Non-destructive testing (NDT) is essential for evaluating the integrity and safety of structures without causing damage. The integration of artificial intelligence (AI) into traditional NDT methods can revolutionize the field by automating data analysis, enhancing defect detection accuracy, enabling predictive maintenance, and facilitating data-driven decision-making. This entry provides a comprehensive overview of AI-enhanced NDT, detailing AI models and their applications in techniques like ultrasonic testing and ground-penetrating radar. Case studies demonstrate that AI can improve defect detection accuracy and reduce inspection times. Challenges related to data quality, ethical considerations, and regulatory standards were discussed as well. By summarizing established knowledge and highlighting advancements, this entry serves as a valuable reference for engineers and researchers, contributing to the development of safer and more efficient infrastructure management practices.
NDT plays a critical role in civil engineering, allowing for the assessment of structural integrity and safety without causing damage to the tested materials. This is particularly important for large-scale infrastructure such as bridges, buildings, dams, tunnels, pipelines, and power plants. Traditional NDT methods have proven to be effective in identifying defects, assessing material properties, and guiding maintenance decisions
[1].
However, these traditional NDT techniques are often labor-intensive, dependent on the expertise of the operator, and can be prone to human error and subjectivity. Additionally, the increasing complexity and scale of modern infrastructure projects demand faster, more accurate, and scalable inspection processes. The integration of AI into NDT provides transformative potential by automating defect detection, improving accuracy and consistency, enabling predictive maintenance, and allowing for data-driven decision-making
[2][3][2,3]. This paper explores how AI enhances traditional NDT techniques by automating data analysis, improving defect detection accuracy, enabling predictive maintenance, and facilitating data-driven decision-making. It offers insights into specific AI algorithms, their applications, and the challenges faced, serving as a valuable reference for engineers and researchers interested in the intersection of AI and NDT.
Ensuring the safety, durability, and serviceability of critical infrastructure has never been more important. Failures in large structures can lead to catastrophic consequences, making accurate and timely inspections essential. While traditional NDT methods have served the industry well, the adoption of computational intelligence opens new horizons for more intelligent, autonomous, and precise inspection systems that can deliver insights far beyond what is possible through manual assessments alone
[4][5][6][4,5,6].
A search was conducted in Scopus on 19 August 2024, using the query “non AND destructive AND testing AND concrete” within the title, abstract, and keywords fields. The search returned 4737 documents with a total of 22,137 keywords. To focus on the relevant terms, the analysis set the minimum keyword occurrence threshold at 20, resulting in 542 keywords. These keywords were visualized using Vosviewer 1.6.20 (
Figure 1). This tool is used for constructing and visualizing bibliometric networks—widely used
[7][8][9][7,8,9] to analyze and map relationships within the scientific literature. The visualization generated four clusters, with 55,120 total links and an overall link strength of 245,644. The central node, labeled “nondestructive examination”, indicates that this concept is at the core of the visualization, connecting to numerous related topics across the NDT domain.
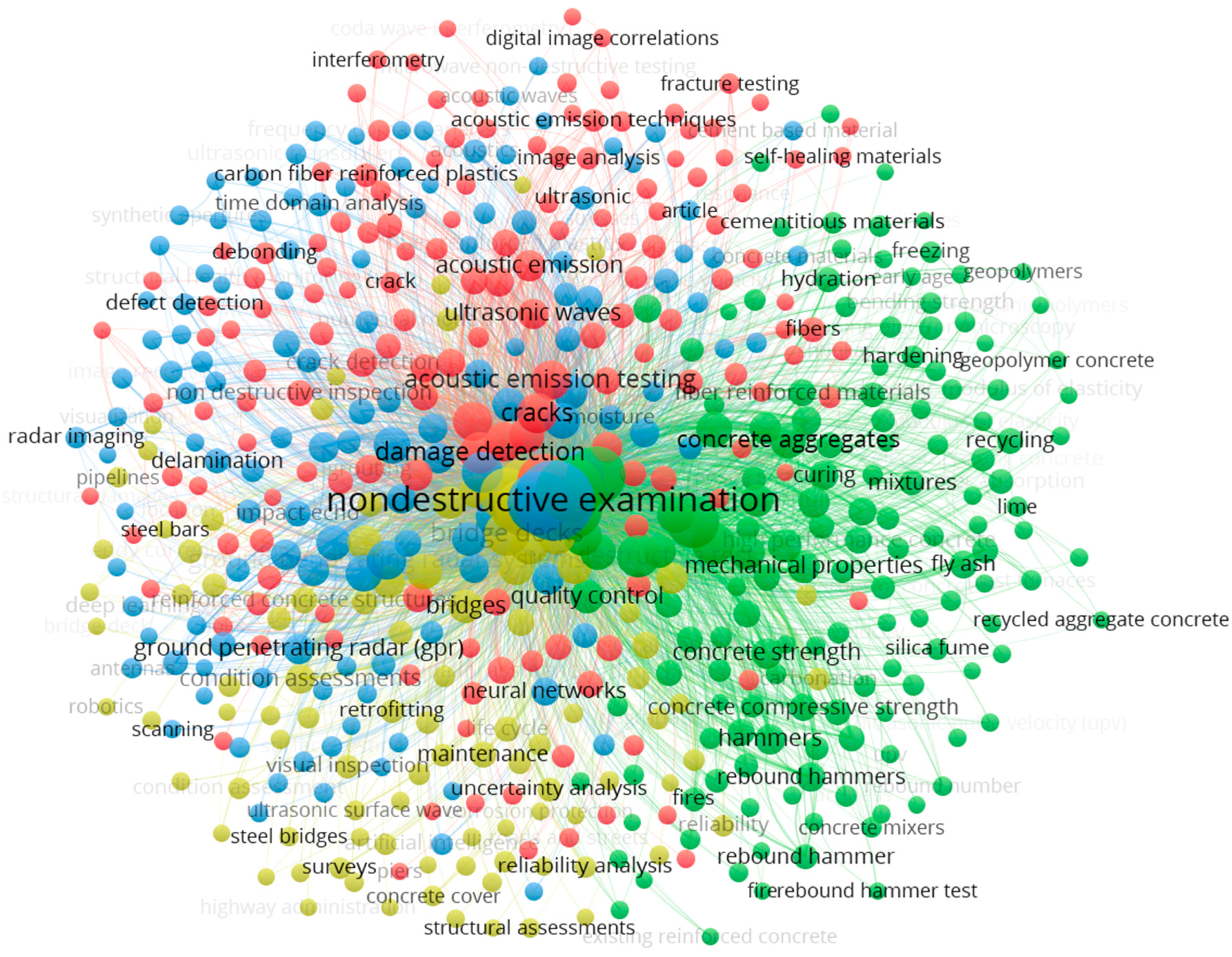
Figure 1. Keyword co-occurrence network of ‘non-destructive testing in concrete’ research generated using VOSviewer 1.6.20, illustrating relationships between key topics in NDT and highlighting areas AI can significantly impact.
The visualization is color-coded with four different clusters representing various subfields or areas within NDT. Here are some key clusters:
-
Red cluster (159 items): Material properties. This group focuses on fracture testing, self-healing materials, digital image correlations, and interferometry. These represent more specialized and emerging techniques in the NDT domain.
-
Green cluster (150 items): Mechanical properties. This section focuses on concrete-related testing methods, aggregate analysis, compressive strength, and mechanical properties. The presence of terms like geopolymer concrete, recycled aggregate, and curing mixtures suggests a focus on sustainable and advanced concrete technologies.
-
Blue cluster (133 items): Acoustic NDT methods. This cluster focuses on methods like acoustic emission, ultrasonic waves, defect detection, radar imaging, and damage detection. These are common techniques used to identify internal flaws in materials.
-
Yellow cluster (100 items): Structure quality. This section appears to emphasize infrastructure assessment and condition monitoring, including ground-penetrating radar (GPR), retrofitting, quality control, and maintenance.
The dense interconnections between clusters suggest that NDT is an interdisciplinary field that combines various methods, materials, and application areas. Nodes with thicker connections indicate key areas where multiple methodologies or topics converge, reflecting the integrated approach often needed in NDT.
Several nodes stand out due to their larger size or central position, indicating their importance, including acoustic emission testing, bridges and decks, radar measurements, and ultrasonic waves, among others. Based on visualization, topics like AI integration, digital twin technology, and smart infrastructure management are not as prominent, indicating potential areas for innovative research and application within the NDT field.