Grain quality involves the appearance, nutritional, and safety attributes of grains. With the improvement of people’s living standards, problems pertaining to the quality of grains have received greater attention. Modern quality detection techniques feature unique advantages including rapidness, non-destructiveness, accuracy, and efficiency in detecting grain quality.
1. Introduction
Grains, containing nutritional ingredients including carbohydrates, proteins, fats, vitamins, and minerals, are daily necessities of human life. However, impurities, unsound kernels, fungal toxins, pesticides, and heavy metal residues pose a risk to human health. With regular economic growth and social progress, consumers have begun to pay more attention to the quality and safety of grains and have increasingly larger demands for high-quality and highly safe grains. The quality attributes of grains acceptable to consumers mainly include appearance attributes (size, shape, and color), nutritional attributes (protein, starch, fat, and vitamin), and safety attributes (contaminants such as mildew, pesticide residues, and heavy metal residues). Detailed and specific requirements for quality indices of grains have been set in Chinese National Standards. For appearance attributes, appearance indices including the color and shape, impurity, and unsound kernels of wheat, maize, paddy, and soybean have been elucidated in detail in the Assistant Atlas of Grain Sensory Inspection
[1][2][3][4]. As to nutritional attributes, the determination methods for nutrients including proteins
[5], starches
[6], fats
[7], ashes
[8], amino acids
[9], dietary fibers
[10], and trace elements
[11] have been specified in the Chinese National Standards for Food Safety. In terms of safety attributes, the maximum residue limits for pesticides
[12], maximum residue limits for fungal toxins
[13], and maximum levels of contaminants
[14] in grains have been listed in the Chinese National Standards for Food Safety. There are also detailed requirements pertaining to the appearance
[15][16][17][18], nutritional attributes
[19][20][21][22][23][24][25], and safety attributes
[26][27][28][29] of grains in international standards.
Grain quality is an important index in the grain circulation process involving the production, storage, trading, and processing. Grain quality detection has always been one of the greatest challenges pertaining to the treatment, processing, classification, and safety guarantees needed in the food industry. Traditionally, grain quality detection is realized through sensory and chemical analyses. However, sensory analysis is time-consuming, inefficient, highly subjective, and susceptible to external interference (influences of physical conditions such as fatigue); chemical analysis is expensive, time-consuming, laborious, and destructive, and requires a laboratory. In recent years, to meet the requirements of modern quality inspection, detection techniques based on physical properties such as acoustic, optical, thermal, electrical, and mechanical properties and sensory features including visual, gustatory, and olfactory features have been developed apace. The references of the state of the art were obtained within the last five years in the core collection database of the Web of Science search engine. The researchers searched the references by combining the keywords cereal, grain, and quality with the technical words of physical properties such as near-infrared spectroscopy (NIRS), hyperspectral imaging (HSI), Raman spectroscopy (RS), optical, dielectric, nuclear magnetic resonance (NMR), X-ray, electromagnetic, acoustic, thermal, and mechanical, and sensory features such as electronic eye (E-eye), computer vision, electronic nose (E-nose), electronic tongue (E-tongue), and sensory, respectively. The researchers classified all the references according to the quality as the first condition and the techniques as the second condition, and summarized and analyzed the research purposes, research contents, research methods and research results of each reference. The previous references have focused on the applications of a particular detection method to different objects, or some detection methods for selected objects. Herein, the researchers analyzed and summarized the advantages and disadvantages of the methods of physical properties and sensory features in terms of appearance, nutritional and safety attributes of cereal grains, and explored the techniques that can detect various quality indicators in different application scenarios. Based on the latest technical references the researchers have collected, the researchers have mapped the latest research progress of research institutes [30][31][32][33][34][35][36][37][38][39][40][41][42][43][44][45][46][47][48][49][50][51][52] (Figure 1) and commercial instruments [53][54][55][56][57][58][59][60][61][62][63][64][65][66][67][68][69][70][71][72][73][74][75][76][77][78][79][80][81][82][83][84][85][86] (Figure 2).
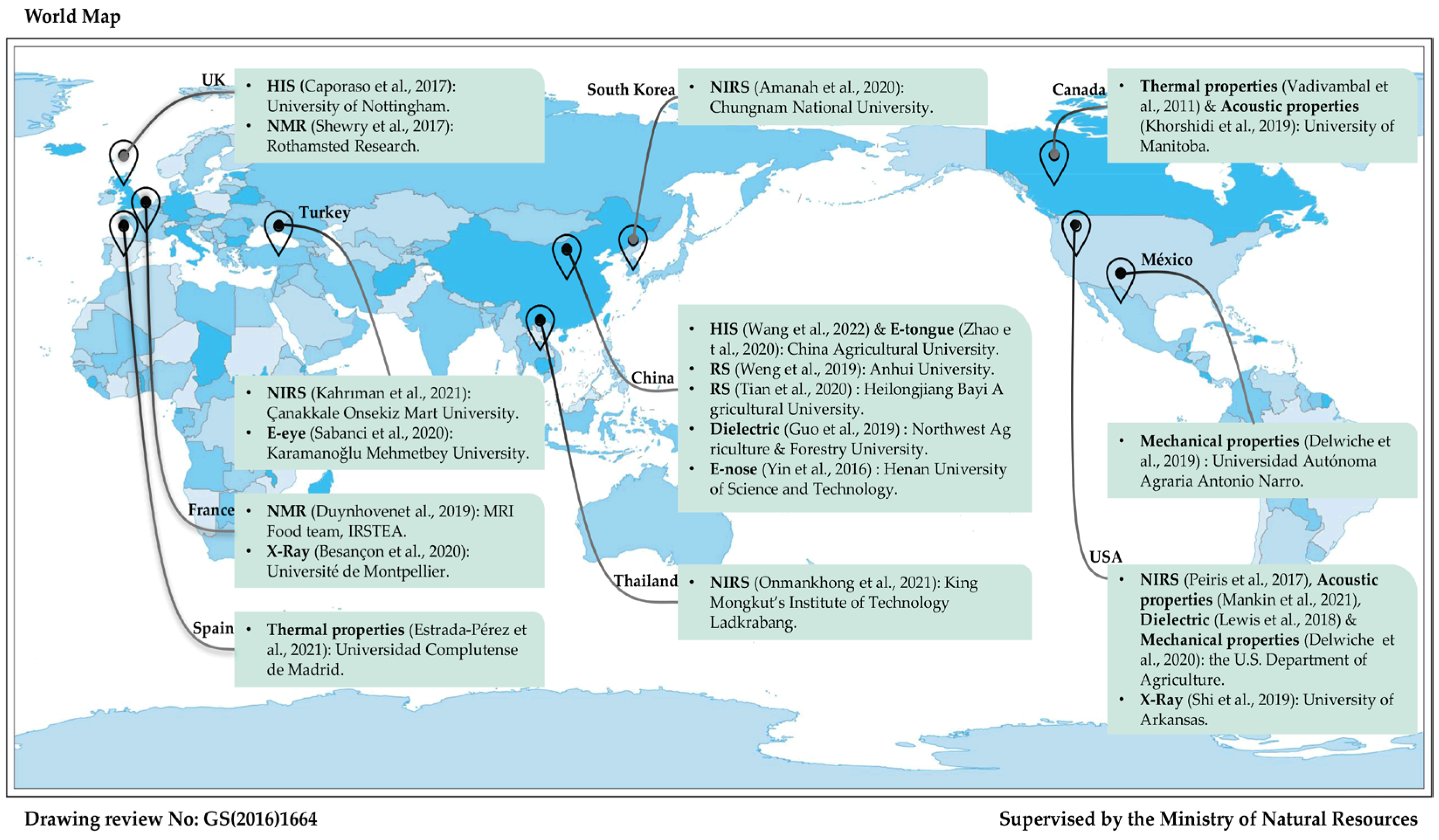
Figure 1. The research progress of research institutions on grain quality detection techniques [30][31][32][33][34][35][36][37][38][39][40][41][42][43][44][45][46][47][48][49][50][51][52].
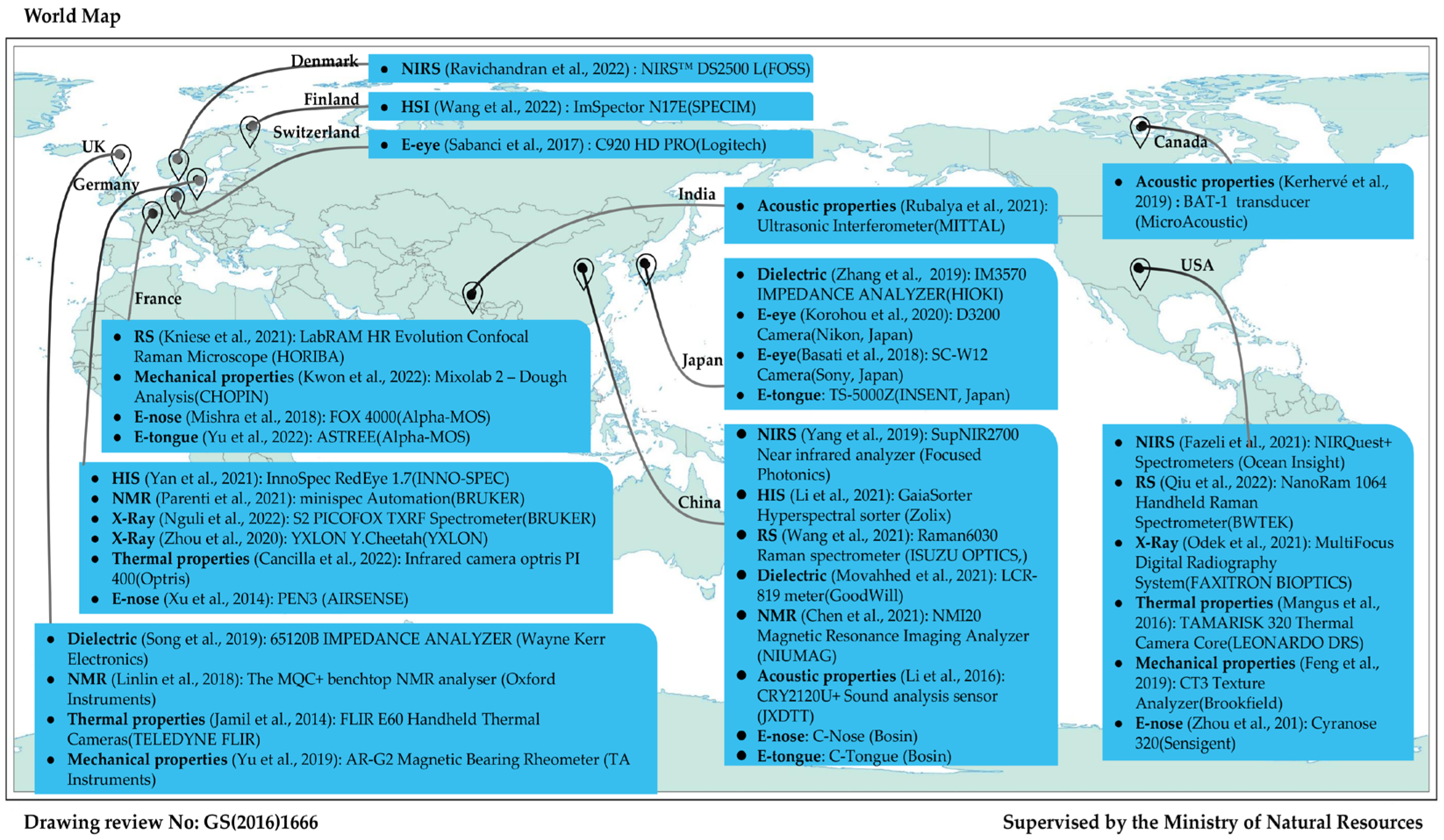
Figure 2. Commercial instruments for grain quality detection [53][54][55][56][57][58][59][60][61][62][63][64][65][66][67][68][69][70][71][72][73][74][75][76][77][78][79][80][81][82][83][84][85][86].
Among detection techniques for grain quality based on physical properties, those based on optical features such as NIRS, HSI, and RS detect nutritional attributes (proteins, starches, and fats) and safety attributes (pesticide residues and fungal toxins) of grains
[87]. The detection is based on the response to light in grains, including its absorption, reflection, transmission, and scattering. In addition, HSI can also be used to determine color and shape
[88]; RS also can be adopted to assay heavy metal residues
[89]. Detection techniques based on electromagnetic properties such as those based on dielectric properties, NMR, and X-ray realize detection according to response signals of grains in the electrical or magnetic fields. They can be utilized to determine the moisture content
[90] and nutritional attributes such as starches and fats
[91]. X-rays also can be used to determine trace elements and heavy metals in grains
[92]. Detection techniques based on acoustic features were adopted to study nutrients including proteins and appearance attributes such as unsound kernels according to responses of grains to acoustic signals (reflection and transmission)
[93]. Detection techniques based on thermal features were used to assess safety attributes involving fungi and appearance attributes including unsound kernels based on differences in thermal radiation of each part of grains
[94]. Those based on mechanical properties were employed to study nutritional attributes including proteins and starches according to mechanical features of grains under all kinds of applied load
[95]. Among detection techniques for grain quality based on sensory features, E-eyes were used to identify appearance attributes of grains including the color and shape, impurities, and unsound kernels according to features such as color and shape in images
[96]. E-noses were employed to evaluate safety attributes involving pesticide residues and fungal toxins and appearance attributes including unsound kernels in accordance with gaseous response signals of volatile organic compounds in grains
[97]. E-tongues are often used to detect nutritional attributes such as proteins and starches and safety attributes about heavy metals based on taste response signals in grain leachates
[98]. The principles and objects of detection techniques for grain quality based on physical properties and sensory features are listed in
Table 1.
Table 1. Modern inspection techniques for grain quality.
| Detection methods |
|
| Principles |
|
| Objects |
|
| Limitation |
|
| Physical |
| properties |
|
| Optical |
| properties |
2.1. Non-Destructive Quality Detection Methods for Appearance Attributes of Cereal Grains
According to descriptions in the Assistant Atlas of Grain Sensory Inspection, the appearance attributes of grains mainly include quality indices such as color and shape, impurities (screen underflows, inorganic impurities, and organic impurities), and unsound kernels (immature, injured, specked, broken, germinated, and moldy grains). Traditionally, appearance attributes are mainly inspected through an artificial sensory analysis, which is both time- and labor-consuming and greatly affected by subjective factors. Among modern detection methods of appearance attributes, the HSI, NMR, X-ray, those based on acoustic and thermal features, and E-eye all can detect indices of appearance attributes (Table 2).
Table 2. Non-destructive quality detection methods for appearance attributes of cereal grains.
| Detection Methods |
|
| Objects |
|
| Devices |
|
| References |
|
|
| NIRS |
|
| Realizing quantitative quality detection and qualitative analysis according to differences in the absorption band and intensity of hydric groups in organic components of grains in the near-infrared region |
|
| HSI |
|
| Color and shape, unsound kernels, and impurities |
|
| Zolix “GaiaSorter” hyperspectral imaging system |
|
| [99][100][101] |
| Nutritional attributes including proteins, starches, and fats, and safety attributes pertaining to pesticide residues and fungal toxins |
|
| High precision instruments are expensive and the NIR spectra of different components overlap. |
|
|
| HSI |
|
|
|
| NMR |
| Realizing accurate detection of grain quality based on hyperspectral and image data |
|
106 | ] |
|
2.2. Non-Destructive Quality Detection Methods for Nutritional Attributes of Cereal Grains
The main nutritional indices of grains include proteins, starches, fats, ashes, amino acids, dietary fibers, and trace elements. Traditionally, nutritional attributes are determined using physical and chemical experimental analysis. The Kjeldahl method for nitrogen determination, hydrolysis, Randall extraction, incineration, amino-acid analyzers, enzymatic gravimetric method, and plasmas are generally adopted to determine protein
[5], starch
[6], fat
[7], ash
[8], amino acid
[9], dietary fiber
[10], and trace element
[11] contents in grains. Traditional detection methods generally call for destructive sample preparation and are time-consuming and inefficient. In modern inspection methods for nutritional attributes, methods including NIRS, HSI, RS, NMR, X-ray, and those based on acoustic properties, mechanical properties, and E-tongues are mainly used (
Table 3).
Table 3. Non-destructive detection methods for nutritional attributes of cereal grains.
| Detection Methods |
|
| Objects |
|
| Devices |
|
| References |
|
| NIRS |
|
| Proteins, starches, and amino acids |
|
| Unity SpectraStar 2500XL-spectrometer |
|
| [107][108] |
|
| Nutritional attributes including proteins, safety attributes pertaining to pesticide residues and fungal toxins, and appearance attributes including color and shape |
|
| HSI is costly, the amount of hyperspectral data is extremely large, and it is difficult to store and analyze. |
|
| HSI |
| Unsound kernels |
|
| NMI20 bench top pulsed NMR analyzer |
|
|
| Proteins, oleic acids, and starches |
| [102] |
|
| RS |
|
| OCI-UAV-1000 hyper-spectrometer |
|
| [31][109][110] |
|
|
| X-ray |
| Based on scattering spectra of different components in grains at different light frequencies; achieving quality detection by analyzing molecular vibration and rotation of these components in grains |
|
| Unsound kernels |
| Nutritional attributes including proteins, and safety attributes pertaining to fungal toxins, pesticide residues, and heavy metals |
|
| Skyscan 1272 X-ray micro-CT scanner |
|
| Fluorescence phenomena on Fourier variation Raman spectral interference, optical systems affecting different vibrational peak overlaps, and Raman scattering intensity. |
|
| RS |
|
| Proteins, starches, amino acids, and oils |
|
| Renishaw Raman spectrometer |
| [48] |
|
| [ | 111 | ][112][113][114][115] |
|
| Electromagnetic properties |
|
| Dielectric |
|
| Acoustic properties |
| According to the response characteristics of grains in the applied electric field |
|
|
| Unsound kernels |
|
| Self-made impulse signal acquisition device |
| Moisture content |
|
| [103] |
|
| NMR |
|
| Oils |
|
| Minispec mq20 NMR spectrometer |
|
| [116] |
|
| High correlation mainly with moisture. |
|
| NMR |
| Thermal properties |
| Atomic nuclei with fixed magnetic moments in grains produce a string of response signals with attenuated intensity in the specific impulse trains. |
|
| Moisture content and nutritional attributes including starches and fats |
|
| Mainly used for moisture state, migration process analysis, high price, complex signal analysis, and imperfect NMR spectrum database. |
|
| Unsound kernels |
|
| MLG-II temperature sensor |
|
| [104] |
|
| X-ray |
|
| Trace elements |
|
| Hard X-ray microprobe |
|
| [71][117 |
| X-ray |
|
| Elements in grains release X-ray fluorescence of specific energy under X-ray irradiation |
|
| Nutritional attributes including trace elements, and safety attributes pertaining to heavy metals |
|
| X-ray control is complicated and dangerous. |
|
] | [ | 118 | ] |
|
| E-eye |
|
| Color and shape, unsound kernels, and impurities |
|
| CCD camera or smartphone |
|
|
| Acoustic properties |
|
| Proteins and ashes |
| [ |
| Physical property analyzer |
| 81] |
| [36][105][ |
|
| Acoustic properties |
|
| According to the reflection, scattering, projection, and absorption characteristics of acoustic waves in grains |
|
| Nutritional attributes including proteins, and appearance attributes such as unsound kernels |
|
| Mechanical properties |
|
| Proteins and starches |
|
| High environmental noise interference. |
|
| CT3 physical property analyzer |
|
| [50][63] |
|
| Thermal properties |
|
| According to differences in thermal radiation of various parts of grains |
| E-tongues |
|
| Starches and proteins |
| Appearance attributes such as fungal infection and unsound kernels |
|
| Self-made three-electrode E-tongue |
|
| High ambient temperature disturbance. |
|
| [ |
| Mechanical properties |
|
| According to the mechanical features of grains under all types of mechanical load |
|
| Nutritional attributes including proteins and starches |
|
| The association between mechanical properties and quality is unclear. |
|
119 | ] |
|
| Sensory |
| features |
|
| E-eye |
|
| According to features including color and shape in images |
|
| Multiple appearance attributes |
|
| High requirements for clarity of acquired images, difficulty to identify early mold and pest images, and difficulty to segment multi-seed images. |
|
| E-nose |
|
| According to gaseous response signals of volatile organic compounds in grains |
|
| Safety attributes pertaining to fungal toxins and pesticide residues |
|
| Early mold or mild pesticide residues produce low gas concentrations that are difficult to detect and environmental gas interference. |
|
| E-tongue |
|
| According to taste response signals of grain leachates |
|
| Nutritional attributes including proteins and starches, and safety attributes pertaining to heavy metals |
|
| The detection object is the leachate of seed samples |
|
The organizational structure of this research is shown in Figure 3.
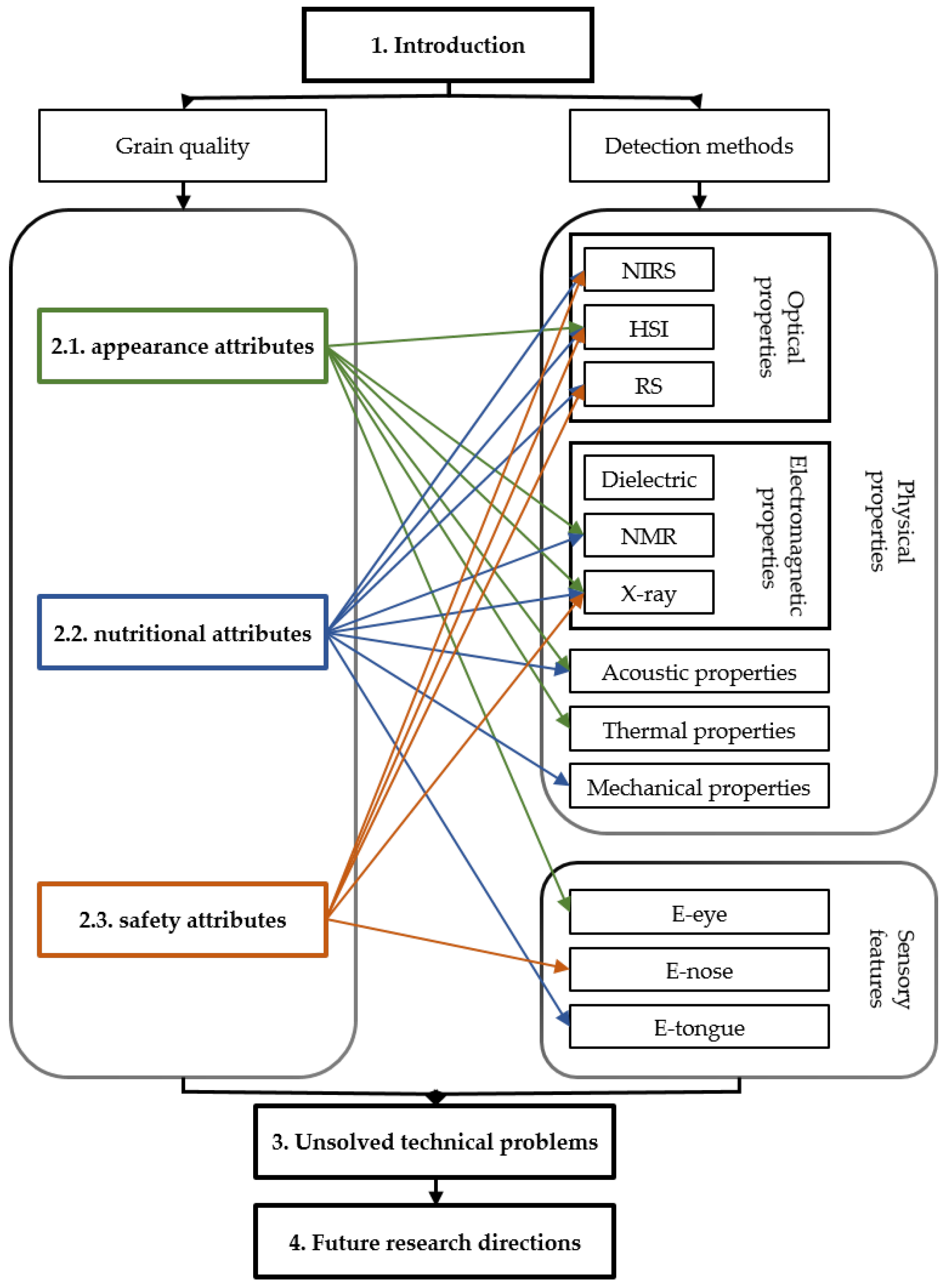
Figure 3. Block diagram of the entry organization.
2. Overseas and Domestic Research Status
2.3. Non-Destructive Inspection Methods for Safety Attributes of Cereal Grains
Regarding safety attributes, the maximum limits for pesticide residues, fungal toxins, and contaminants in grains have been listed in the Chinese National Standards for Food Safety. Traditionally, gas chromatography and liquid chromatography are commonly used for determining the maximum limits of pesticide residues and fungal toxins, and atomic absorption spectrometry (ABS) is utilized to measure heavy metal residues. Modern methods for detecting safety attributes mainly include NIRS, HSI, RS, X-ray, and E-noses (Table 4).
Table 4. Non-destructive inspection methods for safety attributes of cereal grains.
| Detection Methods |
|
| Objects |
|
| Devices |
|
| References |
|
| NIRS |
|
| Fungal toxins |
|
| Zeiss fiber optical spectrometer |
|
| [120][121] |
|
| HSI |
|
| Fungal toxins |
|
| ANDOR EMCCD camera + Xenics LWNIR camera |
|
| [122] |
|
| RS |
|
| Pesticide residues and fungal toxins |
|
| 1064-nm NanoRam Raman spectrometer |
|
| [32][60][123] |
|
| X-ray |
|
| Heavy metals and fungal toxins |
|
| Y. CHEETAN micron-resolution X-ray CT scanner |
|
| [124][125] |
|
| E-noses |
|
| Pesticide residues and fungal toxins |
|
| Fox3000 E-nose |
|
| [126][127] |
|
3. Unsolved Technical Problems
For different quality indices of grains, different types of detection methods can be used to realize high-accuracy detection. However, these methods still have room to improve in terms of grain-quality detection.
- High ① Optical detection methods including NIRS, HSI, and RS have developed to relative maturity, while the detection cost of full-spectrum devices is high. ② NMR instruments also face the problem of high cost.
- Environmental interference. Grain quality detection based on acoustic and thermal features is greatly influenced by the environment (ambient noise and temperature).
- Detection principle. ① The relationship between the mechanical features and quality indices of grains remains unclear. ② Water is an important factor that affects the dielectric property of grains, while the relationship between quality indices and dielectric properties of grains is also poorly understood. ③ Detection objects of E-tongues must be grain leachates, which limits the application thereof to the quality detection. ④ X-rays may contaminate grains.
- E-nose detection is limited by the LOD of gas sensors and the method fails to identify problems including early mildew in grains.
- Moisture detection. Water is an important factor that influences grain quality and must be detected in all stages including the harvest, storage, trading, transportation, and processing of grains.
- Grading and classification of grains. Grains can be graded and classified according to differences in multiple quality indices of grains in accordance with the national or international standards. However, research on grain quality using a single detection technique can only determine one or several indices, which fails to meet the grading and classification demands imposed in practice.
- Practical application issues. In different applications, grain quality inspection equipment faces different challenges; for example, the aerodynamic characteristics of the grain seeds during sowing, and the vibration of the machinery during harvesting can affect the quality inspection results.
4. Future Research Directions
-
① Considering that screening of characteristic wavelengths of different quality indices of grains is still an important part in existing quality detection research, device development based on characteristic wavelengths can substantially reduce the cost of analysis. This is conducive to the popularization and application of optical detection devices. In recent years, NIRS spectrometers
[128][129] have also been upgraded with the development of NIRS analysis and chemometrics methods
[130][131][132][133]. Liu et al.
[134] selected four characteristic wavelengths to develop the portable near-infrared quality detector, which can realize the real-time determination of proteins and moisture in wheat kernels. The development of multispectral imaging
[135] based on characteristic wavelengths can overcome this problem. Sendin et al.
[136] discriminated between high-quality and poor-quality maize using 19 characteristic wavelengths, with the classification accuracy in the range of 83% to 100%. ② NMR can be divided into high-field and low-field ones
[137]. A high-field NMR spectrometer contains expensive superconducting magnets, so it has complex structures and its signals are difficult to process. A low-field NMR spectrometer uses low-cost permanent magnets, so it is preferred in grain-quality detection.
-
Acoustic features include audible sound and ultrasonic waves. Detection based on audible acoustic features is susceptible to the ambient noise, while acoustic detection based on ultrasonic waves can avoid environmental interference, and is an important method of applying acoustic methods to grain-quality detection
[36]. Eliminating interference (including the effect of changes in ambient temperature) with detection based on thermal features is the top priority for improving the accuracy of detection of grain quality. Mangus et al.
[62] performed environmental calibration using a temperature reference plate, which compensates for environmental influences including air temperature, relative humidity, solar radiation, and camera temperature, thus maintaining the measurement temperature.
-
① The difference in mechanical properties of cereal grains is determined by the tightness of bonding of main components including starches and proteins therein. By using an electronic universal testing machine, Cheng et al.
[138] measured the shear resistance of wheat. In this way, they obtained that the shear resistance is significantly positively correlated with the protein content, positively correlated with wet and dry gluten, negatively (albeit insignificantly) correlated with the starch content, and positively (albeit insignificantly) correlated with the bulk weight and thousand-kernel weight. Existing research into the mechanical properties of cereal grains mainly focuses on grain quality evaluation
[139], while the correlation of these properties with quality indices remains to be further studied. ② Moisture detection of grains based on dielectric properties has reached an extremely high accuracy, so developing detection devices applicable to different application scenarios is a potential direction for future development
[140]. ③ Preparation of grain leachates is laborious and the detection electrodes of E-tongues need to be cleaned and polished in a complex process before the next detection
[141]. Therefore, the development of preparation techniques of detection samples for E-tongues and the upgrading of detection electrode materials are the only way to realizing real-time efficient detection of quality indices of grains. ④ X-rays include hard and soft variants
[142]; hard X-rays may damage grains, while soft ones have low penetrability and therefore, they are applicable to detection of quality indices of grains.
-
The multi-scale, systematic organization for imitating biological noses through bionics design is one of the methods to improve the LOD of E-noses. In addition, development of high-LOD gas-sensing materials is also an important approach to improving the performance of E-noses
[143].
-
Research into moisture detection in grains has developed to relative maturity, and high-accuracy moisture detection can be realized based on dielectric properties and NMR
[56][75][144][145][146][147]. Robust progress has been made in moisture detection devices based on dielectric properties
[79][140][148], while those based on NMR have not developed to any substantial extent. Moisture detection devices for grains in different application scenarios should be a focus of future research.
-
The combination of multiple quality detection techniques of grains can detect multiple quality indices in the grading and classing requirements, thus realizing grain grading and classification. At present, some studies have combined multiple detection techniques to improve the detection accuracy
[149][150][151][152][153]. Realizing the grading and classification of cereal grains by combining multiple quality detection techniques remains the focus of future research.
-
To guarantee the normal operation of grain quality detection equipment, the interference factors affecting grain quality detection equipment are studied for specific application scenarios. Gierz et al.
[154] collected seed image data at the air velocity (15, 20, 25 m/s) of a pneumatic seeder pipeline conveying seeds based on the aerodynamic characteristics of grain seeds
[155][156], and constructed a classification model based on multilayer-based perceptron network, which has a correct classification coefficient of 0.99 for contaminants in seeds at a sowing speed of 15 m/s. Studying the influencing factors in different application scenarios is necessary to promote the application of grain quality detection instruments.