Artificial intelligence (AI) refers to machines, mainly computers, working like humans. In AI, machines execute tasks such as speech recognition, solving problems, and learning. Machines can work and act like humans if they have enough instruction and knowledge. Drug development is a costly and time-consuming business, and only a minority of approved drugs generate returns exceeding the research and development costs. As a result, there is a huge drive to make drug discovery cheaper and faster. With modern algorithms and hardware, it is not too surprising that the new technologies of artificial intelligence and other computational simulation tools can help drug developers.
1. Introduction
Artificial intelligence (AI ) systems can be divided into four groups based on machines’ ability to use past experiences to predict future decisions: (1) Machine learning refers to AI where machines are not explicitly programmed to perform tasks, but they learn and improve from training automatically. Deep learning is a subdivision of machine learning based on artificial neural networks for predictive analysis. (2) Natural language processing (NLP) is the interaction between computers and human language. (3) Automation and robotics aim to allow monotonous and repetitive tasks to be performed by machines. (4) Machine vision—machines can capture visual information and then analyze it [7][1]. AI has been used in applications to solve specific problems throughout industry and academia. Like electricity or computers, AI is a general-purpose technology that has a multitude of applications. It has enhanced language translation, image recognition, credit scoring, e-commerce, and many other aspects of our lives.
In the field of computer-aided drug discovery (CADD), the scientific community worldwide is regularly developing new technologies and algorithms to obtain hit compounds in a short time and reduce the overall cost [8][2]. Nowadays, the introduction of artificial intelligence (AI) and all the related techniques such as deep learning (DL), machine learning (ML), and other classical computational chemistry tools to drug discovery has had a significant effect on the success rate and velocity of novel pharmaceutical identification [9][3].
AI and classical CADD tools can be used alone or combined to produce new approaches that integrate a broad range of algorithms with enhanced prediction capabilities. CADD is classically classified into two methods: structure-based drug design and ligand-based drug design. Both are faces of the same coin and massively rely on force fields, scoring functions, and algorithms to evaluate and rank the studied molecules’ energy contribution in the targeted macromolecular biological system. While computer-aided structure-based drug design (e.g., docking) depends on the actual 3D structure of the targeted binding site of the targeted receptor protein to understand the stabilizing interactions at the molecular level between the studied ligand/receptor system, the ligand-based drug design (e.g., 3D-QSAR modeling) approach relies on the recognition of a database of already-known ligands interacting with the target receptor. Both structure- and ligand-based technologies have several success stories and play key roles in the drug modern drug discovery process [10,11,12,13,14][4][5][6][7][8].
2. Structure-Based Artificial Intelligence Methods for Small Molecules
The SARS-CoV-2 spike protein (S protein) is the leading mediator of viral entry into cells, and thereby infection, by binding the human angiotensin-II converting enzyme (ACE2) and therefore represents an attractive target for drug therapies
[16][9]. Recently, Srinivasan et al. developed a surrogate multi-task neural network (MTNN) model that replaces docking simulation in the finding of new molecules targeting the spike protein
[17][10]. Monte Carlo algorithms and recurrent neural networks (RNN) were used to explore the chemical space of millions of potential molecules using SMILES input. In this way, they discovered 97,973 new molecules, not included in the existing starting databases. The molecules were docked using Vina for their ability to bind the pocket region of the SARS-CoV-2 S-protein/ACE2 complex (SARS-CoV-2 S-protein (NCBI Reference Sequence YP_009724390.1)/ACE2 receptor (PDB ID: 2AJF)
[18][11]. The docking calculation search space was chosen as 1.2 nm × 1.2 nm × 1.2 nm and includes the binding pocket located at the S-protein/ACE2 interface
[19][12].
A new machine learning approach, namely SSnet, was used to identify new potential drugs by screening a library of approved drugs from the DrugBank and ZINC databases
[20][13] targeting two different conformations (open and closed) of the ACE2 receptor as well as ACE2 in complex with the S1 domain of the S protein, that is the protein responsible for binding with human cells
[21][14]. After cross-validation of the hits using the Autodock Vina scoring function
[22][15], the SSnet approach was extended to a library of 750,000 molecules in BindingDB to gain additional information regarding de novo drug design.
After that, to accelerate the identification of high-affinity scaffolds to test for their in vitro activity, a web interface, where molecules are grouped according to their similarity on a 2D map and colored based on binding affinity to the protein, was developed. This system allows selecting a certain point of the interface to explore the effect of singular scaffolds and functional groups on the binding score or affinity. Moreover, this approach can be applied to other therapeutical targets besides COVID-19.
High-throughput virtual screening (HTVS) coupled with ML experiments have been performed to obtain potential virtual inhibitors against the targeted protein rather than trusting commercial “corona-focused libraries”. The system was associated with an ML classification experiment where each compound is indexed into the chemical space of M
pro inhibitors, viral protease inhibitors, or a new chemical space. This approach has the advantage of taking into consideration potential drugs that would otherwise have been omitted and gaining information into the possible mechanism of action of the selected compounds
[23][16]. Initially, the ZINC 15 library
[24][17] was employed, and over 9 million compounds with a molecular weight below 200 g/mol were selected. The target SARS-CoV-2 M
pro crystal structure downloaded from the database has the PDB ID 6Y7M
[25][18]. HTVS docking was performed using CmDock docking calculations considering the QuickProp, QPlogS descriptor that indicates possible soluble compounds, and 200 hits were selected. A set of M
pro inhibitors and viral cysteine protease inhibitors collected in the ChEMBL database with experimental IC
50 < 100 μM were selected, and a set of 208 chemical descriptors were calculated to organize the compounds from the perspective of their representative chemical space
[26][19].
Using this HTVS method, a set of top-scoring compounds that could inhibit SARS-CoV-2 main protease were identified for further compound prioritization in biological evaluation experiments (
Table 1).
Table 1.
Selected top-scoring compounds by HTVS on the SARS-CoV-2 main protease.
n |
Structure |
CmDock Docking Score |
1 |
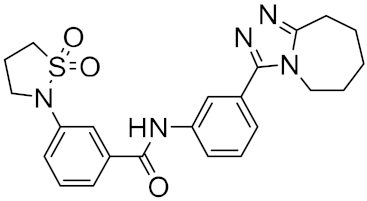 |
−32.51 |
2 |
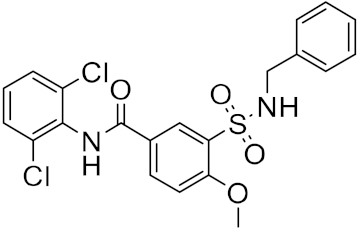 |
−29.02 |
3 |
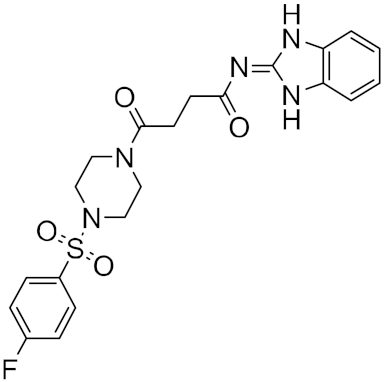 |
−26.80 |
4 |
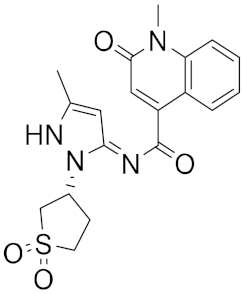 |
−25.58 |
5 |
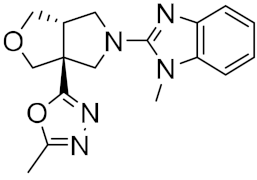 |
−25.53 |
6 |
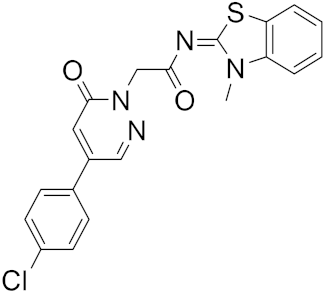 |
−25.05 |
7 |
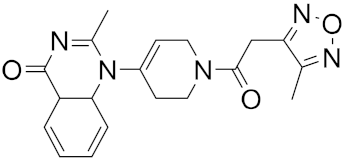 |
−24.76 |
8 |
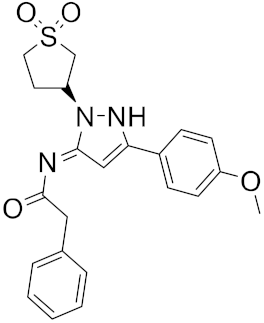 |
−24.51 |
9 |
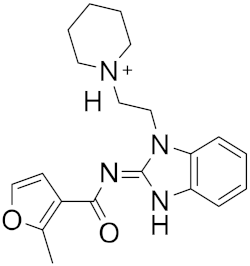 |
−24.17 |
12 |
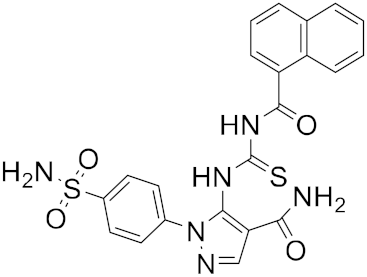 |
−24.01 |
11 |
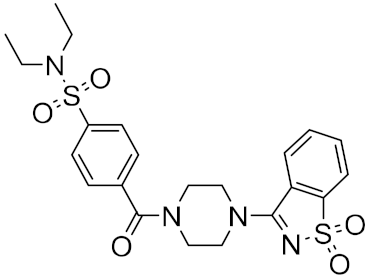 |
−23.98 |
12 |
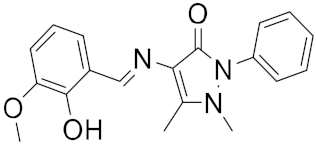 |
−23.61 |
13 |
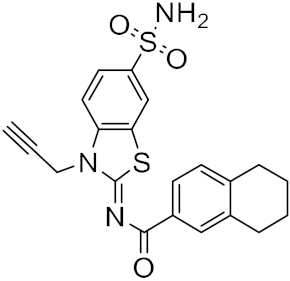 |
−23.53 |
14 |
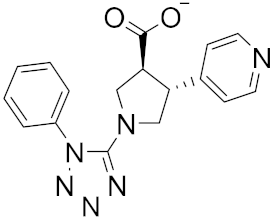 |
−23.26 |
15 |
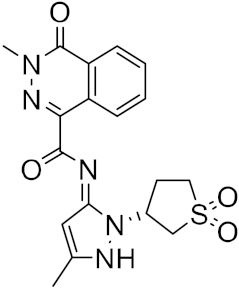 |
−23.18 |
A different research group screened a library of molecules for their potential ability to inhibit SARS-CoV-2’s main protease (Mpro) and the receptor-binding domain (RBD) of the spike protein by using the molecular docking software AutoDock Vina [27][20]. Mpro is a peculiar cysteine protease of the coronavirus family and has a crucial role in mediating viral replication and transcription. The absence of a homologous human protease makes this protein an important target against COVID-19 [28][21].
Recently, Born et al., devised a new method for discovering and synthesizing drugs against SARS-CoV-2
[30][22]. This procedure merges biology and chemistry for target-driven molecular design associated with an automatic synthesis plan generator and can be virtually applied to any protein target. The approach uses deep generative models that implement a conditional molecule generator to propose drug candidates by exploring the latent space of proteins and small molecules. The promise of this approach is the possibility to generalize to unseen targets.
In this way, a conditional molecular generator can produce novel structures expressly designed to target a protein of interest
[31][23].
The founders of COVID Moonshot, a non-profit, open-science consortium of scientists from around the world dedicated to discovering globally affordable and easily manufactured antiviral drugs against COVID-19, demonstrated the utility of a de novo design using machine learning with synthesis route prediction
[33][24]. The purpose
of the study was to generate potential new drugs targeting the main protease (M
pro) of the novel coronavirus. In fact, while classic approaches tend to modify existing compounds, exploring limited chemical space, this machine learning method searches in a larger chemical space. The inconvenience of this approach is the expense of synthetic accessibility, but this can be overcome using machine learning to predict the synthetic route.
Pirolli D. et al., with the support of machine learning approaches, reported a structure-based virtual screening as an effective strategy to discover inhibitors of protein–protein interactions (PPIs) between SARS-CoV-2 RBD and human ACE2 using the ZINC database
[42][25].
Using different ligand- and structure-based approaches, a customized virtual screening (VS) strategy was set up. The first step of the VS strategy was the selection of a library focused on small molecule PPIs from a dataset of 2 million compounds, using a ligand-based approach capable of recognizing chemical characteristics and scaffolds common to known modulators. For this purpose, a convolutional neural network (cNN) was trained to obtain a QSAR model capable of identifying potential PPI modulators within a virtual library of unknown molecules. The molecules classified by the cNN-based QSAR as potential PPI modulators were further filtered by the expected toxicological properties to discard compounds harmful to human health. The resulting virtual library was hooked to ACE2 to identify compounds with the best binding affinity for the spike protein interaction surface. The dataset of 30,029 ligands obtained from QSAR modeling and toxicity analysis against the druggable site-4 pocket region was used to screen for effective inhibitors of the protein–protein interactions of the SARS-CoV-2 RBD/ACE2 tip. Then a virtual Glide screening in SP mode was performed, and the next phase was done by XP docking. Based on the docking score, the first 15,015 classified ligands (50%) were selected and reassessed with Prime MM-GBSA to estimate their binding free energy. The compounds were then filtered based on distance constraints by selecting only small molecules within 4.5 Å away from any atom of Tyr83 and Gln24 residues. The remaining 9730 molecules were then grouped based on their diversity, and the resulting 973 virtual hit compounds were further assembled into 66 clusters using interaction fingerprints.
3. Ligand-Based Artificial Intelligence Methods for Small Molecules
F. Pereira et al., succeeded in predicting five new inhibitors against SARS-CoV-2 M
pro using a CADD method based on a quantitative structure-activity relationship (QSAR) classification model that was built from 5276 organic molecules extracted from the ChEMBL database. Virtual screening was then performed using 11,162 marine natural products (MNPs) retrieved from the Reaxys
® database. From the QSAR approach, 494 MNPs were selected and subsequently subjected to molecular docking against the M
pro. Among the evaluated compounds, five MNPs have been proposed as the most promising marine drugs as inhibitors of SARS-CoV-2 M
pro, among them a benzo[f]pyrano [4,3-b]chromene (Reaxys ID 7450892), notoamide I (Reaxys ID 19384758), hemindole SB beta-mannoside (Reaxys ID 26845562), and two derivatives of bromoindole (Reaxys IDs 10,714,788 and 10720065)
Figure 21 [43][26].
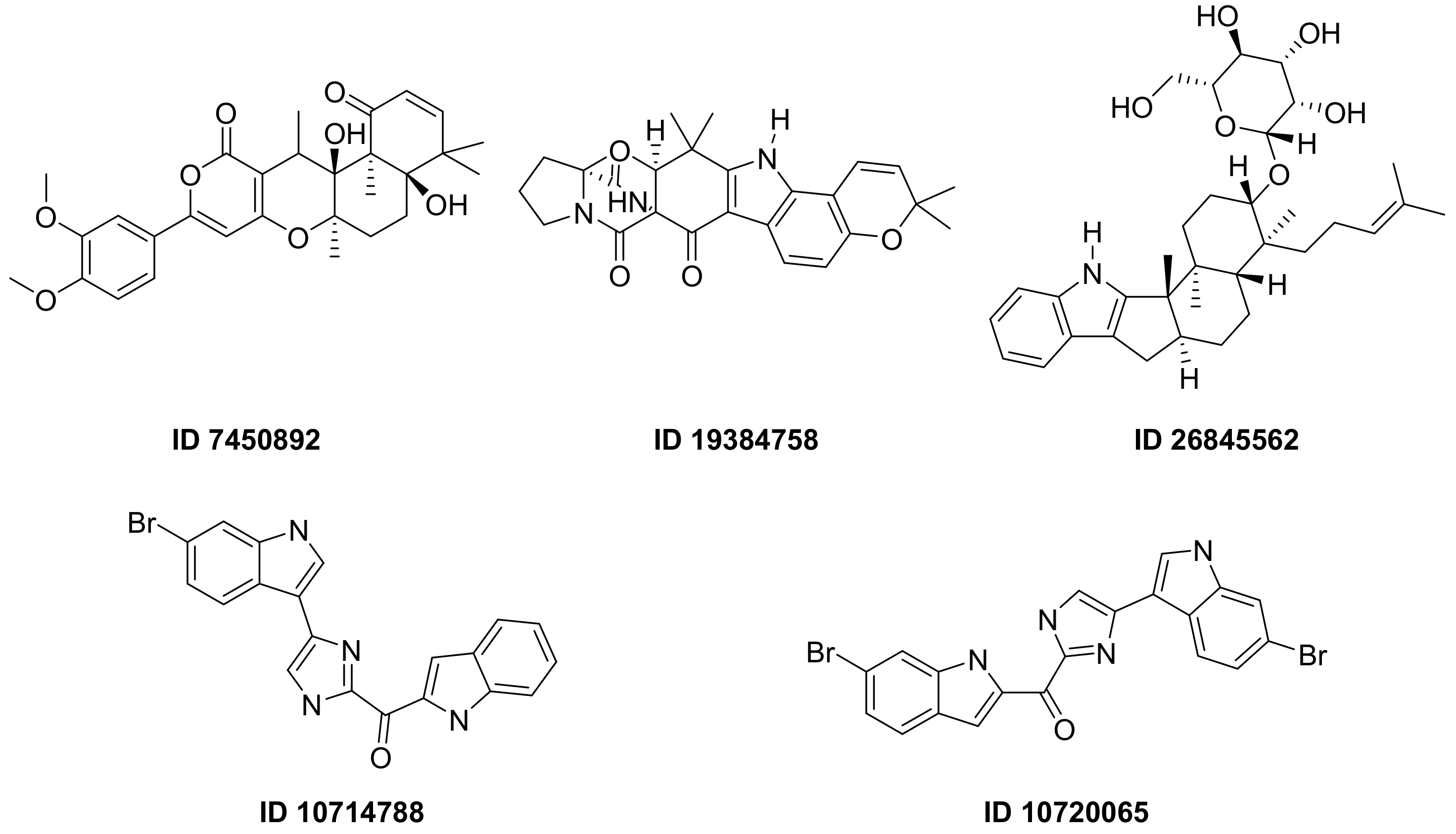
Figure 21. MNPs have been proposed as the most promising marine drug leads as inhibitors of SARS-CoV-2 M
pro.Combining a generative recurrent neural network model with transfer learning methods and active learning algorithms, R. Yassine et al. designed a novel set of small molecules capable of effectively inhibiting the 3CL protease in human cells
[44][27].
The novelty of this work is the use of active learning methods with generative recurrent neural networks (RNNs) containing long-term memory cells (LSTM). The active learning method facilitates the selection process by focusing on the areas of the chemical space that have the best chance of success, considering structural novelties. The authors built a database consisting of multiple datasets such as FDA-approved drugs (from the ZINC database), natural products (from SuperNatural), and a manually developed database representing drug-like bioactive molecules. In the first phase of this study, by applying the RNN deep learning methodology, the LSTM-based RNN model was created to generate reliable and high-quality SMILES to design new drugs. Subsequently, molecules structurally similar to drugs with known activity against the specific SARS-CoV-2 target were generated. In this way, they were able to find a model capable of discovering new drugs using fragment-based drug discovery (FBDD) to create a library containing a series of SMILES inspired by the well-known compounds. The model generated 25,000 small moleAmong these, four molecules
from the learned chemical space as described above. After removing duplicates and identical molecules from the database used for training, the remaining dataset consisted of 22,173 molecules. These molecules were then subjected to other filters such as physicochemical properties, drug similarity, and synthetic accessibility, resulting in a set of 6962 molecules. The generated molecules were then screened for affresulted in a binding affinity
to the 3CL protease. After the virtual screening, a total of 41 molecules were obtained, with a virtual screening score of less than −7.0 kcal/mol. Among these, four molecules resulted in a binding affinity score loscore lower than −18 kcal/mol (
Figure 32).
Figure 32.
The generated molecules by R. Yassine et al., with the lowest binding affinity scores.
The deep learning (DL) model can generate 1D or 2D sequences ligands structures. However, rational drug design requires 3D ligand structures that target the crystalline structure of proteins. To solve this problem, Q. Bai and coworkers developed MolAICal software, which allows to generate 3D drugs in the 3D pocket of protein targets by combining the merits of the deep learning model and classical algorithm. The software essentially consists of two modules. In the first one, FDA-approved drug fragments are used to train the Wasserstein-based deep learning model generative adversarial networks (WGANs). The generated fragments of the deep learning model are further used to grow the 3D ligands in the protein pocket. In the second module, drug-like molecules from the ZINC database are used to train the WGAN-based deep learning model. Then the affinities between the generated molecules and the proteins are evaluated through molecular docking experiments with AutodockVina. The membrane protein glucagon receptor (GCGR) and the non-membrane target SARS-CoV-2 M
pro were chosen to analyze the drug design capabilities of MolAICal. In this way, the software can generate various ligands that have an ever-higher 3D structural similarity with the ligand crystallized in the active site of GCGR or SARS-CoV-2 M
pro [49][28].
4. Artificial Intelligence Methods Vaccine Design
An in silico deep learning approach was proposed to predict and design a multiepitope vaccine (DeepVacPred), in combination with in silico immunoinformatics and deep neural network strategies. The DeepVacPred computing system directly predicted 26 potential vaccine subunits from the SARS-CoV-2 tip protein sequence
[54][29].
The DNN architecture to block 26 fragments in the SARS-CoV-2 spike protein as candidates for the vaccine subunit was the first step. Subsequently, linear B cell, CTL, and HTL epitopes were used to select and construct the final vaccine.
All overlapping protein fragments with a length of 30 aa were generated by the spike protein sequence 1273 aa SARS-CoV-2. DeepVacPred first tested these protein sequences and predicted 132 potential vaccine subunits. Following this prediction, DeepVacPred provided 26 potential vaccine subunits for further evaluation and construction. These subunits were those most likely to contain B cell epitopes and multiple T cell epitopes and have high antigenicity and low allergenicity.
In silico methods were used to study linear B cell epitopes, cytotoxic T cell epitopes (CTL), helper T cell epitopes (HTL) in the 26 candidate subunits.
The B cell epitopes, predicted on the 26 vaccine subunits, are parts of antigens that bind to immunoglobulin or antibody, capable of activating B cells to provide the immune response
[55][30]. Linear B cell epitopes were predicted from four online servers, including BepiPred
[56][31], SVMtrip
[57][32], ABCPred
[58][33], and BCPreds
[59][34]. First, they used BepiPred for the main forecast and the other three servers to check the results of the BepiPred forecast. Additionally, the proprietary RaptorX server was used to evaluate the surface accessibility of SARS-CoV-2 to validate that the B cell epitopes in those subunits were well exposed.
CTLs recognize infected cells using class I MHCs to bind to certain CTL 26 epitopes. The NetMHCpan 4.1 server
[60][35] 43 was used to predict potential CTL epitopes. All overlapping 9aa peptide sequences in the 14 vaccine subunits were tested with the 12 most common class I alleles of human leukocyte antigen (HLA), including HLA-A1, HLA-A2, HLA-A3, HLA-A24, HLA-A26, HLA-B7, HLA-B8, HLA-B27, HLA-B39, HLA-B44, HLA-B58, and HLA-B62, to evaluate their binding affinities and predict potential CTL epitopes
[61,62][36][37].
HTL helps other immune cells’ activity and recognizes infection by using MHC class II to bind with specific HTL epitopes
[63][38]. The NetMHCIIpan 4.0
[64][39] server was used to predict potential HTL epitopes. All overlapping 15aa peptide sequences in the 14 vaccine subunits were tested with the 13 most common HLA Class II alleles, including HLA-DRB1-0101, HLA-DRB1-0301, HLA-DRB1-0401, HLA-DRB1-0701, HLA-DRB1 -0801, HLA-DRB1-0901, HLA-DRB1-1001, HLA-DRB1-1101, HLA-DRB1-1201, HLA-DRB1-1301, HLA-DRB1-1401, HLA-DRB1-1501, and HLA-DRB1-1601, to evaluate their binding affinities and predict potential HTL epitopes
[64][39]. The total HLA score was calculated for each vaccine subunit.
The 3D structure of the designed vaccine was then predicted, refined, and validated by other in silico tools. The GalaxyRefine
[65][40] server was employed to refine the 3D structure model of the final vaccine. Among the five refined models predicted by GalaxyRefine, model 2 was chosen as the final vaccine model based on its quality scores with a reported RMSD of 0.58.
In conclusion, this proposed artificial intelligence (AI)-based vaccine discovery facility accelerated the vaccine design process and built a 694 aa multiepitope vaccine containing 16 B cell epitopes, 82 CTL epitopes, and 89 HTL epitopes, which promise to fight SARS-CoV-2 viral infection with good antigenicity, population coverage, and good physicochemical properties and structures, providing great potential for next stage of COVID-19 vaccine design with actual clinical trials.