Drug reinventing often arrives serendipitously from the surprising effects observed for an approved drug. As ‘chance favors only the prepared mind
[3], serendipity has produced significant advances in the history of medicine and selective optimization of side activities of drug molecules for generating new drugs
[4]. Examples of chemical drugs have been repurposed for benign prostatic hyperplasia, angina, sedation, nausea, and insomnia; later, they were repurposed for use in hair loss, erectile dysfunction, and leprosy, respectively
[5]. Examples of serendipitous discovery include sildenafil, intended for the treatment of hypertension and ended up as the most popular male erectile dysfunction treatment; dimethyl fumarate, developed to treat multiple sclerosis
[6], ended up treating psoriasis
[7]; or the antiviral drug remdesivir under testing to treat Ebola infection, ended up treating COVID-19
[8].
Beyond serendipity, itcan reinvent new drugs using technologies such as drug–target interactions (DTI). Artificial Intelligence (AI)-driven in silico tools significantly helps DTI mapping for drug reinvention. This technique has played a vital role in identifying potential therapeutics during the COVID-19 pandemic. A deep learning model trained on drug–target interaction (DTI), molecule transformer–drug–target interaction (MT-DTI), has uncovered alternate uses of available drugs: atazanavir and remdesivir efavirenz, ritonavir, and dolutegravir as inhibitors against SARS-CoV-2 protein
[9]. CATNIP, a machine learning (ML) model for drug repurposing, uses similarity data of the molecules based on their structure, target, and pathway information for drug reinvention
[10]. Besides identifying clinical targets, AI-based models can also identify adverse effects of therapeutics. For instance, chemical fingerprint data were used to develop a model which predicted that 22 FDA-approved drugs have potential contributions to heart failure. Later, experimental validation confirmed that 8 out of 22 anticipated therapeutics had cardioprotective activities
[11].
Adding a new indication is one of the fastest routes to reinventing therapeutic proteins because of the diversity of pharmacologic responses of therapeutic proteins; they need to be discovered. It is anticipated that new indications can be added to most approved therapeutic proteins, opening a vast treasure of therapies at a much-reduced development cost since the therapeutic protein’s safety is already established. Examples of therapeutic proteins that have received new indications recently include Actemra (tocilizumab), Adcetris (brentuximab vedotin), Dupixent (dupilumab), Enhertu (fam-trastuzumab deruxtecan-nxki), Eylea (aflibercept), Hadlima (adalimumab-bwwd), Imfinzi (durvalumab), Jemperli (dostarlimab-gxly), Kevzara (sarilumab), Keytruda (pembrolizumab), Libtayo (cemiplimab-rwlc), Takhzyro (lanadelumab-flyo), Tecentriq (atezolizumab), Tezspire (tezepelumab-ekko), Trodelvy (sacituzumab govitecan-hziy), and Trogarzo (ibalizumab-uiyk)
[13]. A biosimilar can also obtain a new indication if not protected by a patent, which significantly expands the drug’s utility.
2. Understanding Therapeutic Proteins
“Therapeutic protein” refers to recombinant DNA (rDNA) products that join DNA from different species and subsequently insert the hybrid DNA into a host cell, often a bacterium or mammalian cell, to express the target protein. UC San Francisco and Stanford researchers created this molecular chimera in 1972
[14]. Stanley Cohen of Stanford and Herbert Boyer of UCSF received the US patent in 1980. On 26 July 1974, ten researchers, including six future Nobel Laureates (James Watson, Paul Berg, Stanley Cohen, David Baltimore, Ronald Davis, and Daniel Nathans), wrote a letter in Science
[15] urging that the NIH regulate recombinant DNA technology.
The first rDNA product came in 1982 when the rDNA insulin was approved
[16]; now, hundreds of recombinant proteins are approved by regulatory agencies
[17]. Examples of this diverse class of compounds include interferons, cytokines, interleukins, thrombocytes, growth factors, coagulation factors, blood factors, anticoagulants, Fc fusion proteins, monoclonal antibodies, etc.
[18]. The global biologics market size is expected to reach around USD 719.94 billion by 2030, valued at USD 366.50 billion in 2021 and growing at a CAGR of 7.15% from 2022 to 2030. The current market of therapeutic proteins exceeds USD 380 billion
[19].
Therapeutic proteins replace a protein that is abnormal or deficient in a particular disease or augments the body’s supply of a beneficial protein to help reduce the impact of disease or chemotherapy. Genetically engineered proteins can closely resemble the natural proteins they replace or be enhanced by adding sugars or other molecules that extend the protein’s duration of activity.
For regulatory approval, the FDA treats alpha amino acid polymer with 40 or fewer amino acids as a peptide, not a protein
[20]. It is regulated as a drug under the FD&C Act rather than the Public Health Service (PHS) Act which controls biological drugs. Other definitions of peptide define the range of amino acids from 2 to 50
[21].
The unique properties of proteins arrive from the long chain of amino acids in therapeutic proteins that fold into a three-dimensional (3D) structure of domains that attach to receptors, resulting in pharmacological responses that can be extended to the toxicological response. In addition, proteins are, by nature, immunogenic, a property that can also be modulated by altering the structure.
The 3D structure of proteins defines their functions; specific domains interact with receptors, triggering pharmacological and toxicological responses. The biological assay reflects the known mechanism and thus serves as a link to clinical activity. Therefore, using relevant biological assay(s) of appropriate precision, accuracy, and sensitivity is essential to confirming no significant functional difference.
A key element of protein structure is the domain resulting from its stable structure that can fold and undergo folding without reference to the rest of the amino acid chain. Domains are not necessarily unique; the same gene can be found in many molecules. The binding domain binds to a specific atom or molecule, such as calcium or DNA. Proteins may have a conformational change as a result of binding. Many proteins depend on their binding domains to work correctly. They are necessary because they aid in the splicing, assembling, and translating proteins
[22] (
Figure 1).
Figure 1. Variable and constant antibody domains (Licensed from Shutterstock).
3. Reinvention Scope
Advancement in recombinant technology has enabled developers to fine-tune and increase the therapeutic potential of proteins by targeting their structure and function to enhance their disposition half-life, product yield, and purity
[23]. Modifying disposition kinetics is also an excellent opportunity to reduce the dosing frequency.
The current reinventing approaches are less serendipitous and more based on rational and systematic approaches; libraries of approved compounds are available from many commercial sources. In addition, several computational and high-content screening methods are currently used to discover new indications for existing molecules
[24]. When a hit emerges from a drug reinventing strategy, it can be taken directly into the last phases of clinical trials
[25].
4. Intellectual Property
A major hindrance in reinventing therapeutic proteins is their intellectual property protection for the gene that expresses the molecule. If a new indication is patented, it will be allowed once the gene patent expires. However, this bar is coming down fast as many therapeutic proteins are now off the patent
[26]. In addition, the intellectual property hurdles go beyond gene patents and include process-related patents that can extend the research work to remove any infringement
[27].
5. Artificial Intelligence (AI) and Machine Learning (ML)
ML uses algorithms that can recognize patterns within a data set that has been further classified. A subfield of ML is deep learning (DL), which engages artificial neural networks (ANNs). These comprise a set of “perceptrons”, interconnected sophisticated computing elements mimicking biological neurons with their electrical impulses in the brain
[28]. ANNs constitute a set of nodes, each receiving a separate input, ultimately converting them to single or multi-linked outputs using algorithms to solve problems. ANNs involve various types, including multilayer perceptron (MLP) networks, recurrent neural networks (RNNs), and convolutional neural networks (CNNs), which utilize either supervised or unsupervised training procedures
[29]. The MLP network provides pattern recognition, optimization aids, process identification, and controls based on training in a single direction to enable universal pattern classifications. RNNs are networks with a closed loop, capable of memorizing and storing information, such as Boltzmann constants and Hopfield networks
[30].
AI modeling can significantly reduce preclinical work. The prediction of the toxicity of any drug molecule is vital to avoid toxic effects, as predicted by LimTox, pkCSM, admetSAR, and Toxtree, which are available to help reduce the cost
[31]. Advanced AI-based approaches look for compounds’ similarities or project the compound’s toxicity based on input features. The Tox21 Data Challenge organized by the National Institutes of Health, Environmental Protection Agency (EPA), and US Food and Drug Administration (FDA) was an initiative to evaluate several computational techniques to forecast the toxicity of thousands of environmental compounds and drugs; an ML algorithm named DeepTox outperformed all methods by identifying static and dynamic features within the chemical descriptors of the molecules, such as molecular weight (MW) and Van der Waals volume. It could efficiently predict the toxicity of a molecule based on predefined 2500 toxicophore features
[32].
Developing novel inhibitors against discoidin domain receptor-1 (DDR1) within 46 days and cyclin-dependent kinase-20 (CDK20) as a potential anti-lung-cancer drug within 30 days through AI-driven models is remarkable proof of ‘intelligent’ drug discovery
[33][34]. AI-driven repurposing is many folds faster than the traditional method. The lock and key analogy (
Figure 2) demonstrates the main challenges for artificial intelligence (AI) in drug reinvention
[35]. In contrast to the conventional method of target search, AI-driven methods enable screening of a larger number of locks (targets) and enable testing of available keys (small molecules) through virtual screening in a shorter period. It enables discovery, development, optimization, reinventing, and in silico testing of the exact key for target molecules.
Figure 2. Lock and key analogy of challenges for AI in drug discovery
[35].
5.1. Structure Prediction
With major advancements in machine learning and AI, template-free protein structure prediction methods have also increased accuracy and reliability of structure prediction methods. Template-free AI models are trained on the sequence and structural data from openly available databases, i.e., UniProt, RCSB PDB, Uniclust [36], BFD [37], MGnify [38], etc. Highly accurate protein structure prediction tools independent of templates include AlphaFold2 [39], trTosetta [40][41], Robetta [42], RoseTTA Fold [43], ESMFold [44], and OmegaFold [45]. Each algorithm uses a different AI model to predict protein structures from amino acid sequences. For example, AlphaFold2 uses a deep neural network-based approach with over 200 million protein structures openly available in the AlphaFold2 database [46]. trRosetta uses transfer learning with pre-trained deep neural networks; the Robetta server combines ab initio and homology-based methods with machine learning algorithms.
5.2. Target Identification
In the on-target strategy, a new indication of the drug acting through the originally known target is explored since the mechanism of action is expected to retain the same therapeutic effects. In the off-target strategy, new drug uses are identified acting through an unanticipated target; in this case, the mechanism of action is not apparent. Docking and fingerprinting are standard methods.
The use of AI tools in drug–target identification has dramatically improved the efficiency of drug reinventions by enabling the concurrent screening of active compounds and predicting potential drug targets with greater accuracy. AI-based tools have revolutionized how pharmaceutical companies approach discoveries, significantly reducing the time, cost, error, and bias in finding new disease treatments.
5.3. Molecular Docking
Identifying structure, functional regions, interaction profiles, and immune system responses are crucial for the success of a therapeutic protein reaching the patients. Therefore, researchers have redirected their attention from conventional drug discovery methods to computational techniques to find new and effective therapeutic agents quickly.
Proteins interact with their receptors to initiate therapeutic effects and manipulate disease mechanisms. For years, fluorescence-based assays, isothermal titration calorimetry (ITC), surface plasmon resonance (SPR), NMR, and other methods have been used to study the binding patterns and thermodynamic effects of drug–target interactions. While highly relevant for characterizing interactions, they are time-consuming, expensive, and resource intensive. Using computational tools in molecular docking has expedited the drug discovery process exponentially, enabling repeated testing with the complexities of the classical method.
Structure-based drug discovery (SBDD) and ligand-based drug discovery (LBDD) both involve the identification of non-covalent interactions using molecular docking in the prediction of novel properties of therapeutic compounds following the lock-and-key hypothesis and induced-fit model
[47][48]. In addition, both rely on molecular docking to predict the binding affinity and specificity of small molecules and their targets.
Advancements in computational techniques have led to more precise identification and optimization of binding mode, binding affinity, binding pocket, and solvation effects on drug-target interactions.
5.4. Limitations
Despite its many advantages, the application of AI faces data challenges, such as the data’s scale, growth, diversity, and uncertainty. The data sets available for drug development in pharmaceutical companies can involve millions of compounds, and traditional ML tools might be unable to deal with these data types. The recent natural language-based AI tools, such as the GPT4, are anticipated to resolve some of these issues.
While the QSAR-based computational model can quickly predict large numbers of compounds or simple physicochemical parameters, such as log P or log D, predicting biological properties remains challenging. These limitations will be reduced when larger training sets, experimental validation, and more data error training are added in the future.
AI-based data analysis significantly reduces the burden of research and testing in the early discovery phase, as it can handle a large volume of data for profiling molecules. However, it does stand to replace efficacy testing in patients
[49] due to the safety issues that form the basis of the regulatory requirements. However, it does significantly decrease the work leading to clinical efficacy testing.
6. Structure Modifications
Optimization of the safety and efficacy of drug candidates is a critical step in the drug development process. One approach to optimizing a protein-based drug is to truncate it to enhance its selectivity, potency, and pharmacokinetic–pharmacodynamic properties. Truncation of proteins has been widely used to develop more effective therapeutics and has proven to be a successful strategy in improving bioavailability. Additionally, the optimization of drugs can be combined with reinventing drug strategies to identify new therapeutic uses for existing drugs.
Recently, anti-rheumatoid arthritis effects of native Staphylococcal protein (SpA), recombinant full-length SpA, and a truncated form of SpA were used in a comparative study along with Enbrel (commercial drug) to test reduction in several inflammatory cytokines (IL-8, IL-1β, TNF-α, and IL-6). The truncated SpA had a higher efficacy even when compared to Enbrel. Another study suggested that exogenous truncated inhibitor K562 protein (tIK) has the potential to act as a new therapeutic in patients with Enbrel resistance since their modes of action are contrary to each other
[50]. Furthermore, in vivo and silico analyses suggest that the truncated protein resulted in the exposure of the IgG-binding domain, which led to effective binding through an increased radius of gyration
[51].
7. Drug Conjugates
Chemotherapy damages healthy cells that can be protected by using antibody-drug conjugates (ADCs) that direct chemotherapy towards only cancer cells, making it safer
[52][53][54]. The ADCs deliver chemotherapy when a linker connected to a monoclonal antibody binds to a particular target expressed in cancer cells. After binding to the target (cancer protein or receptor), the ADC releases a cytotoxic drug into the cancer cell. “Fully human” monoclonal antibodies engineered to carry human antibody genes are an ideal delivery platform for ADCs. They are highly targeted and cell specific, have an extended circulating half-life, and offer minimal immunogenicity. The chemical “linkers” that combine the antibodies and cytotoxic drugs are highly stable to prevent cleaving (splitting) before the ADC enters the tumor. Anticancer drugs (or “payloads”) penetrate the tumor and cause cell death by damaging the DNA of cancer cells or preventing new cancer cells from forming and spreading (
Figure 3).
Figure 3. Drug-antibody conjugate design. By Bioconjugator—Own work, CC BY-SA 4.0,
https://commons.wikimedia.org/w/index.php?curid=58772304 (accessed on 10 April 2023).
8. Radioimmunoconjugates (RIC)
Radiation is an effective therapy for many tumor types. However, external beam radiation therapy is associated with many nonspecific side effects. Modern radiation techniques such as intensity-modulated and proton beam therapy have increased precision, delivered higher radiation dosages, and reduced toxicities to the surrounding tissues
[55] (
Figure 4).
Radioimmunotherapy (RIT) has been explored as cancer therapeutics for many decades
[56]. RIT utilizes antibodies directed at an antigen expressed on the tumor cell surface to deliver cytotoxic radionuclides that emit α or β particles to the tumor sites. After the radioimmunoconjugates (RICs) bind to the surface antigen on the tumor cells, the α or β particles emitted by the radionuclides induce DNA damage and trigger tumor cell apoptosis
[57]. RICs have been viewed mainly as a radiation delivery system to treat metastatic cancer unsuitable for an external beam approach. RICs aim to increase the radiation specificity and allow for the delivery of higher radiation dosages with fewer toxicities. However, the current understanding of tumor immunology suggests that RICs may be more than just a radiation delivery system and present a fertile field for reinventing therapeutic proteins.
Because of their high cytotoxic potential, RICs emitting α- or β-particles can be used for targeted cancer therapy. Cancer treatment using RICs requires careful consideration of the choice of radionuclides and their dosage. β-emitters have a deeper penetration range and a lower linear energy transfer than α-emitters, whereas α-particles can release high energy at a relatively shorter distance. However, while α-particles are more efficient in tumor cell eradication without causing much collateral damage, β-particles are currently most commonly used in radioimmunotherapy. Many β-emitters, such as
131I and
90Y, are commercially available and have established techniques for conjugating them to antibodies. For example,
90Y-ibritumomab tiuxetan and
131I-tositumomab are US FDA-approved RICs targeting CD20 for treating B-cell non-Hodgkin lymphoma
[58]. α-Emitters, on the other hand, are not widely commercially available, techniques for conjugating them to antibodies are not well-established, and pharmacokinetics and dosimetry of α-emitters need further investigation for clinical applications. Large-scale production of radionuclides, especially α-emitters, for clinical applications, requires a significant investment.
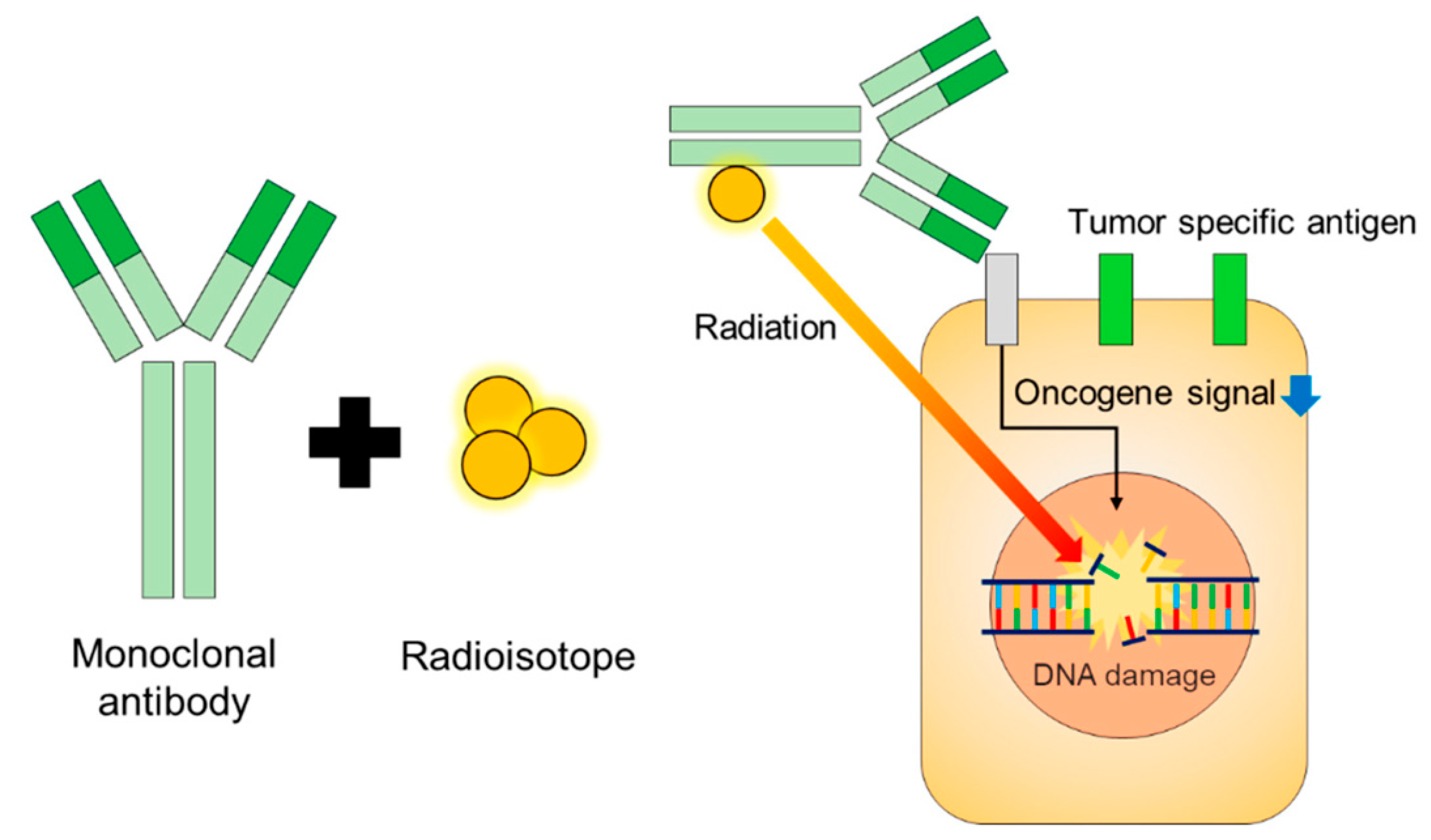
Figure 4. Design and function of radioimmune therapies
[59].
9. Regulatory Perspective
The success of reinventing therapeutic proteins depends significantly on how the regulatory agencies evaluate these products. Sometimes, these are a new class of drugs for which the agencies may need a guideline. In other cases, the agencies may be highly conservative, a mindset that is the responsibility of the developers to change by offering detailed educational discussion in the filing.
The critical reasons for the failure of new drug discoveries include inadequate efficacy or safety, lack of target validation, or inability to meet regulatory requirements. Although computational Drug Design has significantly reduced the chances of riskier drugs entering clinical trials and conserves resources, this should be emphasized in the regulatory filing with justification.
The FDA is leading the perspective of introducing new techniques in structure prediction, target identification, and interaction profiling to revolutionize drug development, setting the industry’s standard for precision and efficiency
[60].
Using AI, the FDA has developed models to classify and clinically monitor organ systems more prone to toxicity
[61] and is currently developing natural language processing algorithms to identify molecular targets associated with pediatric cancer.
10. Regulatory Submission
10.1. Nonclinical Testing
The testing becomes more subjective and less objective, making it a sound argument why a test with higher sensitivity should be reconfirmed with a lesser sensitivity test. Receptor binding remains the most robust and convincing test to demonstrate the safety and efficacy of therapeutic proteins. The receptor binding need not demonstrate a known pharmacodynamic marker, and the marker must correlate with the clinical response. This relationship forms the basis of the thesis that receptor binding alone can be used to substitute clinical efficacy testing; there is no need for the developers to investigate and find a pharmacodynamic marker either.
10.2. Pharmacokinetics–Pharmacodynamics
Another consideration that can significantly improve the PK/PD data is the inclusion criteria of the test subjects; choosing a narrow characteristic population regarding age, gender, BMI, ethnicity, and pharmacogenomics to antibody responses can significantly reduce the study size and add substantial validity to the data
[62].
The PK studies can further support the PD marker utility by extending the data analysis to demonstrate how fast and how much of the parenterally administered drug is leaving the central compartment, thus reaching out to receptor sites; this analysis will demonstrate a similarity in the onset of action. In addition, this property can be compared by adding a pharmacokinetic parameter, the rate of change of distribution volume as a function of time
[63], applied in several clinical efficacy comparisons based on clearance and tissue binding
[64].
Binding affinities to target antigens can significantly influence the PK of mAbs, requiring measurements of affinity or equilibrium dissociation constant (K
d), association rate constant (k
on), and dissociation rate constant (k
off). There is an optimal binding affinity beyond which the distribution of the mAb to target tissue may be impaired
[65][66]. This affinity is readily established by the characterization of binding to FcRn; as this is a pH-dependent interaction, binding affinity should be measured at pH 6.0 (where FcRn binds mAb in the acidic pH of the endosome) and pH 7.4 (physiological pH where FcRn releases mAb at the cell surface). High binding to FcRn at pH 6.0 and low binding at pH 7.4 is essential for low clearance of mAbs
[67][68].
10.3. Function Testing
Specialized cell-based bioassays or potency assays, including ELISA, binding assays, competitive assays, cell signaling, ligand binding, proliferation, and proliferation suppression, are essential in ascertaining the mechanism of action and similarity with the parent molecule. On the other hand, functional tests related to the possible MOA, such as apoptosis, complement-dependent cytotoxicity, antibody-dependent cellular phagocytosis, and antibody-dependent cellular cytotoxicity, among others, are necessary but not essential, especially when it is not relevant. For instance, functional tests (ADCC, ADCP, and CDC) are unnecessary for a product that predominantly targets a soluble antigen
[69][70][71][72][73].
Thus, comparable bioassay results should be sufficient when PD markers are unavailable, such as for mAbs. Therefore, a complete bioassay toolbox is a crucial enabler for applying the proposed clinical development paradigm. The toolbox requires multiple assays, ideally cell-based, to cover all relevant functions of a molecule with accurate and precise quantitative readouts and agreement with the regulators on the bioassay designs, including their validation
[74][75].
10.4. Immunogenic Response
Proteins are immunogenic and capable of producing neutralizing antibodies (NAbs) that bind to drug products and may diminish or eliminate the associated biological activity; these are unintended and undesirable outcomes. Standard immunoassays can detect drug-specific antibodies but cannot distinguish NAbs. Therefore, cell-based assays are often preferred because they closely mimic the mechanism by which NAbs and drug products interact in vivo. However, each cell-based NAb assay is unique and based on several factors, such as the drug product, study population, and development phase (preclinical or clinical). In addition, the type of NAb assay (direct or indirect) depends on the drug’s mechanism of action.