2. State-of-the-Art Recent Surveys
Several surveys have been published to advance the field and address its challenges. Authors of [
18,
19,
20] presented a systematic literature review that summarized and analyzed the research articles conducted on character recognition of handwritten text documents across six languages in order to highlight research directions. Others conducted a survey on automated approaches in character recognition of historical documents [
21,
22]. The studies covered historical manuscripts in various languages, including Indian, Kurdish-Latin, Arabic, ancient Chinese, and others. They also summarized the techniques used for data pre-processing and the types of datasets utilized. Additionally, in [
23], authors conducted a survey on state-of-the-art applications, techniques, and challenges in Arabic language character recognition. In [
24], authors surveyed the challenges of the recognition and classification of named entities in various historical resources and languages, including the French language. Additionally, they discussed the employed approaches in the named entity recognition (NER) field and highlighted directions for future developments.
In [
25], authors introduced an open database of historical handwritten documents that are fully annotated in the Norwegian language. To assess the performance of state-of-the-art HTR models on their dataset, they conducted a systematic survey of open-source HTR models, including twelve models with different characteristics, Their study highlighted the best performing technique and suggested a combination of different models to further improve performance. In [
26], authors present a systematic literature review of image datasets for historical document image analysis at two scales: document classification and layout structure or content analysis. The research aims to assist researchers in identifying the most suitable datasets for their techniques and to advance the field of historical document image analysis. Similarly, others focused on the databases and benchmarks of this field [
27].
On the other hand, authors of [
28] surveyed the major phases of historical document digitization process, focusing on the standard algorithms, tools, and datasets within the field. Their research highlighted the critical importance of transcription accuracy as a prerequisite for meaningful information retrieval in archival documents. In contrast, authors of [
29] focused on the feature extraction phase in handwritten Arabic text recognition.
Additionally, in [
30], authors presented a critical study of various document layout analysis (DLA) techniques aimed at detecting and annotating the physical structure of documents. The survey highlighted the different phases of DLA algorithms, including preprocessing, layout analysis strategies, post-processing, and performance evaluation. This research serves as a base step toward achieving a universal algorithm suitable for all types of document layouts.
In the study [
31], authors discussed the importance of separating machine-printed texts and handwritten texts in hybrid-form documents to enhance the overall system accuracy. The discussion involved techniques employed for the separation process based on feature extraction methods for three categories: structural and statistical features, gradient features, and geometric features.
This research establishes an updated state-of-the-art survey of HTR systems, different languages datasets, HTR competitions, and HTR commercial systems of the French language and other international ones.
3. Handwritten Text Recognition Workflow
The workflow of handwritten text recognition includes classical image processing approaches and deep learning approaches. Figure 2 illustrates the general pipeline of the HTR workflow.
Figure 2. Handwritten text recognition general pipeline.
3.1. Image Digitization
It is the process of transforming a handwritten text image into an electronic form using various devices such as scanners and digital cameras. This form of the image can be used as the input to the pre-processing stage.
3.2. Pre-Processing
Pre-processing is the initial stage in enhancing digital images, it involves several key processes such as:
-
Binarization: This process involves converting digital images into binary images consisting of dual collections of pixels in black and white (0 and 1). Binarization is valuable for segmenting the image into foreground text and background.
-
Noise removal: This process involves eliminating unwanted pixels from the digitized image that can affect the original information. This noise may originate from the image sensor and electronic components of a scanner or digital camera. Various methods have been proposed for noise removal or reduction, such as Non-local means [
32] and Anisotropic diffusion [
33], as well as filters like Gaussian, Mean, and Median filters.
-
Edges detection: This process involves identifying the edges of the text within the digitized image using various methods such as Sobel, Laplacian, Canny, and Prewitt edge detection.
-
Skew detection and correction: Skew refers to the misalignment of text within a digital image. In other words, it indicates the amount of rotation needed to align the text horizontally or vertically. Various methods for skew detection and correction have been proposed to address this objective, such as Hough transforms and clustering.
-
Normalization: This process involves reducing the shape and size variation of digital images. Additionally, it scales the input image features to a fixed range (e.g., between 0 and 1), while maintaining the relationship between these features. This process plays a valuable role in the training stage of deep learning models.
3.3. Segmentation
This process involves dividing the handwritten text image into characters, words, lines, and paragraphs, often utilizing the pixel characteristics within the image. Several methods have been developed to conduct the segmentation process, including threshold methods, region-based methods, edge-based methods, watershed-based methods, and clustering-based methods. The segmentation stage is considered as one of the most crucial steps that can significantly improve the accuracy of HTR models [
34].
3.4. Feature Extraction
This process involves extracting specific information from the images that precisely represents the image, with the goal of reducing the size of high-dimensional data. The feature extraction process aims for higher discriminating power and control overfitting problems within HTR models. However, it may lead to a loss of data interpretability [
35]. The most popular techniques for feature extraction include Principal Component Analysis (PCA), Linear Discriminant Analysis, and Independent Component Analysis (ICA). The accuracy of HTR systems is highly dependent on the choice of feature extraction techniques.
3.5. Classification
This process involves deciding the class membership in the HTR system. The decision-making criteria depend on comparing the input feature with a predefined pattern to identify the most suitable matching class for the input. Two key methods can be employed in this stage. First, the template-based method [
36] calculates the correlation between the input and the predefined pattern. Second, the feature-based method [
37] utilizes the extracted features from the input for classification.
3.6. Post-Processing
This stage aims to improve the results of the classification stage and enhance the overall accuracy of the HTR models. It involves output error correction using various techniques such as Dictionary lookup and statistical approaches. However, this stage is not compulsory in the HTR models development process.
4. Advancements in Handwritten Text Recognition: A State-of-the-Art Overview
Languages, by nature, vary in their letter shapes and word connections. The process of recognizing handwritten text is complicated due to many impediments such as the variety of writing style, documents poor quality, noise, spots on paper, and text alignment.
Researchers have proposed several models for recognizing language scripts using different architectures, including Convolutional Neural Network (CNN), Convolutional Recurrent Neural Network, sequence-to-sequence Transformer, Bidirectional Long Short-Term Memory (BLSTM), and others.
In a recent study, a Deep Learning (DL) system using two CNN architectures, named HMB1 and HMB2, was employed to recognize handwritten Arabic characters [
38]. The models were trained using a complex Arabic handwritten characters dataset (HMBD), as well as CMATER [
39,
40] and AIA9k [
41] datasets. The model demonstrated a significant accuracy rate when testing HMB1 on HMBD, which further improved when a data augmentation process was applied. Additionally, CMATER and AIA9k datasets were utilized to validate the generalization of the model. In the same context, two different architectures, namely the transformer transducer and sequence-to-sequence transformer, have been established in [
42]. These architectures were evaluated using the KFUPM Handwritten Arabic TexT (KHATT) dataset [
43,
44,
45]. Several pre-processing steps, such as text lines rotation and elimination of empty spaces, were performed. An attention mechanism was then applied to reduce the model complexity and achieve satisfactory performance in terms of accuracy and latency, surpassing the previous literature on the KHATT benchmark. Similarly, in [
46], a light encoder–decoder transformer-based architecture was introduced for handwriting text recognition, while in [
47], an end-to-end approach with pre-trained image transformer and text transformer models for text recognition at the word level was proposed. More survey articles and recent research works for recognizing handwritten text can be found in [
17,
48,
49,
50,
51,
52].
Other researchers introduced Attention-Based Fully Gated CNN supported by multiple bidirectional gated recurrent units (BGRU), with a Connectionist Temporal Classification (CTC) model to predict the sequence of characters [
53]. They evaluated this method using five well-known datasets within the HTR community, which encompassed Institut für Informatik und Angewandte Mathematik (IAM) [
54], Saint Gall [
55], Bentham [
56], and Washington [
57] for English, as well as the Russian–Kazakh dataset (HKR) [
58]. The method achieved a remarkably high recognition rate with minimal parameter usage when applied to the latter dataset.
Furthermore, a novel approach was introduced that combines depthwise convolution with a gated-convolutional neural network and bidirectional gated recurrent unit [
59]. This technique effectively reduces the total number of parameters while simultaneously enhancing the overall performance of the model. Authors of [
15,
60] presented Convolutional Recurrent Neural Network (CRNN) architecture; the latter utilized CRNN for handwriting recognition as an encoder for the input text lines while utilizing a Bidirectional Long Short-Term Memory (BLSTM) network followed by a fully CNN as a decoder to predict the sequence of characters. IAM and Reconnaissance et Indexation de données Manuscrites et de fac similÉS (RIMES) [
61] with the newly created dataset (EPARCHOS) [
60] that includes historical Greek manuscripts have been used in the evaluation process of the proposed architecture. Experiments produced improved results compared with the other state-of-the-art methods, especially on the RIMES dataset.
Table 1 summarizes the articles based on the architectures used at different HTR levels for different international languages.
Table 1. State-of-the-art architectures utilized for handwritten text recognition of different international Languages datasets such as English, Arabic, Russian, and others at different prediction levels.
Conversely, many researchers evaluated various models on the French handwritten text. In the competition organized at ICDAR2011 [
63] using the RIMES dataset, authors of [
64] presented a combination of three techniques of multi-word recognition. Firstly, Grapheme-based Multilayer perceptron (MLP)-HMM was used to decompose the words into letters. Secondly, sliding window Gaussian mixture HMM was used to model the letters with a HMM model using a Gaussian distribution mixture for the observation probabilities. Finally, training a MDLSTM-RNN model using raw values of pixels represents words as inputs. The system recorded an advanced recognition rate on the RIMES dataset for both word and multi-word recognition tasks. Similarly, an MDLSTM-RNN-CTC model using Graphics processing unit (GPU)-based implementation was proposed in [
65] to decrease the model training time by processing the input text lines in a diagonal-wise mode, while in [
66], authors applied the concept of curriculum learning to the MDLSTM-Convolution Layers-Fully Connected Network (FCN)-CTC model in order to enhance the learning process speed. Additionally, an attention-based RNN-LSTM architecture was proposed in [
67]. This architecture was evaluated using the RIMES 2011 dataset. Another research study [
66] on datasets such as IAM, RIMES, and OpenHaRT demonstrated significant improvement rates as a result of applying the curriculum learning concept to their model. However, due to alignment issues commonly observed in attention models caused by the recurrence alignment operation, authors of [
68] introduced a decoupled attention network (DAN). The DAN is an end-to-end text recognizer that comprises three components. Firstly, a feature encoder is utilized to extract visual features from the source image. Secondly, a convolutional alignment module is employed. Finally, a decoupled text decoder is used for the prediction stage. The model has undergone numerous experiments using IAM and RIMES datasets, achieving effectiveness and merit recognition rates.
Furthermore, in [
69], authors presented a system based on recurrent neural networks with weighted finite state transducers and an automatic mechanism for preparing annotated text lines to facilitate the model training process. The model used to decode sequences of characters or words on Maurdor [
70] dataset. In the same context, the work was extended to text line recognition; the approach depends on segmenting the text line into words classified based on the confidence score into an anchor and non-anchor words (AWs and NAWs), AWs were equated to the BLSTM outputs, while dynamic dictionaries were created for NAWs by exploiting web resources for their character sequence. Finally, text lines were decoded using dynamic dictionaries [
71].
Additionally, authors of [
72] introduced a combination of a deep convolutional network with a recurrent encoder–decoder network to predict the sequence of characters at the word level within IAM and RIMES datasets images. Furthermore, in [
73], authors combined CTC approaches with Sequence-To-Sequence (S2S) model to improve the recognition rate on the text line level, they developed the model based on a CNN as a visual backbone, BLSTM as encoder, and a Transformer used for character-wise S2S decoding. The evaluation process using IAM, RIMES, and Staatsarchiv des Kantons Zürich (StAZH) datasets shows competitive recognition results with 10-20 times fewer parameters.
Authors of [
74] claimed that Multidimensional Long Short-Term Memory networks might not be necessary to attain good accuracy for HTR due to expensive computational costs. Instead, they suggested an alternative model that relies only on convolutional and one-dimensional recurrent layers. Experiments were carried out using IAM and RIMES 2006 datasets and achieved faster performance with equivalent or better results than MDLSTM models. Similarly, a multilingual handwriting recognition model that leverages a convolutional encoder for input images and a bidirectional LSTM decoder was presented to predict character sequences [
75].
Additionally, as neural networks require large data amount in the training stage in order to improve the accuracy of recognition, and because the process of creating transcription data is expensive and time-consuming, authors of [
76] presented a model architecture that aims to automatically transcribe Latin and French medieval documentary manuscripts produced between the 12th and 15th centuries based on a CRNN network with a CTC loss; the model has been trained depending on The Alcar-HOME database, the e-NDP corpus, and the Himanis project [
77].
Recently, some research studies proposed an end-to-end architecture to recognize handwriting text at the paragraph level [
78,
79,
80], the latter study introduced an end-to-end transformer-based approach for text recognition and named entities from multi-line historical marriage records images in the competition ICDAR 2017 (Esposalles [
81] and French Handwritten Marriage Records (FHMR) [
82]), while in [
78], an end-to-end recurrence-free fully convolutional network named Simple Predict & Align Network (SPAN) was presented, performing OCR on RIMES, IAM, and READ 2016 at the paragraph level. Additionally, in [
79], Vertical Attention Network (VAN), a novel end-to-end encoder–decoder segmentation-free architecture using hybrid attention was introduced.
Furthermore, research in [
62,
83,
84] introduced segmentation-free document level recognition. Authors of [
62] proposed a simple neural network module (OrigamiNet) that can augment with any fully convolutional single line text recognizer to convert it into a multi-line/full page recognizer. They conducted model experiments on the ICDAR2017 [
85] competition and IAM datasets to demonstrate the applicability and generality of the proposed module, while authors of [
83,
84] presented Document Attention Network (DAN), an end-to-end architecture for full document text and layout recognition. DAN trained using a pre-defined labeling module for transcribing pages by tags style equivalent to Extensible Markup Language (XML) style aims to process the physical and geometrical information with language supervision only and reduce the annotation costs. The model predicts the document’s text lines in parallel after determining the first character of each line. It has undergone numerous experiments using RIMES 2009 and READ 2016 and showed highly beneficial recognition rates on a text line, paragraph, and document level.
Table 2 summarizes articles based on the architectures used at different HTR levels for the French language.
More methodologies on the recognition of French and other languages on different prediction levels can be found in: characters [
86,
87], words [
88,
89,
90], lines [
91,
92], paragraphs [
93,
94], and pages [
95,
96].
Table 2. State-of-the-art architectures utilized for handwritten text recognition of French language datasets at different prediction levels.
5. Commercial Systems in Handwritten Text Recognition
There are some online HTR commercial systems available for transcribing both modern and historical text such as Transkribus [
97], Ocelus, Konfuzio, and DOCSUMO. All of these systems use artificial intelligence technology to recognize the target texts in a variety of languages, including English, French, Spanish, and more. These systems can be extremely beneficial in the archives transcribing process. It is important to acknowledge that the majority of these systems demand a cost for their services. Nevertheless, this cost is frequently lower than manual transcription services cost and greatly lowers the time required for the task. Some of these systems have offered free trials of their services, where users have the opportunity to test it on a variety of handwritten text images with diverse writing styles. These HTR commercial systems could be accessed through the links summarized in
Table 3.
Table 3. List of HTR commercial systems and their corresponding links.
6. The Belfort Civil Registers of Births
The civil registers of births in the commune of Belfort, spanning from 1807 to 1919, comprises 39,627 birth records at a resolution of 300 dpi.
These records were chosen for their homogeneity, as they feature Gregorian dates of birth starting from 1807 and are available until 1919 due to legal reasons.
The registers initially consist of completely handwritten entries, later transitioning to a partially printed format with spaces left free for the individual information concerning the declaration of the newborn. The transition to this hybrid preprint/manuscript format varied from one commune to another. In Belfort, it occurred in 1885 and concerns $57.5$\% of the 39,627 declarations. The record contain crucial information, including the child's name, parent' names, witnesses, among other relevant data. Figure 3 provides a visual representation of a sample page from the civil registers, while Table 4 outlines the structure and content of an entry within the archive.
The archive is publicly accessible online until the year 1902 via the following link: https://archives.belfort.fr/search/form/e5a0c07e-9607-42b0-9772-f19d7bfa180e (accessed on 12 November 2023). Additionally, we have obtained permission from the municipal archives to access data up to the year 1919.
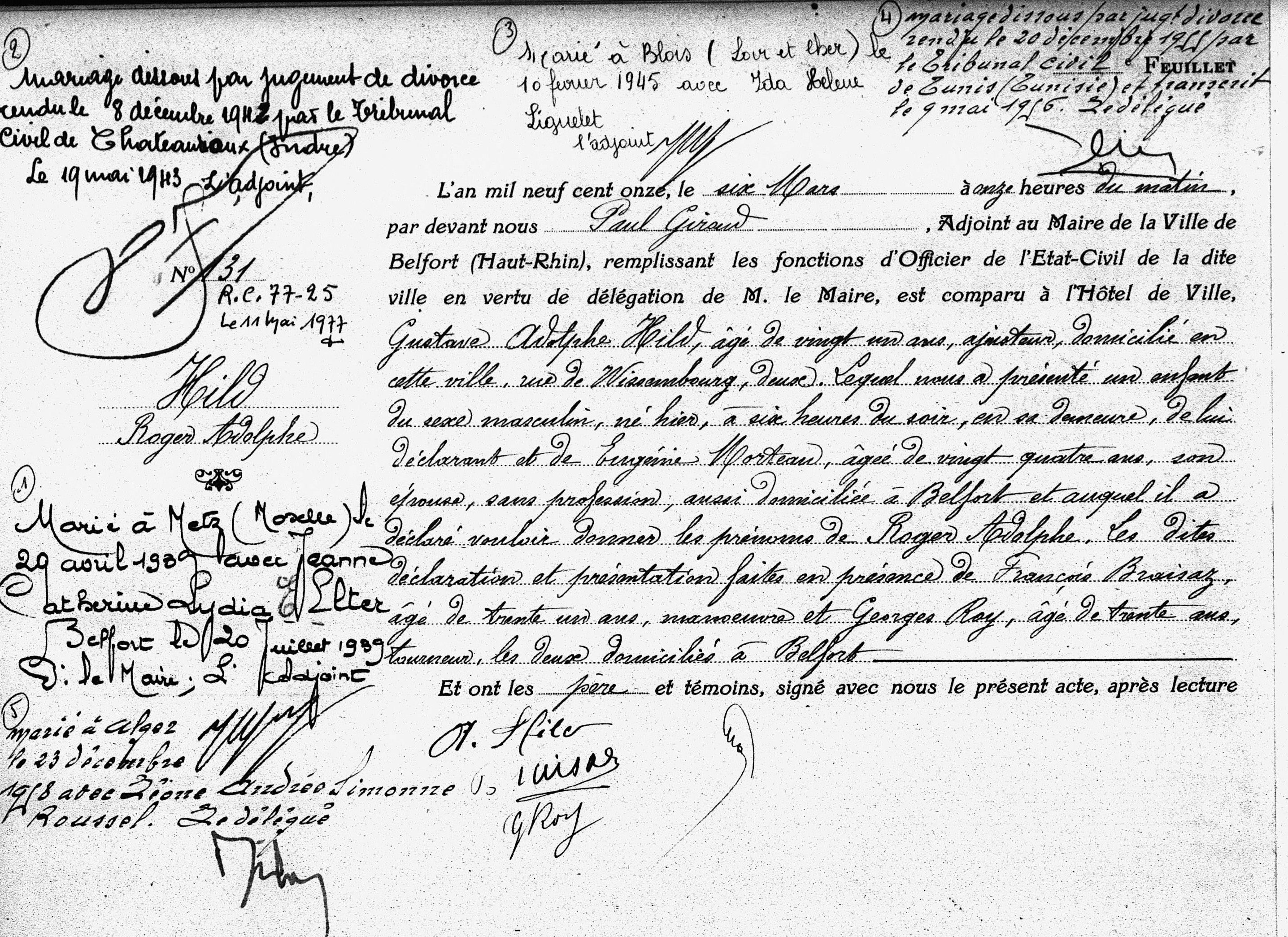
Figure 3. Sample of Belfort civil registers of births, featuring a hybrid mix of printed and handwritten text, along with marginal annotations.
Table 4. The structure of an entry in the Belfort civil registers of births
Structure |
Content |
Head margin |
Registration number.
First and last name of the person born.
|
Main text |
Time and date of declaration.
Surname, first name and position of the official registering.
Surname, first name, age, profession and address of declarant.
Sex of the newborn.
Time and date of birth.
First and last name of the father (if different of the declarant).
Surname, first name, status (married or other), profession (sometimes) and address (sometimes) of the mother.
Surnames of the newborn.
surnames, first names, ages, professions and addresses (city) of the 2 witnesses.
Mention of absence of signature or illiteracy of the declarant (very rarely).
|
Margins (annotations) |
Mention of official recognition of paternity/maternity (by father or/and mother): surname, name of the declarant, date of recognition (by marriage or declaration).
Mention of marriage: date of marriage, wedding location, surname and name of spouse.
Mention of divorce: date of divorce, divorce location.
Mention of death: date and place of death, date of the declaration of death.
|
6.1 Belfort Records Transcription Challenges
- Belfort records pose several challenges that complicate the transcription process of its entries, categorized into seven main areas:
Document layout: The Belfort registers of birth exhibit two document layouts. The first type consists of double pages with only one entire entry on each page, while the second type comprises double pages with two entire entries per page. Each entry within these layouts contains the information outlined in Table 4. However, there are some documents where entries begin on the first page and extend to the second page.
- Reading order: It is important to identify the reading order of text regions, including the main text and marginal annotation text within the entry.
- Hybrid format: Some of the registers consists of entries that includes printed and handwritten text, as shown in Figure 3.
- Marginal mentions: These mentions pertain to the individual born but are added after the birth, often in different writing styles and by means of scriptural tools that can be quite distinct. Moreover, they are placed in variable positions compared to the main text of the declaration.
- Text styles: The registers are written in different handwritten styles that consist of angular, spiky letters, varying character sizes, and ornate flourishes, resulting in overlapped word and text lines within the script.
- Skewness: Skewness refers to the misalignment of handwritten text caused by human writing. Many handwritten text lines in the main paragraphs and margins exhibit variations in text skew, including vertical text (90 degrees of rotation). Effective processes are needed to correct the skewness of the images for any degree of rotation.
- Degradation: The images exhibit text degradation caused by fading handwriting and page smudging (ink stains and yellowing of pages).
7. Results
We presents a comparison of state-of-the-art methods based on French RIMES dataset using Character Error Rate (CER) and word Error Rate (WER) metrics as reported in the publications. This dataset has emerged as a benchmark in the field of handwritten text recognition, many models have been evaluated using this dataset, making it a widely accepted and recognized standard for assessing the performance of such systems. Utilizing the RIMES dataset allows for meaningful and relevant comparisons, ensuring that our research facilitates more accurate assessments of systems performance and highlights the best approaches in this field. Figure 4 depicts the CER and WER of state-of-the-art methods at the line and paragraph levels.
Figure 4. State-of-the-art Character Error Rate (CER) and Word Error Rate (WER) across various studies applied to the French language at two different levels: text line and paragraph. (a) Shows the CER at the text line level, based on studies by Voigtlaender et al.~\cite{voigtlaender2016handwriting}, Puigcerver et al.~\cite{puigcerver2017multidimensional}, Bluche et al.~\cite{bluche2017gated}, Chowdhury et al.~\cite{chowdhury2018efficient}, Wick et al.~\cite{wick2022rescoring}, Pham et al.~\cite{pham2014dropout}, Doetsch et al.~\cite{doetsch2016bidirectional}, and Coquenet et al.~\cite{coquenet2022end}. (b) Depicts the CER at the paragraph level, as reported in studies by Bluche et al.~\cite{bluche2017gated}, Coquenet et al.~\cite{coquenet2021span, coquenet2022end,coquenet2023dan}. (c) and (d) Present the WER at the text line and paragraph levels, respectively, from the same studies.
Additionally, we evaluated the effectiveness of commercial systems in recognizing handwritten text in both English and French languages, three systems: Ocelus, Transkribus, and DOCSUMO were chosen to conduct this experiment, as they are among the most well-known and offer free trials for text recognition.
A text line image of Washington dataset was utilized for the English language, and a margin segment from the proposed Belfort civil registers of births was used for the French language. These experiments allowed us to compare and demonstrate the performance of these systems on the recognition of different international languages and various handwriting styles. Table 5 provides a detailed comparison of their performance. It is worth mentioning that evaluating such commercial systems at a documents level has resulted in improved accuracy rates due to differences in character and word counts.
Table 5. Accuracy comparison (%) of HTR commercial systems on French- and English-language datasets.
System |
RIMES |
Washington |
CER (%) |
WER (%) |
CER (%) |
WER (%) |
Ocelus |
15 |
53 |
2 |
14 |
Transkribus |
18 |
33 |
4 |
29 |
DOCSUMO |
11 |
33 |
2 |
14 |
8. conclusion
Handwritten text recognition systems have made significant progress in recent years, becoming increasingly accurate and reliable. In this study, we have presented several state-of-the-art models and achievements in offline handwritten text recognition across various international language documents. Additionally, we presented a comprehensive survey of French handwritten text recognition models specifically. The research papers were reviewed at four HTR levels: word, text line, paragraph, and page. Furthermore, we provided a summary of available public datasets for both French and other languages.
Despite significant achievements in recognizing modern handwritten text, there is still a need to extend these capabilities to historical text documents. Historical handwritten text recognition poses unique challenges, such as transcription cost, a variety of writing styles, abbreviations, symbols, and reproduction quality of historical documents.
We also observed that some commercial handwritten text recognition systems are performing exceptionally on handwritten text in English. In contrast, they are inaccurate in recognizing the French historical cursive handwritten text. Nevertheless, these systems could be a promise tool that can assist in automatically transcribing large volumes of historical documents with manual corrections. This is attributed to their advantages in automatic segmentation and dictionary support. Hence, decreasing time and cost.
Finally, we facilitate researchers in identifying the appropriate technique or datasets for further research on both modern and historical handwritten text documents. Furthermore, we conclude that there is a compelling need to design a new technique specifically tailored for transcribing the French Belfort civil registers of births.