1. Introduction
Industry 5.0 (I5.0)
[1] is a subset of the larger concept of Society 5.0 (S5.0)
[2], which envisions a super-smart and intelligent human-centered society that leverages advanced technologies such as Artificial Intelligence (AI), the Internet of Things (IoT), robotics, and eXtended Reality (XR) for addressing a plethora of societal problems. Industry 5.0 specifically focuses on the application of these technologies in manufacturing and production systems to enable more efficient human–robot collaboration (HRC)
[3]. More specifically, I5.0 is an upcoming manufacturing concept that aims to improve collaboration between humans and robots by promoting the use of human–machine interfaces (HMI) in manufacturing systems and production networks
[4]. This involves utilizing advanced technologies such as cloud computing, 5G networks, Artificial Intelligence (AI), and digital twins (DTs) for the design of more robust decision making frameworks for engineers. Automation and robotics are key areas of focus in the development of smart factories, which are enabled by various information and communication technologies (ICTs), infrastructure, and control systems such as smart machinery, cyber-physical machine tools (CPMTs), robotics, and processes in factories
[5]. The concept of a Humachine, as has been mentioned, emphasizes the integration of humans and machines, leveraging the strengths of both to enhance their overall capabilities. To improve human–machine interaction (HMI), it is essential to understand the distinct characteristics and capabilities of each. Humans possess certain abilities that machines do not, such as creativity, intuition, empathy, and common sense. Humans can understand complex situations and respond to them appropriately, whereas machines can only respond based on pre-programmed instructions. Humans can also learn and adapt to new situations quickly, while machines require training and reprogramming. On the other hand, machines have some advantages over humans, such as processing speed, accuracy, and consistency. Machines can handle vast amounts of data and perform complex calculations much faster than humans. They are also not subject to human limitations such as fatigue, boredom, and emotional bias. Therefore, in order to address these challenges and improve HMI, new ways to combine the strengths of humans and machines have to be found. For example, machines can assist humans in performing repetitive and time-consuming tasks, freeing up time for humans to focus on more creative and strategic work. Machines can also analyze and process data, presenting it to humans in a way that is easy to understand and use .
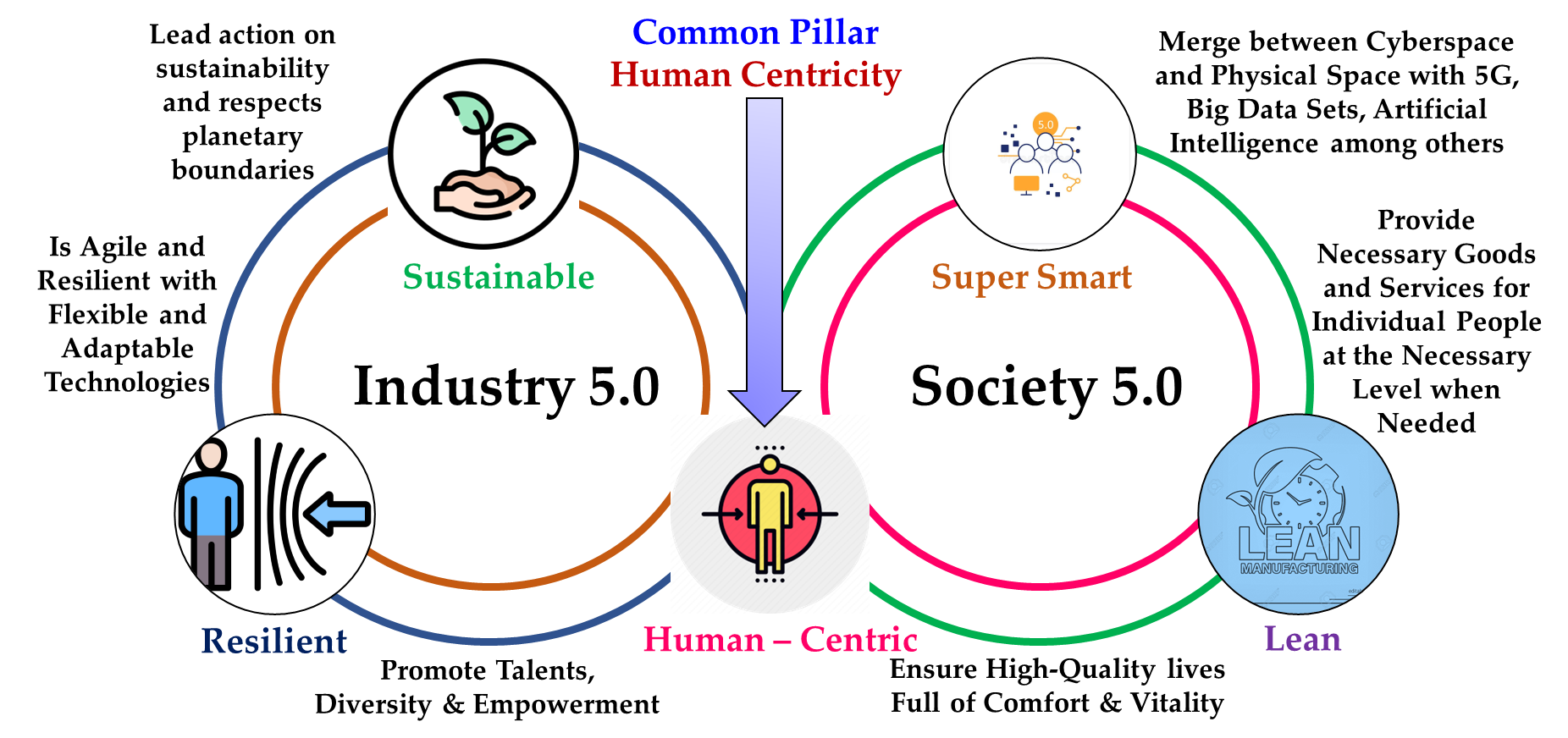
Figure 1. Vision of Industry 5.0 and Society 5.0 (Adapted from
[2]).
2. Human–Computer Interaction (HCI)
Key Milestones
Human–computer interaction (HCI) is the field of study that focuses on optimizing how users and computers interact by designing interactive computer interfaces that satisfy users’ needs. It is a multidisciplinary subject covering computer science, behavioral sciences, cognitive science, ergonomics, psychology, and design principles. The evolution of HCI can be traced back to the early days of computing when computers were large, complex machines that required specialized knowledge to operate. As computing technology became more advanced and accessible, HCI evolved to become more intuitive and user-friendly.
Figure 2. Human perception: context- and situationally dependent (adapted from
[6]).
3. Human–Machine Interaction (HMI)
Human–machine interaction (HMI) is a field of study that focuses on the design, development, and evaluation of interfaces between humans and machines. It is closely related to human–computer interaction (HCI) but includes interactions with a broader range of machines such as robots, autonomous vehicles, and smart home devices. HMI seeks to create interfaces that are intuitive, efficient, and enjoyable for users to interact with. This involves understanding user needs and preferences as well as the capabilities and limitations of machines. The goal of HMI is to create a seamless and natural interaction between humans and machines, improving the efficiency and effectiveness of the interaction. Human–computer interaction is of critical importance because it makes products more usable, safe, helpful, and functional. It creates a seamless and enjoyable user experience, rather than leaving the user frustrated as they try to figure out why the system is not working as they expect it to work and doing what they want it to do. It makes systems more intuitive, intelligible, and useful .
3.1. Key Enabling Technologies and Goals of HMI
Key enabling technologies of human–machine interaction (HMI) include :
Artificial Intelligence (AI) and Machine Learning (ML): these technologies enable machines to learn from data and adapt to user behavior, making interactions more personalized and efficient;
Natural language processing (NLP): NLP enables machines to understand and respond to human language, making interactions more natural and intuitive;
Robotics: robotics technology involves the design, construction, and operation of robots to perform tasks in a wide range of settings;
Computer vision: computer vision allows machines to perceive and interpret visual information, enabling them to recognize objects and understand gestures;
Haptic technology: haptic technology provides tactile feedback to users, enhancing the sensory experience of interacting with machines;
Augmented Reality (AR) and Virtual Reality (VR): AR and VR technologies enable users to interact with virtual objects and environments in real time;
Internet of Things (IoT): IoT technology involves connecting physical devices to the Internet, enabling them to exchange data and interact with each other.
The goals of HMI include:
Improve efficiency: the goal of HMI is to improve efficiency and productivity by automating routine tasks and augmenting human capabilities;
Improve user experience: HMI aims to create intuitive and user-friendly interfaces that make it easy for people to interact with machines;
Increase safety: HMI strives to improve safety by reducing the risk of accidents and errors in high-risk industries such as aviation, transportation, and healthcare;
Enable personalization: HMI enables machines to adapt to individual preferences and behavior, providing a personalized experience for each user;
Foster collaboration: HMI aims to foster collaboration between humans and machines, enabling them to work together to achieve common goals.
Additionally, in the Industry 4.0 era, we encountered a wide variety of human–technology interactions (HTIs); any time a human uses technology, there is some type of hardware and/or software involved that enables and supports interaction. HTI concentrates on the aspects in which technologies facilitate the interaction between the human and the environment. An important goal of HTI is to develop principles and algorithms for autonomous systems to enable safe, direct, effective, and trustworthy interaction with humans .
3.2. Augmented Intelligence
Augmented intelligence, also known as intelligence amplification, refers to the use of Machine Learning, Artificial Intelligence (AI), and other technologies to enhance human decision making and problem solving abilities. Augmented intelligence differs from Artificial Intelligence in that it seeks to augment, rather than replace, human intelligence. Natural language processing (NLP): NLP is a type of AI that enables computers to understand and respond to human language. NLP can be used to analyze large volumes of text data, such as customer feedback, and extract meaningful insights to help humans make better decisions. Predictive analytics: Predictive analytics uses Machine Learning algorithms to analyze data and predict future outcomes
[7]. For example, a retailer might use predictive analytics to forecast sales based on historical data and current trends. Virtual assistants: Virtual assistants, such as Siri or Alexa, use natural language processing and Machine Learning algorithms to help humans perform tasks more efficiently. For example, a virtual assistant might help a person schedule appointments, order groceries, or control smart home devices. Decision support systems: Decision support systems are computer programs that use data and algorithms to help humans make better decisions. For example, a healthcare provider might use a decision support system to help diagnose a patient’s condition based on symptoms and medical history. The goal of augmented intelligence is to help humans make better-informed decisions by providing them with relevant information, insights, and recommendations. By augmenting human intelligence with Machine Learning and AI technologies, humans can perform tasks more efficiently, accurately, and with greater insights, leading to better outcomes .
Prescriptive analytics and augmented intelligence are related concepts that both use Machine Learning and Artificial Intelligence (AI) to provide humans with insights and recommendations for decision making. Prescriptive analytics refers to the use of data, statistical algorithms, and Machine Learning techniques to identify the best course of action for a given situation. It involves analyzing data and identifying patterns to make predictions about future outcomes, then recommending a course of action based on those predictions. Augmented intelligence, on the other hand, refers to the use of AI and other technologies to enhance human decision making and problem-solving abilities. It seeks to augment, rather than replace, human intelligence by providing humans with relevant information, insights, and recommendations to help them make better-informed decisions. Prescriptive analytics is a type of augmented intelligence because it provides humans with specific recommendations for actions to take based on data and analysis. For example, a healthcare provider might use prescriptive analytics to recommend a treatment plan for a patient based on their medical history and current condition. The provider can then use their own judgment and expertise to make a final decision about the course of treatment . In summary, prescriptive analytics and augmented intelligence are both forms of Artificial Intelligence that aim to provide humans with insights and recommendations to improve decision making. Prescriptive analytics specifically provides specific recommendations based on data analysis, while augmented intelligence seeks to enhance human intelligence more broadly by providing relevant information and insights.
3.3. Brain Computer Interface (BCI)
A brain
–
computer interface (BCI) can be realized as a form of the so-called Humachine by enabling direct communication of the human brain and computer systems
. BCIs hold a lot of promise for enabling individuals to control devices and communicate directly with computers using their thoughts. Several approaches have been presented in the literature, with the most commonly applied framework being electroencephalography (EEG)
. It is often, however, that these technologies are coupled with other Industry 4.0 technologies, such as eXtended Reality (XR), in order to improve human perception. For example, in
, a Mixed Reality (MR) framework is proposed in order to facilitate remote user control of robotic arms. However, there are several challenges that need to be overcome to make BCIs more effective and reliable. Some of these challenges include:
-
Signal quality: One of the key challenges in the development of BCIs is obtaining high-quality signals from the human brain. By default, brain signals are weak and can be easily contaminated by noise and interference from other sources, such as muscles and other electronic devices. Therefore, accurate detection and interpretation of brain activity is a challenging task
[11];
-
-
Invasive vs. non-invasive BCIs: The current implementations of BCIs can be divided into two major categories: i) invasive and ii) non-invasive. Invasive BCIs require the implantation of electrodes directly into the brain, while non-invasive BCIs use external sensors to detect brain activity. Despite the fact that invasive BCIs can provide higher-quality signals, they are also riskier and more expensive. On the contrary, non-invasive BCIs are safer for humans and more accessible, at the expense of lower-quality signals
[12];
-
-
Training and calibration: BCIs require substantial effort in terms of training and calibration in order to work effectively. Furthermore, it is imperative for users to learn how to control their brain activity in such a way that can be detected and interpreted by the BCI. As a result, this can be a time-consuming and frustrating process for some users, causing discomfort
[13];
-
-
Limited bandwidth: BCIs often have limited bandwidth, thus allowing only a limited range of brain activity to be detected and interpreted. Therefore, the types of actions that can be controlled using a BCI are still limited
[14];
-
-
Ethical and privacy concerns: Indeed, BCIs have been evidenced to be really useful for the future of human–computer interfaces. However, there are certain ethical and privacy concerns. For example, data ownership legislation needs to be established. Furthermore, issues regarding data misuse and the development of suitable mechanisms to counteract such issues need to be explored
[15].
Figure 3. Comparison between HCI, HMI, and HTI (adapted from [16]).
Figure 3. Comparison between HCI, HMI, and HTI (adapted from [16]).
4. Human–Centric Manufacturing (HCM)
Human–centric manufacturing (HCM) is an approach to manufacturing that places the human operator at the center of the manufacturing process. It aims to create a work environment that is safe, healthy, and comfortable for workers, while also optimizing manufacturing efficiency and productivity. Human–machine interaction (HMI) is a key component of HCM, as it is essential for creating interfaces between humans and machines that are intuitive and easy to use. HMI plays a critical role in HCM by enabling workers to interact with machines in a way that is natural and efficient. This involves designing interfaces that are intuitive and user-friendly while also providing feedback to the user to help them understand the status of the machine and the manufacturing process. Collaborative intelligence is enabled by empathic understanding between humans and machines . By creating interfaces that are intuitive and user-friendly, HMI can help create a manufacturing environment that is safe, healthy, and comfortable for workers, while also optimizing manufacturing efficiency and productivity (Figure 4).
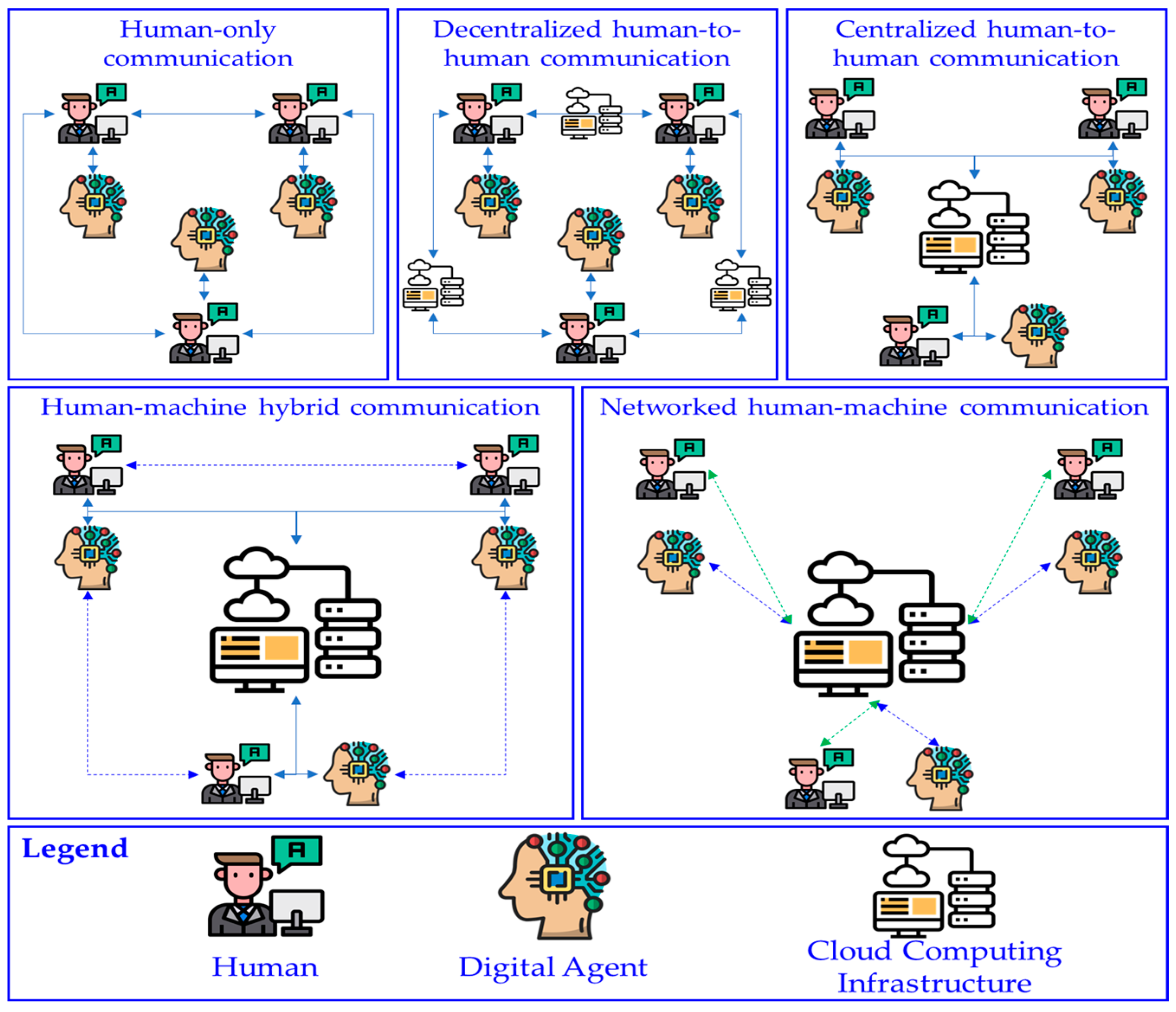
Figure 4. Types of HCPS communications.
5. Human Digital Twins
A human digital twin is a virtual representation of a real human being that is created using digital data. It is a model that replicates the physiological, biological, and behavioral characteristics of an individual, allowing for simulations and predictions of their responses to different stimuli, situations, or environments. Here are some of the key characteristics of a human digital twin (Figure 5):
1) Real time data
2) Multi-dimensional representation
3) Personalization
4) Machine Learning and AI
5) Simulation and Prediction
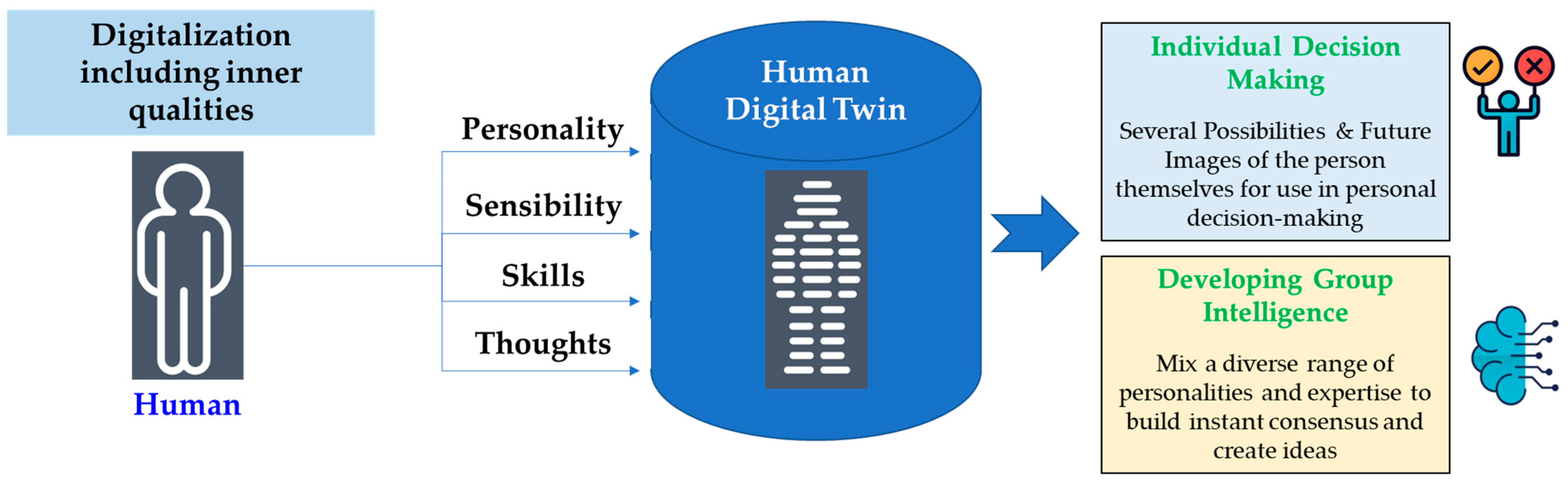
Figure 5. Human digital twins.
6. Discussion
6.1. Humachine Framework
Humachines are necessary for the future because they can bring significant benefits and opportunities in various domains, including:
-
Enhanced productivity and efficiency: humachines can augment human capabilities with the speed, accuracy, and consistency of machines, leading to higher productivity and efficiency in many industries;
-
Improved decision making: combining human reasoning and intuition with Machine Learning algorithms can lead to better decision making, reducing errors and improving outcomes;
-
Advanced healthcare: Humachines can help healthcare professionals in diagnoses, treatment planning, and monitoring, leading to more accurate and personalized healthcare;
-
Innovation and creativity: by collaborating with machines, humans can access vast amounts of data, tools, and insights that can fuel innovation and creativity in various fields;
-
Automation of mundane tasks: automation of repetitive and mundane tasks can free up human time and energy to focus on more meaningful and creative tasks, leading to higher job satisfaction and engagement.
In summary, the Humachine concept provides a vision of a future where machines and humans collaborate to solve complex problems and create new opportunities. This partnership between humans and machines can create a world where we work smarter, not harder, where we can achieve more together than we can alone. A conceptual framework highlighting the necessity of Humachines is presented in
Figure 6.
Figure 6. Humachine intelligence conceptual framework.
7. Conclusions and Outlook
Human–machine interaction (HMI) is a crucial aspect of Society 5.0, in which technology is leveraged for solving social challenges and improving quality of life. The key objective of HMI is to create a harmonious relationship between humans and machines where they work together towards a common goal. This is achieved by focusing on the strengths of each component, with machines handling tasks that require speed and accuracy while humans focus on tasks that require creativity, critical thinking, and empathy. One of the primary challenges in achieving effective HMI is ensuring that machines are designed to be user-friendly, transparent, and accessible. To achieve this, designers must take into account the diverse needs of users, including those with disabilities and elderly populations. Additionally, ethical considerations, such as privacy protection, must be taken into account. Another critical aspect of HMI in Society 5.0 is the development of human digital twins, which are virtual representations of humans that can be used for simulations and predictive analysis. Human digital twins have the potential to revolutionize healthcare, education, and other fields by enabling more accurate and personalized interventions. To fully realize the potential of HMI in Society 5.0, collaboration and cooperation across multiple fields, including technology, social sciences, and humanities, is required. This will require a multidisciplinary approach that prioritizes diversity and inclusivity. In conclusion, HMI is a vital component of Society 5.0, enabling technology to be harnessed for social good. While challenges remain, including ethical considerations and the development of accessible and user-friendly interfaces, the potential benefits of HMI are vast, and a collaborative approach is key to realizing them. The key takeaway from the presented research work is to raise awareness on the integration of machines with humans in order to realize what has been defined as the Humachine. It has to be stressed that machines should be not treated as threats or competitors to humankind. On the contrary, it is suggested to treat them as a set of useful tools in order to vastly expand our capabilities and mitigate the limitations of humankind as we know them. Undeniably, in the long term, the replacement of human operators and workers might be more financially profitable. However, it is more ethical for society to provide certain tools to augment professionals rather than replacing them. The misuse of Artificial Intelligence methods is capable of and has already caused major problems. Decisions that may affect people should be made by people.