Smart city is a collective term for technologies and concepts that are directed toward making cities efficient, technologically more advanced, greener and more socially inclusive. These concepts include technical, economic and social innovations. This term has been tossed around by various actors in politics, business, administration and urban planning since the 2000s to establish tech-based changes and innovations in urban areas. The idea of the smart city is used in conjunction with the utilization of digital technologies and at the same time represents a reaction to the economic, social and political challenges that post-industrial societies are confronted with at the start of the new millennium. The key focus is on dealing with challenges faced by urban society, such as environmental pollution, demographic change, population growth, healthcare, the financial crisis or scarcity of resources. In a broader sense, the term also includes non-technical innovations that make urban life more sustainable. So far, the idea of using IoT-based sensor networks for healthcare applications is a promising one with the potential of minimizing inefficiencies in the existing infrastructure. A machine learning approach is key to successful implementation of the IoT-powered wireless sensor networks for this purpose since there is large amount of data to be handled intelligently.
1. Introduction
Habibzadeh et al.
[1] studied emerging trends in smart healthcare applications and key technological developments that have a direct impact in these transitions. The authors also reviewed different security considerations in smart healthcare systems and their consequences and counter measures. Latif et al.
[2] analyzed security and privacy issues in the IoT’s application in smart cities and identified their proposed solutions. Authors tend to use graph theory for rectification of highlighted issues since the conventional approaches do not provide optimal results for security and safety critical systems. The authors in
[3] consider smart city systems as a whole and discuss how a smart healthcare system interacts and collaborates with smart city infrastructure and how it works for the healthcare field. Several case studies are also reviewed by the authors and they suggest how a more powerful, integrated and effective system can be built out of smart healthcare systems. A comprehensive survey study was performed by Arasteh et al.
[4], describing IoT technologies for smart cities and the main components and features of a smart city. The authors also explained practical experiences and challenges faced by implementers around the world. Alsamhi et al.
[5] presented a comprehensive survey of potential techniques and applications of collaborative drones with the IoT which have been recently used to enhance the smartness of smart cities based on data collection, privacy, security, public safety, disaster management, energy consumption and quality of life in smart cities.
Li et al.
[6] presented a comprehensive review of the applications of machine learning techniques for big data analysis in smart healthcare systems. The authors also highlighted several strengths and weaknesses for existing approaches with a special focus on research challenges in this aspect. Kharel et al.
[7] proposed a model for a smart health monitoring system based on fog computing. The proposed architecture claims to acknowledge the underlying problems of a clinic-centric healthcare system and change it to a smart patient-centric healthcare system. Muhammad et al.
[8] presented a comprehensive review of the published surveys using deep learning-based methods for brain tumor classification, covering the main steps including preprocessing, features extraction, classifications, achievements and limitations. The authors also investigated state-of-the-art convolutional neural network models for BTC by performing extensive experiments using transfer learning with and without data augmentation. Soomro et al.
[9] proposed a model combining artificial intelligence with the IoT to reduce traffic congestion in a smart city environment. Allam et al.
[10] reviewed the urban potential of AI and proposed a new framework binding AI technology and cities, ensuring the integration of key dimensions of culture, metabolism and governance, often considered key factors in the successful integration of smart cities.
Majeed et al.
[11] presented a comprehensive review of the evolution and role of blockchain in the development of IoT-based smart cities. Blockchain evolution in terms of constituent technologies, consensus algorithms and blockchain platforms are presented in step 1. In the second step, several smart applications enabled by blockchain are discussed and evaluated, and finally, real world blockchain applications in smart cities are discussed as case studies. Cugurullo
[12] explored the theory and practice of artificial intelligence’s intersection with the development of smart cities in three steps. First of all, the authors present a theoretical framework for better understanding of AI in the context of urbanism. Secondly, they examined the case study of Masdar city, which is an Emirati urban experiment, and thirdly, they proposed a research agenda for the investigation of an autonomous city. Ullah et al.
[13] performed a review to evaluate the roles of artificial intelligence, machine learning and deep reinforcement learning in the evolution of smart cities. Jha et al.
[14] performed a study to develop an effective system for possible monitoring of losses, traffic management, smart city innovations, digitalized and integrated systems and software. Singh et al.
[15] introduced an ML-based, distributed big data analysis framework for next gen web for the IoT and demonstrated that the suggested distributed framework is more efficient than the conventional frameworks. Singh et al.
[16] proposed DeepBlockScheme, which is a deep learning-based blockchain scheme for secure smart cities. The authors presented a case study of car manufacturing for the proposed scheme and compared it with existing research with parameters such as security and privacy. Energy efficiency in the IoT is also one of the key areas of research. Zhang et al.
[17] systematically analyzed IoT architecture and the power distribution within. A comprehensive summary of the energy consumption model in the IoT and pros and cons of improving energy efficiency were presented. Singh et al.
[18] proposed an OTS (one-time signature) scheme-based secure architecture for an energy-efficient IoT in edge infrastructure. The authors used a blockchain-based, distributed network at the fog layer to improve the security and privacy of the architecture.
A lot of research has been carried out over the course of the past few years for the IoT, smart cities, smart healthcare, blockchain and AI in the IoT. Use of WSN in smart cities and in particular healthcare has opened up several new research avenues and challenges for smart cities which are addressed by employing AI and blockchain technologies. The following Table 1 presents a comprehensive view of the reviewed literature for this study.
Table 1. Reviewed literature.
Sr. |
Title |
Covered Areas |
Smart Cities |
Smart Healthcare |
AI/ML |
Security |
1 |
Toward uniform smart healthcare ecosystems: A survey on prospects, security, and privacy considerations. [1] |
N |
Y |
N |
Y |
2 |
A survey of security and privacy issues in IoT for smart cities [2] |
Y |
N |
N |
Y |
3 |
A Survey on the Status of Smart Healthcare from the Universal Village Perspective [3] |
Y |
Y |
N |
N |
4 |
IoT-based smart cities: A survey [4] |
Y |
N |
N |
N |
5 |
A survey on collaborative smart drones and internet of things for improving smartness of smart cities. [5] |
Y |
N |
N |
N |
6 |
A Comprehensive Survey on Machine Learning-Based Big Data Analytics for IoT-Enabled Smart Healthcare System [6] |
Y |
Y |
Y |
N |
7 |
An architecture for smart health monitoring system based on fog computing [7] |
N |
Y |
Y |
N |
8 |
Deep Learning for Multigrade Brain Tumor Classification in Smart Healthcare Systems: A Prospective Survey [8] |
N |
Y |
Y |
N |
9 |
Artificial Intelligence enabled IoT: Traffic congestion reduction in smart cities. [9] |
Y |
N |
Y |
N |
10 |
On big data, artificial intelligence and smart cities. [10] |
Y |
N |
Y |
N |
11 |
Blockchain for IoT-based smart cities: Recent advances, requirements, and future challenges. [11] |
Y |
N |
N |
Y |
12 |
Urban artificial intelligence: From automation to autonomy in the smart city. [12] |
Y |
N |
Y |
N |
13 |
Applications of artificial intelligence and machine learning in smart cities [13] |
Y |
N |
Y |
N |
14 |
Mitigating and monitoring smart city using internet of things [14] |
Y |
N |
N |
N |
15 |
Machine learning based distributed big data analysis framework for next generation web in IoT [15] |
Y |
N |
Y |
N |
16 |
DeepBlockScheme: A Deep Learning-Based Blockchain Driven Scheme for Secure Smart City. [16] |
Y |
N |
Y |
Y |
17 |
Energy efficiency in internet of things: An overview [17] |
N |
N |
N |
Y |
18 |
OTS Scheme Based Secure Architecture for Energy-Efficient IoT in Edge Infrastructure [18] |
Y |
Y |
N |
Y |
2. IOT and Healthcare
In conjunction with WSNs the IoT brings an unprecedented amount of data that the network infrastructure needs to handle. The solution for these problems is to customize the traditional network designs to the latest standards of network intelligence, which ensures optimal security [19]. Hospitals, clinics and care facilities need one cost-effective network infrastructure, the security of which complies with data protection regulations but is also easy to use and to operate. Figure 1 shows the leading disease types considered in the artificial intelligence literature.
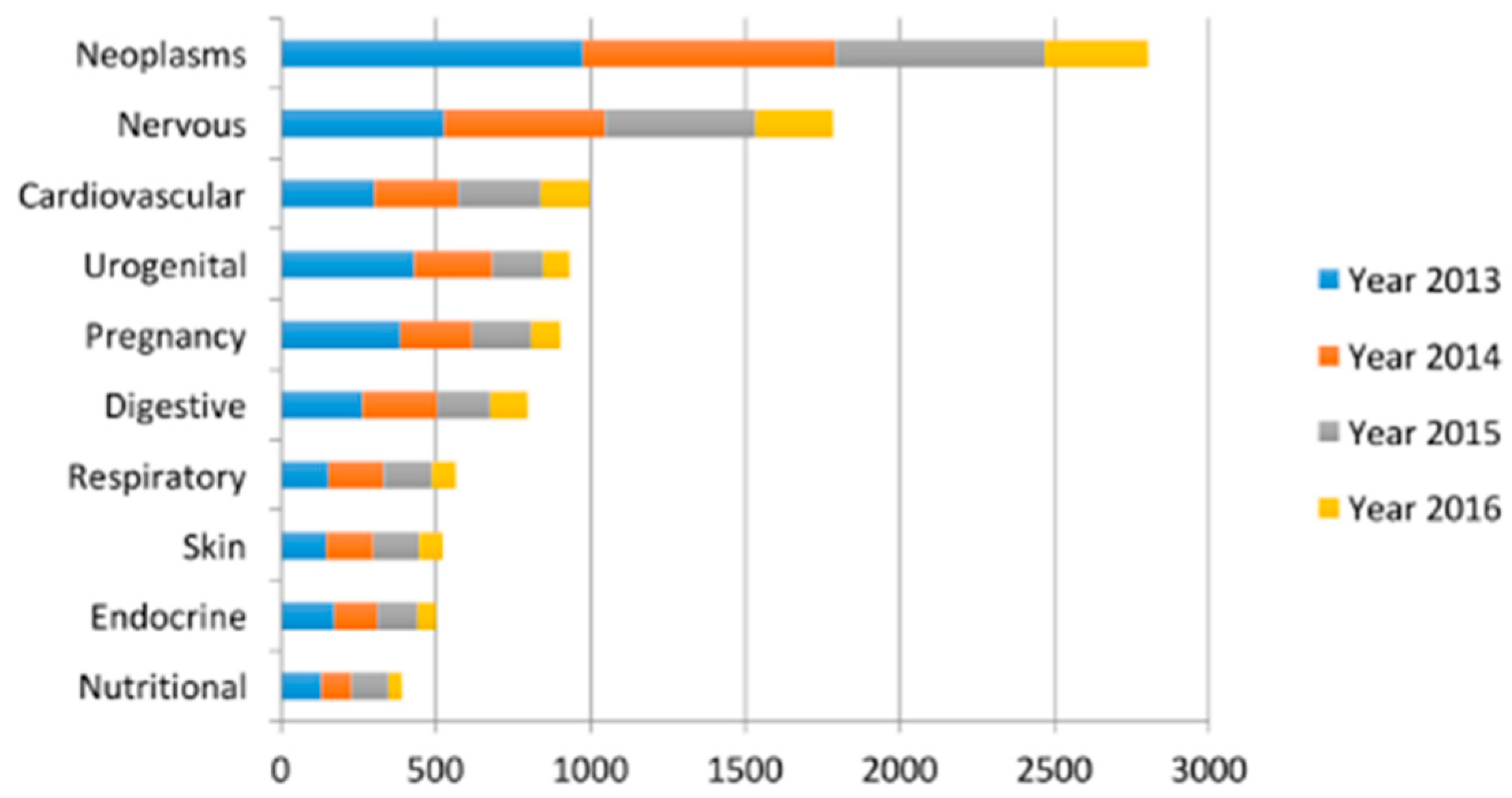
Figure 1. The leading disease types considered in the artificial intelligence literature (PubMed) [20].
2.1. Patient Monitoring
Advanced technologies in health care allow both inpatient and outpatient care to be monitored more closely. Remote patient monitoring (RPM) enables healthcare professionals to monitor vital signs and assess physical reactions to previous treatments without having to be in the same place as the patient. The device used depends on the health of the respective patient. For example, it could be an implanted cardiac device, an airflow monitor, or a networked blood glucose meter. The device in question collects the desired data. If the values are not as they should be, the data are simultaneously forwarded to a database for recording and to the treating doctor. The doctor can analyze the information in real time and react accordingly. Such devices are often used immediately after an operation. They help reduce the number of hospitals stays and avoid re-admissions because problems are identified more quickly. This allows doctors to react earlier and avoid potential complications. With the help of the data collected in real time, it is also possible to adjust and adapt treatment options more quickly, depending on the patient’s physical reaction and condition. This allows doctors to react earlier and avoid potential complications
[21].
2.2. Digital Drugs
One of the newer innovations in the healthcare industry is what is known as “smart pills”. Smart pills are taken like normal medication but are equipped with some kind of monitoring technology in addition to the actual medication. They use it to forward information to a sensor worn on the body. These sensors monitor drug levels in the body based on a patient’s perceived or diagnosed condition. The data from the portable sensors are then transmitted to a mobile phone app, which means that patients can access data on their vital functions themselves. Doctors can do this if the patient agrees. This is how the treating physicians determine whether a drug is working as intended or possibly causing side effects
[21].
In November 2017, Abilify MyCite launched the first FDA-cleared smart pill that timestamped only when the drug was actually taken. As soon as the pill comes into contact with the patient’s gastric acid, it triggers a sensor that marks the time of contact and first forwards this information to the wearable sensor and finally to the mobile phone app. The correct dosage and the prescribed intake are important prerequisites for a successful treatment. Such information is very valuable to medical professionals, and they no longer have to rely on the patient’s word alone when treatment plans must be strictly adhered to. If patients fail to do this, the doctor can seek a discussion and clarify the cause directly
[22].
One of the areas that causes a little more discomfort are so-called “robotic” pills. Once ingested, they are able to perform certain functions directly in the patient’s body. Companies like Rani Therapeutics are developing pills that have the ability to pass through the body to navigate and perform functions that, for some reason, cannot be performed non-invasively. For example, Rani invented a pill that navigates through the stomach into the small intestine, where it injects without the exposure of the active compound to digestive enzymes. When the drug is delivered, the residue dissolves and is digested, an option that is ideal for large, long, chained drug molecules such as proteins, peptides and antibodies.
2.3. Medical Equipment
Wearable medical devices are the most attractive option today for consumers of all ages for tracking their own vital signs in real time. In addition to Fitbit, Apple Watch and Co., other wearable technologies have now been developed. Not only do they record data, but they also perform certain functions based on commands or recognized situations. One example is “intelligent associations”. They are equipped with sensors that assess the size of the underlying wound to determine whether it is healing or not, or if there is an infection, and whether a topical solution may need to be administered
[22].
“Networked contact lenses” are also a form of wearables in health technology. In 2014, Google and Novartis began developing a connected contact lens that could monitor blood sugar levels by analyzing the patient’s tear fluid. The data collected via the contact lenses is then sent to an insulin pump and the patient is informed whether their blood sugar level has reached a dangerous level and needs to be adjusted. This advance in non-invasive monitoring of diabetic patients could be life-changing for many. Because many patients suffer from having to prick themselves several times a day in order to measure their blood sugar level, such innovations give diabetes patients hope that non-invasive techniques are actively being sought and that they are on the verge of becoming a reality
[23].
2.4. Medical Institutions
Many of the benefits of the IoT for the healthcare industry lie in improving the quality of care for patients. However, thanks to the Internet of Things, medical facilities have also improved, for example through more efficient processes and by conserving valuable resources. Intelligent technology in hospitals and care facilities ensures, for example, that doctors can better monitor expensive devices such as MRIs, CT and PT scanners and X-ray machines in terms of effectiveness and service life. In this way, malfunctions or incorrect operation can be avoided. Remote sensors minimize the number of manual tests or may even eliminate the need for them. That frees up time for more urgent tasks. A common problem in medical facilities is the relocation of equipment or systems that are used very often. This becomes a problem if a device cannot be located in an emergency. The use of Bluetooth low-energy location technology enables devices to be located in real time. This avoids anger and stress if you cannot find a device in an emergency situation. A small innovation helps to save countless lives. In contrast, the costs are negligible. Healthcare is one of the industries where the IoT has already made its mark
[24]. A 2017 study by Aruba Networks found that 60 percent of healthcare organizations around the world have already implemented IoT devices in their facilities, and that number will grow dramatically in the years to come. It is obvious that IoT solutions have found their way into the healthcare industry and have become indispensable there. From better patient monitoring to intelligent pills to low energy location solutions, the IoT makes life easier for medical professionals and improves the treatment and care of patients, ultimately the goal of the IoT—to improve the quality of life for as many people as possible.
3. Conclusions
The use of AI, blockchain, machine learning and IoT technologies for the development of smart healthcare systems and broadly for the development of smart cities can truly enhance and optimize the accuracy of expected results. Sensor networks, IoT and machine learning therefore offer untapped potential to relieve doctors and better identify diseases. It is precisely because the health systems are facing major challenges due to an aging society, financial bottlenecks and growing mountains of (analog and digital) data, the use of modern technologies is becoming increasingly inevitable. This will possibly lead to the increased use of robots in everyday medical practice. In the near future, even items of personal health use such as toothbrushes can be equipped with IoT-enabled sensors that will eventually lead to an improved quality of life in general and even help save countless lives.
The future of healthcare is very promising, considering the rapid development in sensor technology, AI and machine learning. For patients, hospitals and doctors as well as for medical device manufacturers, there are not only new opportunities but even the obligation to make use of the Internet of Things. It is obvious that challenges and substantial risks have to be mastered. Throughout the reviewed literature there is a consistency for use of smart technologies in smart cities and in particular healthcare, and AI and blockchain technologies are key driving factors for enhancement and improvement of overall user experience of smart cities. Although there are potential downsides of artificial intelligence and machine learning technologies in the context of smart cities, they still also have a potential to change the way we know smart healthcare and smart cities as of now.