As sessile organisms, plants are at the mercy of an array of abiotic stresses, and, as winter approaches in mid- to high-latitudes and altitudes, one such stress is low temperature. Plants employ various strategies that allow them to recognise and cope with the cold. As autumn progresses, perennials undergo a period of cold acclimation, which in a few days of low temperature exposure allows them to physiologically prepare for freezing conditions. Such preparations include changed levels of hundreds of proteins, the accumulation of fatty acids, lipid remodelling for plasma membrane protection, increased production of cryoprotective metabolites, such as soluble sugars and amino acids, as well as chaperones and reactive oxygen scavengers. The perennial grass and model cereal, Brachypodium distachyon (hereinafter, Brachypodium), is capable of cold acclimation, reaching peak freezing tolerance after two days at 4 °C, and is associated with changes in the abundance of multiple plasma membrane proteins at 2–6 days
1. Introduction
As sessile organisms, plants are at the mercy of an array of abiotic stresses, and, as winter approaches in mid- to high-latitudes and altitudes, one such stress is low temperature. Plants employ various strategies that allow them to recognise and cope with the cold
[1]. As autumn progresses, perennials undergo a period of cold acclimation, which in a few days of low temperature exposure allows them to physiologically prepare for freezing conditions. Such preparations include changed levels of hundreds of proteins, the accumulation of fatty acids, lipid remodelling for plasma membrane protection, increased production of cryoprotective metabolites, such as soluble sugars and amino acids, as well as chaperones and reactive oxygen scavengers
[2]. This acclimation process also appears to coincide with changes in host-associated microbial communities. Such a turnover in microbiota could assist plants in preparing for sub-zero temperature conditions and their vulnerability to psychrophilic pathogens. Indeed, winter seasonality in the plant microbiome has been previously reported
[3][4][5]. Although the impact of cold acclimation on the microbiomes of perennial grass has not been hitherto explored, the identification of their bacterial and fungal communities offers the promise of understanding how the battle against coming winter conditions can be won by partnerships.
The perennial grass and model cereal,
Brachypodium distachyon (hereinafter,
Brachypodium), is capable of cold acclimation, reaching peak freezing tolerance after two days at 4 °C, and is associated with changes in the abundance of multiple plasma membrane proteins at 2–6 days
[6]. In turn, these proteins are involved in complex crosstalk networks that prime the
Brachypodium defensive response to a variety of abiotic and pathogenic stresses. Studies of cold acclimation have, for the most part, ignored the host-associated microbiota
[1][7][8]. Nevertheless, the plant microbiome is emerging as an important factor in stress responses, including symbiont-mediated tolerance
[9][10].
The general beneficial effects of microbes on plant fitness under a variety of stressful conditions have recently come to be known as the “Defence Biome”
[5][10][11][12][13][14][15][16][17]. Symbiont-mediated fitness benefits may be a collective result of microbial exudates and function, for example, by facilitating early stress sensing and more efficient nutrient uptake and transfer, as well as by the induction of plant stress genes
[9][10]. Specifically, symbiont-mediated cold tolerance has been directly demonstrated with some plant species and plant growth promoting bacteria (PGPBs)
[9]. For example,
Burkholderia phytofirmans-inoculated grape vines expressed cold stress-responsive genes earlier than non-inoculated vines
[18] and
Streptomyces neyagawaensis J6-inoculated turfgrass showed enhanced cold tolerance over non-inoculated plants
[19]. Microbes thus have a demonstrated role in plant protection. They excrete a variety of products to benefit host plants, including anti-pathogenic microbial compounds and osmolytes, including proline and trehalose, as well as scavengers of reactive oxygen species, such as superoxide dismutase, catalase, and peroxidases
[9][10][20]. Taken together, plant-associated microbial communities undoubtedly help plants survive cold stress.
The identification of host-associated microbiota that enhance freezing tolerance may lead the way to the development of synthetic cocktails of species that could eventually be used to inoculate crops or seeds to enhance cold tolerance
[21]. Here, shotgun sequencing and metagenomic analysis of the phyllosphere/endosphere and rhizosphere in cold-acclimated
Brachypodium is an important first step towards this goal. Researchers' experimental inoculation of a commercial growing mix with old pasture soil allowed for the exposure and subsequent identification of bacterial and fungal taxa that thrived after transfer of the growing plants to low temperatures and thus are prospective native partners in the cold acclimation process. In addition, researchers contribute to the general appreciation of the robustness of the plant abiotic stress response, which employs communities of diverse organisms for survival.
2. Pre-Processing, Shotgun Sequencing, and Kit Controls
Initial DNA samples representing the cold-acclimated (CA) leaf and rhizosphere were sent for shotgun sequencing without eukaryotic depletion, revealing high host contamination in the leaves (not shown). Subsequent replicate samples undergoing eukaryotic depletion proved successful as the classification of processed reads showed a full order of magnitude better recovery of microbial sequences. DNA and library concentrations and average size, quality control, host read decontamination, and Kraken2 classification results are summarized in the
Supplementary Materials (Figure S4, Tables S1 and S2), supplementary could be found in
https://www.mdpi.com/2223-7747/10/12/2824#supplementary). Although shotgun DNA library construction was attempted on the blank kit controls, a lack of sufficient DNA resulted in no results for this sequencing method. However, the same control samples were subject to amplicon marker gene sequencing. Following QIIME2 processing, it was determined through diversity analysis and PCoA using Bray–Curtis dissimilarities that the microbial compositions associated with the kits were significantly different than the
Brachypodium leaf (
p < 0.001 16S,
p < 0.05 ITS, pairwise PERMANOVA) and rhizosphere microbiomes (
p < 0.001 16S,
p < 0.05 ITS, pairwise PERMANOVA) (
Figure S5).
3. Compatible Results with Shotgun and Amplicon Sequencing
The correlation between taxa identified in both the shotgun data and the amplicon data was assessed at the genus level in order to compare the two methods. In the CA rhizosphere, the genera identified by shotgun metagenomic and 16S rRNA amplicon sequences, as well as shotgun metagenomics and ITS amplicon sequencing, were well correlated (R
2 = 0.93 and R
2 = 0.88, respectively) (
Figure S6). The non-acclimated (NA) rhizosphere shotgun and 16S rRNA, and the shotgun and ITS amplicon results (R
2 = 0.91 and R
2 = 0.45, respectively) also correlated, but less well. It is notable that for the leaf microbiome, bacterial taxa in the CA shotgun and 16S rRNA samples, as well as for the NA leaf samples, showed mixed correlations (R
2 = 0.31 and R
2 = 0.75, respectively). Insufficient fungal reads in the leaves following Bracken re-estimation resulted in no correlation between the shotgun and ITS reads in the leaves.
4. Cold Acclimation and the Rhizosphere Microbiome
In total, 4646 microbial species were identified in the rhizosphere shotgun data with 45 ± 3% of reads remaining unclassified. The majority of identified reads, 99.70 ± 0.06%, represented bacterial microbes with 0.15 ± 0.03% and 0.13 ± 0.02% representing fungi and archaea, respectively. Alpha diversity, assessed using Shannon’s diversity index, across all rhizosphere samples was 4.98 ± 0.21 and was not significantly different between conditions with 5.07 ± 0.29 in the CA and 4.91 ± 0.94 in the NA samples. The rhizosphere was dominated by Streptomyces sp. M2, a PGPB, accounting for approximately one-third of the taxa in all samples. Rounding out the top abundant species across the rhizosphere samples were taxa present at 1–10% abundance, which included Actinocatenispora sera, Actinocatenispora thailandica, Rhodanobacter denitrificans, and Rhodanobacter sp. FDA-ARGOS 1247 (Figure 1A; Table S3). Nearly half of all species in the rhizosphere shotgun data were below a cut-off value (0.2%) for low relative abundance leaving a balance of 53% and 56% of species found in NA and CA samples, respectively.
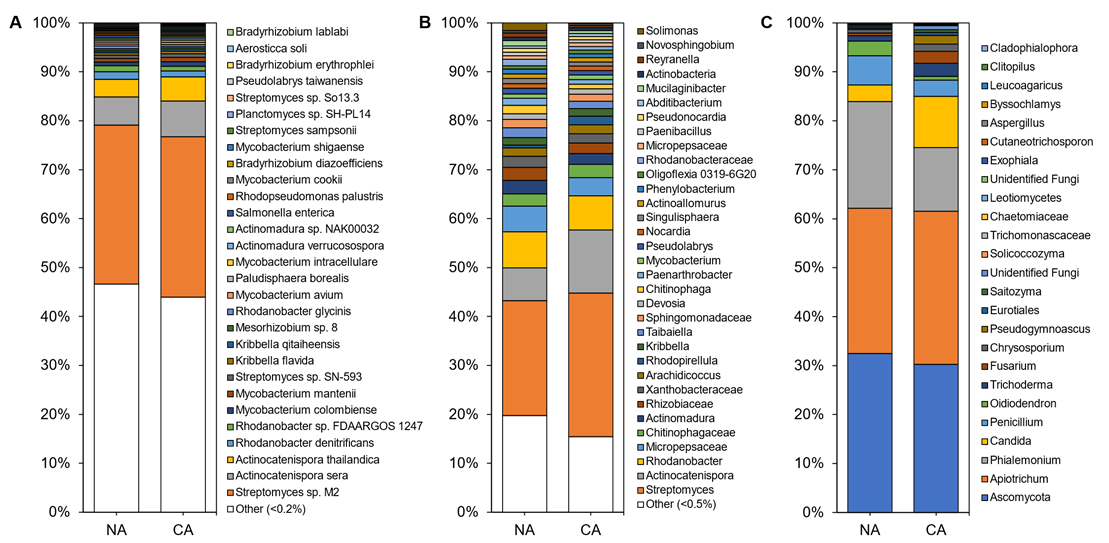
Figure 1. Average relative abundance of the taxonomies of the non-acclimated and cold-acclimated Brachypodium distachyon rhizosphere microbiomes: (A) species identified from shotgun sequencing and metagenomics classified using a custom Kraken2 database, (B) distinct amplicon sequence variants assigned down to the genus or lowest possible level by QIIME2 using the SILVA database for 16S rRNA sequences amplified using the V4 region of prokaryotes, and (C) distinct amplicon sequence variants assigned down to the genus or lowest possible level by QIIME2 using the UNITE database for ITS regions of eukaryotes.
The amplicon analysis identified 651 distinct ASVs at the genus level. Alpha diversity appeared similar in the NA and CA samples (6.79 ± 0.25 and 6.40 ± 0.16, respectively) and differences were not significant. Both conditions were dominated by the genera Streptomyces, Actinocatenispora, and Rhodanobacter (Figure 1B; Table S3). After CA, low abundant taxa (<1% relative abundance) remained equal at 29%. Again, a similar number of ASVs were considered at low abundance under NA and CA conditions (20% and 15%, respectively). ITS analysis showed 25 distinct ASVs at the genus level (Figure 1C). Ascomycota and Apiotrichum each represented a third of the ASVs in the rhizosphere irrespective of conditions (Figure 1C; Table S3). Alpha diversity was significantly different (p < 0.05, two-tailed t-test) at 3.43 ± 0.06 in the CA and 3.05 ± 0.17 in the NA.
Although there were few changes in the rhizosphere community following 6 days at 4 °C, differential abundance testing using ANCOM with bias control and parsed for taxa above the assigned low relative abundance thresholds (Figure 1) identified two modestly differentially abundant species (out of 143; 1.4%) in the shotgun data. Kribbella qitaiheensis (log2 fold change: 0.37) and Kribbella flavida (log2 fold change: 0.38) increased in relative abundance after CA (Figure 2A). In addition, the relative abundance of three fungal genera (out of 25; 12%) changed following CA, including a decrease in Penicillium (log2 fold change: −1.8) and Phialemonium (log2 fold change: −1.7) and a more substantial relative increase in Pseudogymnoascus (log2 fold change: 8.43) (Figure 2B).
Figure 2. Differentially abundant taxa between the non-acclimated and cold-acclimated Brachypodium distachyon rhizosphere microbiomes as determined by ANCOM-BC and showing their average relative abundance in both conditions and log2 fold changes with error bars representing standard error: (A) species identified by Kraken2 from shotgun sequencing data that are differentially abundant and above an average relative abundance threshold of 0.2%, and (B) ITS amplicon sequence variants that are differentially abundant. Only statistically significant changes are shown, as determined by ANCOM-BC.
Although shifts in the rhizosphere community appeared modest, the Bray–Curtis dissimilarity analysis showed that the shotgun rhizosphere communities were significantly different under the two temperature regimes (p < 0.01, pairwise PERMANOVA) (Figure 3A). In contrast, there were no differences in Bray–Curtis dissimilarity for the amplicon analysis, either for 16S (Figure 3B) or ITS data (Figure 3C). Taking all the results together, it appears that overall, the CA regime resulted in only a very minor shift in the rhizosphere microbial community. Researchers speculate that a longer period of low temperature with concomitant changes in root exudates would be required for a more dramatic change in the root-associated microbiota.
Figure 3. Principal coordinate analysis comparing non-acclimated and cold-acclimated conditions in each sample type for each sequencing method, for the following samples: (A) shotgun sequencing in the rhizosphere, (B) 16S rRNA sequencing of the V4 region in the rhizosphere, (C) ITS sequencing of the rhizosphere samples, (D) shotgun sequencing of the leaf samples, (E) 16S rRNA sequencing of the V4 region in the leaf samples, and (F) ITS sequencing of the leaf samples. Pairwise PERMANOVAs were conducted between conditions with significance as noted.
5. Cold Acclimation and the Leaf Microbiome
Although shotgun sequencing of the leaf, representing the endosphere and phyllosphere microbiomes, identified 143 microbial species with the most abundant taxa shown (Figure 4A; Table S4), an average of 92 ± 4% of the reads remained unclassified, with a portion of these likely attributable to as yet unsequenced host mitochondrial sequences (Figure S4C). Bacteria accounted for ~100% of the microbiota except in a couple of samples from which a few fungal sequences were recovered. Overall, alpha diversity was significantly lower (p < 5 × 10−6, two-tailed t-test) in leaf samples (3.18 ± 0.36) compared to rhizosphere samples (4.99 ± 0.21).
Figure 4. Average relative abundance of the taxonomies of the non-acclimated and cold-acclimated Brachypodium distachyon leaf microbiomes representing the endosphere and phyllosphere: (A) species identified from shotgun sequencing and metagenomics classified using a custom Kraken2 database, (B) distinct amplicon sequence variants assigned down to the genus or lowest possible level by QIIME2 using the SILVA database for 16S rRNA sequences, amplified using the V4 region of prokaryotes, and (C) distinct amplicon sequence variants assigned down to the genus or lowest possible level by QIIME2 using the UNITE database for ITS regions of eukaryotes.
Leaf alpha diversity did not significantly change after CA treatment (mean Shannon indices at 3.30 ± 0.29 in NA samples and 3.06 ± 0.47 in CA samples). However, the taxa profile changed with the cyanobacteria Microcystis aeruginosa, decreasing from ~27% to ~13% relative abundance after CA. Streptomyces sp. M2 showed the opposite profile, increasing from ~4% to ~15% average relative abundance after transfer to 4 °C. NA leaves were dominated by the plant pathogens Pseudomonas syringae and ‘Candidatus Liberibacter africanus’, as well as the plant beneficial Rhodococcus qingshengii, whose levels substantially decreased in the CA conditions. Lower abundant reads (<1%) made up about a quarter of the taxa, similar to the CA samples.
Amplicon sequencing of the 16S rRNA from the leaves identified 188 distinct ASVs at the genus level (with the most abundant shown in Figure 4B and Table S4). Again, alpha diversity was not significantly different between conditions (5.04 ± 0.25 and 4.60 ± 0.70 in the CA and NA samples, respectively). Taxa present under both conditions included the genera Solimonas, Rhodanobacter, and Streptomyces. Pseudomonas and Rhodococcus were abundant (21% and 15% average relative abundance, respectively) in NA conditions, but decreased in relative abundance after transfer of the plants to 4 °C with log2 fold changes of −4.18 and −5.41, respectively. The cereal growth-promoting genus Nocardioides and an unidentified genus from the same family, Nocardioidaceae, both increased in abundance to represent 11% of the taxa in CA plants. ASVs at low relative abundance (<1%) made up a similar 18% and 21% of CA and NA 16S samples, respectively. ITS analysis resulted in 20 distinct ASVs at the genus level (Figure 4C).
After shotgun sequence analysis, 3.5% (5/143) of the taxa were identified as differentially abundant between the NA and CA conditions (Figure 5A). After transfer to 4 °C, reads attributed to P. syringae (log2 fold change: −8.68) and R. qingshengii (log2 fold change: −8.33) decreased so that there was a change in the estimated average relative abundance of P. syringae and R. qingshengii from 8.2% and 5.0% to 0%, respectively. At the same time there was a corresponding increase in the relative abundance of Streptomyces sp. M2 (log2 fold change: 2.81), A. sera (log2 fold change: 3.20), and A. thailandica (log2 fold change: 3.87). In 16S CA samples, nine other taxa increased, including the genus Solimonas, which increased in relative abundance but was below the low abundance threshold. In total, 5.9% (11/188) of the identified sequences above the threshold were found to be differentially abundant. For the ITS analysis, the genus Phialemonium represented 5% (1/20) of the ASVs and decreased in relative abundance (log2 fold change: −10.6) (Figure 5C).
Figure 5. Differentially abundant taxa between the non-acclimated and cold-acclimated Brachypodium distachyon leaf microbiomes representing the endosphere and phyllosphere as determined by ANCOM-BC and showing their average relative abundance in both conditions and log2 fold changes with error bars representing standard error: (A) species identified by Kraken2 from shotgun sequencing data that are differentially abundant and above an average relative abundance threshold of 1%, (B) distinct 16S rRNA amplicon sequence variants assigned by QIIME2 and the SILVA database to the genus level that are differentially abundant, and (C) distinct ITS amplicon sequence variants assigned by QIIME2 and the UNITE database to the genus level that are differentially abundant. Only statistically significant changes are shown as determined by ANCOM-BC.
Despite the apparent community differences, Bray–Curtis dissimilarity analysis suggested that the microbial communities identified with the shotgun sequencing approach were not significantly different, undoubtedly due to the low number of sequences (Figure 3D), similar to the leaf ITS communities. Supporting that conclusion, 16S rRNA communities were shown to be significantly different between conditions (p < 0.01, pairwise PERMANOVA) with the analysis supported by high ASV numbers (Figure 3E).
6. Dissimilarity Comparisons and Core Microbiome
The root and leaf-associated microbiomes were further independently characterized with SIMPER to identify taxa that contributed the most dissimilarity between NA and CA regimes (Table 1). For microbiota isolated from the rhizosphere, the taxa contributing to the top ~25% of dissimilarity were Streptomyces sp. M2, A. sera, and A. thailandica for the shotgun data, the genera Actinocatenispora and Streptomyces for the 16S data, and the genera Phialemonium and Apiotrichum for the ITS data. For leaf samples, taxa contributing to the top ~25% dissimilarity were M. aeruginosa and Streptomyces sp. M2 for the shotgun data, the genera Pseudomonas and Rhodococcus for the 16S data, and the genera Aspergillus and Goidanichiella for the ITS data.
Table 1. Similarity of percentage (SIMPER) analysis of microbiota contributing to the top ~25% of dissimilarity (Bray–Curtis) between non-acclimated (NA) and cold-acclimated (CA) samples (showing average relative abundance in %) in both leaf tissue and rhizosphere performed in PAST (v4.08).
Taxa |
NA (%) |
CA (%) |
Average Dissimilarity |
Contribution (%) |
Cumulative (%) |
Shotgun Rhizo (Overall Average Dissimilarity 7.1%) |
Streptomyces sp. M2 |
32.5 |
32.8 |
0.9 |
12.8 |
12.8 |
Actinocatenispora sera |
5.7 |
7.3 |
0.8 |
11.2 |
24.0 |
Actinocatenispora thailandica |
3.6 |
4.9 |
0.6 |
9.1 |
33.1 |
16S Rhizo (Overall Average Dissimilarity 10.7%) |
Actinocatenispora |
8.1 |
11.4 |
1. 7 |
15.7 |
15.7 |
Streptomyces |
24.2 |
26.6 |
1.4 |
13.4 |
29.2 |
ITS Rhizo (Overall Average Dissimilarity 19.0%) |
Phialemonium |
21.3 |
13.4 |
4.0 |
21.1 |
21.1 |
Apiotrichum |
29.9 |
30.8 |
4.0 |
21.1 |
42.2 |
Shotgun Leaf (Overall Average Dissimilarity 52. 6%) |
Microcystis aeruginosa |
12.1 |
27.4 |
9.6 |
18.2 |
18.2 |
Streptomyces sp. M2 |
4.4 |
15.1 |
5.4 |
10.2 |
28.4 |
16S Leaf (Overall Average Dissimilarity 60.9%) |
Pseudomonas |
19.7 |
1.0 |
9.4 |
15.4 |
15.4 |
Rhodococcus |
15.0 |
0.4 |
7.3 |
12.0 |
27.4 |
ITS Leaf (Overall Average Dissimilarity 80.3%) |
Aspergillus |
15.9 |
47.5 |
16.6 |
20.7 |
20.7 |
Goidanichiella |
25.1 |
0.0 |
12.6 |
15.7 |
36.4 |
Highly conserved taxa that are present in most samples, typically ~70%, can be considered part of the “core” microbiome that orchestrates the interactions between the host and the microbiota
[22]. As described in the methods, researchers employed strict criteria that the taxa must appear in all of the samples for each condition (
Table 2).
In the rhizosphere, the core microbiota identified in the shotgun analysis included Streptomyces sp. M2 and Actinocatenispora sera. Core taxa in the leaves included Streptomyces sp. M2 and ‘Candidatus Liberibacter africanus’, both of which persisted across the two different conditions and all samples. The larger number of taxa associated with the rhizosphere ASVs were consistent with the microbes identified by shotgun analysis and indicated bacterial (Streptomyces, Actinocatenispora, and Rhodanobacter) as well as fungal taxa (Ascomycota, Apiotrichum, Phialemonium, and Candida) as contributors to the core microbiome. Leaf ASVs revealed that bacteria (Streptomyces, Rhodanobacter, and Solimonas), as well as a single unidentified fungal sequence, comprised the core.
Table 2. Core microbiota taxa (species or distinct ASVs as indicated) present in 100% of samples for each sequencing and analysis method of shotgun, 16S rRNA, and ITS sequencing methodologies with an average relative abundance >5%.
Phyla |
Class |
Order |
Family |
Genus |
Species |
Core rhizosphere species (shotgun) |
Actinobacteria |
Actinomycetia |
Streptomycetales |
Streptomycetaceae |
Streptomyces |
Streptomyces sp. M2 |
Actinobacteria |
Actinomycetia |
Micromonosporales |
Micromonosporaceae |
Actinocatenispora |
Actinocatenispora sera |
Core rhizosphere genera (16S) |
Actinobacteria |
Actinomycetia |
Streptomycetales |
Streptomycetaceae |
Streptomyces |
|
Actinobacteria |
Actinomycetia |
Micromonosporales |
Micromonosporaceae |
Actinocatenispora |
|
Proteobacteria |
Gammaproteobacteria |
Xanthomonadales |
Rhodanobacteraceae |
Rhodanobacter |
|
Core rhizosphere genera (ITS) |
Ascomycota |
|
|
|
|
|
Basidiomycota |
Tremellomycetes |
Trichosporonales |
Trichosporonaceae |
Apiotrichum |
|
Ascomycota |
Sordariomycetes |
Sordariales |
Cephalothecaceae |
Phialemonium |
|
Ascomycota |
Saccharomycetes |
Saccharomycetales |
Saccharomycetaceae |
Candida |
|
Core leaf species (shotgun) |
Actinobacteria |
Actinomycetia |
Streptomycetales |
Streptomycetaceae |
Streptomyces |
Streptomyces sp. M2 |
Proteobacteria |
Alphaproteobacteria |
Hyphomicrobiales |
Rhizobiaceae |
Liberibacter |
* ‘Candidatus L. a.’ |
Core leaf genera (16S) |
Actinobacteria |
Actinomycetia |
Streptomycetales |
Streptomycetaceae |
Streptomyces |
|
Proteobacteria |
Gammaproteobacteria |
Xanthomonadales |
Rhodanobacteraceae |
Rhodanobacter |
|
Proteobacteria |
Gammaproteobacteria |
Salinisphaerales |
Solimonadaceae |
Solimonas |
|
Core leaf genera (ITS) |
Unidentified Fungi |
|
|
|
|
This entry is adapted from the peer-reviewed paper 10.3390/plants10122824