1. Energy Storage Resources
1.1. Conventional Energy
The world’s primary conventional energy source is non-renewable sources such as fossil fuels. The electricity required by many of the world’s largest power plants, factories, and industries still depends heavily on this conventional energy source. According to the reference
[1], about 85% of the global energy needs are met by fossil fuels. These fuels have disadvantages such as damage to the environment and human health due to phenomena such as climate change. On the other hand, these resources are exhaustible, and the level of reserves of this fuel is low
[2]. Hence, researchers are looking for alternative sources of fossil fuel that create less environmental burdens to the environment.
1.2. Renewable Energies
Unlike conventional energies, renewable energies are inexhaustible and lead to polluting the environment. In addition, these clean energies are always accessible for free. These benefits of renewable energy have made this green energy the best and most suitable alternative to conventional energy and fossil fuels, and they have also attracted the attention of many energy investors and governments around the world
[3][4]. Since these renewable sources are unpredictable and intermittent, the presence of energy storage units in power generation systems from renewable energy sources, such as batteries and fuel cells, is necessary for the power generation system’s stability
[5].
These energy storage units are comprehensively reviewed in the next section. Solar and wind energy have been extensively studied among all the renewable energies. Researchers consider using more than one renewable energy source integrated with energy storage to be the best way to increase the sustainability of power generation systems from renewable sources
[6]. Because renewable energy has very variable amounts at different times, or in other words, has low reliability, researchers have introduced and used different methods to determine the reliability of power generation systems from renewable energy sources, which will be discussed in detail in the following sections. In modeling and designing a clean power generation system equipped with energy storage and considering its reliability, the economic aspects of this system should also be considered. A green power generation system is economical when the size of each of its components is optimized. Researchers propose and use various techniques and algorithms to optimize the size of this power generation unit, which will be reviewed
[7][8][9][10].
2. Energy Storage Technologies
2.1. Battery Storage System
Battery storage banks have been one of the oldest human tools for storing chemical energy. These storage devices are classified into two types: rechargeable and disposable. The rechargeable batteries was considered. These batteries are used in most off-grid green microgrids. The easy access, uncomplicated structure, easy application, and high flexibility of rechargeable battery banks have made them the most widely used type of energy storage. Batteries do not emit pollutant emissions during operation. Another advantage of this energy storage is its high response speed for transmitting electrical power required to power-consuming units. This advantage of batteries means that the power generation system does not face the problem of not responding to the demand load and works stably without interruption. According to research, the battery conversion efficiency is 60–90%. There are various types of battery banks. The most widely used are lead-acid batteries, lithium-ion batteries, sodium-sulfur batteries, and flow batteries. Each of them will be introduced in the following section.
2.1.1. Lead-Acid Batteries
Lead-acid batteries have the same structure as other batteries, meaning that they use two positive and negative electrodes and a separator. The positive electrode consists of lead dioxide, and sulfuric acid is used as an electrolyte and separator of the two electrodes. Sulfate ions supplied by this electrolyte are used to react when the battery is discharged. The following equation shows the chemical reaction of this type of energy storage.
2.1.2. Lithium-Ion Batteries
Lithium-ion batteries, like other batteries, are responsible for storing energy. The positive electrode of these batteries is lithium, and the negative electrode is carbon or graphite. The electrolyte of this type of battery is made of non-aqueous materials such as propylene with lithium salts because lithium reacts very strongly with water. The separators used in the cells of this battery are also fine porous plastic films.
Lithium cobaltite (LCO) is often used as a positive electrode component in lithium-ion batteries. However, other compounds store energy in these batteries in off-grid microgrid applications because it is expensive. These compounds include a combination of oxides of nickel, cobalt, and aluminum (NCA), nickel, cobalt, and manganese (NCM), which are cheaper than lithium cobaltite (LCO). Its negative electrode is mainly composed of carbon or graphite. Lithium-ion batteries are often used in electric vehicles. Since these batteries have a high energy density and a flammable electrolyte, cell construction, charging system, etc., must be done carefully
[11]. These batteries’ advantages are long cycle life, wide operating temperature range, and high energy density
[12].
2.1.3. Sodium-Sulfur Batteries
A sodium-sulfur battery, like other batteries, consists of two electrodes, in which the positive electrode is in the liquid sulfur electrolyte, and the negative electrode is in the liquid sodium electrolyte. A solid beta-aluminum ceramic electrolyte separates the two electrodes. Positive sodium ions combine with the sulfur in the electrolyte to form sodium polysulfide. For a more suitable transfer of sodium ions, the cell temperature should be 300–350 degrees Celsius. To provide this high temperature, these batteries use their stored energy. The efficiency of these batteries is over 90%, and they have no automatic discharge. The energy density of sodium-sulfur batteries is much higher than the energy density of lead-acid batteries.
2.1.4. Flow Batteries
The flow battery consists of electrolytes, membranes, and positive and negative electrodes. Membranes separate the positive and negative electrodes of the flow energy storage. This battery has a high capacity for energy storage, and its different types are vanadium redox battery (VRB), polysulfide bromide battery (PSB), zinc bromide battery (ZnBr), etc.
These batteries are often used in off-grid microgrids, and the most critical issues to consider when using these batteries are cost, reliability, longevity, and depth of discharge
[13][14]. Vanadium redox batteries (VRB) have unlimited capacity and are expensive. These flow batteries are mainly used for energy storage in large power plants
[15][16][17]. Among the advantages of these batteries are the inherent scalability of their capacity and long-term storage of charge
[18]. However, the major disadvantage of these batteries compared to other technologies is that the power/energy density is relatively low
[19].
2.2. Supercapacitors Energy Storage
Another type of energy storage is supercapacitors. In this energy storage, two-layer plates are used to separate the loads from each other. These batteries have a very long life cycle and are suitable for places that require high power and fast response speed. For reliability and safety issues, the voltage of the supercapacitor module is considered in the range of 200–400 volts
[20]. The main advantages of supercapacitors over batteries are very specific power and their number of charge-discharge cycles
[21]. However, supercapacitors’ energy stored per unit weight than batteries is considerably lower
[22]. Because its energy density is very low that can be improved by increasing the capacitance
[23].
2.3. Flywheel Energy Storage
This energy storage stores the kinetic energy caused by the rotation of the disks around an axis. The energy stored in this energy storage is directly proportional to the square of the moment of inertia of the disk and the wheel’s speed. When the consumer needs power, the flywheel energy store uses disk or rotor inertia to convert the stored kinetic energy into electricity and meet the required power
[13]. Flywheel energy storage is used as a backup system for power supply and voltage, transportation and so on. To store higher powers, it needs larger wheels. Depending on the number of losses in different parts of this energy storage, its efficiency is set between 95–90%
[20]. Relatively high efficiency and fast response time (milliseconds) are the advantages of this energy storage
[24][25]. Despite these benefits, compared to batteries and supercapacitors, flywheel energy storage systems have maintenance effort, lower power density, noise, cost, and safety concerns
[21].
2.4. Hydrogen Storage
Hydrogen is obtained by the electrolysis of water using electrical energy in a clean method. Hydrogen is produced at low pressures (30–00 PSI) and stored in tanks at high pressures (3000 PSI). The efficiency of these energy storage devices is about 40–60%. There are several ways to produce hydrogen with fuel cell technology, including solid oxide fuel cell, direct methanol fuel cell, molten carbonate fuel cell, and metal-air battery
[20]. It should be noted that hydrogen storage has many inherent advantages such as potentially high energy storage densities, ease of disposal, and the absence of toxic components
[26].
2.5. Potential Energy Storage
Potential energy storage is used in pumped storage hydropower (PSH) and compressed air energy storage (CAES) systems
[27]. Briefly, PHS is a technology based on pumping water to an upstream reservoir during off-peak or the times that there is surplus power electricity by renewable energy. When electricity is needed, it releases energy through the hydro turbines
[28]. Scarcity of available sites for two large reservoirs, long lead time, high cost for construction, and environmental issues are major drawbacks of PHS
[29]. CAES has been proposed as an alternative to pumped hydro storage for large-scale. In a CAES, surplus power compress air by a rotary compressor and then store it in an underground chamber. It is released from the chamber and passed through an air turbine that generates electricity from the flow of high-pressure air when the power is required
[30]. However, the major disadvantage of CAES is their dependence on geographical location
[31].
2.6. Thermal Energy Storage
It simply dissipates into the environment necessitating fossil fuel combustion if the thermal energy is not stored. Thermal energy storage (TES) is known as one key function in thermal energy management
[32]. In fact, it is a technology that, by cooling or heating a storage medium, stocks thermal energy to store energy and is used later for power generation and cooling and heating applications
[33]. A wide variety of materials are being used for TES that must possess suitable thermophysical properties such as favorable melting point for the given thermal application, high thermal conductivity, high specific heat, and high latent heat. The type of TES material being selected for cold or heat storage classifies TES systems into (1) sensible heat storage systems, (2) latent heat storage systems, and (3) chemical heat storage systems. Sensible heat storage materials are thermally stable and thus have most used for high-temperature applications. Moreover, they are usually low-cost. However, they suffer from temperature stability during the discharge process. Near the phase change temperature, the energy storage density of latent heat storage materials is very high. Because latent heat is 50–100 times larger than sensible heat. However, latent heat storage materials are poor thermal conductivity. Chemical thermal energy storage has the highest density with low heat losses; however, they are still in the laboratory stage. Most commonly TES materials are water, inorganic salts, thermal oils, sand, gravel, and paraffin
[32].
3. Energy Storage Modeling
3.1. Economic Analysis
The economic criteria of the network-independent power generating system must be properly modeled and analyzed to produce a cost-effective solution. Energy savers are a member of this clean power generation system, and their role in modeling and economic evaluation of these power generation systems is critical. To evaluate and learn the economics of renewable energy power production systems, researchers now employ effective and generally accepted economic methodologies and criteria such as total life cycle cost (TLCC), life cost of energy (LCOE), Internal rate-of-return (IRR), Payback period (PBP), etc., which will be fully described in the following section.
3.1.1. Total Life Cycle Cost (TLCC)
One of the economic methods for calculating the entire cost of a renewable energy microgrid equipped with energy storage throughout the course of a project is the net present cost (NPC) or total life cycle cost (TLCC). This economic criterion considers all the factors affecting the cost of such a project, such as maintenance costs, initial costs, installation costs and so on
[34][35]. This approach provides a complete financial picture by considering all costs and benefits over the project’s entire life cycle. Also, it can compare various combinations of measures and select the one that will maximize to save and financial return. These specifications make its application in microgrids with energy storage systems very interesting. However, predictive tools and available databases to estimate operation and maintenance costs are usually inadequate; hence, to apply to real problems, LCC is often very difficult
[36].
3.1.2. Levelized Cost of Energy (LCOE)
Energy generation’s average net present value (NPV) during its operating life is measured by the levelized cost of energy (LCOE), also known as the levelized cost of electricity. Additionally, it serves as a tool for comparing and contrasting various techniques of producing power. Lifetime cost of energy (LCOE) “represents the average income per unit of electricity generated to repay the expenses of constructing and maintaining the plant throughout its estimated financial life and duty cycle”. It is calculated as the ratio between all discounted costs over the lifetime of an electricity generating plant divided by the discounted sum of the actual energy quantities delivered. The estimator is in charge of selecting inputs for the LCOE. Capital costs, finance costs, fuel costs, decommissioning costs, operating and maintenance costs (both constant and variable), and an assumed utilization rate are all included. The LCOE is a useful method since it combines both fixed and variable costs into a single measurement to simplify analysis. However, it doesn’t consider all costs related to an actual financial decision, leading to the oversimplifying project context. It also oversimplifies interest rates and ignores project risks, leading to uncertainty in the results
[37].
3.1.3. Annualized Cost of the System (ACS)
The system’s annual cost is another economic analysis tool for clean power generation systems. This criterion consists of replacement, capital, and maintenance costs
[38]. A limitation of
ACS is that the discount rate or capital cost must be estimated for each project; it may be inaccurate. Since energy storage systems are generally introduced as a project’s capital cost, this limitation can be problematic.
3.1.4. Internal Rate-of-Return (IRR)
The amount of real profit the system generates over the course of the project is known as the internal rate of return (ROI). The time-adjusted rate of return (or return on investment) is another name for this metric. For an execution project, this discount rate equals zero’s net present value (NPV)
[39]. This method provides the exact rate of return for each project than the investment cost, thus allowing the investor to get a sneak peek into the potential returns. It is very promising in microgrid systems based on energy storage systems, which will be more profitable. However, it can only help decide whether a project is worth investing in. Moreover, IRR cannot account for the project size when comparing projects
[40].
3.1.5. Payback Period (PBP)
Payback time is the period during which an investment’s initial cash flow may be expected to be recouped using the investment’s subsequent cash inflows. Calculating the amount of time it will take to recover your initial investment using the payback period is an important part of any financial planning process. The biggest advantage of using this method is that it is easy to calculate
[41]. It may subjectively definite the target payback period; also, it does not note the time value of money
[42].
3.2. Power
In addition, a power generation system from renewable sources should be cost-effective and be investigated from a life cycle assessment (LCA)
[43][44]; it should be considered in terms of reliability. The dependability of a clean microgrid is evaluated using a variety of methodologies, including the loss of power supply probability (LPSP), the level of autonomy (LA), the expected energy not supplied (EENS), and so on.
3.2.1. Loss of Power Supply Probability (LPSP)
To assess a renewable energy system’s dependability, one method is to look at the chance of a power supply interruption. Using a percentage to indicate the performance of a power production system in load supply is a great way to gauge the system’s overall efficiency. When it comes to losing electricity, the odds are always somewhere in the range of 1 to 0. The number zero is the highest, and the number one is the smallest
[45]. In fact, LPSP is the probability that an inadequate power supply gives when the generation resources and energy system storage cannot meet the power required
[46].
3.2.2. Expected Energy Not Supplied (EENS)
Unsupplied power supply (EENS) is another measure of system reliability. This index reflects the amount of energy that is predicted to be unavailable because of the load conditions or insufficient supply of load. An example of EENS is the energy that will be unavailable when the local power surpasses the available generation, in a more precise sense
[47]. It is defined by the expected value for a local power “
L” as follows:
where
Ph is power generated by the system; EENS is calculated per kilowatt-hour
[48].
3.2.3. Level of Autonomy (LA)
This reliability index specifies the fraction of time the intended load can be met. Moreover, its value depends on two crucial factors. 1—Total number of hours of activity or work (
HTotal) and 2—Number of hours in which loss of load (
HLOL) occurs
[49]. LA can be calculated as:
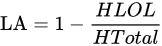
3.3. Energy Storage System Sizing Techniques
Researchers use different techniques to determine the optimal size of energy storage units for clean power plants. Each of these methods has advantages and disadvantages. The complexity of using these techniques also depends on the type and method of optimization. Green microgrid components can be reduced in size using a variety of strategies, from simple techniques to mathematical and nature-inspired approaches. Here, we’ll focus more on procedures that are commonly utilized and found in academic publications
[38][39], a variety of optimization strategies, including artificial intelligence, multi-objective design, analytical technique, probabilistic approach, Iterative approach, graphic method building method, and commercially accessible computer software.
3.3.1. Artificial Intelligence (AI)
Artificial intelligence (AI) techniques, such as artificial neural networks (ANN), genetic algorithms (GA), and division algorithms (DA), are one of the most commonly used tools for optimizing components of power generating systems from network-independent renewable energy sources, particle swarm optimization (PSO), harmony search (HS), biogeography-based optimization (BBO), ant colony optimization (ACO).
3.3.2. Multi-Objective Design
One of the optimization approaches with more than one goal set for optimization in the objective function is multi-objective optimization. This method’s general strategy is to combine all of the single goal functions into a single function or to discover the whole set of possible solutions and then assess them to obtain the best response from this set of possible answers
[50][51][52][53][54][55]. When it is applied to solve multi-objective problems, its main advantage is that it typically generates sets of solutions that allow computation of an approximation of the entire Pareto front. Since solving problems related to energy storage systems usually depends on several different objectives (e.g., cost vs. environmental burdens), this approach can be very helpful in solving them. However, it still has its weaknesses. Among the disadvantages of multi-objective optimization is that it cannot tolerate randomness inside the objective function and suffer from a slow convergence rate.
3.3.3. Iterative Approach
The performance assessment of the power generating system is accomplished in an iterative process utilizing a recursive program in this method, resulting in an optimal system. The net present value (NPV) approach, levelized cost of energy (LCOE), or energy cost model is used as an economic indicator for this method, and the LPSP is also utilized to estimate the dependability of the power production system
[56][57][58][59][60][61]. This approach allows developers to find functional/design-related flaws as early as possible to take corrective measures on a limited budget. However, it suffers from high risk and uncertainty, so it may not be appropriate for complex and object-oriented projects.
3.3.4. Analytical Method
Another optimization technique is the analytical method. Using computer models, optimal solutions are analyzed and determined in this process. This tool is used by researchers to calculate the appropriate size for various combinations of green microgrid components
[62][63]. However, it can lead to underestimating, unknown, and measurement errors.
3.3.5. Probabilistic Approach
There are several ways to assess all conceivable occurrences, their probabilities of occurrence, and related losses so that a more realistic depiction of risk may be portrayed in the future. This is a broad method. The consequences of climate change are considered when evaluating the ideal size of a microgrid independent of the green grid, and adequate and accurate models for power generation and consumption are created. This results in a risk model. This approach provides vital data for decision-making processes at the operational and planning level
[64]. While exhaustive and time-consuming are disadvantages of the probabilistic approach
[65].
3.3.6. Graphical Construction Method
The graphic construction technique is dependent on producing enough products to match the average demand. The average quantity of electricity provided by renewable sources like wind and solar will satisfy the average power needs for a clean microgrid. There are just two possible outcomes when using this tool. However, in the optimization process by this method, only two parameters can be included
[66].
3.3.7. Computer Tools and Software
For those interested in optimizing the scale of off-grid microgrids from an economic standpoint, there is a variety of computer software available. One of the most popular tools for determining the optimal size of green microgrids is HOMER. User expectations and life cycle costs can be used to model an energy-producing system. HOMER allows the user to select the most cost-effective and efficient setup from a list of options. Additionally, the power generating system size may be precisely optimized with HYBRID2. Opt Quest, General Algebraic Modeling System (GAMS), TRNSYS, LINDO, Photovoltaic Energy Simulation Systems (Sim Pho Sys), WDILOG2, RETScreen, and many more are examples of computer software for developing power production systems
[67][68][69][70][71][72][73][74][75][76][77][78][79][80][81][82][83].
It should be noted that computer tools and software can solve mono or multi-objective optimization problems; also, they are comprehensive in terms of optimization variables. However, some of them, such as HOMER cannot enable the user to select appropriate system components intuitively. Moreover, tools, e.g., HYBRIDS, only can simulate one configuration at a time
[66].