In each agro-climatic environment, yields of maize plants are influenced by several components linked to agronomic practices that are used. These are plant population density (determines number of cobs and kernels harvested in a given area), the shelling percentage, and the amount of water in the harvested grains. The magnitude of yield components is a function of the physiological response of a crop to the growth environment, which is of great importance to maize physiologists, modelers, and breeders.
2.1. Plant Density and Kernel Number
Plant density refers to the total number of plants grown in a given area, and it is an important factor in yield estimation. Maize density is calculated based on row spacing, row length, number of plants per planting station, and the distance between two consecutive planting stations. Ali et al.
[12] estimated maize plant density per plot at physiological maturity by counting the total plants in the plot and dividing by soil area. Maize density has been widely investigated worldwide. An increase in plant density results in relatively higher yield increments if the appropriate fertilization rates and agronomic practices are adopted
[13]. Huang et al.
[14] found a 5% yield increase of diamond plants at high density (90,000 plants ha
−1) resulted in increased kernel numbers per ear in the top and middle canopy layers. Moreover, they reported that the optimum distribution of light in the canopy delayed leaf senescence, especially for plants with a triangle shape. In a dense population, canopy architecture becomes an important factor determining yield because of interplant competition for light distribution and absorption
[15].
Maize grain yield is normally highly and positively correlated with kernel numbers. The number of kernels per plant depends on the number of ears per plant and the number of mature kernels per ear
[16]. To produce more grain per unit area, the genetic potential of most recent hybrids takes advantage of their capacity to withstand higher densities
[17]. Qian et al.
[18] reported that spring maize hybrids attained an average increase of 17.9 g per plant per decade, which corresponds to an increase of 936 kg/ha per decade over the period from 1970 to 2010 in Northeast China. The yield gain was attributed to an increase of yield per plant, resulting from an increase in number of kernels per ear and an increased 1000 kernel weight under appropriate agronomic practices.
Several spacings have been recommended, given a required number of plants per unit area in various regions in the world, as indicated in the map (Table 1). The various distances between rows and hills in a row have been estimated for a resultant density of 53,000 plants per hectare. Plant spacing impacts the number of individual plants grown on a given area and will therefore influence the number of ears harvested and the resultant yield. Plant spacing is usually based on the agro-climatic conditions, the plant material used, and the cropping system (monoculture or mixed culture). Therefore, spacing varied from region to region and sometimes from one country to another. Large distances between rows facilitate use of mixed cropping systems. The number of plants can be increased by either reducing the spacing between hills in a row or reducing the distance between rows. When the distance between two consecutive hills is very small, the number of plants per hill tends to be one.
Table 1. Some plant densities and spacings used in various agro-ecological environments for maize production.
Country |
Environment |
Plant Density/ha |
Spacing between Rows (cm) |
Spacing within the Row (cm) |
Average Mean Yield per Region (t/ha) |
References |
Hungary |
Humid forest zone |
67,486 to 70,161 |
70 |
20 |
8.5 |
[19] |
Kenya |
Tropical climate and bimodal rainfall Nairobi |
44,444 53,333 |
75 75 |
60 25 |
2.0 |
[20] [21] |
Serbia |
Calcareous chernozem on loess terrace |
60,606 |
75 |
22 |
4.9 |
[22] |
Rwanda |
Congo–Nile Crest region |
55,000 |
60 |
30 |
4.0 |
[23] |
Pakistan |
Faisalabad |
66,500 |
75 |
20 |
2.9 |
[24][25] |
India |
Coimbatore |
66,667 |
60 |
25 |
2.7 |
[26] |
Cameroon |
Low and high land areas |
53,333 |
75 |
50 |
1.8 |
[27][28][29][30][31] |
Nigeria |
Northern Guinea Savana agro-ecological zone |
53,333 |
75 |
25 |
5.5 |
[32] |
Brazil |
Frederico Westphalen |
70,000 |
70 |
20 |
5.6 |
[33] |
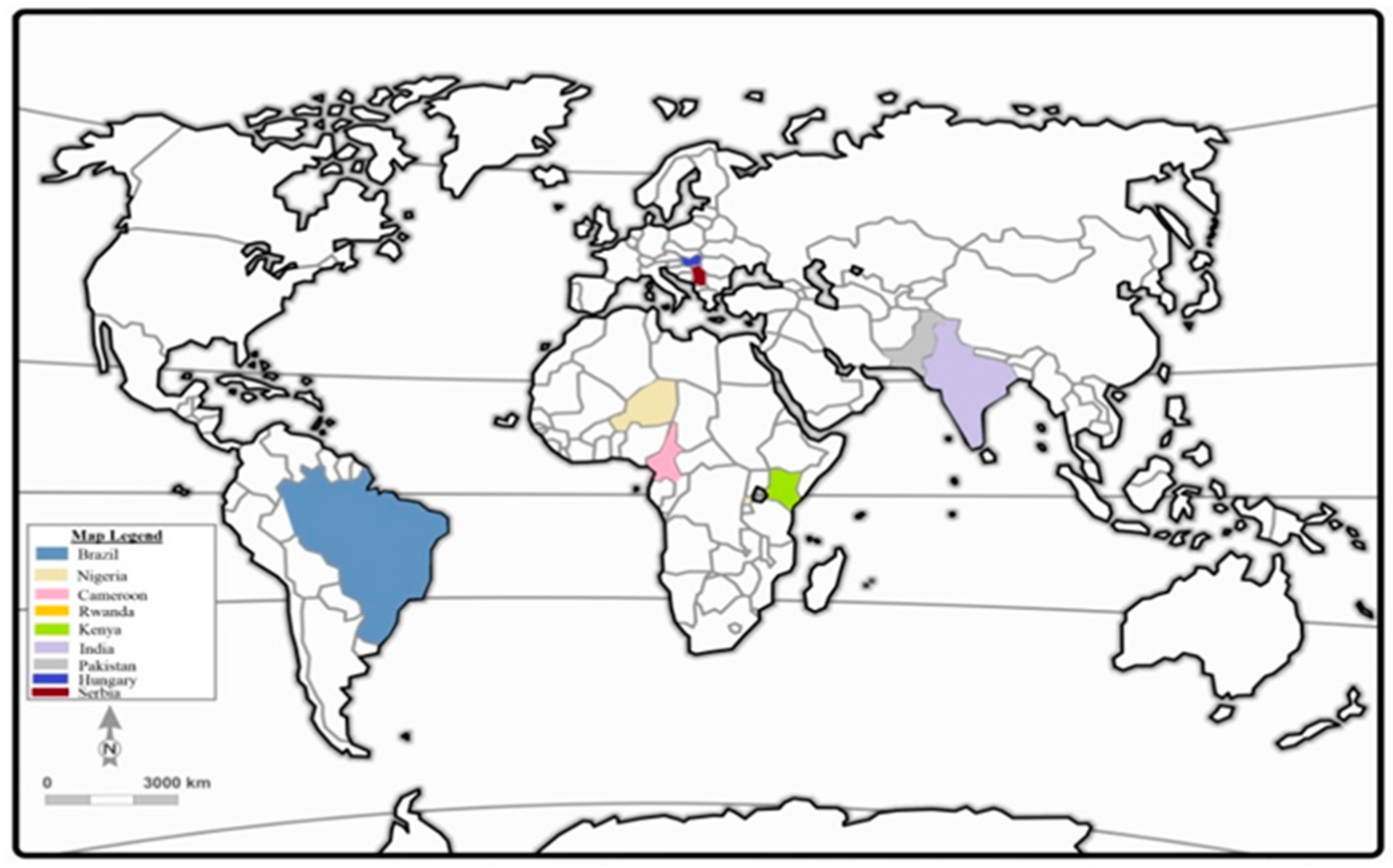 Map modified from [34]: World view of the countries listed above. |
An increase in maize density significantly increased plant height, ear height, and yield. However, some yield parameters such as ear length, number of grains per row, number of grains per ear, grain weight per ear, cob weight, and 1000 grain weight were decreased by increase in maize density
[35]. This is because of the competition among plants for nutrients uptake from the soil and for light absorbance as well. Some individuals would tend to grow taller and some failed to bear ears, resulting in significant decreases in overall yield. Short maize varieties could be grown at higher plant populations than the taller varieties, which may be susceptible to lodging under high population density. The environmental conditions and genetic potential of some maize genotypes allow them to tolerate high plant density. Mandić et al.
[35] reported that a crop density of 71,429 plants ha
−1 was optimal for growing Dijamant 6 hybrid maize in the Srem region of Serbia, because at that density, the cited hybrid efficiently used available resources to achieve higher grain yield (grain yields increased by about 0.72 to 1.51 t/ha with the increase of plant density). The newly developed maize hybrids by Tokatlidis and Koutrubas
[36] resulted in a yield increase at optimum plant density. This could be attributed to their genetic potential to perform well in high plant density.
The factors facilitating modern maize genotypes to produce maximum yield in high plant density include orientation, size, and distribution of leaf area which determines light interception and overall photosynthesis in a crop canopy. Therefore, optimum architecture allows modern maize genotypes to be more productive with improved tolerance to high plant density. It is also expected that maize tolerates high plant density when grown at high altitude because of light availability, less daytime heat stress, and low night temperature. In general, plant density is closely related to grain yield estimates. Additionally, high density is appropriate for early-planted crops under high rainfall or irrigated conditions with good management standards.
2.4. Harvest Area
The use of global positioning system (GPS) technology provides an affordable and more reliable alternative method for measurement of plot area harvested in large scale production. Ngie and Ahmed
[51] used combine harvesters that recorded the grain weight per hectare within 20 m × 20 m ranges (in kg/ha), associated with a GPS system which recorded the coordinates of the plots against the dry weight of the harvested grain. However, the easiest way of estimating the harvest area for small plots of land consists of multiplying row length by the space between two consecutive rows, and factoring in the number of rows in the plot using the following formula:
where harvest area is in m
2, row length is in m, and intra–row distance is in m.
3. Grains yield estimates
Crop yield represents a culmination of the efficiency of the plant population to use available environmental resources for its growth [53].Crop productivity per unit area is one of the indicators for agricultural development. The estimation of crop yield involves estimation of crop area and quantity of harvested products [11].
3.1 Yield estimation from experimental plots
According to Cassman [54], yield potential (Yp) is defined as the biophysical yield obtained with adequate water to avoid deficits, appropriate temperature regime determining the length of the growing season, and the optimum amount of solar radiation during the growing season. However, potential yield (Yw) is obtained from non-irrigated crops or rain-fed crops, which are exposed to water-limited conditions depending on the quantity, the timing of rainfall, and the capacity of soil to store water. The potential yield is obtained from the well-managed crop in a given set of conditions. The determination of grain yield (GY) based on grain weight can be adjusted to a required percentage of moisture content (varies from 10 to 15%) using the following formula [38]:
GY (t/ha) = [Grain weight x 10 x (100 - MC) / ((100 - Adjusted MC) / (Plot area))] (3) Where GY is in kg, moisture content (MC) is in percentage and plot area is in meter square.
If GY is to be calculated using the ear fresh weight and the adjusted MC percentage (between 10 to 15%), the following formula is recommended:
GY (t/ha) = [(Fresh ear weight (kg/plot) x 10 x (100-MC) x 0.8) / ((100 - adjusted MC) x Plot area)] (4) where 0.8 is the shelling coefficient.
3.2 Yield estimation from Farmers' fields
The harvest yield from farmers' fields is known as actual or realized yield. Most often, in smallholder farms, maize planting does not follow any rule making the estimation of plant density difficult as well as the estimation of the harvest yield. The estimation of crop yield can be complicated by heterogeneous performance of a given crop within a plot, continuous planting and use of mixed cropping systems.
The estimation of crop yield using test weight technique is one of the easiest and quickest pre-estimation methods under farm conditions. This method is based on the sampling frame and can be applied in any farm. The number of ears per planting station is counted in one meter square area, repeated at least 5 to 7 times within the entire plot, where yield is to be determined and the average number is taken. Similarly, the number of kernel rows per ear is counted in 20 to 25 ears randomly selected and the average is used. 1000 kernels are sampled randomly from the ear and weighed. The yield of the crop is then calculated using the following formula modified from Sapkota et al. [69]:
Yield (kg/ha) = [(number of kernel rows per ear x number of ears per meter square /100) x (weight of 1000 kernels (g) / 1000) x 10,000] (5)
The result obtained from the above formula can be multiplied by 1000 to express the yield in t/ha.
3.3 Complex models used in yield estimation
Crop simulation methods can be used to estimate crop growth, yield and improve agricultural management systems by allowing farmers to be prepared for climatic conditions of the forthcoming season [9,72-75]. Crop modelling and remote sensing are two methods that enable government agencies, private industry and researchers to estimate yield before harvest. Numerous studies have been conducted to predict crop yield at regional scale using remote sensing approaches, yield modelling and a combination of the two methods. There are numerous crop simulation models that take into account the interactions of a crop with climatic conditions, soil properties, and agronomic management practices.
4. Yield simulation
Crop simulation models are mathematical representations of complex real-world systems [76], which can mimic crop growth and estimate crop yield on the basis of weather (precipitation, temperature and solar radiation), soil, and crop management conditions [77,81]. Field crop yield production is important for relevant design of grain storage facilities, agricultural field management, as well as national agricultural decision-making. However, complex models are not always appropriate and do not provide reliable information in all situations because they may require inputs that cannot practically be obtained in field situations [79]. Table 2 summarizes some models that were previously used at various stages of maize production.
Table 2. Some simulation models applied in maize production.
Simulation Model |
Objective |
Reference |
Multi-model forecast and single model forecast.
CORN-CROPS model
Conformal Cubic
Atmospheric model (CCAM) and ECHAM 4.5 model
CERES-Maize crop simulation model
World Food Studies (WOFOST) model
|
Early warning during preparation foe the new season
Simulate the interaction of management practices and weather in determining maize yields
Yield estimation
Potential yield estimation of maize
Simulate the growing process of spring maize
|
[84]
[87]
[88]
[89]
[90]
|
5. Remote sensing
Remote sensing is a technique to observe the earth's surface, the atmosphere from space using satellites (space borne), or from the air using aircrafts (airborne). In other words, it refers to the activities of recording / observing / perceiving (sensing) objects or events from faraway (remote) places [91]. Remote sensing is a dynamic monitoring yield estimation technique used in diverse types of crops which can estimate crop yields on a large scale and provide relevant results. It is an important tool for generating agricultural statistics because of the synoptic view and online information provided in a short period of time which can be used to predict yield before harvesting [11,51].
Remote sensing can be integrated with geographic information system (GIS) technologies and / or with satellite method. The estimation method relates the vegetation indices with the final yield at a specific growth stage of the plant (vegetative and reproductive stages) durng the growing season [92-95].
Remote sensing forms a base for estimating parameters of spatial variability through a very large area frame sample design. It provides an efficient and low-cost stratification based on crop proportion derived from visual interpretation or digital classification of remote sensing data.Remote sensing makes the estimates based on ground surveys near-real time monitoring of crops, very easy derivation of vegetation (covers hilly terrains well) and reduces the amount of field data to be collected [11,99].
When prediction yield using remote sensing, the leaf area index (LAI) is an important variable contributing to determination of the reflectance value of a crop in an image, and as NDVI, which is related to crop vigor and biomass.
5.1 Yield gap between potential and actual yield
In farmers' fields, yields obtained from maize crops (actual or attainable yield) are usually lower than the expected yield (potential yield). A multitude of factors contribute to such yield decreases, and they include poor agricultural policies that restrict affordability and access to production inputs.Other constraints contributing to yield losses in farmers' fields are inadequate or incorrect fertilizer application rates, biotic and abiotic constraints, unavailability of improved seeds, and high cost of labor [54].
6. Conclusion
Yield estimation is critical in any crop production system. The estimation of potential yields takes into account production are, plant density, kernel moisture content and sometimes shelling percentage. In smallholder farms, the estimation of yield is challenging because of continuous planting and use of mixed inter-cropping systems. Nevertheless, maize yield production can be broadly estimated under such conditions. Crop yield simulation models and remote sensing provide government agencies, private industries and researchers the option to estimate yield before harvest and can help farmers to be well prepared for the forthcoming growing season. These methods of yield estimation are expensive and not accurate for small plot sizes. Farmer estimation techniques remain the cheapest and are faster compared to any other methods of field estimation at farmer level.