Terahertz (THz) band will play an important role in enabling sixth generation (6G) envisioned applications. Compared with lower frequency signals, THz waves are severely attenuated by the atmosphere temperature, pressure, and humidity. Thus, designing a THz communication system must take into account how to circumvent or diminish those issues to achieve a sufficient quality of service. Different solutions are being analyzed: intelligent communication environments, ubiquitous artificial intelligence, extensive network automation, and dynamic spectrum access, among others.
1. Introduction
The fifth generation (5G) wireless network technology is already commercialized worldwide. The study groups and partnership projects have been designing the 5G standard since the last decade. About the key performance indicators (KPIs) set for 5G, it can be mentioned peak data rate of 20 Gbps, peak spectral efficiency of 30 bps/Hz, maximum channel bandwidth of 1 GHz, area traffic capacity of
10 Mbps/m2, end-to-end latency of 1 ms, a packet error rate of
10−5, and mobility of 500 km/h
[1]. This set has been chosen to support the following new applications: virtual and augmented reality (VAR), e-commerce, Internet of things (IoT), and enhanced mobile broadband. Compared to the fourth generation (4G), 5G has expanded the mobile broadband and added support to IoT
[2]. As a continuous evolution, the next step in wireless network technology, the sixth generation (6G), aims to at least double 5G KPIs on its deployment after 2030
[1][2].
In order to achieve 6G requirements, research groups have pointed out some solutions: terahertz (THz) band communications, intelligent communication environments, ubiquitous artificial intelligence (AI), extensive network automation, dynamic spectrum access, cell-free (CF) massive multiple-input multiple-output (mMIMO), Internet of space things (IoST), millimeter wave (mmWave) band communications, ambient backscatter communications, space-air-ground-sea integrated networks, and built-in network security
[1][2]. THz band communications deserve special attention among the other solutions due to its overarching support. THz communication possesses enough spectrum bandwidth from
0.1 THz to 10 THz. It can achieve data rates from hundreds of Gbps to several Tbps, enabling new spectrum access, connecting IoST, and aiding network automation
[3][4].
In order to understand better the contributions of THz communications, it can be important to mention some 6G envisioned applications that THz communications will support. Home wireless networks will exhibit Super Hi-Vision digital video format with a
7680×4320 resolution by sending data at a rate higher than 24 Gbps
[5]. The wireless cognition function is able to control the transmission over a communication link between a device and a base station with enough bandwidth and data rate to work as an edge server to carry complex tasks in real-time computations that encompass contextual awareness, vision, and perception
[4]. THz over fiber system is proposed to connect central stations and distributed THz wireless antennas to support high-resolution mobile multimedia services, wireless video distribution systems, and wireless local area networks (WLANs)
[6]. THz will also contribute to ultra-high-speed small cell systems, create secure wireless communication for military and defense applications, and enable advanced health monitoring and chemical attack prevention systems
[7]. Applications other than telecommunications can also be addressed using the following THz characteristics: lower single-photon energy than X-rays, high spectral resolution, and power penetration through non-metallic or non-polar materials
[8].
However, the use of THz communication is still challenging in terms of hardware and environment. Electromagnetic waves are more or less attenuated at traversing the environment according to their frequency and the atmosphere temperature, pressure, and humidity. Experiments have shown that THz waves can suffer an attenuation from 10 to
10,000 dB/km in standard atmospheric conditions
[9]. Water vapor absorbs and refracts THz waves significantly to separate a single THz pulse into two overlapping pulses after 1000 m of propagation
[10]. Human-made structures also impose an excess loss on THz communications.
Wireless communication systems seek the most negligible absorption of electromagnetic waves as they reach an obstacle. Two waves originate from the incident wave at the occasion: reflected and refracted. The thicker the obstacle is regarding the wavelength, the more the incident wave is absorbed. In
[11], two
2.92 mm thick glass layers with
1.96 mm air in between were analyzed to absorb 500 GHz waves by an absorption coefficient of 15 and a refractive index of almost
2.6. THz communications will experiment with multiple reflections in non-line of sight (NLoS) propagation, causing significant variations around the local average power. 6G systems must take those phenomena into account to be able to capture the arriving THz signal. In addition, solid-state circuits, antennas, and beamforming and beamtracking techniques still need to be enhanced to be cost-efficient and energy-efficient
[12].
To overcome the challenges mentioned earlier, one of the enabling THz communication technologies being considered is the reconfigurable intelligent surface (RIS). This is essential to smart radio environment (SRE), which signifies a wireless environment that is programmable and controllable. RISs are generally defined as an intelligent thin composite material sheet that can cover walls, ceilings, buildings, and other structures capable of modifying radio waves impinging on it in a configurable way by means of external stimuli
[13]. They are compared to mMIMO technology, except they can alter the channels’ characteristics between the transmitter and receiver. RIS can be used to create NLoS links in dead zone coverage areas, steer signals towards specific directions, suppress interference, build desired destructive interactions, increase channel capacity, enhance focus, uplift radio localization, recharge sensors, and recycle radio waves
[13][14].
2. IS in Wireless Communication
2.1. A Historical Perspective
Ancient people have already succeeded in controlling electromagnetic wave propagation. For example, the concepts of linear optics were stated more than 2400 years ago in China
[15]. With the growth of studies and dissemination of knowledge over the globe, the beginning of the twenty-first century brought breakthrough discoveries on shaping electromagnetic waves as desired. Initially, simultaneous negative electric permittivity and magnetic permeability responses were achieved
[16]. In addition, the negative index of refraction was verified experimentally
[17].
In
[18], the authors presented an experimental high-gain reconfigurable sectoral antenna that uses an active cylindrical FSS structure to obtain a directive radiation pattern. The structure was made of metallic discontinuous strips with positive-intrinsic-negative (PIN) diodes in their discontinuities and placed cylindrically around a dipole. Switching the diodes on the structure allowed a 360° radiation pattern sweep. In other words, the transmission and reflection coefficients of the FSS structure could be controlled by the state of the diodes.
Digital metamaterials, containing
30×30 particles, were proposed in
[19]. Their metamaterial particles were two planar symmetrical metallic structures printed on the top surface of the F4B commercial dielectric board substrate and joined by a PIN diode. The total dimension of the particle was
0.172×0.172×0.057𝜆3 at the central frequency of
8.6 GHz. Applying a biased voltage of
3.3 V to the diodes, the particle behaved as a “1“ digitally coded material. When there was no biased voltage, it behaved as a “0” digitally coded material. The tests showed that the phase response difference between those states was exactly 180° at
8.6 GHz. The authors in
[19] were able to alter the scattering pattern of normally incident beams on the digital metamaterial from being directly reflected to being reflected in two different directions, forming an angle of approximately 60°.
Based on the modeling and design of tunable reflect-array elements in
[20], Tan et al.
[21] constructed smart reflect-arrays that were hung on the walls of a room to enhance the indoor spectrum sharing capacity by changing the phases of the reflected signals and changing the spatial distribution of the signal strength. The experiment showed that the interference was canceled to
−73 dBm, and the signal-to-interference-plus-noise ratio (SINR) was increased to about 30 dB.
A programmable metasurface capable of modeling incident electromagnetic waves polarization, scattering, and focusing was proposed in
[22]. Using a digital metasurface similar to the one in
[19], the authors fabricated a prototype with 1600 metamaterial particles, obtaining an aperture size greater than 20 wavelengths. With coding matrices being used to control the PIN diode states of each particle, they obtained reconfigurable polarization conversion, scattering, planar focusing, beam steering, and beam forming.
LIS was proposed in
[23] as an extension of mMIMO systems. Assuming that an entire surface is used as an intelligent receiving antenna array, the area is sufficiently large, and matched-filtering is employed, the authors derived the channel capacity for three different positioning scenarios of the terminals communicating with the LIS: along a line, a plane, and a cube. The conclusion was that the inter-user interference of two users at the LIS is close to a sinc-function. The inter-user interference is negligible as long as the distance between two users is larger than half of the wavelength. The equivalence between the LIS continuous surface model in
[23] and the discrete dense grid of antennas model was assessed in
[24] and corroborated in
[25].
Metasurfaces incorporating networked hardware control elements were extensively detailed in
[26]. They are called hypersurfaces tile-coat environmental objects and communicate with existing systems, such as localization services and cloud computing, to create the optimum wave scattering and combat path loss and multipath phenomena. The researchers elaborated on the first model to describe programmable wireless indoor environments, focusing on hardware, networking, and software components. They also evaluated the potential of programmable environments via a full 3D ray tracing in
2.4 and 60 GHz cases.
2.2. IS Architecture
An IS is a flat structure made of specific materials, which are the base of the IS classification. Copper and graphene have been used. Researchers in wireless communications have focused on two main implementations: antenna-array-based and metasurface-based structures. The radio waves impinging on these electromagnetic material surfaces can be reconfigured to adjust the wireless propagation environment. By controlling the phase shifts and wavefront, the waves are reflected to propagate toward their desired directions. By making the wireless environment programmable and controllable, these surfaces monitor the propagation to improve the efficiency of the received signal.
The different types of IS found in the literature include active and passive surfaces, denominated according to the capacity to perform operations on the incident radio waves. In addition, ISs whose functions can or cannot be modified after manufacturing or deployment are denominated dynamic and static surfaces, respectively.
Figure 1 depicts the IS architecture shared among most technical papers in the literature. A top-down IS perspective view can be seen as a thin composite material sheet controlled electronically. Composite means that the planar structure is typically made of three layers. The reflecting elements constitute the outer layer, and the inner layer is a control circuit board responsible for adjusting the reflection amplitude and phase shift of the IS through feeding lines and via holes. The middle layer is a conductor between the outer and inner layers to avoid signal energy leakage. The IS controller is used for both programming the surface response and interacting with other network elements, such as base stations (BSs), APs, and user devices. Most prototypes have used field programmable gate array (FPGA) as the IS controller.
Figure 1. Example of IS architecture.
From a bottom-up perspective, the IS outer layer comprises multiple reflecting elements, or meta-atoms, of electric thickness. Electric thick means this reflecting element dimension is in the order of the sub-wavelength of the operating frequency. The meta-atom signal phase response is modified according to the electronic device states embedded in it. Devices used in practice include PIN diodes, field-effect transistors (FETs), micro-electromechanical systems (MEMSs) switches, and microcontrollers. Applying a biasing voltage to the feeding line energizes the reflecting element and the electronic devices through the via hole. The combination of the electronic device states defines the phase shift pattern. The meta-atom signal reflection amplitude can be controlled via a variable resistor in the element design. Varying the element resistance dissipates distinct portions of the arriving signal energy.
Finally, prototypes have combined iterative algorithms, integrated with the IS controller, to pursue the optimum configuration of each reflecting element, therefore achieving an efficient operation of the IS in accordance with the application. Such algorithms have also been used to alter the IS element states cyclically so that the reflected signal power is distributed harmonically.
2.3. IS Operation
Each IS surface element is considered the same size, i.e., they share the
Δ𝑥 width and
Δ𝑦 height. Also, they contain
N PIN diodes assembled on dielectric substrates that connect the reflective element to the ground when the via hole is electrically charged. The IS element impedance is an association of all layers impedance
[27].
As the IS controller applies a bias voltage to the feeding lines, the IS element is energized across the via hole, and the PIN diodes change their states between “on” and “off” accordingly. The point in incorporating PIN diodes is to adjust the IS element frequency response dynamically
[28].
Figure 2 shows the equivalent circuit for the IS element and both PIN diode states. For a horizontally polarized incident wave, the ground bars around the IS element central patch act as inductors horizontally
[29]. With the PIN diode insertion on the IS element, its reverse bias or forward equivalent circuits can be applied to estimate the transmission coefficient and 3 dB cut-off angular frequency of the IS element.
Figure 2. IS element and PIN diode state equivalent circuits.
The combination of the N PIN diode states leads to distinct phase shifts for each IS element. Figure 3 illustrates a signal reaching an IS k-th element. As the signal arrives at the IS k-th element within a direction ξA(k)=(θA(k), ϕA(k)), the IS k-th element PIN diode states redirect the corresponding reflected or transmitted signal by a phase shift ψ(k), such that the departure direction is ξD(k)=(θD(k), ϕD(k)).
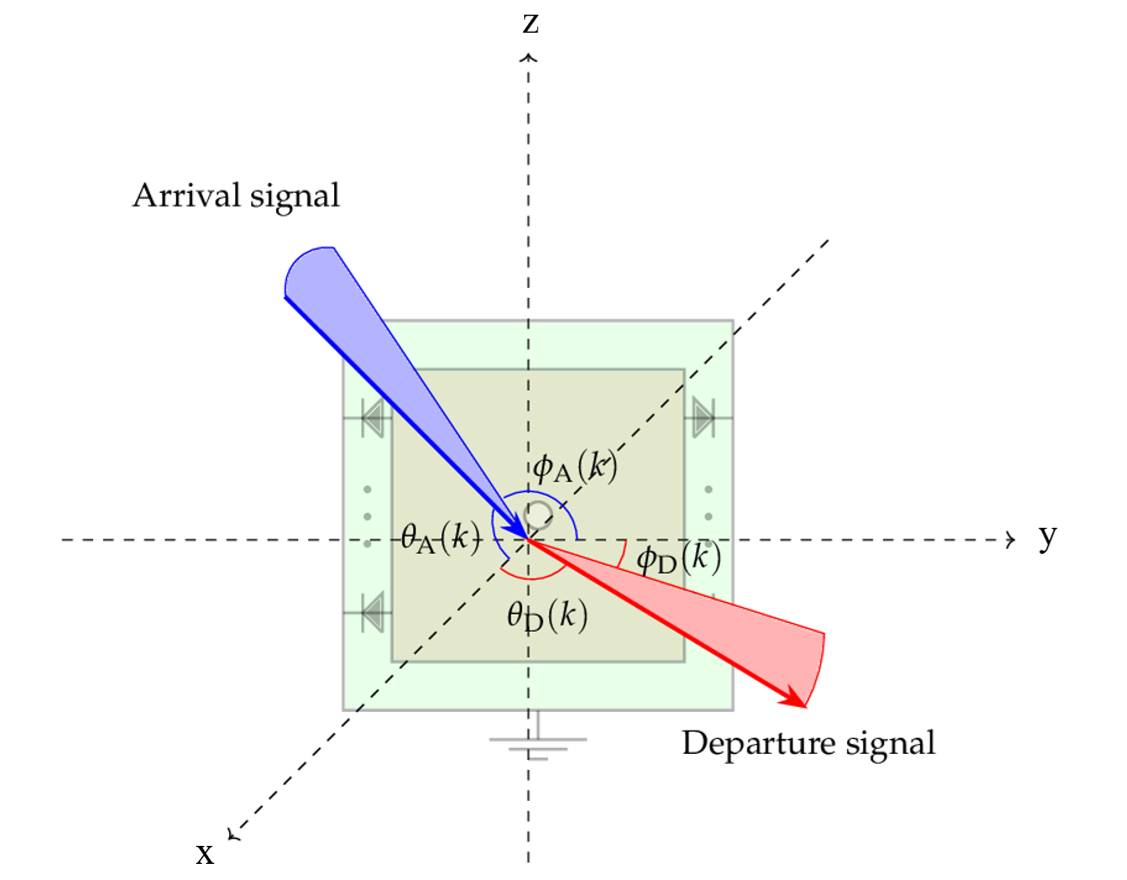
Figure 3. Angles of arrival and departure for a signal reaching the IS k-th element.
3. THz Channel Modeling
The THz frequency bands beyond 100 GHz and below 10 THz comprise a still largely unexplored part of the electromagnetic spectrum. They can adequately meet the demands of the 6G networks due to their multiple gigahertz of bandwidth
[4]. This multi-gigahertz bandwidth opportunity can increase the number of users, provide signal location and detection, and satisfy some of the requirements of the next generation of wireless networks. As a result, THz communication will allow higher data rates with lower latencies
[30]. Despite their large bandwidth, THz communication suffers from strong atmospheric attenuation, molecular absorption, and severe path loss, limiting its propagation
[31]. These drawbacks make the implementation of a THz communication system very challenging.
It can be said that part of the most substantial restrictions regarding the design of a communication system is imposed by the channel through which the signal is transmitted
[32]. Thus, the increase in frequency requires an analysis of the best-explored propagation routes. Furthermore, the increasing demand for efficient communication services has led devices to operate in a system composed of different channel environments
[33]. In this context, the adequate modeling of the wireless channel is essential, mainly in the THz band.
The channel models in THz can be classified as statistical, deterministic, and hybrid, depending on the number of antennas implemented
[34]. This study exploits the deterministic and statistical models of single-input single-output (SISO) and MIMO systems.
3.1. Deterministic Channel Model for THz Band
Generally, the two deterministic models found in the literature are the ray-tracing (RT) and the finite-difference time domain (FDTD) models
[35][36][37][38]. These deterministic channel models are based on the theory of electromagnetic waves. However, their properties depend on the materials, propagation medium, and spatial positions of transmitter and receiver antennas. In this subsection, the RT is explored firstly and the FDTD secondly.
Wireless communication channels are well-known to be subjected to the multipath phenomenon
[39][40]. This phenomenon occurs when the transmitted radio signal finds multiple paths between the transmitter and the receiver. However, its occurrence is connected to the propagation environment and the obstacles between the transmitter and receiver.
Figure 4 shows from a pictorial perspective the main propagation phenomena.
Figure 4. The ray-tracing method for deterministic channel modeling.
As mentioned before, the RT technique analyzes the propagation of electromagnetic waves for each of these geometric paths. The absorption by water vapor molecules is one of the main factors that affect the propagation of waves in the THz band
[41]. In addition to introducing thermal noise, absorption causes an attenuation of the signal sent to the receiver. As occurs in the most explored bands, also in the THz band, the propagation in free space must consider the propagation loss. Complete ray tracing models were developed for 0.06–10 THz
[35] and
0.3 THz
[36] frequency bands, respectively.
The LoS channel transfer function can be represented by
where is a spreading loss function, is a molecular absorption loss function, 𝜏LoS is the delay and 𝑓 is the observed frequency.
The channel transfer function of the reflected rays can be written according to
in which c is the speed of light, is the time-of-arrival of the reflected ray, 𝑟1 is the distance between the transmitter and the reflector, 𝑟2 the distance between the reflector and the receiver, is the reflection coefficient, and 𝑘(𝑓) is the absorption loss. The channel transfer function of the scattered ray propagation is
where is the time-of-arrival of the scattered ray, 𝑠1 is the distance between the transmitter and the scattering point, 𝑠2 is the distance between the scattering point and the receiver, and 𝑆(𝑓) is the scattering coefficient. Finally, the transfer function of the channel in the diffraction ray can be written as
in which
is the time of arrival of the diffracted ray,
𝑑1 is the distance between the transmitter and the diffracting point,
𝑑2 is the distance between the diffracting point and the receiver, and
is the diffracting coefficient.
3.2. Modeling of Statistical Channels
The previously presented deterministic models provide accurate channel modeling results at the cost of high computational complexity. As a result, elaborating deterministic channel models can be far too time-consuming
[42]. In addition, geometric knowledge of the propagation environment is required for those models. Statistical propagation models were proposed and designed to overcome those limitations, and numerous models are found in the literature. Some common models are the Rayleigh
[43], Nakagami
[44], and Rice
[45] models. However, in general, they are used to represent narrowband fading and do not provide a complete analysis of the physical phenomena that occur in the THz band.
For fast channel realizations, a statistical impulse response model for the range 275–325 GHz was presented in
[46]. The proposed approach includes fully polarimetric path amplitudes, amplitude-frequency dispersion, phases, direction of departure (DoD), direction of arrival (DoA), time of arrival (ToA), propagation phenomena, and antenna characteristics, and is suitable for multi-antenna systems. The authors conducted RT simulations for 3201 frequency points and then compared data histograms with analytical distribution models. Based on the results obtained by the authors, they provided parameter values that can be used for a universal stochastic spatiotemporal model to generate ultra-broadband THz indoor radio channel realizations.
4. IS-Aided THz Channels
IS has been considered a promising technology and an indispensable part of THz communication systems. As channels in the THz band are highly frequency-selective, IS applications in these frequencies can solve the limitations on propagation distance and coverage, which are easily affected in THz frequencies due to very high propagation attenuation and molecular absorption
[41]. In addition, it can provide an additional propagation path where NLoS link exists and, consequently, assist the communication where the LoS path is blocked by obstacles
[14].
In addition to creating additional links between the transmitter and receiver, the ISs also use wireless mm-wave intercell communication for tunability. The IS architecture is based on FPGAs, which are responsible for enabling the control and intelligence of IS. To transfer information among unit cells, wireless signals not leaked outside the IS, and no interference with the network, frequency and time domain and path propagation analysis have been performed
[47][48][49]. The thorough analysis of the authors has provided that wireless communication to control the IS is an optimal choice over wired communication for large or dense IS employments.
Moving from the perspective of the IS controlling channel to the one used to enable communication,
Figure 5 shows a typical application scheme of an IS for THz communication. As shown, User 1 desires to connect to the BS; thus, the system requires a LoS link between User 1 and the BS. Nonetheless, the wireless link is blocked by obstacles. In a traditional approach, the User 1 would try to find another BS with a weaker signal due to the longer distance from User 1. Also, high path loss bounds THz waves to a short propagation range
[50]. However, there is an IS with a total
𝑚=𝑚𝑥𝑚𝑦 elements, where
𝑚𝑥 accounts for the number of elements in each row and
𝑚𝑦 accounts for the number of elements in each column. Due to the IS installation position, the signals from the BS can be transmitted to User 1 by reflection from the IS. The changes in directions of the reflected beam are controllable, according to the generalized Snell’s law, which is derived from Fermat’s principle to interpret the anomalous reflection and refraction phenomena
[51]. This means that when the IS elements alter the phase and magnitude of the incident waves, occurs constructive or destructive interference of these incident waves with the reflected waves from IS in desired directions. Each element introduces a phase shift, ideally given by the generalized Snell’s law to create a single beam. Still, the phase shift must be explicitly optimized since a superposition of multiple beams should be created.
Figure 5. Example of communication with IS in THz band.
The information sent to the desired user in
Figure 5 must be received only by User 1. Therefore, the information-bearing signal and the reflected signal constructively interfere, and consequently, a constructive reflection is sent toward the desired user (User 1). There is also an interfering user (User 2) that must not participate in the communication between User 1 and the BS. Therefore, destructive interference occurs between the waves that are incident on IS and those that are reflected in the direction of User 2. In this case, there is no communication path between User 2 and BS, so that information remains secure with the desired user. As ISs can reduce the propagation loss with intelligent reflections in order to enhance the end-to-end system performance
[52], channel modeling strategies for IS-aided communications systems are fundamental to provide detailed channel characteristics.
In that context,
[53][54] evaluated the system performance when the LoS path from the transmitter to the receiver in a downlink THz indoor wireless communication is blocked. The system performance under the phenomenon of the receiver antenna beam misalignment was evaluated in terms of average SNR, ergodic capacity, and OP in
[53]. The phase shift that each IS element must impose to steer the radiated beam towards the receiver in terms of the channel attenuation and its cumulative distribution function are presented in
[54]. The study in
[53] showed that receiver beam misalignment deteriorates the system performance, but increasing the IS size improves overall system performance. The authors in
[54] showed the importance of considering the molecular absorption loss for IS-aided THz channel estimation and that there is a minimum transmitter power that can guarantee a high coverage probability.
The system was modeled similarly to
Figure 3 with the IS placed on the origin of
x-
y plane, the dimensions of the IS elements, denoted by
𝑑𝑥 and
𝑑𝑦, were in the range
[𝜆/10,𝜆/2] [55], and the transmitter and receiver located at distances
𝑑1 and
𝑑2 from the IS center, respectively. The arrival and departure angles of the signals from the transmitter and to the receiver in relation to the IS center, respectively, were then determined. Based on these assumptions, the resulting expression for the channel gain
|ℎ| at the receiver is given as
[53][54]
in which
|𝑅|,
𝜏(·,·),
𝐹𝑡,
𝐹𝑟,
𝐺𝑡,
𝐺𝑟,
𝐺IS(·,·),
𝜃𝑟,
𝜙𝑟, and
𝜖𝑟 stand for the amplitude of the programmable reflection coefficient of each IS unit cell, transmittance of the absorbing atmospheric medium
[56], transmitter normalized power radiation pattern, receiver normalized power radiation pattern, transmitter antenna gain, receiver antenna gain, an IS unit cell gain, receiver antenna bandwidth, angle of boresight direction, and receiver beam steering error angle, respectively. The IS unit cell gain
𝐺IS(·,·) is calculated as
[53]
in which , and 𝑏𝑟 represent the unit step function, forward-to-backward power ratio, and an antenna specific constant.
Physically, it can be seen from the previous expressions that each IS unit cell impacts the channel gain at the receiver by means of their normalized received power ratio, aperture, phase shift, and reflection coefficient (see Appendix A in
[54]). THz channels impairments are mainly represented by the transmittance of the absorbing atmospheric medium. Stochastically, the channel gain at the receiver is interpreted as the summation of the order of the IS size of products of channel coefficients between the transmitter and the IS and the IS and the receiver
[52]. Mathematically,
in which
N,
ℎiTx,
𝑔𝑖,
ℎiRx are the number of IS elements, transmitter to IS channel coefficient,
i-th IS element response, and IS to receiver channel coefficient. All components on the right side of (
8) are composed of amplitude and phase, and researchers consider the IS controller knows the channel, producing phase shifts that cancel out the phase response of the channels
[57]. In addition, IS are assumed to work passively and have unitary amplitude. The different probabilistic channel models used to investigate IS-aided THz channels have been mixture Gamma
[58], Rayleigh
[59][60], Rice
[61][62], Nakagami
[61][63][64][65], and shadowed
𝜅-
𝜇 [66] models.
Due to the intelligent reflections, an IS-aided communication has dynamic control capability over the wireless propagation channel phenomenon. This IS capacity to change propagation characteristics of wireless channels promotes the development of the THz communication systems to meet the ever-increasing user demand for higher data rates. The benefits of the IS applications in the next-generation wireless technologies depend on the development of an accurate channel model, which is necessary for designing and deploying future wireless communication systems.
To enable the efficient transmission of THz waves, which suffer because of high path loss, the IS operation in THz wireless communication links has been analyzed for the proposed path loss models. For instance, the study in
[67] employs electromagnetic theory to deduce the expression that returns the phase shifting of each IS reflection unit.
Table 1 compares the related articles and explores some aspects of the THz communication channels. The topics cover channel modeling characteristics at THz band, including performance evaluation models, system setup for performing channel design objectives, and channel optimization techniques.
Table 1. Comparison of recent research works regarding IS on THz wireless communication channel modeling in terms of performance evaluation, system model, design objective, and optimization technique.
Reference |
Performance Evaluation |
System Model |
Design Objective |
Optimization Techniques |
[68] |
Path gain and capacity |
D–band indoor downlink |
Maximize signal reflection and restore LoS link between the transmitter and receiver blocked by obstacles |
Antenna theory |
[69] |
Adaptively selecting step size |
Indoor MIMO downlink |
Spectral efficiency with phase shift adjust |
Taylor expansion aided gradient descent |
[67] |
Path loss |
THz systems downlink |
Expression that determines the optimal phase shift |
Electromagnetic theory |
[70] |
Path loss |
MIMO |
Power gain and energy efficiency |
Beamforming |
[71] |
Beam pattern and quantization error |
mMIMO |
Channel estimation and transmission solutions with hybrid beamforming architectures |
Geometric channel model and IAP-SP for CSI acquisition. |
[72] |
NMSE |
Indoor MIMO systems |
Channel estimation |
Beam training |
[73] |
NMSE |
MIMO |
Channel estimation |
IAP-SP scheme |
[74] |
SNR feedback |
MISO downlink |
Minimization transmit power while maximizing the system achievable rate |
Beamforming optimization based on statistical CSI and genetic algorithms |
[75] |
Success rate and beamforming gain ratio |
mmWave/THz downlink |
Perfectly aligned transmitter-receiver channel |
Efficient beam training with cascade channel |
[76] |
Secrecy rate |
mmWave/THz systems |
Optimal discrete phase shifts to maximize the secrecy rate |
SDP-based method and the element-wise BCD method |
[77] |
Secrecy rate |
MISO downlink |
Secure operation with secrecy rate maximization |
Active beamformer and passive reflecting phase shifters |
[78] |
Path loss |
Not detailed |
Intensity for the electric field reflected by RISs in the short and long transmission distance regimes |
General scalar theory of diffraction and the Huygens-Fresnel principle |
[79] |
SNR |
Not detailed |
Improve the average receive SNR |
Beamforming |
[80] |
SNR, SNDR, small-scale amplitude fading, OP, and ergodic capacity |
THz system |
Exact PDF and CDF expressions of end-to-end SNR and SNDR of the system |
FTR distribution and multivariate Fox’s H-function |
[81] |
Path loss and beam pattern |
THz systems |
Beamfocusing |
Beamforming with physical optics channel model |
[82] |
Beam pattern |
THz massive MIMO systems (downlink and uplink) |
Beamforming design and channel estimation performance |
Beamforming with physical channel model |
[53] |
SNR, ergodic capacity and OP |
THz indoor systems downlink |
Receiver beam misalignment and restore the transmitter/receiver connection blocked by obstacles |
Electromagnetic theory |
[83] |
UAV trajectory, phase shift, THz band allocation and power control |
UAV systems downlink |
To maintain reliable THz communication and minimize average rate of users |
SCA |
[84] |
Energy-efficient, SNR, latency and success rate |
3-D indoor THz wireless communication |
Minimizes latency using ray tracing techniques to find the best THz signal propagation path |
Ray searching and beam-selecting |
[85] |
Achievable rate, phase compensation and normalized array gain |
IS-aided THz system |
Achievable rate maximization for distributed IS-assisted THz communications |
Analog beamforming, digital beamforming vector |
[86] |
Phase shifts, ergodic net throughput, blocking probability |
CF mMIMO |
Channel estimation and unblock links |
OP and fading spatial correlation with increased element numbers |
[87] |
Misalignment fading |
THz inter-satellite links |
Compensation for the high path loss associated with high carrier frequencies and to improve SNR |
Antenna theory |
[88] |
Coverage area and sum rate |
mmWave or THz indoor communication |
Placement optimization to maximize the long-term sum rate and then optimization of transmit beamforming and reflecting procedure in real time |
Antenna theory and deep learning |
[89] |
BER and modulation speed |
Full-wave simulation |
Eliminate on-chip signal attenuation and inter-symbol interference |
Binary IS optimization algorithm |