Computer-vision-based target tracking can be applied to structural vibration monitoring, but current target tracking methods suffer from noise in digital image processing. In this paper, aA new target-tracking method based on the sparse optical flow technique is introduced to improve the accuracy in tracking the target, especially when the target has a large displacement. The proposed method utilizes the ORB technique to maintain a variety of keypoints and combines the multi-level strategy with a sparse optical flow algorithm to search the keypoints with a large motion vector for tracking. Then, an outlier removal method based on Hamming distance and interquartile range (IQR) score is introduced to minimize the error. The proposed target tracking method is verified through a lab experiment---a three-story shear building structure subjected to various harmonic excitations. It is compared with existing sparse optical flow-based target tracking methods and target tracking methods based on three other types of techniques, i.e., feature matching, dense optical flow, and template matching. The results show that the performance of target tracking is greatly improved through the use of a multi-level strategy and the proposed outlier removal method. The proposed sparse optical flow-based target tracking method achieves the best accuracy compared to other existing target tracking methods.
1. Proposed Target Tracking Method
In this sentudry, a novel sparse-optical-flow-based target tracking approach for structural vibration monitoring is proposed. As shown in Figure 1, the conventional sparse optical flow algorithm (i.e., LK) is enhanced to track a set of sparse keypoints accurately. A multi-level strategy is applied to the LK algorithm to enhance the large motion vector calculation. Moreover, Oriented Fast and Rotated Brief (ORB), a corner extraction algorithm, is used to detect the keypoints, and an outlier removal method based on Hamming distance and interquartile range (IQR) score is introduced to minimize the error between the experimental response versus the vision-based response.
Figure 1. Flowchart of the proposed sparse-optical-flow-based target tracking method. ORB: Oriented Fast and Rotated Brief, MLK: multi-level Lucas–Kanade algorithm, IQR: interquartile range.
2. Vision-Based Sensing System
The visual sensing system used for structural vibration monitoring is based on target tracking techniques. As shown in
Figure 2, this system takes the video frames that record the vibration of a structure as an input and outputs the acceleration time histories of the structural vibration. It consists of two components: (i) camera calibration and scale conversion; and (ii) frame tracking strategies and displacement calculation. To save on computational resources, an ROI is defined in the first frame.
Figure 2. Flowchart of the visual sensing system.
3. Experiments Setup
The overview of the experimental setup is shown in Figure 3. A three-story shear building structure (see Figure 4) is fixed on the shake table and subjected to harmonic loads excitation using an excitation system. A reference system measures the acceleration time series response for the vibration of each floor under different excitation frequencies. A vision sensor system records the structural vibration for acceleration calculation.
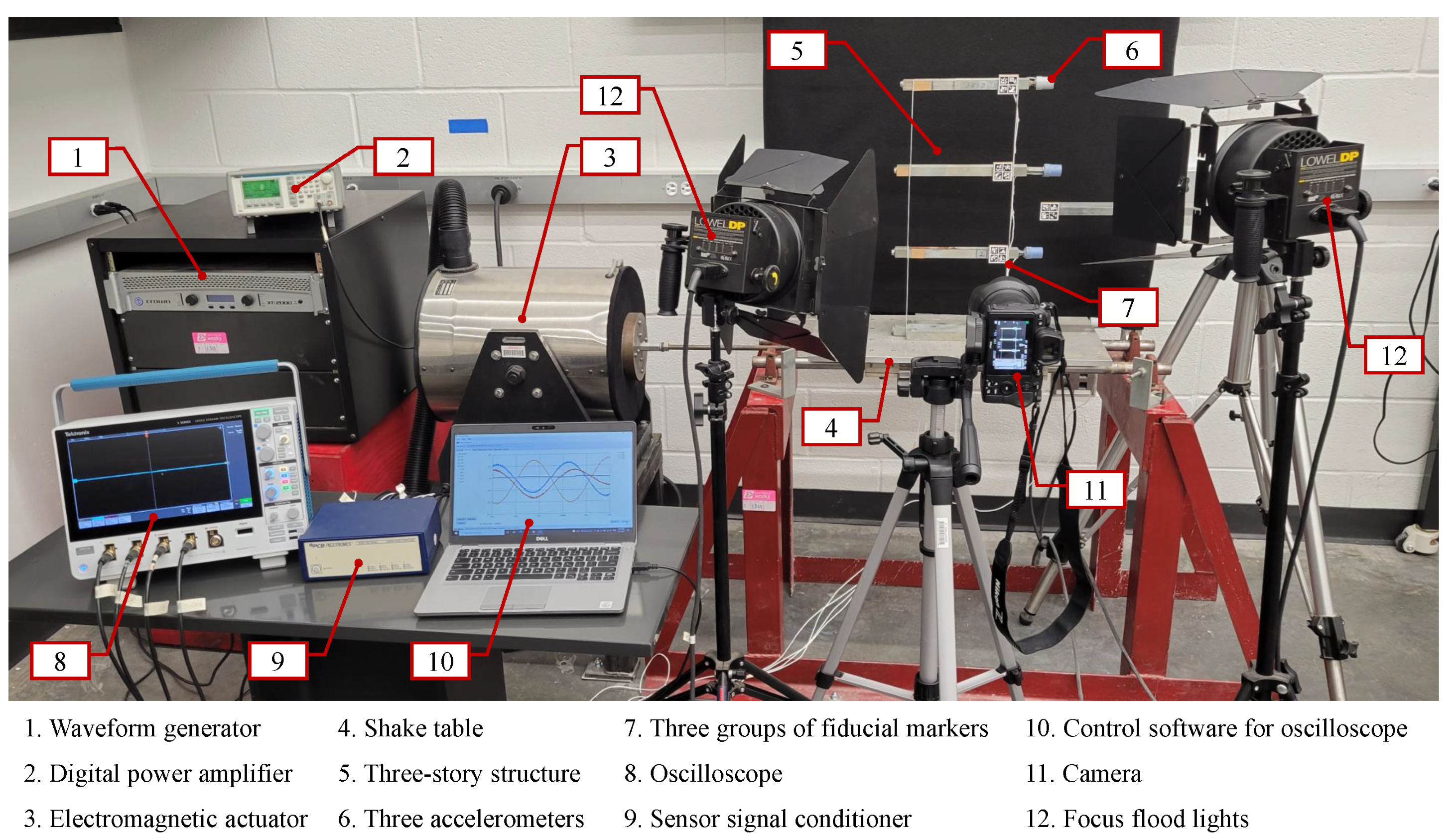
Figure 3.
Overview of the experimental setup.
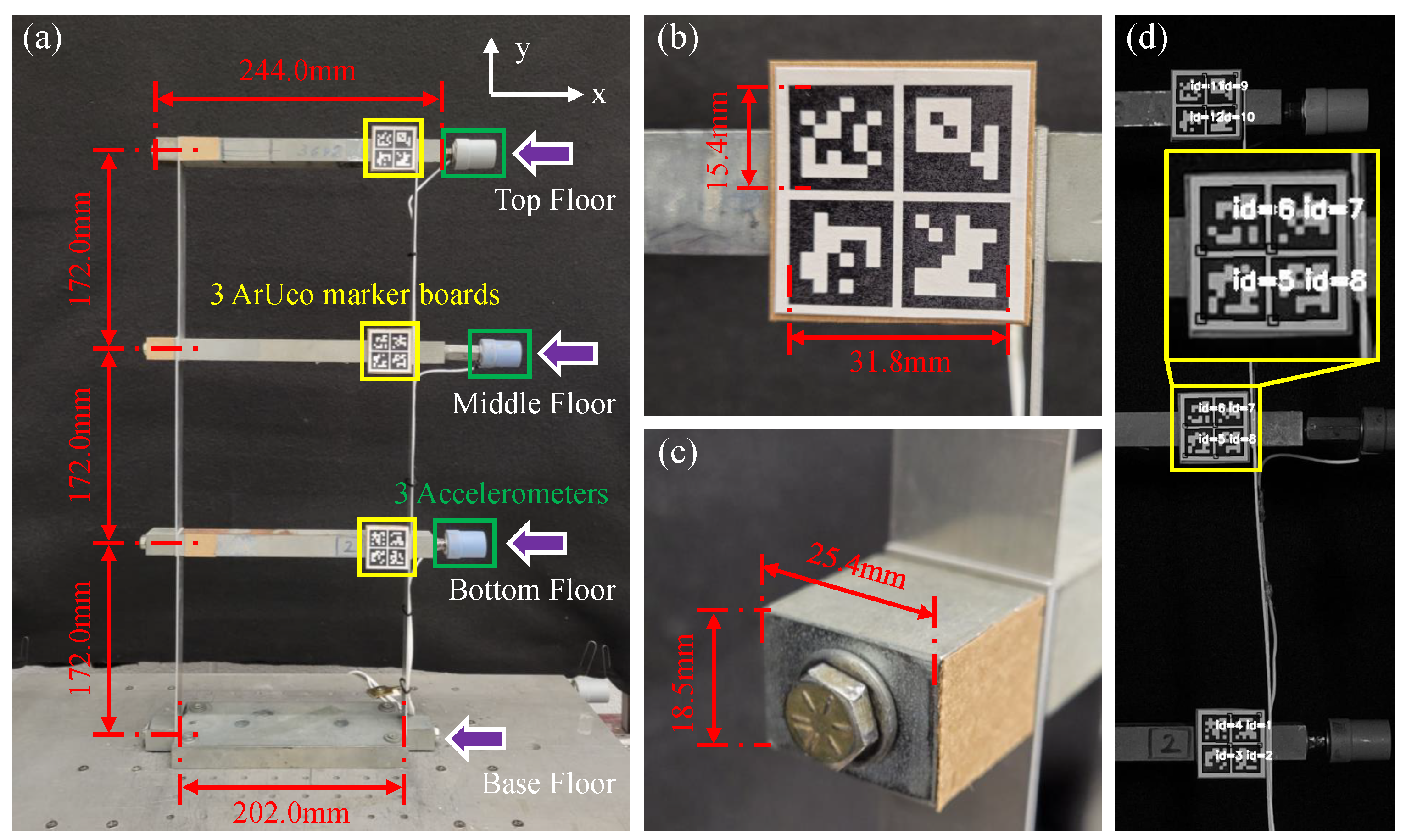
Figure 4. Experimental three-story shear building structure. (
a) Overview of the structure; (
b) close-up shot of the ArUco marker board fixed on the top floor; (
c) left end of the lumped mass steel stick of the middle floor; (
d) detected ids of the fiducial markers.
4. Qualitative and Quantitative Assessment of the Proposed Method
The proposed vision-based target tracking method for structural vibration monitoring is evaluated qualitatively and quantitatively through the laboratory-scale experiment.
The proposed target tracking method is implemented by carrying out three key steps: (i) sparse optical flow calculation, (ii) Hamming distance-based outlier removal method, and (iii) IQR score-based outlier removal method.
Figure 5 shows two instances of the frame
(It, It+1) to compare the effects of three steps qualitatively. The colored circles in
Figure 5 represent the keypoints 2D position:
(xi, yi) detected by the ORB detector in frame
It and the optical flow algorithm in frame
It+1, and each of them is a local extremum whose pixel intensity is greater or smaller than all its neighbors. Images in
Figure 5a show the pairs of matched keypoints in the ROIs of the previous frame,
It, and the current frame,
It+1, after implementing all three steps. The colored lines connect the matched keypoints in the ROI of
It and their corresponding keypoints in
It+1. Images in
Figure 5b show the motion trajectories (green lines) for the matched keypoints (greens dots) in the ROI of
It from time
t to
t+1. The matched keypoints shown in
Figure 5(a-iii) are much more distinct and have greater clarity compared to the keypoints observed in
Figure 5(a-i). As seen in
Figure 6, the response obtained from the proposed method matches closely with the measured response.
Figure 5. Qualitative example of the sparse-optical-flow-based target tracking. Pairs of matched keypoints in the ROIs of the previous frame, It, and the current frame, It+1, after implementing (a-i) sparse optical flow calculation, (a-ii) Hamming distance-based outlier removal method, and (a-iii) IQR score-based outlier removal method. Motion trajectories for the matched keypoints in the ROI of It from time t to t+1, after implementing (b-i) sparse optical flow calculation, (b-ii) Hamming distance-based outlier removal method, and (b-iii) IQR score-based outlier removal method.
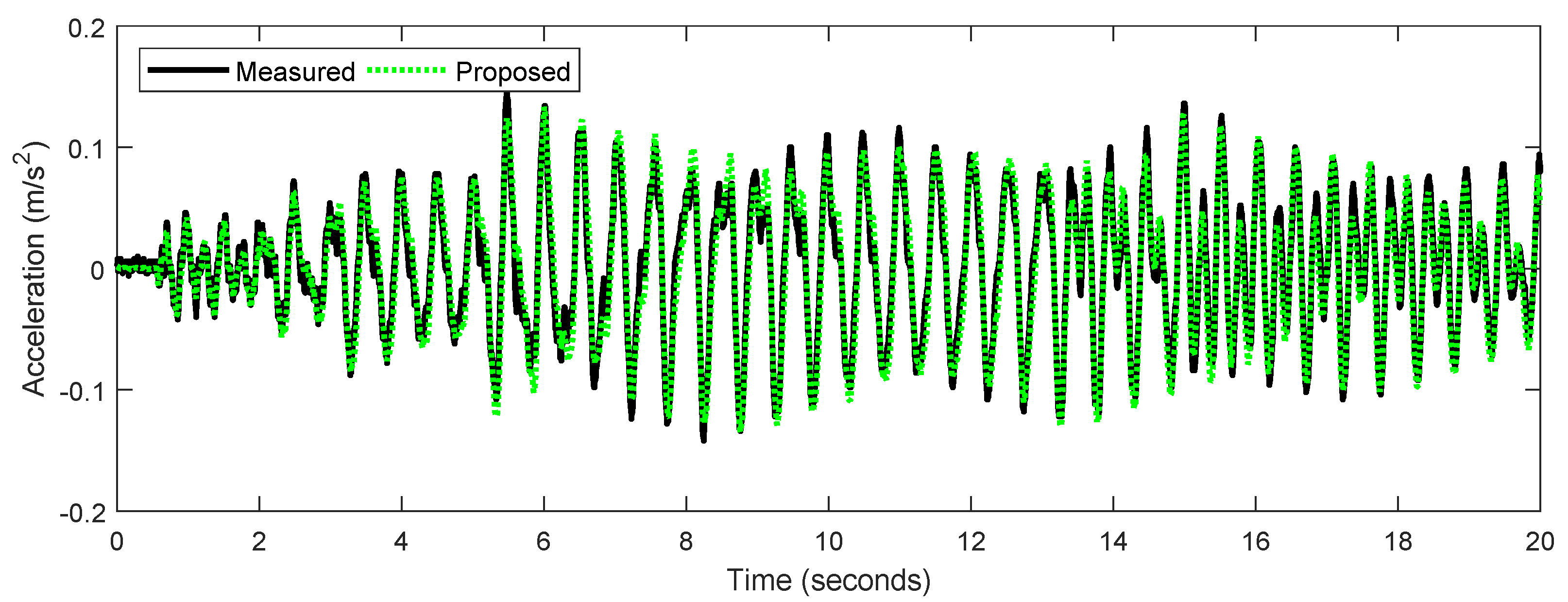
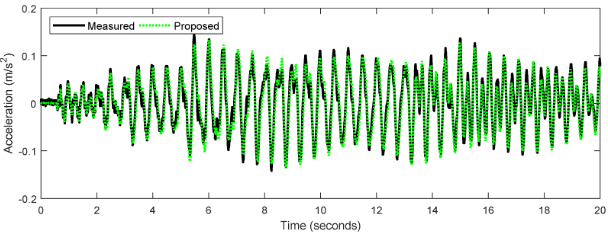
Figure 6. Comparison of middle floor acceleration response from the accelerometer and the proposed method at 2 Hz.
The proposed method is compared with existing sparse-optical-flow-based target tracking methods and target tracking methods based on three other types of techniques, i.e., feature matching, dense optical flow, and template matching. The results show that the performance of target tracking is greatly improved through the use of a multi-level strategy and the proposed outlier removal method. The proposed sparse-optical-flow-based target tracking method achieves the best accuracy compared to other existing target tracking methods.