The snowmelt model is a hydrological model that simulates the processes of snow accumulation, melt, and confluence. Since the snowmelt process involves the complex energy transfer and water transfer processes between the atmosphere and snow, between different snow layers, and between snow layers and soil, it is more complicated than the formation process of rainfall runoff. If there is a rainfall event on snow, it is very difficult to simulate the runoff generation process due to the mixture of rain and snow. Snowmelt simulation is the basis for the simulation of snowmelt flood formation processes, and it plays a crucial role in accurately modelling snowmelt floods.
1. Introduction
Hydrological models and hydraulic models are important tools for hydrology studies. The former focus on simulating the process of flow generation and convergence from the basin to the main river channel, while the latter focus on simulating the evolution process of water flow in the main river channel. Figure 1 shows the formation process of a snowmelt flood. Massive snow accumulation in mountainous areas may easily cause flooding once there is a rapid heating or rain event. In the meantime, mountainous terrain is conducive to the rapid concentration of snowmelt water. Therefore, when modelling snowmelt flood processes, it is better to couple two different types of models. The results of Hydrological modelling for different river sections can be used as input conditions for the hydraulic model, together with previous observations, so as to realize a rolling iterative flooding forecast [1][7].
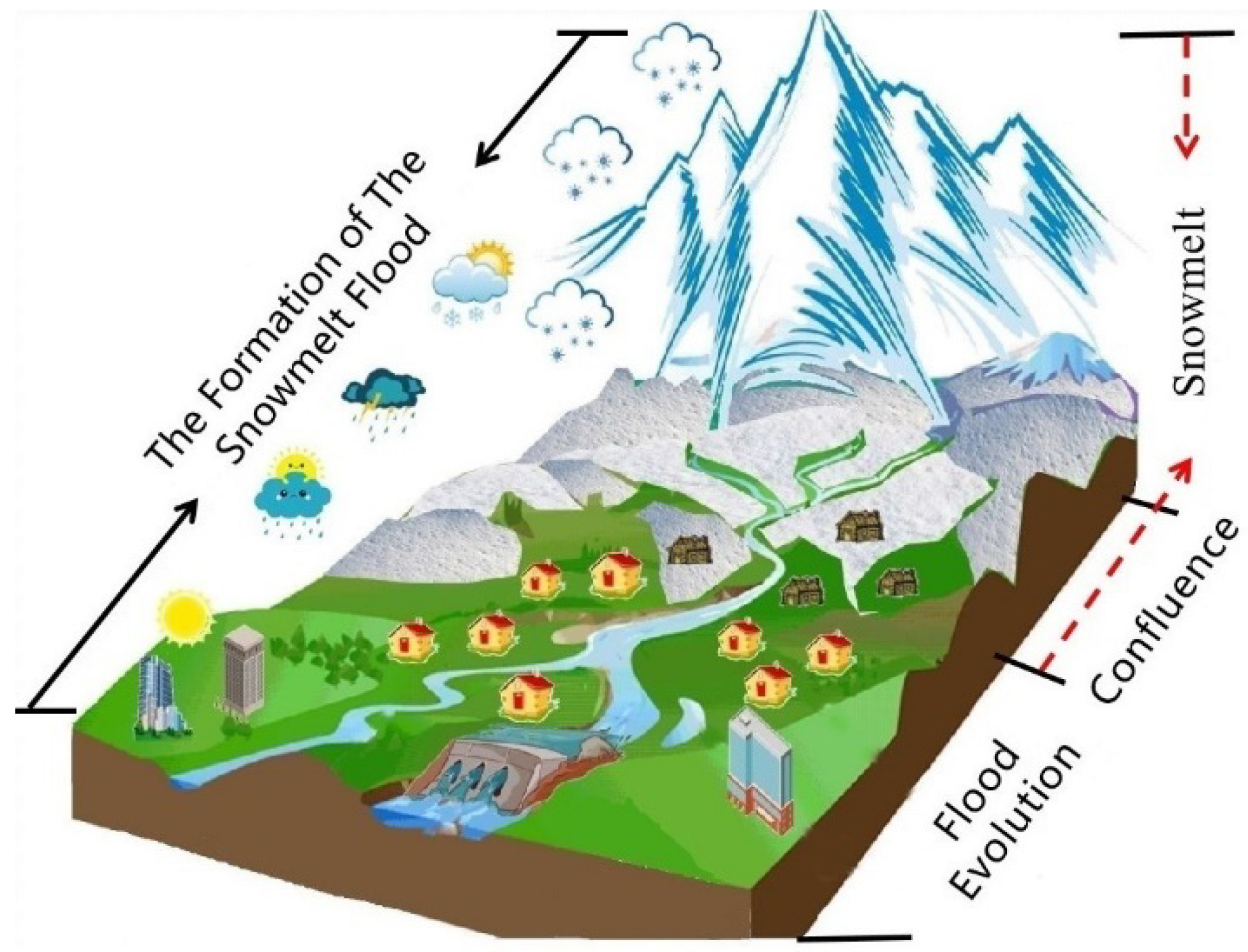
Figure 1. Snowmelt runoff is the first step in the formation of snowmelt floods (the snowmelt model is applied to the simulation of snowmelt runoff in the area marked by the red arrow).
One remaining issue of snowmelt flood forecasting is the low accuracy of early warnings
[2][5] for rain-snow-ice mixed floods, particularly under the background of rapid warming. A comprehensive review of the related progresses and challenges of snowmelt process models is critical to further development of the related field. Therefore, this study reviews the current status of different snowmelt models by extensively consulting relevant literature, summarizes the trends and challenges, and offers perspectives on the future of snowmelt flood simulation.
2. Categories of Snowmelt Models
According to the different ablation algorithms used, snowmelt models can usually be classified into four categories: statistical, conceptual, physical and data-driven (green categories in
Figure 2). Statistical snowmelt models use statistical methods or black box modules to establish the relationship between a certain snow hydrological characteristic parameter (e.g., snow area) and runoff to predict runoff. Conceptual snowmelt models usually establish an empirical relationship between snowmelt and temperature. These models, such as S
now R
unoff Model (SRM) M and HBV
[3][25], have physical meanings and are mature and widely used methods. The physical snowmelt models, such as
numerical layered snow thermal model (SNTHERM
) and physical snow pack model ( and SNOWPACK
), calculate snowmelt based on the energy balance of snow cover. They have strict physical meaning and have been widely used in distributed modelling of snowmelt. The emergence of data-driven models has benefited from the growth of massive data and the rapid increase in computational power. These models, Such as
artificial neural network (ANN)ANN [4][26] and
long short-term memory (LSTM
) [5][27], simulate the changes in snowmelt runoff using machine learning algorithms to select appropriate parameters (e.g., daily rainfall, temperature, solar radiation, snow area, snow water equivalent) from different data sources.
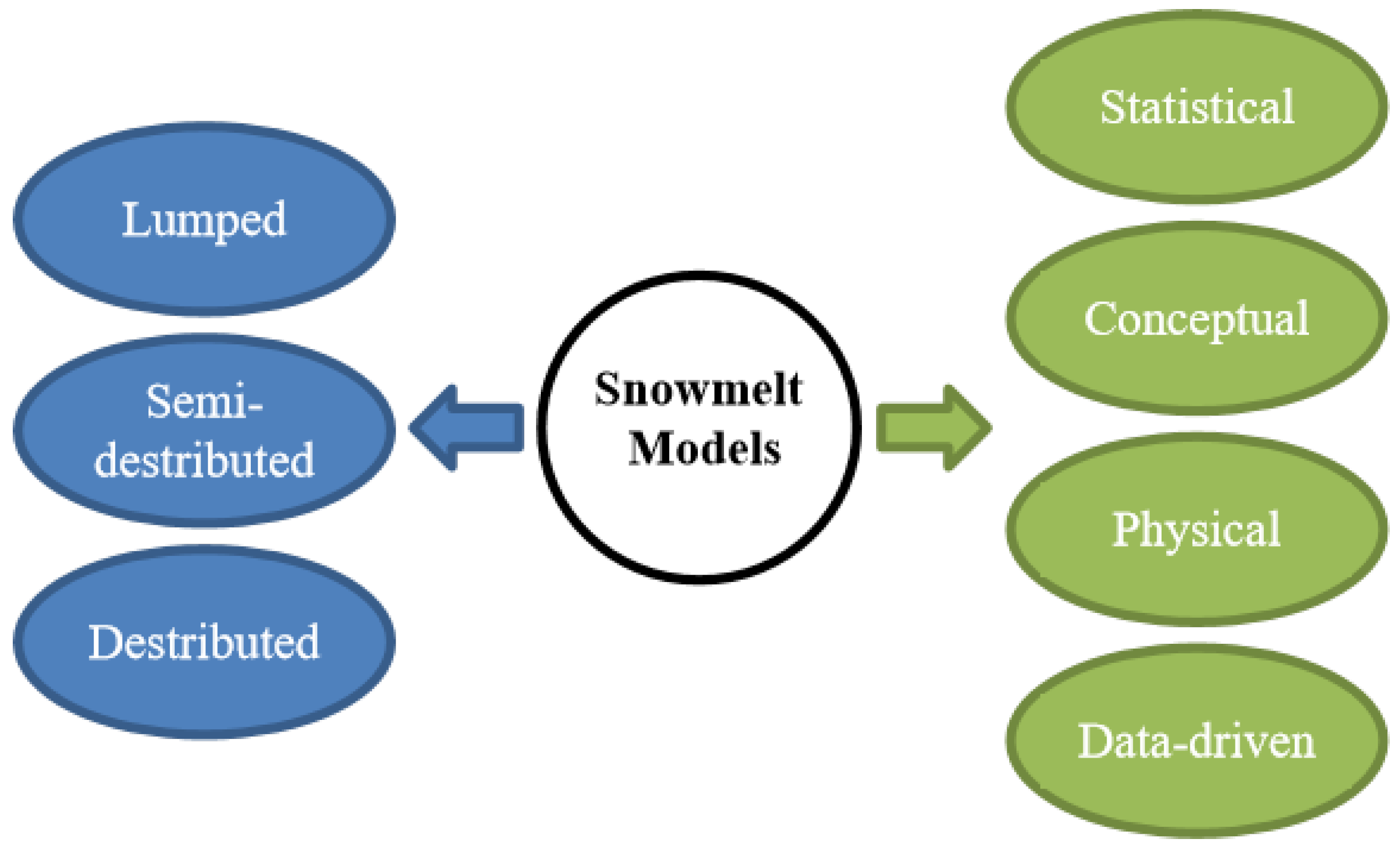
Figure 2. Categories of snowmelt models. Blue categories are derived according to the spatial distribution characteristics of the models. Green ones are derived according to different ablation algorithms.
According to the spatial distribution characteristics of models, the snowmelt model can be divided into lumped, semi-distributed and distributed models (blue categories in
Figure 2). Lumped models, representing by SRM, assume that snow cover and melting rate are consistent across the watershed or sub-basin. Semi-distributed models, including HBV, SWAT and the Precipitation-Runoff Modeling System (PRMS)
[6][28], usually divide the watershed into sub-watersheds or hydrological response units according to a certain characteristic of the watershed (e.g., elevation, vegetation coverage, land use types, and topographic factors). Distributed models, such as
European Hydrological S
ystem (SHE), Distributed Hydrology Soil Vegetation Model (DHSVM)HE, DHSVM, and V
ariable Infiltration Capacity (VIC)IC, divide the watershed into grids, and assign different parameter values to each grid and then include wind, snow, and soil freezing processes in each individual grid. In recent years, with the rapid improvement of computing power, multi-layer hybrid and nested models are appearing, such as the Water and Energy Budget-based Distributed Hydrological Model (WEB-DHM)
[7][29]. Sub-catchments, grids, and slopes are used for nested calculations, thereby improving the ability to adequately describe the snowmelt process.
From the perspective of modelling complexity, snowmelt models vary greatly. Therefore, when selecting a snowmelt runoff simulation model, it is necessary to comprehensively consider data availability, watershed characteristics, and time scale of simulation.
3. Challenges of the Snowmelt Models
Starting from the requirements of snowmelt flood forecasting, we sorted the existing challenges facing runoff generation models for snowmelt flood process simulation into four aspects: data, model, forecast and early warning, and prediction and estimation (
Table 14).
Table 14. Challenges of the snowmelt models.
Aspect |
Challenges |
1. Data |
1.1 High-resolution data (air temperature, precipitation, snow, vegetation, soil moisture, etc.) 1.2 Accurate snow related estimation (snow cover, snow depth, snow water equivalent, etc.) 1.3 Fusion of different data sets and accuracy evaluation |
2. Model |
2.1 Data assimilation 2.2 The reliability test of runoff simulation 2.3 Process modeling of rain on snow and river ice 2.4 Coupling blowing snow, frozen ground, rain-on-snow 2.5 Coupling with atmospheric model 2.6 Deep learning algorithms |
3. Forecast and early warning |
3.1 High-resolution, accurate weather forecast 3.2 Uncertainty of weather forecast 3.3 Uncertainty of Snow algorithm 3.4 Robustness of the snowmelt model to floods of different scales |
4. Prediction and estimation |
4.1 Uncertainty of climate change scenarios 4.2 Uncertainty of downscaling method 4.3 New phenomena such as ROS under rapid heating Carbon black, aerosol, etc. |
Snowmelt occurs mostly in mountainous areas in high latitudes, and the acquisition of various monitoring data is still a big problem. In particular, the acquisition of snow-related data with high temporal and spatial resolutions has serious limitations. Current automated measurements include sonic depth measurement, snow pillows, Gamma radiation measurement
[8][178], and cosmic ray neutron measurement
[9][179]. These automated methods have higher temporal resolution but lack important spatial variability due to their limited spatial footprints. LiDAR measurement
[10][180] and GPS interferometric reflectometry (GPS-IR)
[11][181] can measure snow cover in a small area, but cannot provide regularly repeated observations. Microwave remote sensing, InSAR techniques in particular, provides effective means to monitor snow cover in a large area, but how to balance the spatial and temporal resolution, and how to retrieve snow parameters in a complex terrain environment still require continuous exploration and research. At present, downscaling technology, data fusion methods, and deep learning algorithms provide us with a powerful way to obtain high-resolution data. In the future, we will need to establish three-dimensional air-space-ground monitoring networks to better monitor and simulate the snow melting process.
In the construction of the snowmelt model, we still need to consider more snow hydrological processes, such as blowing snow, frozen soil, and rain-on-snow mentioned above. In addition, the model needs to be designed to be more flexible and easier to couple with other models, such as climate models, land surface models, and hydraulic models, so that the model can simulate the snow melting process more reasonably and accurately.
The main purpose of snowmelt modeling is to provide more accurate snowmelt prediction simulation for early flood warning. Using the results of numerical weather forecasting as the input of the snowmelt model can help us predict the snowmelt runoff in advance
[12][182]. At present, the most advanced numerical weather prediction models in the world include the
the Fifth-Generation Penn State/NCAR Mesoscale Model (MM5)MM5 model jointly developed by Pennsylvania State University and the U.S. Center for Atmospheric Research
[13][122], the NCEP and WRF models developed by the National Environmental Center of the United States
[14][15][183,184], the European mid-term ECMWF model developed by the Weather Forecast Center
[16][185], and GRAPES developed by the China Meteorological Administration
[17][186], etc. Different models usually provide several modes, with different forecast time-space resolution and forecast time effect. For example, the ECMWF model has a forecast time effect of up to 15 days. Its high-resolution TL639L62 mode aims for a 0–10 day forecast and has a horizontal resolution of 30 km, while the lower resolution TL319L62 mode aims for a 10–15 day forecast with a horizontal resolution of 65 km. The reliability and uncertainty of numerical weather forecasting are major problems faced by flood forecasting. We should further study the construction of high-resolution numerical weather prediction to reduce the uncertainty and improve the accuracy of flood forecasting. At the same time, we are required to test the robustness of the snowmelt model to deal with floods of different scales, and better deal with the uncertainty of future snowmelt flood modelling.
Snow cover responds quickly to climate change. Under the background of global warming, snowmelt flood risk rises accordingly
[18][187]. Assessing the risk of snowmelt floods under future climate change scenarios is an urgent task. A major method of understanding the impact of climate change on floods is to couple climate models with hydrological models, and use future meteorological data to drive hydrological models to predict future flood risks
[19][188]. Future climate scenarios are a reasonable description of the temporal and spatial distribution of future climate states based on certain driving forces and scientific assumptions. This can be roughly divided into two categories: emission scenarios and radiative forcing (socio-economic scenarios). Many global climate models have been developed so far. They can give the overall trend of future climate change, however, there are considerable differences among the models. Uncertainties broadly exist in climate simulation, for example the uncertainty of the model itself, the uncertainty of the emission scenario, and the uncertainty of the regionalization technology
[20][189]. In addition to the uncertainties caused by the climate model, in the context of future climate change, new phenomena such as rain erosion and snow caused by rapid warming, carbon black, aerosols, etc., will also induce uncertainties to snowmelt models. The enhancement has also brought greater challenges to the future prediction of snowmelt floods.
4. Summary
The main conclusions are as follows:
(1) The physical distributed snowmelt model has high temporal and spatial resolutions, and strict physical meaning. The spatial scheme of the energy balance component and the hydrological parameters of the physical snowmelt model, and the research on the spatial scheme of forcing data, require further progress. This type of model will be an important tool and a key development object for the simulation of the snowmelt flood process as computing power increases in the future.
(2) Machine learning adds new tools to hydrological modeling. The integration of hydrological knowledge, process-based models and machine learning for snowmelt modeling can further improve the simulation capabilities.
(3) At present, most snowmelt models still lack wind-blown snow modules and frozen ground modules. An explicit consideration of the effects of blowing snow and soil freezing and thawing in the snowmelt modeling process is expected to enhance the capabilities of snowmelt runoff simulation in land surface models and hydrological models.
(4) In the context of climate warming, global ROS events have become more and more extensive and intense, bringing great challenges to the snowmelt runoff simulation. At present, the runoff-generation mechanism for rain and snow mixed floods remains unclear. Therefore, the observation and simulation of Rain-on-Snow (ROS) processes are an important direction in this field.