1. Introduction
Autonomous systems can play a vital role in assisting humans in a variety of problem areas. This could potentially be in a wide range of applications like driver-less cars, humanoid robots, assistive systems, domestic systems, military systems, and manipulator systems, to name a few. Presently, the world is at a bleeding edge of technologies that can enable this even in our daily lives. Assistive robotics is a crucial area of autonomous systems that helps persons who require medical, mobility, domestic, physical, and mental assistance. This research area is gaining popularity in applications like autonomous wheelchair systems [
,
], autonomous walkers [
], lawn movers [
,
], vacuum cleaners [
], intelligent canes [
], and surveillance systems in places like assisted living [
,
,
,
]. Data are one of the most important components to optimally start, continue, or complete any task. Often, these data are obtained from the environment that the autonomous system functions in; examples of such data could be the system’s position and location coordinates in the environment, the static objects, speed/velocity/acceleration of the system or its peers or any moving object in its vicinity, vehicle heading, air pressure, and so on. Since this is obtained directly from the operational environment, the information is up-to-date and can be accessed through either built-in or connected sensing equipment/devices. This survey is focused on the vehicle navigation of an autonomous vehicle. We review the past and present research using Light Imaging Detection and Ranging (LiDAR) and Imaging systems like a camera, which are laser and vision-based sensors, respectively. The autonomous systems use sensor data for tasks like object detection, obstacle avoidance, mapping, localization, etc. As we will see in the upcoming sections, these two sensors can complement each other and hence are being used extensively for detection in autonomous systems. The LiDAR market alone is expected to reach $52.5 Billion by the year 2032, as given in a recent survey by the Yole group, documented by the “First Sensors” group [
].
In a typical autonomous system, a perception module inputs the optimal information into the control module. Refer to
. Crowley et al. [
] define perception as "
The process of maintaining an internal description of the external environment.
"
Figure 1.
High-level Perception Framework.
2. Data fusion
Data fusion entails combining information to accomplish something. This ’something’ is usually to sense the state of some aspect of the universe
Data fusion entails combining information to accomplish something. This ’something’ is usually to sense the state of some aspect of the universe
. The applications of this ’state sensing’ are versatile, to say the least. Some high level areas are: neurology, biology, sociology, engineering, physics,and so on[15,16,17,18,19,20,21]. Due to the very versatile nature of the application of data fusion, throughout this manuscript, we will limit our review to the usage of data fusion using LiDAR data and camera data for autonomous navigation. Kolar et. al.,
[118] performed an exhaustive data fusion survey and docmented several details about data fusion using the above two sensors.
performed an exhaustive data fusion survey and docmented several details about data fusion using the above two sensors.
Sensors and their Input to Perception
3. Sensors and their Input to Perception
A sensor is an electronic device that measures physical aspects of an environment and outputs machine(a digital computer) readable data. They provide a direct perception of the environment they are implemented in. Typically a suite of sensors is used since it is the inherent property of an individual sensor; to provide a single aspect of an environment. This not only enables the completeness of the data but also improves the accuracy of measuring the environment.
The Merriam-Webster dictionary defines a sensor[22] as
A device that responds to a physical stimulus (such as heat, light, sound, pressure, magnetism, or a particular motion) and transmits a resulting impulse (as for measurement or operating a control)
The initial step is raw data capture using the sensors. The data is then filtered and an appropriate fusion technology implemented this is fed into localization and mapping techniques like SLAM; The same data can be used to identify static or moving objects in the environment and this data can be used to classify the objects, wherein classification information is used to finalize information in creating a model of the environment which in turn can be feed into the control algorithm[
A sensor is an electronic device that measures physical aspects of an environment and outputs machine(a digital computer) readable data. They provide a direct perception of the environment they are implemented in. Typically a suite of sensors is used since it is the inherent property of an individual sensor; to provide a single aspect of an environment. This not only enables the completeness of the data but also improves the accuracy of measuring the environment.
The Merriam-Webster dictionary defines a sensor[22] as a device that responds to a physical stimulus (such as heat, light, sound, pressure, magnetism, or a particular motion) and transmits a resulting impulse (as for measurement or operating a control).
The initial step is raw data capture using the sensors. The data is then filtered and an appropriate fusion technology implemented this is fed into localization and mapping techniques like SLAM; The same data can be used to identify static or moving objects in the environment and this data can be used to classify the objects, wherein classification information is used to finalize information in creating a model of the environment which in turn can be feed into the control algorithm[
27]. The classification information could potentially give details of pedestrians, furniture, vehicles, buildings, etc. Such a classification is useful in both pre-mapped ie., known environments and unknown environments since it increases the potential of the system to explore its environment and navigate. ]. The classification information could potentially give details of pedestrians, furniture, vehicles, buildings, etc. Such a classification is useful in both pre-mapped ie., known environments and unknown environments since it increases the potential of the system to explore its environment and navigate.
Multiple Sensors vs. Single Sensor
4. Multiple Sensors vs. Single Sensor
It is a known fact that most of the autonomous systems require multiple sensors to function optimally. However, why should we use multiple sensors? The individual usage of any sensor could impact the system where they are used, due to the limitations in each of those sensors. Hence, to get acceptable results, one may utilize a suite of different sensors and utilize the benefits of each of them. The diversity offered by the suite of sensors contributes positively to the sensed data perception[38,39]. Another reason could be the system failure risk due to the failure of that single sensor[21,27,40] and hence one should introduce a level of redundancy. For instance, while executing the obstacle avoidance module, if the camera is the only installed sensor and it fails, it could be catastrophic. However, if it has an additional camera or LiDAR, it can navigate itself to a safe place after successfully avoiding the obstacle, if such logic is built-in for that failure. Many researchers performed a study on high-level decision data fusion and concluded that using multiple sensors with data fusion is better than individual sensors without data fusion. The research community discovered that every sensor used provides a different type, sometimes unique type of information in the selected environment, which includes the tracked object, avoided object, the autonomous vehicle itself, the world it is being used, and so on, and the information is provided with differing accuracy and differing details[27,39,44,45,46].
It is a known fact that most of the autonomous systems require multiple sensors to function optimally. However, why should we use multiple sensors? The individual usage of any sensor could impact the system where they are used, due to the limitations in each of those sensors. Hence, to get acceptable results, one may utilize a suite of different sensors and utilize the benefits of each of them. The diversity offered by the suite of sensors contributes positively to the sensed data perception[38,39]. Another reason could be the system failure risk due to the failure of that single sensor[21,27,40] and hence one should introduce a level of redundancy. For instance, while executing the obstacle avoidance module, if the camera is the only installed sensor and it fails, it could be catastrophic. However, if it has an additional camera or LiDAR, it can navigate itself to a safe place after successfully avoiding the obstacle, if such logic is built-in for that failure. Many researchers performed a study on high-level decision data fusion and concluded that using multiple sensors with data fusion is better than individual sensors without data fusion. The research community discovered that every sensor used provides a different type, sometimes unique type of information in the selected environment, which includes the tracked object, avoided object, the autonomous vehicle itself, the world it is being used, and so on, and the information is provided with differing accuracy and differing details[27,39,44,45,46].
There are some disadvantages while using multiple sensors and one of them is that they have additional levels of complexity; however, using an optimal technique for fusing the data can mitigate this challenge efficiently. There may be the presence of a level of uncertainty in the functioning, accuracy, and appropriateness of the sensed raw data[47]. Due to these challenges, the system must be able to diagnose accurately when a failure occurs and ensure that the failed component(s) are identified for apt mitigation.
There are some disadvantages while using multiple sensors and one of them is that they have additional levels of complexity; however, using an optimal technique for fusing the data can mitigate this challenge efficiently. There may be the presence of a level of uncertainty in the functioning, accuracy, and appropriateness of the sensed raw data[47]. Due to these challenges, the system must be able to diagnose accurately when a failure occurs and ensure that the failed component(s) are identified for apt mitigation.
Need for Sensor Data Fusion
5. Need for Sensor Data Fusion
Some of the limitations of single sensor unit systems are as follows:
Deprivation
: If a sensor stops functioning, the system where it was incorporated in will have a loss of perception.
Uncertainty
: Inaccuracies arise when features are missing, due to ambiguities or when all required aspects cannot be measured
Imprecision
: The sensor measurements will not be precise and will not be accurate.
Limited temporal coverage
: There is initialization/setup time to reach a sensor’s maximum performance and transmit a measurement, hence limiting the frequency of the maximum measurements.
Limited spatial coverage
: Normally, an individual sensor will cover only a limited region of the entire environment—for example, a reading from an ambient thermometer on a drone provides an estimation of the temperature near the thermometer and may fail to correctly render the average temperature in the entire environment.
Some of the advantages of using multiple sensors or a sensor suite [
38,
44,
46,
50,
51] are as follows:
Extended Spatial Coverage: Multiple sensors can measure across a wider range of space and sense where a single sensor cannot'
Extended Temporal Coverage: Time-based coverage increases while using multiple sensors
Improved resolution: A union of multiple independent measurements of the same property, the resolution is better, i.e., more than that of single sensor measurement.
Reduced Uncertainty: As a whole, when we consider the entire sensor suite, the uncertainty decreases, since the combined information reduces the set of unambiguous interpretations of the sensed value.
Increased robustness against interference: An increase in the dimensionality of the sensor space (measuring using a LiDAR and stereo vision cameras), the system becomes less vulnerable against interference.
Increased robustness: The redundancy that is provided due to the multiple sensors provides more robustness, even when there is a partial failure due to one of the sensors being down.
Increased reliability: Due to the increased robustness, the system becomes more reliable.
Increased confidence: When the same domain or property is measured by multiple sensors, one sensor can confirm the accuracy of other sensors; this can be attributed to re-verification and hence the confidence is better.
Reduced complexity: The output of multiple sensor fusion is better; it has lesser uncertainty, is less noisy, and complete.
Data Fusion Techniques
6. Data Fusion Techniques
Over the years, scientists and engineers have applied concepts of sensing that occur in nature and implement them into their research areas and have developed new disciplines and technologies that span over several fields. In the early 1980s, researchers used aerial sensor data to obtain passive sensor fusion of stereo vision imagery. Crowley et al. performed fundamental research in the area of data fusion, perception, and world model development that is vital for robot navigation [
,
,
]. They have developed systems with multiple sensors and devised mechanisms and techniques to augment the data from all the sensors and get the 'best' data as output from this set of sensors, also known as a 'suite of sensors'. In short, this augmentation or integration of data from multiple sensors can simply be termed as multi-sensor data fusion. In the survey paper, we discuss the following integration techniques.
K-Means
6.1. K-Means
K-means is a popular algorithm that has been widely employed; it provides a good generalization of the data clustering and it guarantees the convergence.
Probabilistic Data Association (PDA)
PDA was proposed by Bar-Shalom and Tse, and it is also known by the "modified filter of all neighbors" [86]
K-means is a popular algorithm that has been widely employed; it provides a good generalization of the data clustering and it guarantees the convergence.
6. The functionality is to assign an association probability to each hypothesis from the correct measurement of a destination/target and then process it2. PDA is mainly good for tracking targets that do not make abrupt changes in their movement pattern
Distributed Multiple Hypothesis Test
A very useful technique that can Probab
e used i
n distributed and decentralized systems[90]. Thlisti
s is an extension of the multiple hypothesis tests. It is effic
ient at tracking multiple targets in cluttered environments [91]. This can be used as an estimation and tracking technique [90]. The main disadvantage is the high computation cost, which is in the exponential order.
State Estimation
Data A
ls
o known as tracking techniques, they assist with calculating the moving target's state, when measurements are given. These measurements are obtained using the sensors [87]. This is a fairly soc
ommon techni
que in data fusion mainly for two reasons: (1) measurements are usually obtained from multiple sensors; and there could be noise in the measurements. Some examples are Kalman Filters, Extended Kalman Filters, Particle Filters, etc
Covariance Consistency Methods
These methods were proposed initially by Uhlmann et al[84,87]. This is a distributed technique that maintains covariance estimations and means in a distributed system. They comprise of estimation-fusion techniques.
Distributed Data Fusion
ation (PDA
s the name suggests, this is a distributed fusion system and is often used in multi-agent systems, multisensor systems, and multimodal systems[84,94,95]. Efficient when distributed and decentralized systems are present. An optimum fusion can be achieved by adjusting the decision rules. However, there are difficulties in finalizing decision uncertainties.
)
Decision Fusion Techniques
PDA was proposed by Bar-Shalom and Tse, and it is also known by the "modified filter of all neighbors" [86]. The functionality is to assign an association probability to each hypothesis from the correct measurement of a destination/target and then process it. PDA is mainly good for tracking targets that do not make abrupt changes in their movement pattern.
These techniques can be used when successful target detection occurs [87,92,93]. They enable high-level inference for such events. When a user has a situation where multiple classifiers are present, this technique can be used. A single decision can be arrived at using the multiple classifiers. For the enablement of multiple classifiers to be achieved, apriori probabilities need to be present and this is difficult.
Hardware
Li6.3. DAR
Light Detection and Ranging (LiDAR) is a technology that is used in several autonomous tasks and functions as follows: an area is illuminated by a light source. The light is scattered by the objects in that scene and is detected by a photo-detector. The LiDAR can provide the distance to the object by measuring the time it takes for the light to travel to the object and back [104, 105, 106, 107, 108, 109 ].
Camera
The types of cameras are Conventional color cameras like USB/web camera; RGB [115], RGB-mono, and RGB cameras with depth information; RGB-Depth (RGB-D), 360 degree camera [28,116,117,118], and Time-of-Flight (TOF)camera[119,120,121].
Implementation of Dasta Fusion using a LiDAR and camera
We review an input-output type of fusion as described by Dasarathy et al. They propose a classification strategy based on input-output of entities like data, architecture, features, and decisions. The fusion of raw data in the first layer, a fusion of features in the second, and finally the decision layer fusion. In the case of the LiDAR and camera data fusion, two distinct steps effectively integrate/fuse the data.
- Geometric Alignment of the Sensor Data
- Resolution Match between the Sensor Data
Geometric Alignment of the Sensor Data
The first and foremost step in the data fusion methodology is the alignment of the sensor data. In this step, the logic finds LiDAR data points for each of the pixel data points from the optical image. This ensures the geometric alignment of the two sensors [28].
Resolution Match between the Sensor Data
Once the data is geometrically aligned, there must be a match in the resolution between the sensor data of the two sensors. The optical camera has the highest resolution of 1920 × 1080 at 30 fps, followed by the depth camera output that has a resolution of 1280 × 720 pixels at 90 fps, and finally, the LiDAR data have the lowest resolution. This step performs an extrinsic calibration of the data. Madden et al. performed a sensor alignment [126] of a LiDAR and 3D depth camera using a probabilistic approach. De Silva et al. [28] performed a resolution match by finding a distance value for the image pixels for which there is no distance value. They solve this as a missing value prediction problem, which is based on regression. They formulate the missing data values using the relationship between the measured data point values by using a multi-modal technique called Gaussian Process Regression (GPR), developed by Lahat et al. [39]. The resolution matching of two different sensors can be performed through extrinsic sensor calibration. Considering the depth information of a liDAR and the stereo vision camera, 3D depth boards can be developed out of simple 2D images
Chaributed Multiplenges with Sensor Da Hypothesis Testa Fusion
Several challenges have been observed while implementing multisensor data fusion. Some of them could be data related to like: complexity in data, conflicting and/or contradicting data, or they can be technical such as resolution differences between the sensors, the difference in alignment between the sensors, etc. We review two of the fundamental challenges surrounding sensor data fusion, which are the resolution differences in the heterogeneous sensors and understanding and utilizing the heterogeneous sensor data streams while accounting for many uncertainties in the sensor data sources [28, 39]. We focus on reviewing the utilization of the fused information in the autonomous navigation, which is challenging since many autonomous systems work in complex environments, be it at home or work, which is to assist persons with severe motor disabilities to handle their navigational requirements and hence pose significant challenges for decision-making due to the safety, efficiency, and accuracy requirements. For reliable operation, decisions on the system need to be made by considering the entire set of multi-modal sensor data they acquire, keeping in mind a complete solution. In addition to this, the decisions need to be made considering the uncertainties associated with both the data acquisition methods and the implemented pre-processing algorithms. Our focus in this review is to survey the data fusion techniques that consider the uncertainty in the fusion algorithm.
Some researchers used mathematical and/or statistical techniques for data fusion. Others used techniques comprised of reinforcement learning in implementing multisensor data fusion [70], where they encountered conflicting data. In this study, they fitted smart mobile systems with sensors that enabled the systems to be sensitive to the environment(s) they were active in. The challenge they try to solve is mapping the multiple streams of raw sensory data Smart agents to their tasks. In~their environment, the tasks were different and conflicting, which complicated the problem. This resulted in their system learning to translate the multiple inputs to the appropriate tasks or sequence of system actions. Crowel et al. developed mathematical tools to counter uncertainties with fusion and perception [133]. Other implementations include adaptive learning techniques [134], wherein the authors use D-CNN techniques in a multisensor environment for fault diagnostics in planetary gearboxes.
A very useful technique that can be used in distributed and decentralized systems[90]. This is an extension of the multiple hypothesis tests. It is efficient at tracking multiple targets in cluttered environments [91]. This can be used as an estimation and tracking technique [90]. The main disadvantage is the high computation cost, which is in the exponential order.
6.4. Sensor data noise and rectifictate Estimation
Noise filtering techniques include a suite of Kalman filters and their variations. We discuss the following:
Kalman Filter, Extended Kalman Filter, Unscented Kalman Filter, Distributed Kalman Filter, Particle Filter
Also known as tracking techniques, they assist with calculating the moving target's state, when measurements are given. These measurements are obtained using the sensors [87]. This is a fairly common technique in data fusion mainly for two reasons: (1) measurements are usually obtained from multiple sensors; and there could be noise in the measurements. Some examples are Kalman Filters, Extended Kalman Filters, Particle Filters, etc
Autonomous Navigation
Robot navigation has been extensively studied in the community for several decades [161,162,163,164,165,166,167]. It can be termed as the safe mobility of the robot from a source location to a target location, without hurting people or properties in its environment, and without damaging itself, and these tasks are performed with no or limited need for a human operator. This means that the navigation system is also responsible for decision-making capability when the system faces situations (critical or otherwise) that demand negotiation with humans and/or other robots. Autonomous navigation is a task that takes in the output from a sensor data fusion module. Autonomous navigation means that a vehicle can plan its path and execute its plan without human intervention. An autonomous robot is one that not only can maintain its stability as it moves, but also can plan its movements. They use navigation aids when possible, but can also rely on visual, auditory, and olfactory cues. Decision-making relies on data fusion which comprises combining inputs from various sources to get a more accurate combined sensor data as output [35,38,44,51]. Figure 2 gives a simple sensor data fusion and its implementation in an autonomous dynamic model genrator. Sub-systems mapping, localization, path planning, and obstacle avoidance is detailed below.
Figure 2. Tasks that accept integrated sensor information .
Mapp
6.5. Covariance Consistency Methods
These methods were proposed initially by Uhlmann et al[84,87]. This is a distributed technique that maintains covariance estimations and means in a distributed system. They comprise of estimation-fusion techniques.
6.6. Ding
When a system maps an environment, the mapping module senses the environment that the robot operates in and provides data to analyze it for optimal functioning. It is also a process of establishing a spatial relationship among stationary objects in an environment. Efficient mapping is a crucial process that gives rise to accurate localization and driving decision making. Usage of LiDARs for mapping is beneficial as they are well known for their high-speed and long-range sensing and hence long-range mapping, while cameras RGB, and RGB-Depth are used for short-range mapping and also used to efficiently detect obstacles [170,171,172].
Localizastrion
Localization is one of the most fundamental competencies required by an autonomous system, as~the knowledge of the vehicle's location is an essential precursor to take any decisions about future actions, whether planned or unplanned. In a typical localization situation, a map of the environment or world is available and the robot is equipped with sensors that sense and observe the environment as well as monitor the robot's motion [188,198,199,200]. Hence, localization is that branch in autonomous system navigation, which deals with the study and application of the ability of a robot to localize itself in a map or plan. The localization module informs the robot of its current position at any given time. A process of establishing the spatial relationship between the intelligent system and the stationary objects Localization is achieved using devices like Global Positioning Systems(GPS), odometric sensors, Inertial Measurement Units (IMU), etc. These sensors give the position information of the autonomous system, which can be used by the system to see where it is in the environment or the robot world [198,201,202]. Some of the localization techniques are dead reckoning [205,206], GPS [212,213],signal-based localization[207],vision-based localization[217,218], indoor-VR localization [219], networked sensor-based localization [214].
Pabuthed planning
Path Planning is an important subtask of autonomous navigation and is generally termed as a problem of searching for a path which an autonomous system has to follow in a described environment and requires the vehicle to go in the direction closest to the goal, and, generally, the map of the area is already known[220,221,222,223]. Path planning when used in conjunction with techniques of obstacle avoidance gives a more robust deployment of the path planner module by enabling the system to avoid hazardous collision objects, no-go zones, and negative objects like potholes and similar objects. Some types of path planners are global, local, heuristic, static, dynamic path planners.
ObsDatacle avoidaFusionce
For successful navigation of an autonomous system, avoiding obstacles while in motion is an absolute requirement. The vehicles must be able to navigate in their environment safely [30,32,33,35,170]. Obstacle avoidance involves choosing the best direction among multiple non-obstructed directions, in real-time, hence obstacle avoidance can be considered to be more challenging than path~planning[223]. Obstacles can be of two types (i) Immobile Obstacles (ii) Mobile Obstacles. Static object detection deals with localizing objects that are immobile in an environment, for example, indoor static obstacles, can be a table, sofa, bed, planter, TV stand, walls, etc. Outdoor static obstacles can be buildings, trees, parked vehicles, poles (light, communication), (standing or sitting) persons, animals lying down, etc. Moving object detection deals with localizing the dynamic objects through different data frames obtained by the sensors to estimate their future state example of indoor moving objects can be walking or running pets at home, moving persons, operating vacuum robots, crawling baby, people moving in wheelchairs, etc. Outdoor moving obstacles can, for instance, by moving vehicles, pedestrians walking on the pathway, moving ball thrown in the air, flying drone(s), running pets, etc. The object's state has to be updated at each time instance. Moving object localization is not a simple task even with precise localization information. The challenge increases when the environment is cluttered with obstacles. The obstacles can be detected using two approaches that rely on prior mapped knowledge of the targets or the environments [33,37,48,180,244]. These are the (i) Feature-based approaches that use LiDAR and detect the dynamic features of the objects; and (ii) Appearance-based approaches that use cameras and detect moving objects or temporally static objects. Autonomous vehicles must be able to navigate their environment safely. We can broadly classify obstacle avoidance into static and mobile obstacle avoidance [245,246]. As the name suggests, static obstacle avoidance deals with navigating around obstacles that do not move, and only the autonomous vehicle is in motion. Static obstacle avoidance is a process of establishing the temporal and spatial relationship between the mobile vehicle and the immobile obstacles—for example, a sofa in a living room. In contrast, mobile obstacle avoidance is a process of establishing the temporal and spatial relationship between the mobile objects in the environment, in addition to the vehicle and stationary objects. While path planning requires the vehicle to go in the direction nearest to the goal [223], and generally the map of the area is known, obstacle avoidance entails selection of the best direction among several unobstructed directions in real-time. Some of the obstacle avoidance techniques are as follows: static obstacle avoidance, mobile obstacle avoidance. Some of the approaches are grid-based, topological, hybrid, vector field histogram, dynamic window, and neural network-based approach.
Integration of Sensor Data for Autonomous Navigation
In this section, we discuss the four subtasks that are prominently used in autonomous navigation.
Figure 3. High-level Perception Architecture.
Mapping
We discuss several algorithms in our survey paper including the ones developed by Thrun [190]. Wherein he presented a novel algorithm which is strictly incremental in its approach. The basic idea is to combine posterior estimation with incremental map construction using maximum likelihood estimators. This resulted in an algorithm that can build large maps in cyclical environments in real-time, even on a low footprint computer like a micro-computer.
The posterior estimation approach enables robots to localize themselves globally in maps developed by other linked robots and thus making it possible to fuse data collected by more than one robot at a time[165,176]. They extended their work to generate 3D maps, where multi-resolution algorithms are utilized to generate low complexity 3D models of indoor environments. The assumption is that, when a robot receives a sensor scan, it is not likely that an obstacle is perceived in future measurements when it scans space previously perceived as free. The likelihood is inversely proportional to the distance between previous and current measurements
Localization
Localization of an autonomous vehicle typically uses sensors like GPS, odometric, IMU with magnetometer, accelerometer, and so on. The data fusion in these sensors is challenging due to the presence of drift, as in a GPS module. The data fusion should also consider the drift and counter it with applicable measurements to have the system localize itself [96] accurately. The input-output method of data fusion, first proposed by Dasarathy et al. [96]. After the data are successfully fused in the perception module, the information is passed on to the control module and the control module iteratively uses this information. When the data fusion system detects an obstacle, it passes this information as well to the controller, and it invokes the obstacle avoidance segment as required. Consider simultaneous localization and mapping (SLAM). In SLAM, the integrated output of the perception module is input to Zhang et al. [257], who proposed a robust model that used the MM-estimate technique for segment-based SLAM in dynamic environments. The raw 2D laser rangefinder data were split into laser segments and enhanced with outliers of the moving objects
Path planning
Path planning is an important task in autonomous navigation in which a system can perform global planning using pre-existing maps or local planning when no maps exist a~priori. This means that the path planning is dependent on mapping. In cases where the autonomous vehicle encounters static or moving obstacles, it uses obstacle avoidance techniques. Hence, the usage of sensors is vital. Some researchers developed a vision-based sensor fusion platform for path planning on a mobile robot. They use a pseudo-range processing method for vision-based sensor fusion using heterogeneous sensors [220,221,222,223]. They also use precise GPS, inertial, and orientation sensors.
Wang et al. [260] developed a vision-based sensor fusion platform for path planning on a mobile robot. They use a pseudo-range processing method for vision-based sensor fusion using heterogeneous sensors. They also use precise GPS, inertial, and orientation sensors. Ali et al. [261] developed an approach for a three-wheeled mobile robot in the online navigation of road following and roundabout environments. They developed a complete planner in which the sensor fusion was used to remove noise and uncertainties from the sensors. The motion controller was used to control the kinematics of the vehicle by using a resolved acceleration control integrated with an active force controller to reject high disturbances. Sabe et al. [264] used occupancy grids to find the path from robot source or current location to its goal; using this, the robot can safely reach the target location. They achieve this by defining every occupancy grid cell as a node that connects to a neighboring cell and also defines the path planning problem as a search problem, using an A* search algorithm.
Obstacle detection
In addition to cameras, LiDARs can be used to detect objects. A 3D point cloud is an output from the LiDAR. For efficient operation, the autonomous vehicle needs accurate data from each of its sensors. The reliability of the operation of an autonomous vehicle is hence proportional to the accuracy and hence the quality of the associated sensors. Each type of sensor has its limitations. Table \ref{fig:sensor_character} gives a comparison of the sensor types and their properties that are useful for navigation tasks [30,32,33,35,170].
Sensor data fusion is effective whenever multiple sensors (homogeneous or heterogeneous) are utilized and data fusion is not limited to the field of robotics [215] and in fact surveillance [268], gesture recognition [18], smart canes [7], guiding glasses [269] use this concept efficiently. The effective temporal, spatial, and geometrical alignment of this suite of heterogeneous sensors and the diversity utilization is called sensor data fusion [38,39]. Depth perception cameras provide limited depth information in addition to data-rich image data. Although cameras have the advantage of providing extremely rich data almost equivalent to the human eye, they need significantly complex machine vision techniques that require high computing power. In addition to his challenge, the operational limitation can be attributed to adequate lighting and visibility. Cameras are used very efficiently in detecting sign recognition, pedestrian detection [171,270], lane departure [271], identification of objects [116,272,273]. Cameras are much cheaper compared to radars or LiDARs [28]. Hence the community prefers them over other sensors in certain applications. Both LiDARs and Depth Cameras contain depth-sensing sensors. While the cameras estimate the depth information using disparity information in the image, the LiDAR generates depth information from the environment. Each sensor has its pros and cons. The depth cameras provide rich depth information, but their field of view is quite narrow. In contrast, the LiDARs contain an excellent field of view but do not provide rich environment information and instead provide sparse information [214,269,274]. The LiDAR provides information in the form of point cloud while the camera gives luminance We can see that these sensors can complement each other and can be used in complex applications. This is the advantage that we focus on in this study. Caltagirone et al. successfully developed a neural network that detected the road [93]. They projected an unstructured and sparse point cloud on the camera plane and un-sample it to obtain a set of dense 2D images. Multiple CNNs were trained to detect the roads. They found out that the fused data from the two sensors were better in terms of data accuracy and detail as compared to the individual sensors.
Applications of data fusion
Autonomous mobility systems
As the name sistive Autonomous mobility suggests, this is a distributed fusion system and is often used in multi-agent systems, multisensor systems play, and multimodal systems[84,94,95]. Efficient when distributed and important role in the lidecentralized systems are present. An optimum fusion can be achieved by adjusting the decision rules. However, there are difficulties in finalizing decision uncertainties.Decision Fusion TechniquesThese techniques can be used when successful target detection occurs [87,92,93]. They enable high-level inferences of persons who are unable to u for such events. When a user has a situation where multiple classifiers are present, this technique can be used. A single decision can be arrived at using the multiple classifiers. For the enablement of multiple classifiers to be achieved, apriori probabilities need to be present and this is difficult.
6.7. HardwareLiDAR
Light Detection and Ranging (LiDAR) is a te their limbs, especially thechnology that is used in several autonomous tasks and functions as follows: an area is illuminated by a light source. The light is scattered by the objects in that scene and is detected by a photo-detector. The LiDAR can provide the distance to the object by measuring the time it takes for the light to travel to the object and back [104, 105, 106, 107, 108, 109 ].CameraThe types of cameras are Conventional color lcameras like USB/web camera; RGB [115], RGB-mono, and RGB cameras wer ith depth information; RGB-Depth (RGB-D), 360 degree camera [28,116,117,118], and Time-of-Flight (TOF)camera[119,120,121].Implementation of Data Fusion using a LiDAR and cameraWe review an input-output type of fusion as described by Das. In continuation of our dataarathy et al. They propose a classification strategy based on input-output of entities like data, architecture, features, and decisions. The fusion of raw data in the first layer, a fusion of features in the second, and finally the decision layer fusion. In the case of the LiDAR and camera data fusion, two distinct steps effectively integrate/fuse the data.
Geometric Alignment on research, autonomous mof the Sensor DataThe first and foremost step in the data fusion methodology is the alignment of the sensor data. In this step, the logic finds LiDAR data points for each of the pixel data points from the optical image. This ensures the geometric alignment of the two sensors [28].Resolution Match between the Sensor DataOnce the data ility vehicles are in the processs geometrically aligned, there must be a match in the resolution between the sensor data of the two sensors. The optical camera has the highest resolution of 1920 × 1080 at 30 fps, followed by the depth camera output that has a resolution of 1280 × 720 pixels at 90 fps, and finally, the LiDAR data have the lowest resolution. This step performs an extrinsic calibration of the data. Madden et al. performed a sensor alignment [126] of a LiDAR and 3D depth camera using a probeiabilistic approach. De Silva et al. [28] performed a resolution match by finding developed. These vehicles are smart wheelchairs ta distance value for the image pixels for which there is no distance value. They solve this as a missing value prediction problem, which is based on regression. They formulate the missing data values using the relationship between the measured data point values by using a multi-modal technique called Gaussian Process Regression (GPR), developed by Lahat et al. [39]. The resolution mat ching of two different sensors can be controlled by human thought and will assist the disabled in a range of indoor mobility tasksperformed through extrinsic sensor calibration. Considering the depth information of a liDAR and the stereo vision camera, 3D depth boards can be developed out of simple 2D imagesChallenges with Sensor Data FusionSeveral challenges have been observed while implementing multisensor data fusion. Some of them could be data related to like: complexity in data, conflicting and/or contradicting data, or they can be technical such as resolution differences between the sensors, the difference in alignment between the sensors, etc. We review two of the fundamental challenges surrounding sensor data fusion, which are the resolution differences in the heterogeneous sensors and understanding and utilizing the heterogeneous sensor data streams while accounting for many uncertainties in the sensor data sources [28, 39]. We focus on reviewing the utilization of The users will be able to command the wheelchair(s) by just thinking of their destinationthe fused information in the autonomous navigation, which is challenging since many autonomous systems work in complex environments, be it at home or work, which is to assist persons with severe motor disabilities to handle their navigational requirements and hence pose significant challenges for decision-making due to the safety, efficiency, and accuracy requirements. For reliable operation, decisions on the system need to be made by considering the entire set of multi-modal sensor data they acquire, keeping in mind a complete solution. In addition to this, the decisions need to be made considering the uncertainties associated with both the data acquisition methods and the implemented pre-processing algorithms. Our focus in this review is to survey the data fusion techniques that consider the uncertainty in the fusion algorithm.
Some researchers Wuse will be integratid mathematical and/or statistical techniques for data fusion. Others used techniques comprised of reinforcement learning in implementing multisensor data fusion [70], where they encountered conflicting Brain Computing Interface(BCI) into this s data. In this study, they fitted smart mobile systems with sensors that enabled the systems to be sensitive to the environment(s) they were active in. The challenge they try to solve is mapping the multiple streams of raw sensory data Smart agents to their tasks. In~their environment, the tasks were different and conflicting, which complicated the problem. This resulted in their system learning to translate the multiple inputs to the appropriate tasks or sequence of system to enabactions. Crowel et al. developed mathematical tools to counter uncertainties with fusion and perception [133]. Other implementations include adaptive learning this echniques [134], wherein the authors use D-CNN techniques in a multisensor environment for fault diagnostics in planeaturetary gearboxes.Sensor data noise and rectificationNoise filtering techniques include a suite of Kalman filters and their variations.
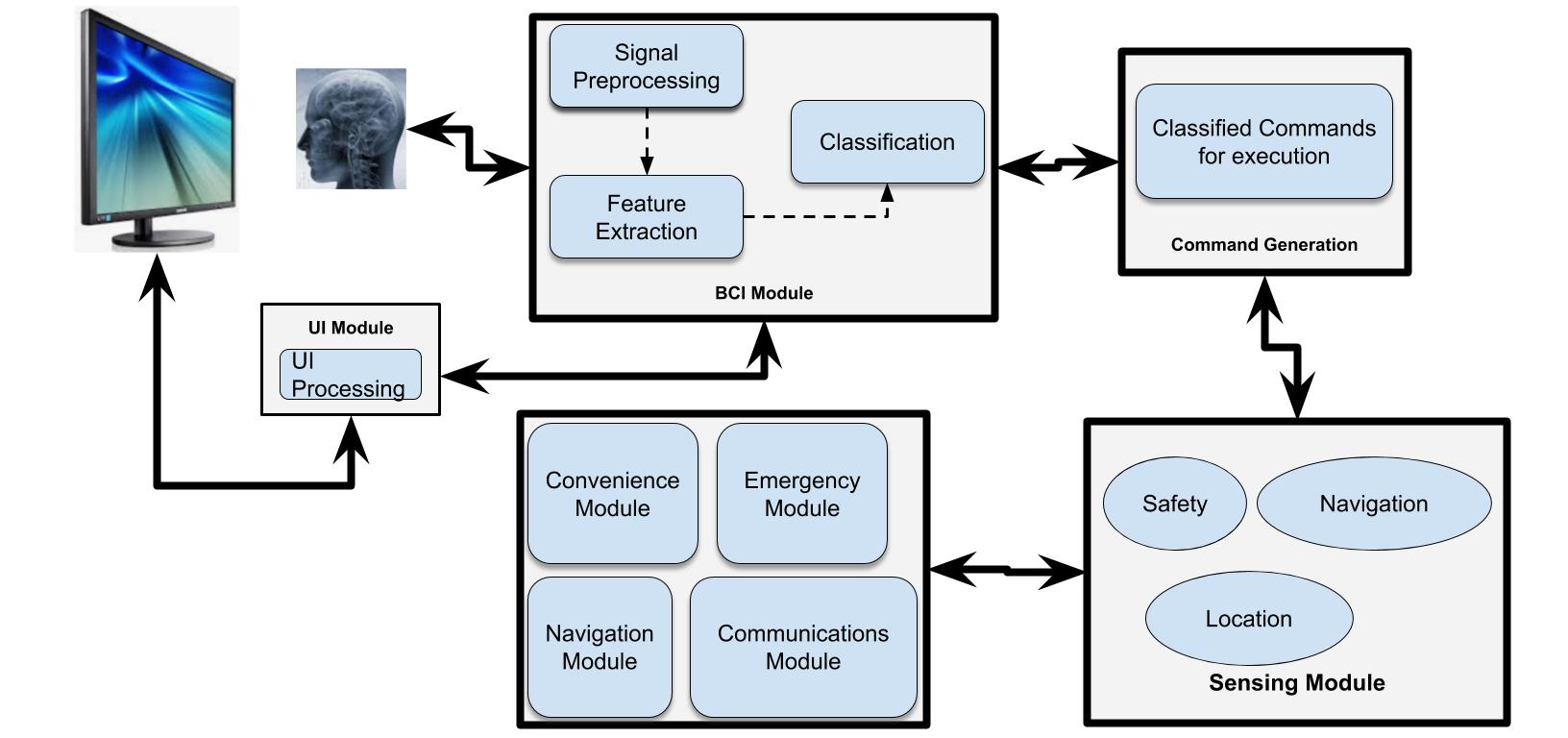
Pedestrian detection during autonomous vehicle navigation
Vehicles like the town busses, inter-city busses, or interstate busses can be fitted with the above-mentioned sensors in order to warn the drivers or autonomously avoid pedestrians and hence averting fatal accidents. Especially in cities like Newyork, NY, Los Angeles, CA, Chicago IL, San Antonio, TX, have a higher number of pedestrians during office/ peak hours [171,172]. There have been instances of pedestrians getting hit by the City vehicles. The city of San Antonio. Although Pedestrian detection is predominantly based on cameras and the usage of neural networks(NN), if the vehicle has only split seconds in the order of 100 Milliseconds, we will need to augment the camera data with LiDAR data. The first layer of the LiDAR could be implemented to detect the pedestrian quickly and obtain the dimensions. The camera data could be used to get finer details of the pedestrians, such as the face height, etc for further usage.
Intelligent Agriculture
Sensor data integration is being successfully implemented in the area of smart/intelligent agriculture. Farm vehicles like tractors, tillers, wagons, harvesting vehicles, and so on are being automated, and one of the main areas is navigation around the farm. The farms like vineyards have an acute problem of precision farming and an accurate, robust autonomous navigation technique is of the essence. Some of the key sensors are cameras, LiDAR, sonar, thermal cameras, IMU, GPS, to name a few. This is an area in autonomous systems, that has exploded in the number of applications over the past 5 years. For the purpose of precision farming, topological mapping is used. Topology mapping is performed usually with LiDAR; however, for activities like vineyard mapping or crop row detection, the LiDAR is coupled with a thermal camera or a high-resolution imaging camera. The mapping is usually represented as graphs and is based on connectivity, the environmental structure, and dense surface information [173]. Topological approaches determine the position of the robot relative to the model primarily based on the environment’s landmarks or distinct, the temporal sensor features [176].
7. Conclusion
LiDAR and Camera are two of the most important sensors that can provide situation awareness to an autonomous system which can be applied in tasks like mapping, visual localization, path planning, and obstacle avoidance. We are currently integrating these two sensors for autonomous tasks on a power wheelchair. Our results have been in agreement with past observations that an accurate integration does provide better information for all the above mentioned tasks. The improvement is seen since both sensors complement each other. We have been successful in using the speed of the LiDAR with the data richness of the camera.
We used a 3D Lidar Velodyne and integrated it with an Intel Realsense D435. Our results showed that the millisecond response time of the LiDAR when integrated with the high resolution data of the camera, gave us accurate information to detect static or moving targets, perform accurate path planning, and implement SLAM functionality. We have plans to extend this research to develop an autonomous wheelchair controlled by brain computing interface using the human thought.
LiDAR and Camera are two of the most important sensors that can provide situation awareness to an autonomous system which can be applied in tasks like mapping, visual localization, path planning, and obstacle avoidance. We are currently integrating these two sensors for autonomous tasks on a power wheelchair. Our results have been in agreement with past observations that an accurate integration does provide better information for all the above mentioned tasks. The improvement is seen since both sensors complement each other. We have been successful in using the speed of the LiDAR with the data richness of the camera.
We used a 3D Lidar Velodyne and integrated it with an Intel Realsense D435. Our results showed that the millisecond response time of the LiDAR when integrated with the high resolution data of the camera, gave us accurate information to detect static or moving targets, perform accurate path planning, and implement SLAM functionality. We have plans to extend this research to develop an autonomous wheelchair controlled by brain computing interface using the human thought.