Constructing an accurate human body model from various types of input data, such as single images, multi-view images, videos, or depth maps, is a great challenge. Existing methods for fitting a pose to the input data typically rely on parametric, yet statistical, human body models. Such an approach usually requires the indication of body joints, which is mostly carried out manually, but automatic and semi-automatic methods
[28] also exist. Further, deep neural networks have been recently used to compute statistical models’ parameters
[29]. These types of modelling techniques have become an integral component in the recent methods for 3D human body reconstruction and animation. Here, the researchers present two of the most popular statistical body models and one that is a promising improvement of the second presented model.
3. Human Body Datasets
Human bodies are flexible, moving in various ways and deforming their clothing and muscles. Another complicating issue, like the occlusion of different body parts during movement, may necessitate comprehensive scene modelling in addition to the peoples in the scenario. Such image understanding scenarios push, for example, the avatar body animation system’s ability to use prior knowledge and structural correlations by constraining estimates of unseen body components using limited visible information. Insufficient data coverage is one of the most significant issues for trainable systems. So, many researchers have concentrated their efforts on creating publicly available datasets that can be used to build operational systems for realistic scenarios.
One of the largest motion capture datasets is the Human 3.6 M dataset
[30]. It consists of 3.6 million fully visible human poses and corresponding images. All of them are captured by a high-speed motion capture system. The recording setup consists of 15 sensors (4 calibrated high-resolution progressive scan cameras that acquire video data at 50 Hz, 1 time-of-flight sensor, and 10 motion cameras), using hardware and software synchronization. This allows for accurate capture and synchronization. The dataset contains activities performed by 11 professional actors (6 male, 5 female) in 17 scenarios—taking photos, discussing, smoking, talking on the phone, etc. Also, accurate 3D joint positions and joint angles from high-speed motion capture systems are provided. Other useful additions are 3D laser scans of the actors, high-resolution videos, and accurate background subtraction.
The MPI-INF-3DHP
[31] is a 3D human body pose estimation dataset. It consists of both constrained indoor and complex outdoor scenes. The dataset comprises eight actors (4 male, 4 female) enacting eight distinct activity sets, each lasting about a minute. With a diverse camera setup, 14 cameras in total, over 1.3 M frames have been obtained, with 500 k originating from cameras at chest height. The dataset provides genuine 3D annotations and a skeleton compatible with the “universal” skeleton of Human 3.6 M. To bridge the gap between studio and real-world conditions, chroma-key masks are available, facilitating extensive scene augmentation. The test set, enriched with various motions, camera viewpoints, clothing varieties, and outdoor settings, aims to challenge and benchmark pose estimation algorithms.
The Synthetic Humans for Real Tasks (SURREAL) dataset
[32] contains 6.5 M frames of synthetic humans, organized into 67,582 continuous sequences. The SMPL
[33] body model is employed to generate these synthetic bodies, with body deformations distinguished by pose and intrinsic shape. Created in 2017, it is the first large-scale person dataset to generate depth, body parts, optical flow, 2D/3D pose, surface, normal, and ground truth for Reed Green Blue (RGB) video input. The provided images are photorealistic renderings of people in different shapes, textures, viewpoints, and poses.
Dynamic Fine Alignment Using Scan Texture (DFAUST)
[34] is considered a 4D dataset. It consists of high-resolution 3D scans of moving non-rigid objects, captured at 60 fps. A new mesh registration method is proposed. It uses both 3D geometry and texture information to register all scans in a sequence according to a common reference topology. The method makes use of texture constancy across short and long time intervals, as well as dealing with temporal offsets in shape and texture.
Microsoft Common Objects in Context (MS COCO)
[35] is a large-scale object detection, segmentation, and captioning dataset. It consists of many other objects, but also humans and human photos. The dataset offers recognition in context, superpixel stuff segmentation, and 250,000 people with key points.
Leeds Sports Pose (LSP)
[36] and its extended version—LSPe
[37]— are human body joint detection datasets. The LSPe dataset contains 10,000 images gathered from Flickr searches for the tags ‘parkour’, ‘gymnastics’, and ‘athletics’ and uses poses that are challenging to estimate. Each image has a corresponding annotation that might not be highly accurate because it is gathered from Amazon Mechanical Turk. Each image is annotated with up to 14 visible joint locations.
The Bodies Under Flowing Fashion (BUFF) dataset, as delineated by the authors of
[38], offers over 11,000 3D human body models engaged in complex movements. It is distinctive in its inclusion of videos featuring individuals in clothing paired with 3D models devoid of clothing textures. This dataset emerges from a multi-camera active stereo system, utilizing 22 pairs of stereo cameras, color cameras, speckle projectors, and white-light LED panels operating at varied frame rates. This system outputs 3D meshes averaging around 150 K vertices, capturing subjects in two distinct clothing styles. Of the initial six subjects, the data from one were withheld, resulting in a public release of 11,054 scans. To derive a semblance of “ground truth”, the subjects were captured in minimal attire, with the dataset’s accuracy showcased by the proximity of more than half of the scan points to the mean of the estimates. The BUFF dataset efficiently captures detailed aspects of human movement while also considering the impact of different clothing on a body’s shape and motion.
The HumanEva datasets
[39], comprising HumanEva-I and HumanEva-II, offer a blend of video recordings and motion capture data from subjects performing predefined actions. HumanEva-I encompasses data from four subjects executing six distinct actions, each with synchronized video and motion capture, and one with only motion capture. This dataset leverages seven synchronized cameras, utilizing multi-view video data coupled with pose annotations. On the other hand, HumanEva-II focuses on two subjects, both of whom are also present in HumanEva-I, performing an extended “Combo” sequence, resulting in roughly 2500 synchronized frames. The data, collected under controlled indoor conditions, capture the intricacies of natural movement, albeit with challenges posed by illumination and grayscale imagery.
The UCLA Human–Human–Object Interaction (HHOI) dataset
[40] is a novel RGB-D (Reed Green Blue—Depth) video collection detailing both human–human and human–object–human interactions, captured using a Microsoft Kinect v2 sensor. Comprising three human–human interactions—-hand shakes, high-fives, and pull ups-—and two human–object–human interactions—-throw and catch and hand over a cup—the dataset features an average of 23.6 instances for each interaction. These instances are performed by eight actors, recorded from multiple angles, and spanning 2–7 s at a frame rate of 10–15 fps. While objects within the dataset are discerned using background subtraction on both RGB and depth images, the Microsoft Kinect v2’s skeleton estimation is also utilized. The dataset, divided into four distinct folds for training and testing, ensures no overlap of actor combinations between the sets. The training algorithm demonstrates robust convergence within 100 iterations, operating on a standard 8-core 3.6 GHz and yielding an average synthesis speed of 5 fps using an unoptimized Matlab code.
To address prevalent challenges in viewpoint invariant pose estimation, a novel technical solution has been presented in
[41]. It integrates local pose details into a learned, viewpoint-invariant feature space. This approach enhances the iterative error feedback model to incorporate higher-order temporal dependencies and adeptly manage occlusions via a multi-task learning methodology. Complementing this endeavor is the introduction of the Invariant Top View (ITOP) dataset, a comprehensive collection of 100 K depth images capturing 20 individuals across 15 diverse actions, encompassing a wide range of views, from front, top, to side, inclusive of occluded body segments. Each image in the ITOP dataset is meticulously labeled with precise 3D joint coordinates relative to the camera’s perspective. With its unique blend of front/side and top views—-the latter captured from ceiling-mounted cameras—-the ITOP dataset stands as a significant resource for benchmarking and furthering advancements in viewpoint-independent pose estimation.
The 3D Human Body Model dataset established by the authors of
[42] is a synthetic dataset that consists of 20,000 three-dimensional models of human bodies in static poses and an equal gender distribution. It is generated with the STAR parametric model
[43]. While generating the models, two primary considerations were maintained: the natural Range of Motion (ROM) for each joint and the prevention of self-intersections in the 3D mesh. Existing research on the human ROM was referenced to define the limitations of joint rotations. Despite adhering to ROM constraints, certain non-idealities sometimes result in self-intersections in areas like the pelvic region, knees, and elbows. To address this, each vertex of the mesh is associated with a specific bone group. Self-intersections between non-adjacent bone groups are considered forbidden, and an algorithm flags such meshes as invalid.
The 3D Poses in the Wild Dataset (3DPW)
[44] offers a unique perspective by capturing scenarios in challenging outdoor environments. This extensive dataset encompasses more than 51,000 frames featuring seven different actors donning 18 distinct clothing styles. The data collection process involves the use of a handheld smartphone camera to record the actions of one or two actors. Notably, 3DPW enhances its utility by providing highly accurate mesh ground truth annotations. These annotations are generated by fitting the SMPL model to the raw ground truth markers.
The Max Planck Institute for Informatics (MPII) dataset serves to evaluate the accuracy of articulated human pose estimation. This dataset comprises approximately 25,000 images, featuring annotations for over 40,000 individuals, including their body joints. These images were systematically compiled, capturing a wide array of everyday human activities. In total, the dataset encompasses 410 different human activities, with each image labeled according to the specific activity depicted. The images are extracted from YouTube videos.
4. Evaluation Metrics
Applying evaluation metrics is crucial to quantitatively assessing the reconstruction quality of generated models in the field of 3D human body reconstruction. Here, the researchers briefly introduce some of the most commonly utilized metrics for this purpose. These metrics provide a standardized and objective means of evaluating the accuracy and fidelity of reconstructed 3D human body models, ensuring a reliable assessment of the reconstruction process.
The MPJPE
[45] is a common metric that evaluates the performance of human pose estimation algorithms. It measures the mean distance in mm between the skeleton joints of the ground truth 3D pose and the joints from the estimated pose. The formulation is provided in the following Equation:

where
𝑁𝑆 corresponds to the total number of skeleton joints,
𝑚(𝑓)𝑓,𝑆(𝑖) is a function that returns the coordinates of the
i-th joint of skeleton
S in frame
f, and
𝑚(𝑓)𝑔𝑡,𝑆(𝑖) is a function that refers to the i-th joint of the skeleton in the ground truth frame. A commonly used modification of the MPJPE is the Procrustes-aligned MPJPE (PA-MPJPE), which is calculated in a similar way with the difference that the reconstructed model and the ground truth one are previously aligned using the Procrustes algorithm.
The MAVE
[46] is used to find the averaged distance between the vertices of the reconstructed 3D human model and the vertices of the ground truth data. It is defined by
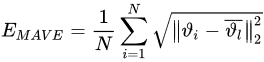
where
N is the total number of vertices of the 3D model,
𝜗𝑖 is a vertex from the predicted 3D human body model, and
𝜗𝑖¯ is a vertex from the corresponding ground truth data.
The symmetric point-to-point Chamfer distance measures the similarity between two point clouds P and Q. A common formulation is given in the following Equation:
The VSD metric quantifies the average distance between the vertices of a point cloud and their corresponding points on the surface of a triangular mesh. These surface points can either be the vertices of the mesh or points that reside on its faces or edges. The authors of
[47] incorporate this metric into their 3D model-fitting algorithm, employing a lifted optimization technique. Points on the surface are defined via surface coordinates, represented as
𝑢={𝑝,𝑣,𝑤}. Here,
𝑝∈ℕ denotes the triangle’s index where the point is situated, and
𝑣∈[0,1],
𝑤∈[0,1−𝑣] are the coordinates within the unit triangle. Therefore, the 3D coordinates of a point on the surface can be defined as shown in the following Equation, where
𝐯1,𝐯2 and
𝐯2 are the vertices of the
p-th triangle.

The distance between a point from a point cloud and its correspondence on the surface of the mesh can be further calculated as described in the following Equation, where D is the number of points 𝑥𝑖 in the point cloud and 𝑈={𝑢𝑖}𝐷𝑖=1 are the surface coordinates of those points.
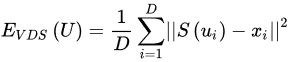
Within the confines of the algorithm described in
[47], the mesh under consideration is parametric. This implies that its vertex coordinates are contingent on the parameter vector,
𝜃. Given that the algorithm adopts lifted optimizations, both
𝜃
and the surface coordinates, U, are optimized concurrently.
5. Taxonomy of Existing 3D Human Body Modelling and Reconstruction Techniques
Figure 1 presents a taxonomy of existing 3D human body modeling and reconstruction techniques based on the input data. In essence, 3D human body modeling is a task in computer vision and computer graphics aimed at generating a 3D photorealistic representation of the human body. This task often involves, but is not limited to, processes such as data acquisition, 2D pose estimation through the detection of 2D body joints, camera calibration and triangulation in the case of multiple views, 3D pose estimation to derive the pose of the future 3D model, model shape optimization when using parametric body models, surface reconstruction and texturing for obtaining a detailed 3D representation of the model’s surface, and post-processing and refinement to increase the quality of the reconstructed 3D model. However, it is challenging to compose a concrete framework of operations that applies universally across different input data types and reconstruction approaches. Nevertheless, several key steps, which are visualized in Figure 2, are commonly accomplished in most of the examined methods, including 3D pose estimation, coarse 3D shape estimation, 3D shape refinement, and texture recovery. The blocks surrounded with dash lines indicate that the utilization of the parametric body model and the appliance of 3D pose estimation is sometimes omitted by some of the examined algorithms, most of which are deep learning based. The figure also illustrates the different types of input data.
Figure 1. Taxonomy of 3D human body modelling and reconstruction techniques based on input data.
Figure 2. A 3D human body model reconstruction. (Parametric body model
[33]).