Emotion regulation is the human ability to modulate one’s or other emotions to maintain emotional well-being. Despite its importance, only a few computational models have been proposed for facilitating emotion regulation. To address this gap, a computational model for intelligent agents has been proposed for facilitating emotion regulation in individuals. This model is grounded in a multidimensional emotion representation and on J. Gross’s theoretical framework of emotion regulation. In this apporach, an intelligent agent selects the most appropriate regulation strategies to reach or maintain an individual’s emotional equilibrium considering the individual’s personality traits and specific characteristics.
1. Introduction
Over the course of human evolution, emotions have played an essential role that has allowed humans to progress as a species. Emotions play an adaptive function by preparing the individual to face new situations
[1]. They also have a motivational function that helps guide humans in their decision-making and behavior. Finally, emotions also play a social function by allowing other humans to know our state of mood. Given the importance of emotions for our evolution and individual and collective well-being, numerous studies have been carried out from the perspective of psychology, neuroscience, sociology, and lately also from artificial intelligence, to better understand the process of emotion generation and to modify or at least regulate them for our benefit
[2].
The process of emotion generation (
Figure 1) has its origin in the perception of changes in the individual or in the environment in which the individuals find themselves
[3]. This change is evaluated by the individual and an activation is produced, which involves several physiological changes. From this evaluation, and depending on the social and cultural context of the individual, the external expression of the emotion appears, and this external expression can be recognized by other individuals
[4].
Figure 1. The process model of emotion generation.
From birth, the human being begins to learn how to have some control over the emotion generated and expressed. This process is called emotion regulation
[5][6]. Emotion regulation can be informally defined as an important aspect of emotional well-being that involves the ability to manage and modify one’s or other’s emotions effectively
[7]. Emotion regulation is crucial in mental health and can help individuals deal with emotional disorders such as stress, anxiety, or depression. In addition, emotion regulation has practical applications in fields such as education, marketing, and entertainment
[8][9][10].
James J. Gross, a renowned psychologist, has contributed significantly to the understanding of this field through his development of the process model of emotion regulation
[2]. This widely accepted theory identifies different stages in the generation of emotions that can be modulated using different strategies. In his proposal, Gross establishes a framework for classifying these strategies, that facilitates a precise identification and specification of the actions involved in emotion regulation
[11].
Within the computing domain, emotion regulation is part of the broader field of affective computing. Affective computing, as an interdisciplinary domain, seeks to develop computational intelligent systems with the ability to recognize, interpret, and simulate human emotions
[12].
2. Process Model of Emotion Regulation
One of the most used and referenced model of emotion regulation is the Emotion Regulation Process Model proposed by the psychologist James J. Gross
[5][13]. This model proposes that an individual can influence various phases of the emotion generation process through the implementation of specific actions or strategies.
The model also distinguishes between antecedent-focused regulation strategies and response-focused regulation strategies (see
Figure 2). Antecedent-focused strategies for emotion regulation involve the regulation of emotions before the emotions are triggered, as noted in
[14]. These strategies can be implemented by either modifying the trigger of the emotion or altering one’s cognitive processes. On the other hand, response-focused strategies are aimed at managing the response elicited by the emotion.
Figure 2. The process model of emotion regulation.
Gross categorizes regulation strategies into different families based on the stage of the emotion generation process they influence
[5]. These families include:
-
Situation selection: Strategies within situation selection focus on deciding what situations a person faces, for example, avoiding confronting situations that evoke negative emotions such as sadness. However, to apply this strategy effectively, it is necessary to have the ability to predict the emotional response that the situation will produce, which is difficult in many situations
[11][15].
-
Situation modification: These strategies involve altering a situation to achieve a more desirable emotional response. This type of modification pertains specifically to altering the external physical environment. Sometimes, it may be difficult to distinguish between selection and modification strategies since the changes made in one situation may be perceived as creating a new situation instead
[5][11][13].
-
Attentional deployment: Strategies within this family are aimed to redirect attention between elements of the external environment or between personal thoughts
[16][17]. Distraction and concentration are the most common strategies. Distraction consists of redirecting attention from the emotional aspect of the situation to another, avoiding its emotional charge. Concentration would be its counterpart and refers to drawing attention to emotional features of a situation
[18].
-
Cognitive change: Cognitive change strategies consist in altering the individual’s evaluation or appraisal of a situation. The most commonly reported technique is reappraisal, which involves altering the individual’s internal interpretation or understanding of the situation. Another strategy is decentering, which consists of seeing an event from a broader perspective, observing one’s inner experiences as transient and separate from one’s self
[19][20].
-
Response modulation: Response modulation involves influencing the emotional response in its behavioral, experiential, or physiological components. A well documented strategy in this family is expressive suppression, which consists of inhibiting the externalization of emotional expressions. Exercise, sleep, and alcohol or drug use are also considered ways of response modulation.
3. Affective State Representation
The notion of emotional equilibrium is crucial when considering emotional stability
[21]. Emotional equilibrium denotes an individual’s natural emotional state in the absence of external or internal events. This state differs from one person to another and is influenced by factors like cognitive development, personality, and past experiences.
In order to adequately represent and reason with a person’s emotional state and detect the need to use emotion regulation, it is necessary to use an emotional state representation model. One of the most commonly used methods of emotion representation is the multidimensional model proposed by J. Rusell
[22]. That model represents emotions in a two-dimensional space, where valence is placed on the horizontal axis and arousal is placed on the vertical axis (see
Figure 3). Valence is a subjective measure of emotional experience that indicates whether the emotion is pleasant or unpleasant to the person. Arousal, on the other hand, refers to the level of activation or stimulation a person feels in response to an emotional stimulus. In addition, a pair of arousal and valence values can be associated with a category of emotions. For example, the emotion of happiness is associated with a high level of positive valence and a low level of positive arousal. In contrast, the emotion of fear is associated with a high level of arousal and a negative valence. The measurement of a person’s valence and arousal values can be estimated in several ways. Some physiological parameters, which can be measured by smart wearables like wristbands or watches, have been proven to be effective measures of the levels of arousal and valence experienced by a person in response to an emotional stimulus
[23].
Figure 3. Emotion regulation process.
4. Emotion Regulation Agent
The agent model is composed of three fundamental components. An emotion recognition process, a personalized dynamic planner and a process in charge of executing the actions focused on regulating the user’s emotion. The agent’s behaviour relies on the BDI (Beliefs-Desires-Intentions) model [24], which is a conceptual framework for modeling agents based on practical reasoning.
The affective intelligent agent interacting with an individual will detect the emotions expressed by the individual. This emotion recognition process relies on the analysis of physiological data of the individual. To minimize the impact of the physiological data collection process on the individual’s behavior, only minimally invasive techniques are considered. Among the possible techniques [25], researchers currently consider facial recognition, body temperature, and galvanic skin response.
Different people show different emotion regulation abilities. Similarly, different regulation actions will have different effects depending on the person performing the regulation action. Whenever possible, it is important to know the personality characteristics of the individual so that the intelligent agent is able to select the emotion regulation strategies that a priori are most appropriate to the personality traits of the individual. These personality traits can be obtained through personality tests. The proposal employs the Big Five personality model (Five-Factor-Model), also known as OCEAN [26][27].
Based on the emotion recognized in the individual, their personality traits, and their detected state of emotional equilibrium, the intelligent affective agent must select a set of emotion regulation actions and carry out the actions to achieve the emotion regulation of the individual and bring them back to their emotional equilibrium. However, the emotion regulation strategies proposed by Gross are not specific enough for application in a computational model. Therefore, emotion regulation strategies should be divided into more specific sub-strategies so that they can be converted into real actions to be applied by the agent and their emotional impact can be estimated.
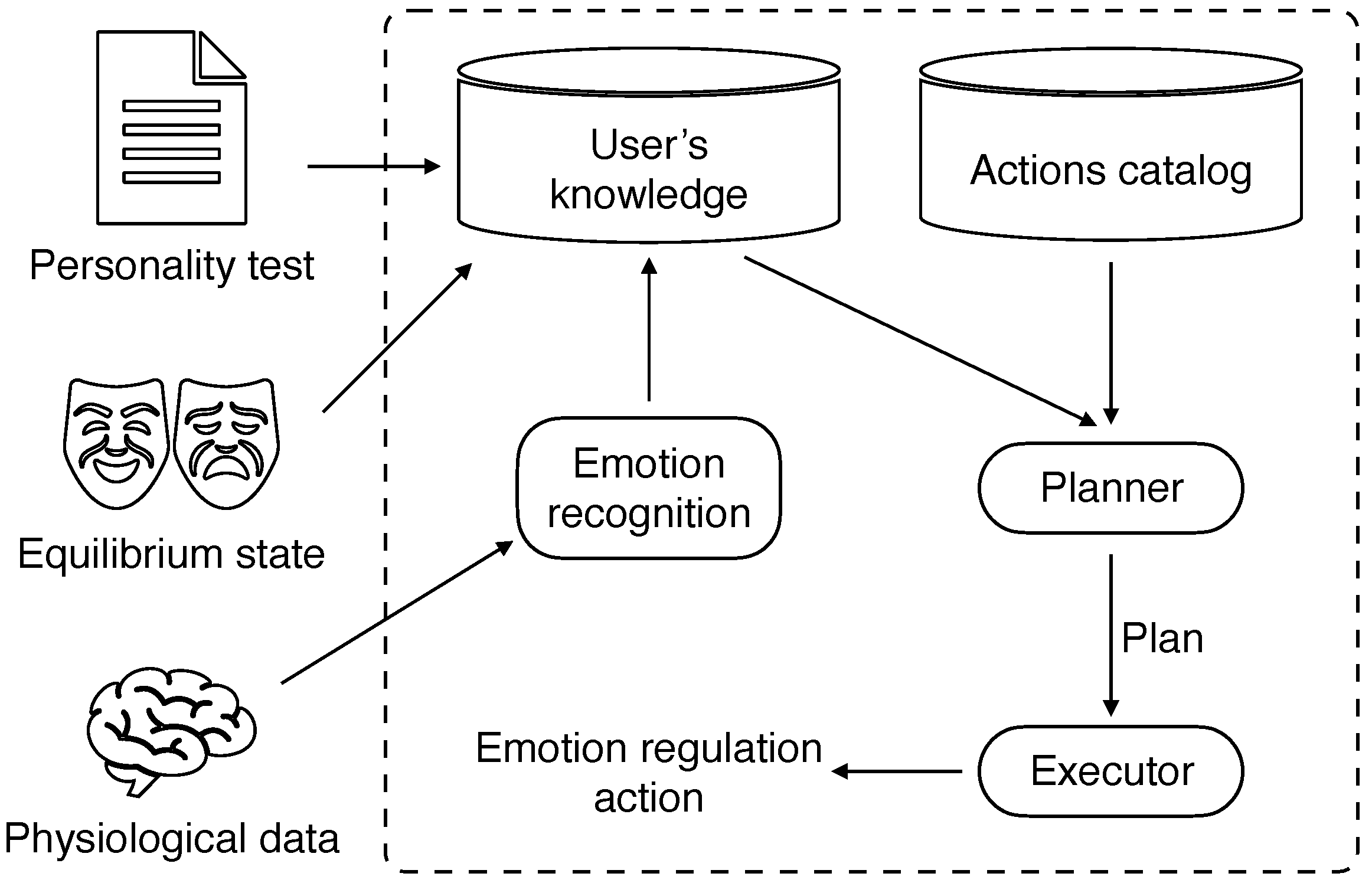
Figure 4. Emotion regulation system.
The computational model associates each emotion regulation action with the possible changes that can produce in the individual’s emotional state and the costs of applying that regulation action. The intelligent agent uses a planning algorithm to select the best strategy based on the individual’s emotional state, the effect of the regulation actions, the cost of applying each regulation action, and an equilibrium emotional state adjusted to the individual’s emotional equilibrium and personality. This is an iterative process in which a dynamic planner analyzes the actual effect that each applied emotion regulation strategy has produced in the individual until the desired equilibrium emotional state is reached.
This process, which considers general knowledge derived from personality traits as a first approximation and individualized responses observed in real-time interactions to enhance personalization to the individual, ensures that the agent continuously improves its ability to assist individuals in managing their emotions effectively, contributing to long-term emotional well-being.