Forensic facial comparison is a human observer-based technique employed in forensic facial identification. Facial identification falls under the broader discipline of facial imaging, and involves the use of visual facial information to assist in person identification. Through the analysis of photographic or video evidence (e.g., CCTV), forensic facial identification is routinely utilized to associate persons of interest to criminal activity in a judicial context. The recommended approach to forensic facial comparison is facial examination by morphological analysis, whereby a facial feature list is used to analyze, compare, and evaluate visible facial features between a target image and a potential matching image. This process is then validated by a second analyst. Forensic facial comparison, and its broader discipline of facial identification, should not be confused with automated facial recognition technology or the innate psychological process of facial recognition.
1. Introduction
Depicting faces
[1], facial anthropometry
[2], and facilitating crime scene investigations
[3][4] have relied on the use of photography in a forensic context almost since its development
[1]. Probably the most recognized use of photography in a forensic setting, and its derivative in the form of video recording, is surveillance. Closed-circuit television (CCTV) was the natural progression of improved use of video technology that allowed for consistent monitoring and review of potential criminal activities
[5]. CCTV surveillance systems have since the 1990s become increasingly more common and relied upon throughout the world
[6][7][8][9] and are in fact considered by many communities the norm in public areas
[10][11].
Other than general surveillance and criminal activity monitoring, facial examination is often of interest for the data extracted from many CCTV surveillance systems. This has become more evident as the deployment of CCTV systems and increases in crime have led to an increase in demand for facial identification
[12][13][14]. This rise in demand is a direct outcome of the increased availability of image data, from both CCTV data
[6][12] and photographic and video evidence from other sources, such as mobile phones
[15]. F
orensic facial comparison (FFC) for identification has remained largely untested, despite this increasing demand [16][17].
2. Terminology
Colloquial confusion in terminology exists between facial identification and recognition. It should be clarified that facial identification is reliant on perfect agreement, which is different from facial recognition
[18]. Facial recognition is defined as the innate psychological process humans employ at a glance to recognize a face, usually based on familiarity
[18].
Recognition is employed generally as part of the investigative process of facial comparison and is holistic, rapid, and methodologically inconsistent with a high predisposition to error [19][20]. Attempting facial identification from a forensic anthropological perspective requires the application of a methodical human-based examination of facial images for identity confirmation [21][22][23].
Another prominent misconception in facial identification (ID) involves the misuse of the term “facial recognition” to specifically refer to automated or semi-automated facial recognition systems, with this being fully adopted by many in the field of automated facial recognition (e.g.,
[24][25]). To avoid this miscommunication, certain studies refer to automated facial recognition as facial recognition technology (FRT) or systems
[26]; however, this practice is not universally applied. A primary reason to distinguish FRT and facial comparison in practice is the association of FRT with
high false positive rates [27][28], strong racial biases [29], and other ethical concerns around privacy and consent that need to be resolved prior to its the employment in a legal context. Currently, FRTs are still reliant on human-based validation in their operating loops
[30].
Human observer-based facial image comparison is considered the preferred approach to facial ID [21][31][32][33] and will likely persist as the validation method of choice despite the improvement and widespread deployment of FRT systems.
3. Forensic Facial Comparison
Facial examination, also referred to as forensic facial comparison (FFC), must be applied using the Analysis, Comparison, Evaluation, and Verification (ACE-V) approach
[23], commonly used in other forensic practices, such as fingerprint identification
[34]. The ACE-V methodological approach is intended to integrate principles of the scientific method in forensic comparisons in order to enhance their implementation and reliability
[34].
In the past, approaches to FFC included photo-anthropometry, facial superimposition, and morphological analysis (MA)
[23][35], with morphological analysis being the currently accepted method as advised by both the Facial Identification Scientific Working Group (FISWG) (
https://fiswg.org/index.htm) and the European Network of Forensic Science Institutes (ENFSI) (
https://enfsi.eu/)
[23][36]. Application of MA relies on the detailed examination of specific facial features to reach a conclusion with regard to the similarity or dissimilarity of two or more faces
[23]. The facial features are assessed subjectively, evaluated, and compared between the faces
[23]. The selection of individual facial features often depends on the feature list utilized. Feature lists generally include both overall face composition and structure, individual anatomical feature components (e.g., hairline shape, ear helix morphology, nasal alae protrusion, etc.), and distinguishing characteristics such as scars, blemishes, piercings, and tattoos (e.g.,
[37]). The current standard feature list used for facial comparison relies on criteria developed by the FISWG
[37]. An example of how this analysis is conducted is shown in
Figure 1, using sample facial images from the Wits Face Database
[38].
Figure 1. Example of a forensic facial comparison analysis process between a wildtype (WT) photograph and a standardized (ST) photograph from the Wits Face Database [38] sample images in the South African Police Services court chart format [39]. The individual facial features are numbered, analyzed, compared, and evaluated between the two images using the FISWG feature list [37]. Features marked in blue indicate morphological similarity between the two images, while features marked in red indicate morphological dissimilarity. In the example provided, skin color appears different due to lighting discrepancies in the two images (red 1); however, skin texture appears similar (blue 1). The facial images can be found in the Wits Face Database data note, including the supplementary material for the Wits Face Database [38].
4. Validation of Forensic Facial Comparison and Recommended Practice
Recent work testing the validity of MA-based FFC across various settings found the method to be overall accurate and reliable under optimal conditions. Optimal conditions include high-resolution photographs
[17] and high-resolution Internet Protocol (IP) CCTV recordings captured
at both ceiling height and eye-level [40]. While disguises, such as brimmed caps can lower performance of MA [41], sunglasses did not decrease the overall accuracy [41]. Images captured from low-resolution analogue CCTV at ceiling height were, however, found to greatly hinder analysis [40]. A detailed overview of these validation studies is shown in Figure 2.
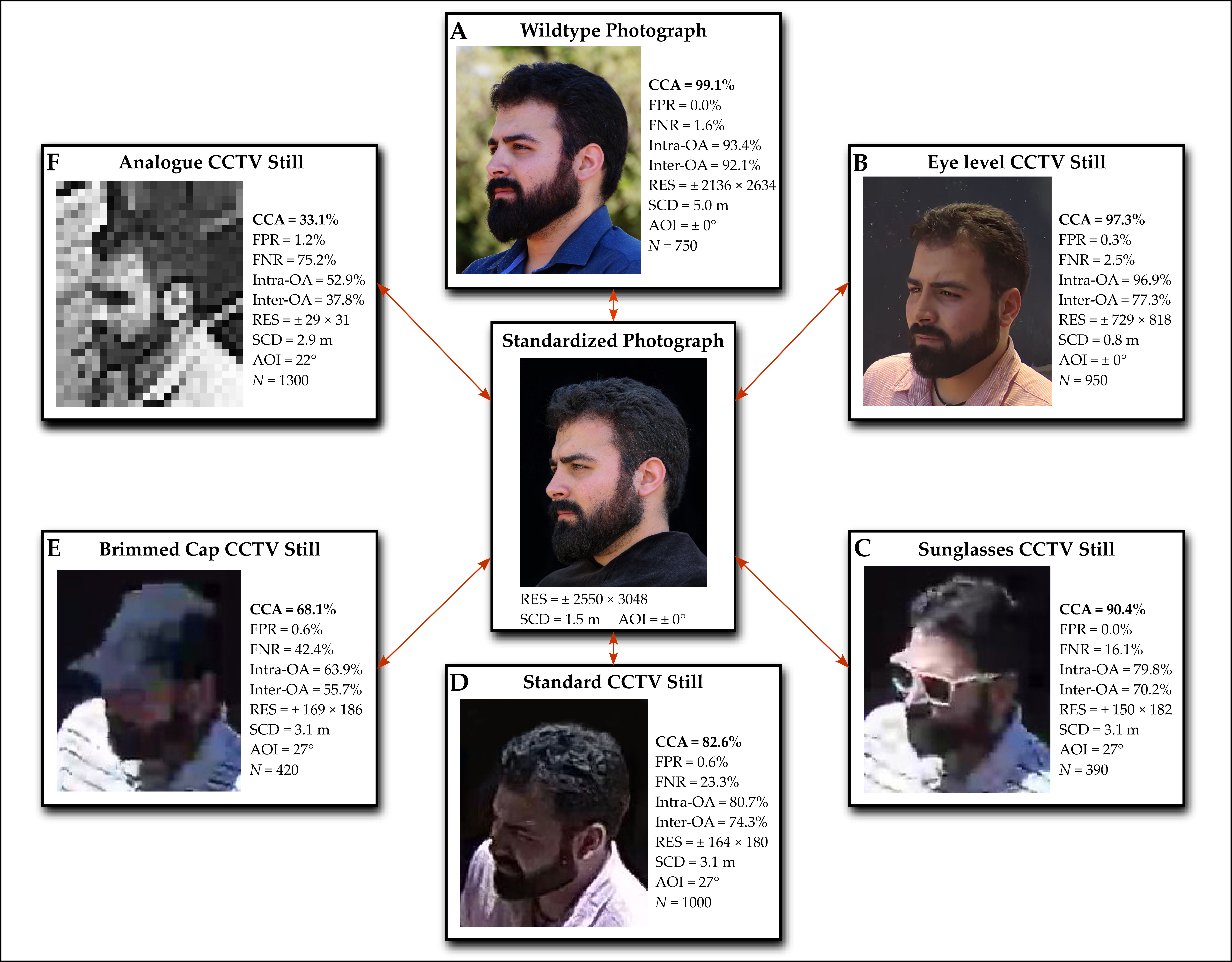
Figure 2. Visual summary of the validation studies testing morphological analysis across realistic photographic and CCTV conditions [17][40][41] using sample photographs and CCTV stills from the Wits Face Database [38]. Images (A) to (F) are samples of the target images from each set of conditions analyzed that were compared to the central image arising from the standardized photographs captured for each participant. All major statistical results and the details of the conditions of each comparison cohort are presented. Representative images of each condition are arranged from A to F in a clockwise order according to descending chance-corrected accuracy. The conditions of analysis were as follows: wildtype informal photographs (A) of similar quality to the standardized photographs; eye level digital CCTV still images (B); standard digital CCTV still images (D) with sunglasses (C) and with brimmed caps (E); and monochrome analogue CCTV still images (F). Key: CCA = chance corrected accuracy; FPR = false positive rate; FNR = false negative rate; OA = observer agreement; RES = resolution; SCD = subject-to-camera distance; AOI = angle of incidence; N = number of comparisons. The facial images can be found in the Wits Face Database data note, including the supplementary material for the Wits Face Database [38]. This figure is replicated from https:// doi.org/10.3390/biology10121269 [39].
Based on these recent studies
[17][40][41] as well as the recommendations from the FISWG and ENFSI
[23][36], the step-wise approach to conducting FFC by MA is presented in the flow diagram below (
Figure 3).
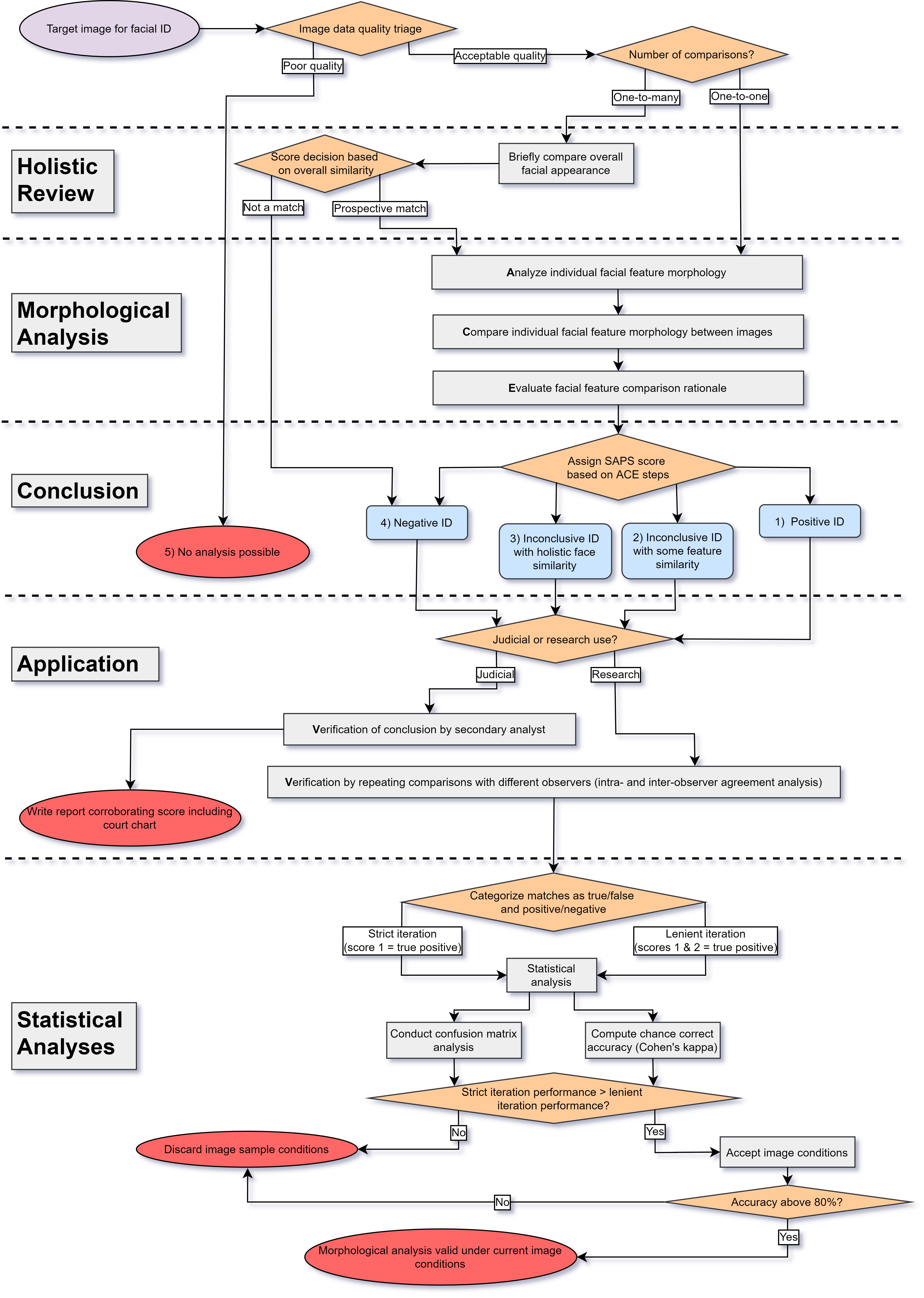
Figure 3. Flow diagram of the recommended morphological analysis process [39]. This approach to morphological analysis uses an ACE-V method in conjunction with the FISWG feature list [37], with the inclusion of the ENFSI’s image quality triaging [36] and the use of the South African Police Services (SAPS) scoring criteria [13] as adapted for research application [17]. Statistical analyses for research use are also recommended based on recent work [40] to allow for more detailed result interpretation and comparison among future studies.
5. Forensic Facial Comparison Limitations
The minimum criteria for facial examination across various CCTV installations, are not clearly highlighted, and a more thorough understanding of the limitations imposed on footage by specific installations is needed. While the global increase in CCTV data is beneficial to criminal investigation and facial comparison, there is a concerning lack of standardization of required installation, recording conditions, and image quality [35][42][43][44][45][46]. As a result, the usefulness of CCTV-derived facial images is difficult to assess and makes facial comparison challenging in contrast to controlled photographs and mugshots.
These limitations along the CCTV imaging chain are often acknowledged; however, few studies have assessed their implication in facial comparison accuracies [17][40][42][46][47][48]. Successful facial identification assessment is hindered by inconsistent recording conditions and poor image quality. Facial comparison accuracy and data quality are, thus, directly correlated [49][50], especially in terms of individual accuracy variation across multiple analysts [51] and individual analyst ability overestimation [52].
An overview of the general and more specific limiting factors of CCTV data in the application of MA and their specific effects in the process of facial comparison is presented in Table 1.
Table 1. Summary of CCTV systems’ technical limitations in the application of morphological analysis.
General Limitations
|
Specific Limitations
|
Effects
|
Camera placement
|
Camera height above ground [17][40][41][53][54]
Angle of incidence [17][40][41][54]
Subject-to-camera distance [55][56][57]
|
Image composition affected—target size and screen/picture height [17][40][41][53]
Reduction of observable facial features [17][40][41][54]
Perspective distortion [55][56][57]
|
Camera specifications
|
Analogue or digital [42]
Sensor size [53]
Pixel count [40][53]
Lens focal length [53]
|
Reduced image quality [17][40][53]
Image distortion and artefacts [53]
|
Lighting conditions
|
Ambient lighting [53][58][59]
Infrared vision [47][60][61]
|
Loss of facial detail [40][41]
Shadows and overexposure form artificial boundaries and altered facial appearance [41][62]
Optical distortions [53]
|
Image quality
|
Resolution [17][40]
Pixelation [40][46]
Noise/grain [53]
Video compression [44]
Color [40]
|
Low clarity [53][55]
Reduced useable detail [17][40][42][44][46]
Face matching ability reduced [17][40][44][63][64]
|
Data loss and corruption
|
Network infrastructure [38]
Software [53]
Hardware [38][53]
Imminent weather [38]
Power outages [38]
Compression rate [44]
Anti-forensic techniques [65][66][67][68]
|
Inconsistent network connection and coverage—transfer corruption [38]
Partial or complete data loss [38]
Data tampering and removal [65][66][67][68]
|
This entry is adapted from the peer-reviewed paper 10.3390/biology10121269