1. DNA Methylation in Sputum and Plasma for Early LC Detection
As epigenetic changes in LC are common, this offers several targets that can concurrently be probed
[1]. LC genome analysis reports global hypomethylation that results in the destabilisation of DNA with the exception of CpG dense regions
[2][3][4]. In NSCLC, epigenetic changes are associated with cigarette smoking and aggressive tumour behaviour, and as such these changes can be used for risk stratification and histological and molecular characterisation
[5][6][7][8][9][10][11][12]. Non-invasive, sputum-based epigenetic testing for the detection of epigenetic changes/promoter DNA hypermethylation at early stages of tumorigenesis is well documented. Palmisano et al. showed that in sputum samples, collected 3 years prior to clinically detectable lung cancer, the hypermethylation of
MGMT and/or
CDKN2A genes could be effectively detected, indicating that epigenetic markers can indeed play a role in early cancer diagnosis
[13]. This was validated in other studies as well
[14][15][16]. Moreover, in a study of five participants,
RASSF1A methylation, detected in sputum samples, correlated with the development of LCs within 12 to 14 months from the sputum test in three patients
[17]. Similarly, a prospective study on 92 high risk individuals and a matched control group identified promoter methylation of 14 genes in the sputum that can be used for risk stratification. It was found that 6 of 14 genes correlated with a >50% increased LC risk. Furthermore, simultaneous methylation of three or more of these six genes correlated with 6.5-fold increased risk of LC
[18]. These detected genes are involved in many important biological functions, such as cell cycle regulation (
p16 and
PAX5 β), apoptosis (
DAPK and
RASSF1A), signal transduction (
GATA5), and DNA repair (
MGMT)
[18][19][20][21][22].
The detection of DNA methylation in plasma, as a tool for screening and diagnostic purposes in LC, has also shown promise. Bearzatto et al. reported an increased frequency in
p16INK4A methylation in plasma samples of early-stage adenocarcinoma
[23]. Similarly, methylations of
RASSF1A and
CDKN2A detected in blood samples were frequently identified in early-stage LC with a reported sensitivity of 22 to 66% and specificity of 57–100%
[23][24][25]. Another study on 70 participants showed significant differences in the methylation pattern between LC and benign lung lesions. The participants who developed lung cancer showed methylation changes in four tumour suppressor genes, i.e., Kif1a, DCC, RARB, and NISCH. The differences were correlated with LC diagnosis, and it was observed that participants who were finally diagnosed with LC exhibited significant differences in methylation pattern
[26]. Another, larger study on 360 participants showed similar results. The methylation status of
PTGER4 and
SHOX2 genes detected in the plasma of patients with indeterminate pulmonary nodules was distinct as compared to participants with benign lung nodules
[27]. Therefore, integrating DNA methylation expression patterns (in plasma/sputum) as a screening tool in national LC screening programs is now needed to progress to novel algorithms for early LC detection. In lieu of this, Kang et al. developed a probabilistic method called Cancer Locator, based on cfDNA detected in blood samples. The study utilized data from a genome-wide DNA methylation profile and DNA methylation microarrays of solid tumour samples to train the model. The model was able to identify the histological type and the site of the tumour together with cancer load in NSCLC
[28]. The study could not offer firm conclusions because of small sample numbers; however, the authors foresaw that when more paired samples (tumour sample and the matched adjacent non-tumour sample) become available, Cancer Locator could identify not just the existence but also the location of the tumour
[28].
2. The Role of microRNAs in LC Detection
miRNAs are small non-coding RNAs of 18–25 nucleotides in length which are involved in the post-transcriptional regulation of gene expression
[29][30]. They are found to be aberrantly expressed in many pathological conditions, including cancer, and can be detected in bodily fluids including urine, sputum, and blood, making them exciting biomarkers for cancer detection
[31][32]. In 2002, their role in LC pathogenesis (proliferation of LC cells, invasion of basement membrane, and metastasis) was reported by Calin et al.
[33]. Interestingly, based on the cellular context, miRNAs can act as tumour suppressors or oncogenes and even both
[34][35]. Moreover, miRNAs preserve their stability throughout cancer progression from initiation to metastasis, because they are too small to degrade, and some miRNAs are further protected in exosomes. Hence, miRNAs are considered an appealing biomarker for cancer diagnosis and monitoring
[36].
Another non-coding RNA type, circRNAs, which have a stable covalently closed circular structure and show a specific expression pattern in different tissues and cells, have also been implicated in LC growth and progression
[37]. However, the exact mechanisms remain poorly understood and require more in-depth studies
[38]. Using technologies such as RNA-seq and Ribo-Zero, thousands of circRNAs have been discovered (
[37], and it is predicted that valid circRNA biomarkers for diagnosis, prognosis, and therapy in LC will increasingly be found. A better understanding of the exact role of circRNAs in the pathogenesis of LC will likely also lead to improvement of the detection of “clinically significant” circRNAs and understanding of the temporal relationship between such circRNAs and the development of preinvasive or early LC.
3. The Role of Circulating Tumour DNA (ctDNA) in LC
ctDNA (circulating tumour DNA) includes both encapsulated (in circulating vesicles) and non-encapsulated free DNA in the blood or other body fluids
[39]. ctDNA escapes cancer cells via several mechanisms, namely apoptosis, necrosis, and secretion from extracellular vesicles as well as from CTCs
[40][41]. Therefore, analysing ctDNA is a promising approach that could accelerate efforts for body fluid-based LC detection and overcome some of the challenges posed by invasive tissue biopsy, as summarised in
Table 1.
Table 1. Important differences between LB (analysis of ctDNA) and tissue biopsy.
An important feature of ctDNA is that it can be found in blood prior to clinical diagnosis
[51]. Advances in technologies of DNA sequencing made it possible to detect cDNA before clinically evident LC
[52]. However, a major challenge in using ctDNA is that most patients have ctDNA levels of less than 0.1%
[53][54]. Nonetheless, new techniques have continuously been developed and tested to improve the detection of ctDNA in low concentrations in plasma. There is also evidence of a positive correlation between disease burden and the plasma concentration of ctDNA
[52]. A study by Jacob et al.
[51] used deep sequencing (CAPP-Seq) and improved protocol for the extraction of unique cfDNA fragments and the segment of cfDNA duplexes for sequencing of both strands
[51]. The authors genotyped tumour tissue, analysed pre-treatment cfDNA in plasma and leukocyte DNA from 85 subjects diagnosed with stage I–III NSCLC using targeted deep sequencing of 255 frequently mutated genes in NSCLC, and reported that most somatic mutations in the cfDNA of LC patients and of risk-matched cohorts replicate clonal haematopoiesis and are not recurring. In contrast with mutation driving carcinogens, clonal haematopoiesis mutations are present on longer cfDNA fragments and do not show mutational marks that correlate with tobacco smoking. Incorporating these results with other tumour characteristics such as cell proliferation and lymphovascular invasion, the authors applied and prospectively validated a machine-learning-based method called “LC likelihood in plasma” (Lung-CLiP)
[53]. Three control groups were used as a validation cohort: a low-risk group of 42 adult blood donors, a matched risk control group of 56 age, sex, and smoking status matched adults who had negative low-dose CT (LDCT) screening scans, and a third group comprising 48 risk-matched participants receiving LDCT screening recruited prospectively at a different centre.
One of the key shortcomings of molecular analysis by studying ctDNA is that it provides no information on histology; therefore, invasive biopsy will be required to make a histological diagnosis of LC. False-negative results from analysing ctDNA is a further important issue in the context of low tumour load or low rate of shedding of ctDNA to the systemic circulation
[55]. Moreover, the precision of the data acquired by analysing ctDNA is affected by the location of the metastatic disease. A pooled analysis of EGFR-mutated NSCLC revealed that the detection rate of ctDNA EGFR mutation was considerably higher in patients with extrathoracic compared to intrathoracic lesions
[50]. Furthermore, the false-positive results can be acquired using ctDNA as mentioned above (molecular alterations originated by clonal haematopoiesis rather than the tumour)
[56]. Identification of unintended germline mutations during ctDNA evaluation that are not linked to the pathogenesis of LC is not an infrequent occurrence that mandates disclosure to the patient and referral for genetic counselling clinics
[57]. For example, in the molecular analysis using ctDNA of 10,888 unselected patients with metastatic cancer (41% were lung malignancies), 1.4% were discovered to have possible hereditary cancer mutations in 11 genes
[57]. Finally, technical aspects in relation to ctDNA specimen acquisition and handling can affect the quality of the data. Despite the many advantages of LBs compared to tissue biopsies, the SN and SP of detecting specific molecular changes in NSCLC from LB remain affected by technology, clinical trial methodologies, and logistics, which in turn affect the safe and effective integration of LB into clinical practice
[58]. In a first published systematic review of 34 studies involving 1141 patients with NSCLC by Esagian et al., the positive percent agreement (PPA) in detecting common mutations using targeted NGS between LB and tissue biopsy was provided
[59]. The authors stated that they used PPA rather than SN, SP, and PPV and NPV because NGS was not validated in all the studies they reviewed, and hence PPA was deemed more appropriate. The calculated PPA rates were 53.6% (45/84) for ALK, 53.9% (14/26) for BRAF, 56.5% (13/23) for ERBB2, 67.8% (428/631) for EGFR, 64.2% (122/190) for KRAS, 58.6% (17/29) for MET, 54.6% (12/22) for RET, and 53.3% (8/15) for ROS1. The above findings are consistent with other publications that concluded that the detection of specific mutations via NGS from LB is less sensitive compared to tissue biopsy
[60][61].
4. Urine Cell-Free DNA (ucfDNA) in the Diagnosis of LC
Improvements in the knowledge and the technologies for the isolation and analysis of biomarkers from urine provide novel opportunities for the clinical applications of cancer urine biomarkers. The presence of biomarkers such as exfoliated bladder cancer cells, ctDNA, proteins, miRNAs, and exosomes in the urine have been investigated in the context of different primary cancers such as bladder, prostate, pancreas, and lung; the cost-effectiveness and convenience of use make urine biomarkers attractive choices for patients and physicians alike
[62][63][64][65]. Using urine biomarkers for assessing treatment efficacy and resistance is a major advantage when compared to tissue biopsies and radiological imaging
[66]. Furthermore, another advantage of urine biomarker analysis is that cfDNA extraction is technologically easier
[66][67][68], when compared with plasma, as urine contains a lower concentration of interfering proteins
[69]. The evidence for the reliability and sensitivity of the detection of gene mutations and DNA methylation in the urine is growing, especially as the technologies used are consistently undergoing refinement
[70][71][72].
Methods associated with the extraction and classification of urinary constituents are multifarious and diverse and can vary from methods for protein and genomic profiling to microfluidic techniques
[73]. In recent years, the detection of EGFR mutation and the subsequent mutation profile in patients with metastatic NSCLC who might be eligible to receive first and second lines of anti-EGFR tyrosine kinase inhibitors (TKIs) has grown rapidly. A study by Reckamp et al. showed that EGFR mutations (T790M, L858R, and exon 19 deletions) were successfully identified in the urine of NSCLC patients and the results were congruent with the EGFR mutation state identified through tissue biopsy
[74]. A comparative study was reported by Ren et al., who measured the concentration of ucfDNA, using qPCR, in 55 LC patients and a cohort of 35 healthy participants
[75]. The study reported that the concentration of ucfDNA is consistently higher in LC patients, especially with lymph node involvement, compared to the healthy cohort, suggesting that ucfDNA could potentially play a role in the early diagnosis of LC
[75]. Another study compared the urine cell-free DNA (ucfDNA) of 55 NSCLC patients of different disease stages with 35 healthy volunteers by means of quantitative real-time PCR (qPCR)
[76]. The study showed that concentrations of urinary cell-free DNA (ucfDNA) were considerably greater in individuals with stage III/IV than in those with stage I/II and the disease-free cohort. The receiver operating characteristic curves (ROCs) for distinguishing participants with stage III/IV from disease-free volunteers showed areas under the curve (AUCs) of 0.84 and 0.88, respectively. In another study
[75], ucfDNA concentration and integrity indexes were explored as biomarkers for early LC detection. The cohort included 55 LC patients and 35 healthy participants. The study found that concentration and integrity indexes of ucfDNA were considerably higher in LC patients compared to the healthy individuals. Moreover, the ucfDNA integrity indexes in patients with metastasis to lymph nodes were significantly higher compared with patients without lymph node involvement, suggesting that ucfDNA could potentially play a role in the early diagnosis of LC
[75].
5. RNA Airway and Nasal Signature
The approach of analysis of RNA acquired from airway samples centres on gene expression profiles of cancer-associated processes affecting the tracheobronchial tree
[77]. A study identified a 23-gene biomarker panel from endobronchial brushings of patient who received bronchoscopy to investigate LC
[78]. Consequently, two separate prospective cohorts showed an SN of 88% to 89% and an SP of 48% for such a gene-expression classifier. As biomarkers, these 23 genes were especially indicative of possible underlying cancer in patients with an intermediate (10–60%) pre-test risk of LC (91% negative predictive value, NPV). These results suggest that the NPV of a negative bronchoscopy could be improved if combined with the 23-gene panel, which could potentially circumvent the need for invasive lung biopsy by monitoring such patients with less invasive tests such as follow up CT scans
[79].
6. Radiomics Signatures of Primary and Secondary Pulmonary Malignant Lesions
In the past decade, medical imaging has progressed from chiefly being a primary diagnostic tool to acquiring an important role in providing vital molecular data required for targeted based therapy through the adoption of advanced hardware, novel imaging agents, streamlined scanning protocols, and improvements in computational power
[80]; thus, the researchers will briefly discuss its role here. The technological advances have enabled the extraction and processing of a large amount of data from quantitative imaging, in a process called radiomics
[80]. By utilising a characterisation algorithm, radiomics has the potential to unveil disease features that cannot be seen by the naked eye
[81]. The process of radiomics involves obtaining sub-visual, yet quantitative, image characteristics in order to produce usable datasets from radiological films
[82]. Radiomics data extracted from medical scans (e.g., CT and MRI scans) can be utilised to discover diagnostic, predictive, and prognostic data in patients with malignancy through comparison with objective response criteria such as overall and progression-free survival, and can also be combined with tumour molecular and genetic profile (genotype); the latter is referred to as radiogenomics
[83]. The process of converting medical imaging into meaningful data typically involves four steps: (a) image acquisition and reconstruction, (b) region of interest segmentation, (c) feature extraction and quantification, and (d) building predictive and prognostic models, as illustrated in
Figure 1.
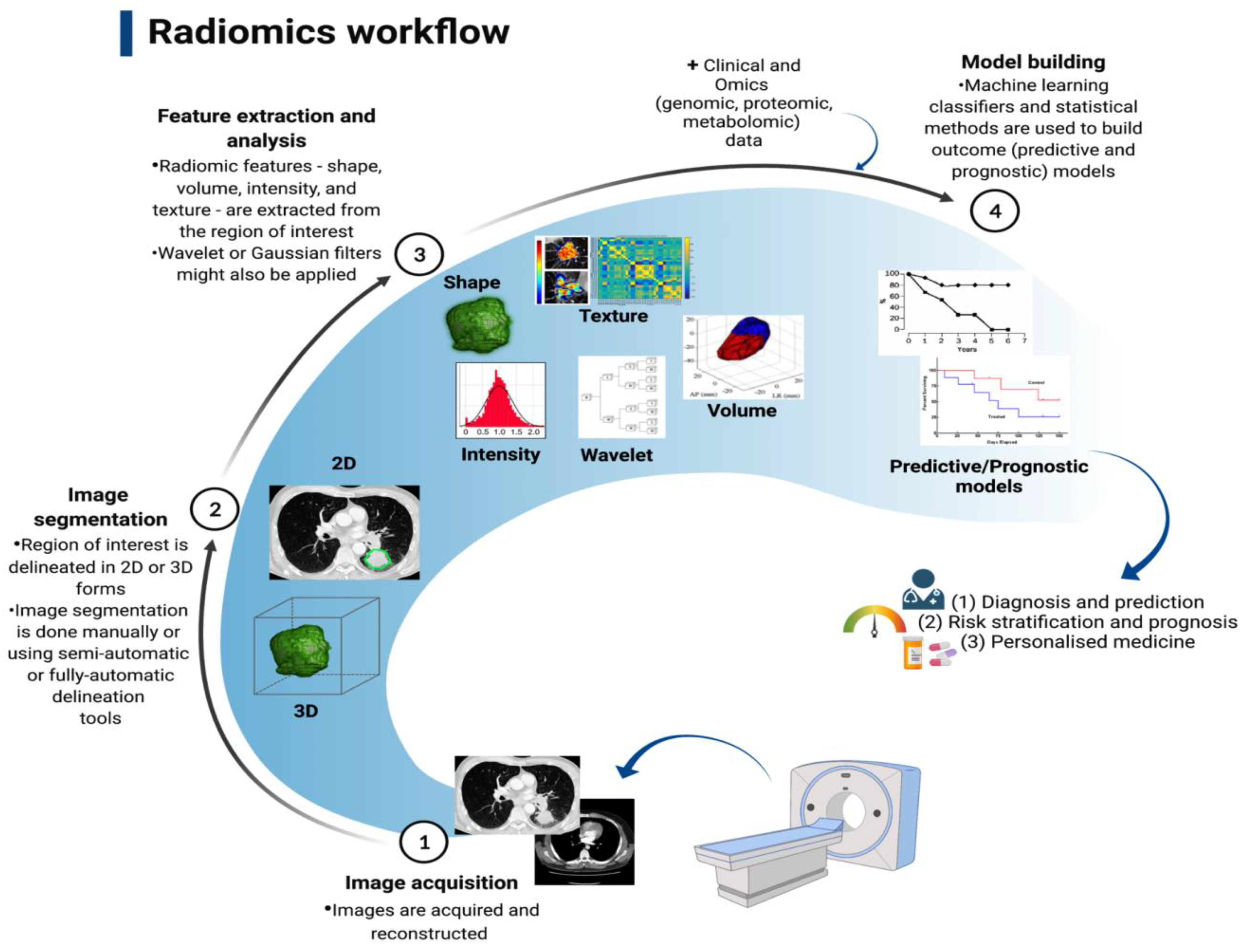
Figure 1. Radiomics workflow that involved four stages, Lambin et al.
[81].
As a new technology, radiomics is in its infancy; therefore, its clinical application is still limited. In the context of primary LC, a significant interest in using radiomics to predict the histological and molecular characteristics, response to treatment, and overall prognosis is raised. Several studies have been able to identify specific radiomics signatures that differentiate NSCLC from other benign and pre-invasive lesions, including the prediction of EGFR status and response to treatment with TKI
[84][85][86][87][88][89][90][91], as well as histological subtype. For example, a retrospective study of 148 patients with histologically confirmed NSCLC found thirteen radiomics features that predict histological subtype (ALC vs. SqCLC) with AUCs of 0.819 and 0.824, respectively
[92]. Several studies of radiomics signatures have reported features distinguishing benign from cancerous lung pathologies and are shown in
Table 2.
Table 2. Summary table showing studies of radiomics signatures to distinguish benign from cancerous lung pathologies.
To conclude, radiomics offers a tangible opportunity for even wider use of medical imaging in oncology, especially in difficult to access lesions or lesions in patients in whom invasive lung biopsy could be detrimental.
This entry is adapted from the peer-reviewed paper 10.3390/cancers14235782