Ts are corrected topographically using a Digital Elevation Model. Both methods differ in the calculation of H as in METRIC the temperature gradient of the wet point is expressed as:

The assumption that ET rate (
ETr) is about 5% above that of the standard
ETr in the METRIC model is assumed because, for a large population of fields, some fields will have a wet soil surface beneath a full vegetation canopy that will tend to increase the total ET rate
[38][54].
Since METRIC is directly based on SEBAL model, all the modifications previously discussed in
Section 3.1.3 can also apply to METRIC. However, it is interesting to note a recent study
[39][138], where calibration variability in METRIC is examined through the anchor pixels selection. Following METRIC original procedure to detect “cold” and “hot” pixel in a scene, the
resea
rcheuthors detected a range of different “cold” and “hot” pixels that conformed a total of five sets of calibration pixel pairs (based on combination of maximum and minimum “cold” and “hot”, as well as values closest to the flux station). So, different slopes and intercept values were retrieved from the plot
δT vs. Ts. In summary,
resea
rcheuthors observed significant spatial differences in ET across the study area, which suggested that candidate calibration pixels cannot be completely quantified using only NDVI and T
s. Therefore, it can be presumed that the large differences in
δT among the candidate pixels were not random noise, and that the inclusion of available energy into the calibration pixel selection process could potentially improve the calibration process and provide better estimates of ET.
3. Land Surface Temperatures–Vegetation Index Triangle/Trapezoidal ET Models
Vegetation Indices (
VIs) offer important information from
Ts−VI triangle/trapezoidal relationship. These diagrams can be useful for estimating regional ET values under the condition that full ranges of
SM content and vegetation are present in the remote sensing scene.
The Triangle method was described by
[40][146], and provides a biophysical justification for the combination of T and vegetation indices (VI) time series for land-cover mapping and land-cover change analysis. The lower envelope represents the wet edge, which is nearly constant and represents the lowest
Ts for the different types of surfaces, assuming here that the
ET is potential. Theoretically the two envelopes (dry and wet edges) intersect in a point of full cover vegetation and maximum
ET.
Based on the Priestley-Taylor formulation
[41][147] and fully remotely sensed data, an expression proposed by
[42][148] allows estimating the
LE according to the
Ts−VI triangular relationship as:
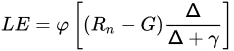
where
φ varies from 0 to 1.26, and it is estimated with a two-step linear interpolation. This interpolation consists in: (1) once set a
φmin = 0 (driest bare soil) and
φmax = 1.26 (largest
VI and lowest
Ts), for each intermediate VI a
φmin,i is estimated by interpolation between
φmin and
φmax and a
φmax,i is assigned to the minimum
Ts value of such VI (usually 1.26), (2) Different
φi can be estimated from the
Ts range at each
VI.
VI can be either the
NDVI,
FVC, the Soil Adjusted Vegetation Index (
SAVI), Enhanced Vegetation Index (
EVI), etc.
The
Ts−VI Trapezoidal method based on the proposed Water Deficit Index (
WDI) by
[43][70], an index related to the ratio of actual and potential evapotranspiration, which evaluates the evapotranspiration rates of both full-cover and partially vegetated sites. WDI based
Ts−VI Trapezoidal method considers that full covered vegetation can both water-stressed and well-watered in a satellite scene, so there is no intersect in a point of full cover vegetation and the
Ts−VI diagram become a trapezoid. Moran et al.
[43][70], extended the ET estimation from full to partially vegetated surface areas, considering that temperature gradient (
Ts−
Ta) varies linearly with the vegetation status. So,
WDI is linearly related at each
VI to the maximum (dry bare soil) and minimum (probably well-watered full-cover vegetation) gradient temperature, taking values between 0 and 1 and
LE is calculated similar to Equation as the ratio of potential
ET calculated from Penman-Monteith equation
[7],
LE for the trapezoidal method is expressed as:

Stinsen et al.
[44][149], combined the triangle method with thermal inertia information obtained from MSG-SEVIRI sensor, by changing the surface temperature over time.
ResearcAuth
eors also applied a slightly modified version of the two-step interpolation scheme to obtain
φ, based on a interpolation where the decomposition is regarded as non-linear with the argument that non-linear intersections are observed in the dry edge and in the iso-lines of equal moisture availability of the
Ts−VI tringle feature. Adopting equations
from this study,
[45][150] proposed a Time-Domain Triangle Method (TDTM), based on a pixel by pixel T
s–VI feature space, obtained by exploring the temporal domains over each single image pixel, since the triangle contains the whole climatic variability of the T
s–VI. Also, in
[46][151], an algorithm to determine quantitatively the dry and wet edges for the T
s–VI triangular space in arid and semi-arid areas, where wet pixels are not generally easily identified, was developed with an iterative process that automatically retrieve the two edges in the T
s–FVC triangle space in ten steps. The process starts dividing the range of FVC in the T
s–FVC triangle space into
M intervals evenly and ends with a linear regression to obtain the dry edge.
Another interesting modification of the
Ts−VI triangle method is proposed by
[47][152] that estimate daily ET directly using the top of atmosphere (TOA) radiances without performing atmospheric correction or other processes, to avoid the uncertainty associated with the estimation of ET, attributed to satellite data products. Furthermore, a recent study
[48][153] estimates the soil heat flux (G) in the trapezoidal space of Ts-FVC, prior to apply the original
Ts−VI trapezoidal method afterwards.
4. Dual (Two)-Source Energy Balance (TSEB) Model
A two-layer model of turbulent exchange that includes the view geometry associated with directional radiometric surface temperature was developed and evaluated by
[49][43]. The main assumption of TSEB model is that
Ts estimated from remote sensing measurements is the composite of soil and canopy surfaces, and it can be expressed as:

where
Tc is the temperature of the canopy and
Tsoil is the temperature of the non-vegetated soil. Note the term FVC in this Equation is a function of LAI and sensor viewing angle
[50][44]. The original TSEB does not consider the weighting by the emissivities of each component, but some
resea
rcheuthors have claimed for its importance
[51][52][53][132,159,160].
The key of the TSEB model is the partition of sensible heat flux into the canopy and soil layers. If
we assume th
at there is an interaction between the fluxes of both components, due to a heating of the in-canopy air by heat transport from the soil, the resistance framework in TSEB can be considered to be in series
[49][54][55][56][43,161,162,163]. A parallel configuration was also introduced
[49][43], based on the assumption that the resistance of each source interacts independently with respect to the surface-atmosphere, although the canopy still has an effect on the radiation transmitted towards the soil and the wind attenuation through the canopy to the soil surface. Each component has its own SEB equation expressed as:


A variety of radiative transfer models have been implemented within TSEB to estimate R
n components. Traditional versions of TSEB estimate R
n using the physically-based radiative transfer model described in
[57][164], first implemented within TSEB by
[58][165]:
In this revised two-source model, the separation of net radiation between canopy and soil can be expressed as:
where
S is solar radiation,
τs is solar transmittance in the canopy,
αs is soil albedo,
αc is canopy albedo,
LnS and
LnC are longwave radiation for soil and canopy, respectively, and are estimated via the following expression:

where
LSky,
LS, and
LC are longwave radiation from the sky, soil, and canopy, and can be calculated from air, soil, and canopy temperature, Ω is the clumping factor, and κ
L is an extinction coefficient. Campbell and Norman
[57][164] described how to estimate the transmittance, albedo, and extinction coefficients for soil and canopy.
Soil and canopy sensible heat fluxes
H of Equations, can be deduced easily as:
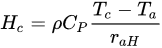
where the parameter
rs is the resistance to the heat flow in the boundary layer immediately above the soil surface, and it can be expressed as: