Water diffuses freely and isotropically in most conditions. When impenetrable barriers are encountered, it becomes anisotropic with an ellipsoidal shape
[23][11], with rapid, unrestricted diffusion by the boundaries and slower, constrained diffusion vertical to the boundaries, based on the cell size and density. Diffusion in the ductal/glandular system is rapid due to the breast’s high water content and low cell density. When cancer cells obstruct the ducts and lobules, water transport is restricted, decreasing diffusion coefficients in all directions
[24][12]. Although DWI does not detect this ellipsoidal shape, DTI has overcome this restriction. Theoretically, in DTI, at least six independent diffusion gradients measurements accompanied by six non-collinear directions are needed to evaluate all diffusion tensor elements
[23][11]. At present, the most commonly used invariant anisotropy test is fractional anisotropy (FA)
[25][13]. However, the FA has defined no complete tensor form or distribution because different combinations of eigenvalues will produce the same FA values.
In diffusion, forms of pulse sequences include spin-echo, stimulated-echo, and steady-state free precession (SSFP). A spin-echo pulse sequence is the most common method for producing MR imaging sensitive to diffusion. The diffusion-weighted stimulated echo sequence is important for tissues with short T
2 relaxation times (liver). It can be combined with reading techniques, such as echo-planar imaging (EPI) or spiral imaging. To mitigate the effects of subject motion and maintain a high signal-to-noise ratio (SNR), the EPI method is used to achieve fast image acquisition. Echo-planar imaging is vulnerable to artifacts such as ghosting, chemical shift, and distortions
[26][14].
2. DWI in the Field of Diagnostic Breast Cancer
Diffusion (Brownian motion) is a term that refers to the arbitrary, tiny development of water and other small molecules due to thermal excitation. When diffusion is similar in every direction, it is isotropic and is denoted by a single diffusion coefficient (D) as follows:
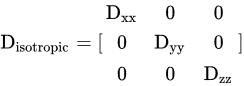
where (
Dxx =
Dyy =
Dzz =
D).
The signal intensities in DWI are:

where diffusion trace is expressed as
Dxx+Dyy+Dzz and average trace is ADC.
It is noteworthy that ADC in the breast (fibro-glandular tissue) is equal to 2.37 × 10
−3 mm
2/s
[29][15]. DWI can be obtained by placing two additional diffusion-sensitizing gradients on each side of a spin-echo sequence’s 180° radiofrequency (RF) pulse. The diffusion weighting’s magnitude is achieved as follows:
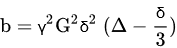
where
γ
is the gyromagnetic ratio, G is the magnitude of the diffusion sensitizing gradients, δ is the temporal duration of each gradient, and Δ is the time interval between the application of the gradients.
The ADC value is a function of the chosen b values. Thus, when greater b values are used, the ADC values become smaller, and the specificity of DWI can be increased by higher b values
[13][16]. Images with a high b value exhibit a decreased SNR and higher image distortion
[30][17].
In a study, Dorrius et al. evaluated the b value’s effect and the contrast’s pre-admission on the diagnostic accuracy using 1.5 T for breast DWI. According to their research, b = 0 accompanied by 1000 s/mm
2 is suggested to distinguish between normal and cancer tissues when time constraints allow only diffusion images obtained at a couple of amounts of b
[16][18]; however, high b-value DWI accompanied by conventional MRI sequences can facilitate the diagnosis closely to DCE-MRI for breast cancer detection
[31][19]. In many studies, it has been hypothetically shown that the ideal pair amounts of b to alleviate noise effects are in the range of 0 to 1000 s/mm
2 in the brain (with moderately slow diffusion), while 0 and 800 s/mm
2 are ideal in most low-water-content tissue such as breast
[32,33][20][21].
Diffusion-weighted imaging signal is generally T
1- and T
2-weighted, and higher-water-content lesions may have a high brilliance on DWI pictures; however, this is because of their exceptionally high T
2 signal and is not associated with diffusion (T
2 shine-through effect). One way to deal with this issue is to measure the ADC, which relies solely on diffusion
[34][22].
The common readout for breast DWI is single-shot EPI sequences, and readout segmented multi-shot EPI sequences. Echo planar imaging sequences are fast and insensitive to move, but they are vulnerable to geometric distortions that are most noticeable in the direction of phase encoding, leading to lower precision of quantification
[36][23].
In parallel imaging, the k-space lines are omitted, and the distance between them is increased.
Parallel imaging is widely used at a magnetic field strength of 3 Tesla since susceptibility effects are increased at greater field strengths. However, applying them at magnetic field strength 1.5 Tesla provides other advantages, such as increasing the number of slices for the same T
R and decreasing acquisition time. Although parallel imaging can reduce artifacts by decreasing the length of the echo train and hence reducing the T
2* blurring, it is constrained by the hardware of the RF coil
[40][24].
In rFOV, sharp images of a target region can be obtained by decreasing the matrix size, resulting in reduced susceptibility artifacts and higher spatial resolution, with the tradeoff of increased acquisition time, compared to single-shot EPI DWI. The depiction of lesion boundaries and heterogeneity partitioned from the encompassing fibro-glandular or adipose tissue can be troublesome with conventional EPI
[7]. However, the latest research on breast imaging with rFOV DWI has demonstrated that the lesion visibility is increased, image quality is improved, and high resolution is obtained in comparison to images acquired with conventional bilateral DWI, representing them as a viable alternative of DCE-MRI in breast cancer patients
[41][25].
3. Comparison of DWI with Other Modalities
Generally, breast cancer can be examined with various techniques such as ultrasound, mammography, perfusion, and diffusion. According to many cohort and systematic studies, the sensitivity of MRI is higher than mammography, ultrasound, and a combination of ultrasound and mammography
[42,43,44,45,46][26][27][28][29][30].
Many studies have shown that MR imaging has 91–100% sensitivity, and the specificity of DCE-MRI is about 37–97%
[43,47][27][31]. Dynamic contrast enhancement MRI has disadvantages, such as a high cost, and cannot be applied to renal dysfunction patients. Based on the literature, among these techniques, DWI helps distinguish between benign and malignant breast lesions
[48,49][32][33].
Zhang et al. conducted a study to determine which DCE-MRI, DWI, and T
2-weighted image descriptors are most closely related to breast cancer diagnosis. Their study set the b value to 50 and 850 s/mm
2. On DWI, malignant lesions exhibited a significantly lower average ADC mean (0.90 × 10
−3 mm
2/s) than benign lesions (1.43 × 10
−3 mm
2/s). They showed that DCE-MRI and DWI quantitative and qualitative variables are included in a multi-parametric MRI modality for breast cancer diagnosis. Indeed, they noted that models using the American College of Radiology (ACR) provide high diagnostic accuracy. Breast Imaging Reporting and Data System (BI-RADS) descriptors of margins and enhancement kinetics on DCE-MRI and ADC mean (either with DWI using a cutoff value or as a continuous variable) are mainly connected with a breast cancer diagnosis. Conventional T
2-weighted imaging did not remarkably contribute to breast cancer diagnosis
[51][34].
4. Different Models in DWI
As indicated in the literature, numerous models are available for extracting quantitative characteristics from images such as mono-exponential, intravoxel incoherent motion (IVIM) or bi-exponential, diffusion kurtosis imaging (DKI), stretched-exponential, Padé exponent (PE), statistical, and fractional-order calculus.
Igor Vidić et al. assessed different non-Gaussian representations of DWI signals for benign and malignant breast lesions in the b range of 200 to 3000 s/mm
2. According to their study, diffusion signal models provided parameters with a high area under the curve (AUC > 0.9) for classifying benign and malignant lesions. In their reports, the highest AUC of 0.99 was achieved for f (bi-exponential), K (kurtosis), and 0.989 for D (fractional calculus). Additionally, non-Gaussian representations are required for fitting the DWI curve at high b values in breast lesions. Moreover, the single voxel analysis showed that the SNR provided high classification accuracy for the statistical and fractional calculus diffusion model. Meanwhile, the other non-Gaussian representations gave lower classification accuracy than the mono-exponential model
[55][35].
5. DWI in Treatment Evaluation of Breast Cancer
There are many uses for treatment planning in DWI that can help detect and characterize tumors and predict and evaluate therapy response in malignancies, wherein hyper-cellular metastases and fibrosis-restricted diffusion is mainly observed
[9]. It is specified that tumors with a more significant mean ADC pretreatment are more likely to be necrotic
[58][36], and may therefore include more hypoxic areas. Additionally, diffusion continues to increase during radiotherapy because of cell membrane destruction and treatment-induced cell death
[59,60][37][38].
Mammography, sonography, and MRI can be used to check the size changes after neoadjuvant chemotherapy (NAC). MRI may be an effective strategy for studying the impact of NAC, and it may be accommodating to choose the extent of surgery. In this regard, the sensitivity of MRI in the initial staging of breast cancer and detecting the residual cancerous tissues following neoadjuvant chemotherapy was reported to be higher in comparison to breast-specific gamma imaging (83.9% to 76.8%), while their specificity was comparable, with 58.8% and 70.6%, respectively
[61][39]. The same results were acquired in the study by Lee et al., where the detectability rate of MRI for breast cancer recurrence was considerably higher in contrast to ultrasound imaging
[62][40]. However, in another study to investigate the detection efficacy of MRI in breast cancer patients for axillary lymph node metastasis, post-neoadjuvant chemotherapy, its sensitivity, specificity, and positive and negative predictive value fell short compared to ultrasound imaging
[63][41]. Moreover, the role of PET/CT for the expectation of pathologic reaction is not predominant in MRI
[64][42]. Therefore, there is a tendency to use MRI.
To direct treatment decisions, precise loco-regional staging and preoperative evaluation of breast cancer are considered crucial
[68][43]. In 2021, Hashem et al. assessed the role of DCE and DWI in the preoperative staging of breast cancer. It showed that DCE could detect the ductal carcinoma in situ (DCIS) component of the malignant lesions and give better tumor size and hence superior staging outcomes over sono-mammography. Moreover, they stated that qualitative DWI could be accompanied by ultrasonography to better assess the axillary nodal status.
6. DTI in the Diagnosis of Breast Cancer
When diffusion in specific directions is more than in other directions, it is called anisotropic. In anisotropic diffusion, it cannot use a single diffusion coefficient; instead, it need to use a diffusion tensor.

DTI assesses water diffusion in a minimum of six directions. Parameters of DTI are ADC or mean diffusivity (MD), fractional anisotropy (FA), mean axial diffusivity λ
1, mean radial diffusivity [(λ
2 + λ
3)/2], and empirical difference [λ
1 − λ
3].
The equation of ADC or MD is written as follows:
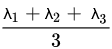
FA is given by:

Comparing DWI with DCE shows that DWI is faster than DCE
[3]. Moreover, DCE has false-positive results and unnecessary biopsies.
7. DTI in Treatment Evaluation of Breast Cancer
The potential of DTI and DCE to monitor response to neoadjuvant chemotherapy was assessed by Edna Furman-Haran et al.
[79][44]. In their study, the cancers scanned before neo-adjuvant chemotherapy in the three directional diffusion coefficients, λ
1, λ
2, and λ
3, and the mean diffusivity. They found that the maximal anisotropy and l1–l3 had lower levels in cancerous locations than normal tissue, which were reported in other studies, as well
[80,81][45][46]. They also reported an increase in the eigenvalues and mean diffusivity in response to neoadjuvant chemotherapy. Indeed, they showed that DTI can monitor alterations in the size and diffusion tensor parameters of breast cancer in response to neoadjuvant chemotherapy with an accuracy comparable to that of DCE
[79][44].
8. Amide Proton Transfer-Weighted Imaging in Breast Cancer Diagnosis
As a molecular imaging technique, amide proton transfer-weighted imaging (APTWI) measures the concentration of unbound proteins and polypeptides in tissue with no need for extrinsic contrast agents. Instead of relying on water molecule diffusion within tissues, APTWI’s capacity to represent lesion information is achieved by monitoring the chemical rate of exchange between water and amide protons
[84][47]. A study on the application of APTWI to assess breast lesions recently commenced. While several research findings have evaluated the utility of APTWI in tumor grading, cell proliferation
[85][48], and its importance in treatment-related lymphedema therapy
[86][49], few studies have examined APTWI’s effectiveness in diagnosing benign and malignant lesions and the connection among its parameters and prognostic factors
[87][50]. Meng et al. attempted to evaluate the roles of diffusion kurtosis imaging (DKI) and APTWI in distinguishing benign and malignant breast lesions and examine the correlation coefficients between the obtained parameters and breast cancer prognostic factors to develop novel concepts for breast cancer diagnosis, treatment, and prognostic assessment. The results indicated that the DKI and APTWI both offer useful information about the characteristics of breast lesions, apparent kurtosis coefficient (Kapp), non-Gaussian diffusion coefficient (Dapp), and magnetization transfer ratio asymmetry (MTRasym (3.5 ppm)), which are all viable variables for determining the microstructure of tissue, and overall, DKI was found to be superior to APTWI in discriminating benign from malignant breast lesions for three main reasons. Firstly, the discrepancies in water molecule diffusion in cancer cells are larger than the protein and polypeptide composition changes. Secondly, the key contributors to alterations in the protein and polypeptide content of the microenvironment remain unknown. Finally, the APTWI technique is still being developed, and scanning precision could be improved
[88][51].
9. Diffusion Kurtosis Imaging in Breast Cancer Diagnosis
While pure liquids and gels exhibit a Gaussian distribution of diffusion, obstacles induced by complicated tissue constructs effectively alter the probability distribution of diffusion. Kurtosis is the statistical term for assessing the true probability distribution within tissue. By obtaining supplementary images with a higher b-value (where b is an operator-defined parameter corresponding to the strength and duration of diffusion in imaged tissues), on the order of b = 1000–3000 s/mm
2 and at least 15 diffusion gradient directions, the diffusion kurtosis imaging method can trace numerous structures inside a single voxel, for example, crossing white matter fibers in the brain. In the case of breast imaging, diffusion kurtosis imaging is susceptible to intracellular structures such as membranes and organelles
[61][39] and can offer a diffusion heterogeneity index sensitive to tumor microstructure in addition to a mean kurtosis map
[62][40]. Notably, when the unsuppressed fat signal is corrected for, diffusion kurtosis analysis of the breast improves
[63][41].
10. Conclusions
Dynamic contrast-enhanced MRI (DCE-MRI) has high sensitivity and changeable specificity for breast cancer. DWI and its characteristics can show tumor cell thickness and microstructure or microvasculature at the cellular level without contrast agents. Recent findings have confirmed that DTI as an MR imaging modality has the potential for differential breast cancer diagnosis and could aid DCE-MRI and assess molecular subtypes in breast cancer detection. The diagnostic performance of new advances may be helpful in DTI, DWI, and DCE-MRI parameters and can help in the diagnosis of breast cancer in the early stages. Overall, recent findings showed that DWI and DTI and their characteristics may facilitate earlier and more accurate breast cancer diagnosis and treatment.