1. Ligands for Classical Detection Techniques Improvement
A ligand is a molecule that reversibly binds to a targeted macromolecule, protein, or nucleic acid. Ligands are extensively used for pathogen detection. By binding specifically to microorganisms, they can be used to obtain a specific and easy to detect signal. They can also be used to isolate and concentrate pathogens, easing their detection. In both cases, the sensitivity of the method associated is increased, allowing an earlier detection.
1.1. Broad-Spectrum Ligand
The choice of the appropriate ligand is an important issue in detection methods
[40][1]. A multitude of ligands seems potentially useful for the detection of bacteria, such as phage, aptamers, anti-microbial peptides, or antibodies (
Figure 1). Ligands can be used for both detection and identification. A ligand is usually very specific to a bacterial species. As a result, general detection requires numerous ligands at the risk of increasing the analysis time and cost. A ligand that can bind to several bacterial species or genera is thus more relevant for detection and appears as an objective to achieve. A search for ligands with a broader recognition spectrum was therefore undertaken. Their role would be to interact with several potential microorganisms responsible for the contamination of samples and to allow the detection of any bacterial species. This is however challenging because there is a trade-off between the affinity of the ligand, and its ability to bind to a broad spectrum of bacteria.
Figure 1. Schematic representation of the binding of bacteria to a surface that can be coated (or functionalized) with different molecules acting as ligands such as antibodies, bacteriophages, antimicrobial peptides (AMPs), or DNAzyme.
Antibodies are the more widely used ligands and can be good candidates. Antibodies form immune complexes with antigens. The long-standing use of antibodies provides an extended literature on their engineering and use for bacterial detection. Moreover, a large variety of antibodies recognizing a wide range of antigens is commercially available. However, the specificity of the antibodies makes it difficult to detect a large number of different bacterial species simultaneously. Although some antibodies are capable of recognizing conserved epitopes in many bacteria, in practice, due to bacterial proteolytic capabilities, these antibodies have not proven conclusive for broad-spectrum detection
[40][1].
Bacteriophages, or phages, are also interesting ligands. Phages are viruses that specifically infect bacteria. They are abundant on Earth and are involved in the regulation of bacterial species. Using a phage as a ligand makes it possible to detect, fix, and eliminate bacteria. The recognition of bacteria by bacteriophages is performed via binding proteins with strong affinities to structures on the surface of the bacterial host. Modifications of these proteins can allow biotechnological adaptation to specific detection requirements
[40,41][1][2]. Whole bacteriophages or fragments of phage proteins can be grafted onto biosensing surfaces. In addition, the size of whole bacteriophages limits the sensitivity of some detection methods. While there is a growing interest in phages as ligands
[22,42][3][4], it is mainly used for identification application as the binding is very specific to the species of the bacteria.
Enzymes are another example of molecules used for recognition. They are catalytic proteins that facilitate certain chemical reactions. They can be used for detection, taking advantage of their highly specific affinity for their substrates. In the case of pathogens, enzymes directed to specific proteins have been used for detection
[43][5]. Upon recognition of the target, enzyme activity can be detected by optical (fluorescence, colorimetry) or electrochemical means. Redox enzymes have been widely used in biosensors as their redox activity can be monitored relatively easily with electrodes
[44][6]. The specificity panel of the different enzymes makes them suitable for detection of broad-spectrum detection (esterases) or of single species of bacteria (bacterial enzyme considered as one of their virulent factor), and in specific cases for identification. While enzymes can be used as receptors, they are more frequently used to produce a signal after the binding to another type of receptor
[45][7], as shown in the examples provided in the following sections.
The fabrication of ligands is largely inspired by nature, but synthetic ligands are gradually emerging. The performance of these alternative ligands is often compared with that of antibodies, which remain a reference probe. Technologies using aptamers and Anti-Microbial Peptides (AMPs) are increasingly emerging (Figure 1). In the following section these two new ligands and the technological advances concerning them are developed.
1.2. The Most Promising Ligands
Aptamer and DNAzyme
Aptamers are short oligonucleotide sequences with a specific affinity for various targets: peptides, proteins, even whole cells, bacteria, or viruses
[46][8]. They have received increasing attention in the last two decades as a complement or alternative to antibodies. Aptamers are cheaper and easier to produce than antibodies and can be more easily dried for storage. They can also be selected against small very small molecules (MW > 1000 g.mol
−1)
[47][9] or toxins
[48][10].
Aptamers can be isolated by in vitro selection from a random-sequence DNA pool
[49][11], using the systematic evolution of ligands by exponential enrichment (SELEX) method
[50][12]. The SELEX method is an in vitro screening method to determine binding affinities of molecules, peptides, or proteins to DNA or RNA strands. For the detection of pathogenic bacteria, aptamers specific to different genus have already been isolated:
Vibrio, Staphylococcus, Streptococcus, Haemophilus, or
Escherichia [51][13]. Detection methods classically used with antibodies are also practicable with aptamers
[52][14] such as fluorescence, optical, or electrochemical
[53][15] methods.
Recently, a detection method more specific to aptamers emerged, RNA-cleaving fluorescent DNAzyme (RFD)
[54,55,56][16][17][18]. The principle of operation of RFDs is illustrated in
Figure 2. DNAzymes are a special class of functional nucleic acids that are artificial single-stranded DNA molecules with catalytic abilities. RFD combines a DNAzyme with a pair of donor and acceptor fluorophores or a fluorophore and a quencher, which emits a fluorescent signal upon activation of the DNAzyme. Coupled with an aptamer, the inactive form of the RFD is allosterically converted to an active form upon interaction with the target molecule. Ali et al. demonstrated the detection of
Escherichia coli at a single-cell sensitivity
[57][19]. Kang et al. further elaborated the method by coupling RFD with droplet microencapsulation and 3D particle counter system
[58][20]. The final system detects
E. coli cells in blood with a concentration as low as 1 bacterium per mL.
Figure 2. Representation of RFD-based sensor. The targets produced by the bacterium bind to the inactive DNAzyme sequence, which undergoes a conformational change to activate the DNAzyme. Then, activated DNAzyme catalyzes the cleavage of the fluorogenic substrate at the ribonucleotide junction (R), leading to the separation of the fluorophore (F) and the quencher (Q) to produce a fluorescence signal.
Some aptamers can also be used after being split into two or three fragments. In the presence of a specific target, they can assemble and activate or inhibit a fluorescent signal. In comparison to whole aptamers, split ones are easier to synthesize, and less prone to form unwanted secondary structures, leading to a false-positive
[59][21].
In conclusion, aptamers represent a new class of ligand that can target new elements in bacteria with a cheaper cost of production. They are increasingly used in biosensors as new aptamers are regularly synthesized. To detect a large spectrum of bacteria, it would be interesting to isolate aptamers specific to common elements in Gram-positive or Gram-negative cells. For example, this is what Shin et al. undertook with aptamers targeted against outer membrane vesicles of Gram-negative bacteria
[60][22].
Antimicrobial Peptides
Antimicrobial peptides (AMPs) are another promising possibility for broad-spectrum ligands. AMPs are short (usually less than fifty amino acids) and natural peptides found in most living animals, both eukaryotic and prokaryotic organisms. They are powerful broad-spectrum antibiotics. They can destabilize biological membranes to form trans-membrane channels. In addition, they are effective against biofilms, where antibiotics are often ineffective
[61,62,63][23][24][25].
Apart from antibiotic applications, their strong affinity to bacterial membranes makes them interesting ligands for biosensing applications
[64,65][26][27]. Through molecular engineering, the bactericidal portion of the AMP can be removed, allowing the bacteria to bind without being destroyed so that a signal can be produced for later detection. Furthermore, AMPs are highly stable in storage and relatively easy and cheap to produce
[66][28]. Surprisingly, their use for biosensors is only recent. So far, several examples of surface-immobilized AMPs used for detection have been described in the literature
[67,68][29][30]. Lillehoj et al. devised a microfluid chip for multiplexed detection of bacterial using AMPs
[69][31]. Demonstrated on
Streptococcus and
Pseudomonas, bacteria were detected at minimum concentrations of 10
5 CFU/mL within 25 min, due to electrical detection. Using an AMP called Nisin and an impedimetric detection, Malvano et al. successfully detected
Salmonella with an LOD of 15 CFU/mL with a 30 min incubation period
[70][32]. This represents a significant improvement in the detection limit compared with
[69][31].
The broad-spectrum affinity of AMPs can enable biosensors to detect multiple bacteria strains at once. To have an exhaustive detection, few AMPs are sufficient instead of a panel of antibodies that are more specific to a bacterial genus. It would be interesting to evaluate the effectiveness of AMPs inside blood or other complex environment and evaluate the possibility of profiling bacteria with only a small set of AMPs.
2. Improvements and Developments in Analytical Methods Requiring Sampling
2.1. Paper Sensors
Paper sensors are strips of paper on which reagents, molecules, or ligands, can be grafted to make sensors. They can be used to detect the presence or absence of an analyte and can be useful in some diagnoses. The best-known example of the use of paper sensors is the pregnancy test. Paper sensors were initially intended for use in the field in developing countries where the installation of expensive and cumbersome equipment is impossible. However, their many advantages make them good laboratory tools too. With their low cost, ease of use, and portability (not very cumbersome and heavy) it becomes possible to multiply sampling for pathogen detection. During an industrial process using cell bioreactors or in the manufacturing of innovative therapy drugs, one can now easily imagine more controls at all critical steps of the process. Moreover, paper sensors can be integrated in a more complex device in order to automate the task by a robot and allow a quick reading of the result afterwards. Indeed, sampling takes time, it requires an operator and can lead to contamination. These constraints make it impossible to repeat the controls while they should be regular and representative of the whole sample. With the help of an automaton and paper sensors, these problems can be solved, in particular the problem of sterility breakage by the operator. Several techniques are possible, and several different ligands can be used with paper sensors.
Paper sensors are affordable devices for food
[71,72,73][33][34][35] or environmental quality control
[74][36]. They can also be used for the detection of pathogens or the rapid and accurate detection of diseases
[75,76,77][37][38][39]. Their versatility makes them useful for virus diagnosis
[78][40] or detection of bacterial spores
[79][41].
Hossain et al. reported an approach for the selective and sensitive multiplexed detection of E. coli using a lab-on-paper test strip based on the activity of an intracellular enzyme. The objective is to trigger a rapid enzymatic hydrolysis of the substrate, causing a color change from colorless to blue or from yellow to red depending on the bacteria. With a step of preconcentration using immunomagnetic nanoparticles, the detection limit was about 10 CFU/mL for E. coli in 30 min and without cell culture. Thus, these paper test strips may be suitable for the detection of viable pathogens in water, blood, or cell therapy products. In addition, if a culture step is added, it allows the detection of less than 1 CFU in 100 mL in 8 h, making the paper test strips useful for the detection of multiple pathogens in complex samples. However, a filtration step is required to remove enzymes from the medium before lysis of the microorganism. This allows selective detection of viable bacteria. The test strips can be adapted to different bacteria, or different intracellular enzyme markers by varying the nature of the reagents printed on the paper test strip.
Ali et al. presented a simple all-in-one paper-based sensor for the detection of
E. coli using a composite ink
[77][39]. The paper consists of a fluorogenic DNAzyme probe for bacterial recognition and signal generation. It uses lysozymes that lyse the bacteria and sugars that stabilize the printed bioactive molecules. A fluorescent signal can be detected within 5 min of contact between the bacteria and the paper probe. The detection limit is 10
2 cells per mL, in different sample matrices, without sample enrichment (
Figure 3).
Figure 3. Representation of paper sensors principle. In each microzone, DNAzyme sensors are printed. Then, biological samples are deposited into test zones. If a sample contains the target bacteria, the DNAzyme produces a fluorescent signal. This figure is a partial reproduction from reference
[77][39], licensed under CC BY 4.0.
In recent years, many efforts have been made to develop easy-to-use and inexpensive detection methods using paper sensors. Paper is readily available and can be modified by printing several types of reagents. Biorecognition items can be antibodies, nucleic acids, or proteins to detect a variety of analytes. The availability of high-throughput printers combined with microfabrication techniques allows the precise design of small diagnostic devices. The field of early detection of infectious organisms in clinical samples is the preferred area for the use of paper-based sensors. The cited works used E. coli as a target. The same approach can be extended to the detection of other bacteria. This is possible by selecting specific DNAzyme probes from available libraries. These scientific papers focused on food control, but an adaptation to ATMP control is largely feasible.
Several research avenues are promising; paper sensors can be combined with other techniques such as acoustophoresis
[80,81][42][43] for analyte sorting, discussed in
Section 3.2.2, and Raman spectroscopy
[82][44] to improve accuracy (
Section 3.3.1). The system and sampling can also be automated to operate under sterile conditions. A final avenue to explore would be to integrate the paper sensor or DNAzyme directly into the industrial culture process to approach a real-time reading of the ATMP bioreactor.
2.2. Microfluidics
Recent advances in the microfluidic field makes it the most promising technique and place it at the crossroads of sample-based and real-time techniques. The major limitation of microfluidics remains the low volumes processed, but due to other sorting techniques (acoustophoresis) or multiplexing, microfluidics can become a near real-time technique.
Microfluidics is the science of fluid manipulation at the micrometer scale. This field of research is inspired by the nature that already mastered these techniques perfectly, such as blood capillaries or sap transport in plants. Microfluidics can also be considered as a technology for manufacturing “lab-on-a-chip” with innovative applications in the field of chemistry, biology
[83][45], medicine
[84][46], and the food industry
[85][47]. Microfluidic systems include a set of miniaturized components that can replace bulky and very expensive instruments
[86,87][48][49]. This true “microprocessor for biology” represents a revolution for biology and chemistry similar to that brought by microprocessors to electronics and computers.
Sorting by Acoustophoresis
Combined with a classical detection method, microfluidics can be used as a first step to sort pathogens from the rest of the complex environment. This can greatly help the detection of the signal of pathogens that would be otherwise hidden by the strong signal of other components. Several microfluidic methods exist to separate bacteria from a sample
[88,89][50][51]. By applying intense sound waves on micro channels, flowing particles are sorted according to their size because the acoustically induced velocity of a suspended object scales with the square of their radius. For example, small bacteria can be separated from the larger blood cells as depicted in
Figure 4. Blood flows in the periphery of the channel and when the acoustic field is turned on, it applies a force on blood cells. They are thereby moved into the central stream, which then flows in a channel separated from the rest of the blood. On the contrary, small bacteria are much less affected by the acoustic field and stay in the peripheric streams.
Figure 4. Schematic description of acoustic separation principle. The acoustic field separates blood cells by pushing them in the central stream while bacteria stay in the side streams.
Li et al. successfully sorted a mixture of
E. coli and human blood cells using acoustophoresis
[80][42]. The resulting solution containing the bacteria showed a purity of more than 96% bacteria (less than 4% blood cells). This made the following electrochemical detection more sensitive as the non-specific signals due to blood cells were eliminated. As for most of microfluidic systems, their device has the advantages of being cost-effective, automated, and miniaturized. Those characteristics are well-suited for bacterial detection in industrial production.
Similarly, Ohlsson et al. invented a device for acoustic separation of bacteria from blood combined with PCR detection for rapid sepsis diagnostics
[90][52]. The acoustic separation of whole blood was performed as in
Figure 4, then bacteria were trapped onto suspended polystyrene particles. Finally, a PCR was performed on the bacteria trapped in a polymer microchip containing dried PCR reagents. Tested with
Pseudomonas putida, they showed a detection under two hours with an LOD of 10
3 bacteria/mL. Compared with blood culture on samples from septic patients, their system detected half of the septic cases, indicating the real need for further improvement to compete with the reference method. Consequently, they presented a new microfluidics design two years later
[81][43] to increase the throughput of bacteria separation from whole blood. By matching the acoustic impedance of the central stream to that of the blood sample, they could process 1 mL of undiluted blood within 12.5 min, with a bacteria recovery rate of 90% and a blood cell removal rate above 99%.
Dow et al. combined a similar acoustophoresis system with a bacteriophage-based luminescence assay
[91][53]. Tested on
Pseudomonas aeruginosa,
E. coli, and
Staphylococcus aureus, they demonstrated an LOD of six bacteria from blood with initial concentration of 10
2 CFU/mL. Acoustophoresis greatly improved the LOD compared with unpurified samples.
In conclusion, acoustophoresis can be used to separate bacteria from blood cells with excellent bacterial recovery. The high throughput enables the processing of clinical samples in a short period of time. It can also allow real-time monitoring without the need to collect samples during cell therapy production. Rate flow can further be increased through the use of higher blood cell concentrations, larger channels, or multiple parallel separation channels to monitor all types of ATMPs. In combination with downstream bacterial detection, this separation method paves the way for the potential development of fast detection methods. More detection methods should be tested with acoustophoresis in order to find the most suited and reduce the LOD and the time of response. This method can also be used to remove bacteria from a sample for sterilization purposes and not just for detection. The manufacturing of cell or gene therapies can be facilitated.
As for previous methods, acoustophoresis should be validated on a larger number of bacterial species and strains. Other individual bacteria should behave in a similar way during acoustic separation, because the size difference with blood cells is always large. However, pathogenic bacteria are known to form clumps, to reside within white blood cells, or to adhere to blood cells or platelets. The risk is therefore that bacteria are sorted out with the large cells. One solution could be to chemically prevent the adhesion of bacteria to blood cells during the assay with the risk of denaturing the ATMP sample.
Microdroplets and 3D Particle Counter
Droplet microfluidics is another microfluidic method that can improve the detection of pathogens. The idea is to analyze a solution by decomposing it into a microdroplet surrounded by oil. By doing so, every microliter of the solution is screened individually, allowing a finer detection. By sorting the droplets of interest, it is as if the initial solution was concentrated, or enriched, by a factor of several hundred
[92][54].
While droplet microfluidics can improve detection sensitivity and specificity, initial flow rates limited its application to sample with a volume in the microliter range. However, recent developments resulted in a 3D particle counter able to process, within minutes, samples of several milliliters, as usually required for clinical relevance. Kang et al. presented a technology termed Integrated Comprehensive Droplet Digital Detection (IC 3D) based on the microencapsulation of bacteria and DNAzyme-based detection
[58,93][20][55]. Blood samples are mixed in a microfluidic channel with the DNAzyme sensor solution comprising a bacterial lysis buffer (
Figure 5). This mixture is then immediately encapsulated in hundreds of millions of individual droplets. The confinement of the bacteria in the droplets increases the concentration of the bacteria’s target molecules. This allows individual bacteria to be detected more quickly by DNAzyme’s sensors. The droplets are then collected and analyzed using a high-throughput particle counting system. Tested with
E. coli, their system could detect the bacteria from milliliters of diluted blood with an LOD of 1 bacteria/mL. The detection does not need culture or amplification steps and can thus provide a result within 1.5–4 h. It can also detect slow growing organisms such as
Mycobacterium tuberculosis.
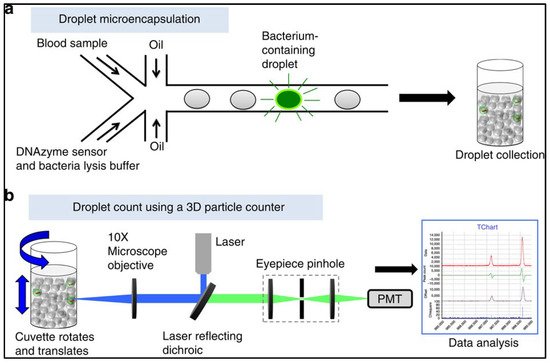
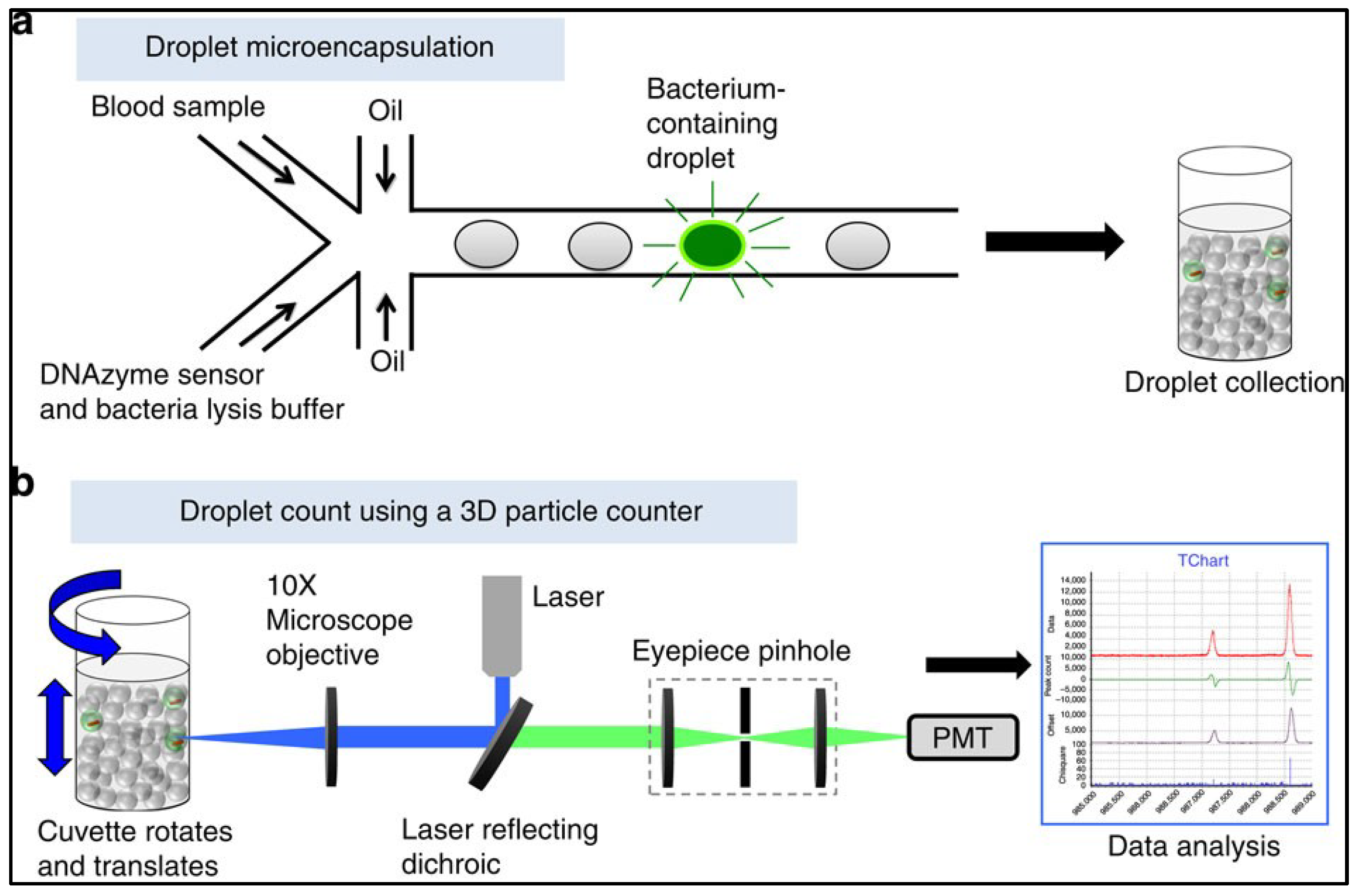
Figure 5. Schematic description of the IC 3D technology. This figure is a reproduction from reference
[58][20], licensed under CC BY 4.0. (
a) DNAzyme and blood samples are mixed and then encapsulated in microdroplets. DNAzyme sensors produce a fluorescent signal in the droplets with bacteria. (
b) The 3D particle counter scans several milliliters worth of droplets within minutes and detects precisely single-fluorescent droplets.
This set of technologies can also detect other pathogens, genetic material, or tumor markers
[94][56]. Hedde et al. also showed how 3D particle sorting enables the detection and isolation of
E. coli from whole blood within minutes for clinically relevant fluid volumes (1–10 mL)
[92][54]. Other combinations of microsystems coupling high throughput bacterial immunomagnetic capture to non-contact cell lysis using an alternating current magnetic field allow bacterial detection in the range of 10
2 CFU/mL with a flow rate of 50 mL/h
[95][57].
In conclusion, microfluidics combined with sorting methods, microdroplets, innovative particle counters, and the use of good ligands is becoming the most efficient and promising method to address the problem of broad-spectrum detection. It allows to process larger volumes and thus to overcome the low throughput problem encountered by flow cytometry. Future perspectives are the development of an automated, compact, and multiplexed device allowing the rapid detection of several bacterial species in any sample, in particular innovative cell therapies. Furthermore, these microfluidic techniques can be coupled to a variety of biosensors for detection and identification.
3. Development of Physical and Computer Analysis Methods
3.1. Raman Spectroscopy
Among instrumental analytical methods, vibrational spectroscopy techniques have long been established; they allow the identification and quantification of the chemical composition of samples in a non-destructive manner. Raman spectroscopy exploits the physical phenomenon in which molecular vibrations in a sample are excited by incoming monochromatic light. This energy transfer results in a small shift in the frequency of the subsequent scattered light. Consequently, measuring the spectrum of the Raman scattered light provides molecular information about the sample. The preparation time is zero or almost zero and samples can be of different types: solid, liquid, or gaseous. Raman spectroscopy offers the possibility of in situ measurements.
Combined with microscopy, Raman spectroscopy allows the detection of a single cell
[98][58]. This avoids the time-consuming step of incubation, and thus increases greatly the rapidity of the analysis. This is similar to solid phase cytometry, but the Raman spectrum allows for a more specific detection. In particular, auto-fluorescent particles can no longer be confused with pathogens. One drawback of the high resolution of microscopy is the time that is required to scan the sample looking for bacteria. Strola et al.
[99][59] overcame this issue by using lens-free imaging instead of a classical microscope, providing a wide field of view. Moreover, lens-free imaging is cheaper and allows for more compact systems. With such a setup, they could localize and detect single bacteria under 1 min, though the concentration of bacteria in the solution, after drying, was high (10
8 CFU/mL).
For bacterial detection applications, other approaches used Surface-Enhanced Raman Spectroscopy (SERS) to enhance the spontaneous Raman signal, which is normally of low intensity
[101][60]. For example, Yang et al. combined an aptamer, a DNA walker on magnetic nanoparticles, and SERS detection to reach an LOD as low as 4 CFU/mL for
S. typhimurium [102][61]. This limit was made possible both by SERS and the enzymatic augmentation performed by the DNA walker. However, the method did not seem specific at this concentration. As another promising example of SERS-based detection, Cheng et al.
[100][62] used electrokinetic microfluidics to isolate and concentrate bacteria on a SERS substrate for detection directly from blood samples. They demonstrated the detection of
S. aureus, E. coli, and
P. aeruginosa under 5 min, with a concentration of 5 × 10
3 CFU/mL.
In conclusion, Raman spectroscopy allows for the detection and identification of pathogens by looking for their specific spectrum inside samples. Compared with infrared spectroscopy, Raman spectroscopy is less hindered by the signal of water and is thus more suited for biological complex media. Consequently SERS-based biosensors are a growing field. There are already many databases of pathogens spectra, but applications to detection in complex biological media are rare. Spectra are difficult to interpret and time consuming to analyze but this technique can be greatly improved by artificial intelligence as described in the next paragraph.
3.2. Deep Learning for Microscopy-Based Sampling Methods
Recent progress in artificial intelligence, and more specifically deep learning, opened the way to an automatic and robust analysis of images. This is also true for the analysis of microscopic images, and deep learning was applied to the detection and identification of microorganisms
[103,104][63][64].
Deep learning is part of the larger family of artificial neural networks (ANNs). ANNs were inspired by the functioning of the brain, with neurons organized in successive layers connected between them (Figure 76), from the input (e.g., images) to the output (e.g., bacteria presence). By training them on labelled data (e.g., image of known bacteria), they can find patterns allowing them to recognize unknown data afterwards. Deep learning refers to ANNs with many consecutive layers and corresponding ANNs are called deep neural networks (DNNs). Such structure was only recently enabled from the development of computational power.
Figure 76. Diagram of deep learning applied to the detection and identification of microorganisms in microscopic images.
In the case of image analysis, DNNs are capable of extracting shape features of images to classify them according to pre-defined labels (e.g., different species of bacteria), or to extract a quantity of an image (e.g., a concentration in bacteria). In practically all fields of image analysis, deep learning has proved to be more accurate than other methods, and even more accurate than human eyes for some applications
[105][65]. Deep learning is mainly used for classification of objects, and in the field of microbiology, to identify various species of virus
[106][66], bacteria
[107[67][68],
108], fungi
[109][69], or parasites
[110][70]. Those studies include the analysis of different modalities of microscopy such as electron microscopy (for viruses), fluorescence microscopy, brightfield microscopy, or even vibrational microspectroscopy. Trained on labelled datasets, deep neural networks are then able to identify microorganisms based on their shape or spectrum for spectroscopic images.
For detection purposes, DNNs can detect automatically bacteria over a large field of view, and distinguish them against other particles. Kang et al. combined several DNNs to detect and identify single cells of five strains of bacteria (
Campylobacter,
E. coli,
Listeria,
Salmonella, and
Staphyloccocus) from visible spectroscopy images
[111][71]. Wang et al.
[112][72] used a DNN to detect bacterial growth inside an agar plate from coherent microscopy images taken every 30 min. Three strains (
E. coli,
Klebsiella aerogenes, and
Klebsiella pneumoniae) were tested for detection and subsequent identification, with an LOD of ~1 CFU/L in less than 9 h.
Deep learning allows for a finer analysis of images for increased sensitivity and specificity in detection. Public databases of different types of microorganisms are emerging
[103][63], which allows a broader use of deep learning for microbiology. For now, studies are mainly carried out on isolates, but it would be interesting to extend on bacteria within complex environments.
In conclusion, while Raman spectroscopy took a long time to analyze and process data in the past, it is becoming fast and very sensitive thanks to deep learning.
4. Improvements and Developments in Real-Time and Online Analysis Techniques
In methods requiring sampling, each breach of containment can potentially lead to a risk of contamination of the sample, whether in the production of advanced therapy drugs, or the industrial production of complex media. On the other hand, not enough sampling may lead to delayed or unrepresentative results. For this reason, methods allowing real-time monitoring of biological samples were developed.
4.1. Bio-Conjugated Nanoparticles
Conjugating nanoparticles (NPs) with ligands such as antibodies, make them interesting tags for pathogens detection
[113][73]. Zhao et al. demonstrated the use of bio-conjugated NP as fluorescent tag in bioassays for the detection of bacteria
[114][74]. Silica NPs were mixed with the sample to be analyzed and bounded to presumed bacteria thanks to specific antibodies (
Figure 87). Then, unbound NPs were eliminated by centrifugation, and finally, the fluorescence of the sample was measured either in well plates by a fluorometer or by flow cytometry. As the NPs encapsulated a thousand fluorophores, they provided a fluorescence intensity a thousand times greater than what a single antibody-conjugated fluorophore could provide. Tested on
E. coli,
Salmonella, or
Bacillus cereus in ground beef samples suspended in a 10 mL solution, the high fluorescence signal allowed for the detection of a single bacterium under 20 min. Moreover, the measurement was quantitative. Their method also works for DNA detection.
Figure 87. Drawing of bacterial cells with nanoparticles conjugated with antibody. The fluorescence intensity is strong, enabling single-bacterium cell identification in aqueous solution.
NPs can also be used as SERS tags for pathogen detection, as Paul et al. showed with Dengue and West Nile viruses
[115][75]. Conjugating NPs with selective antibodies, they reached an LOD of 10 plaque-forming units/mL in less than 30 min. Using magnetic bio-conjugated NPs, it is also possible to selectively isolate bacteria or aptamers specifics to bacteria
[102][61].
In conclusion, bio-conjugated nanoparticles, combined with various detection systems, allow sensitive and specific pathogen detection. They can be used for real-time detection in industrial cell bioreactors or in the manufacture of innovative therapy drugs. However, the question arises as to whether the nanoparticles are safe for humans or cells, and the quantity required for high-volume applications allowing rapid detection of several bacterial species.
4.2. Surface Plasmon Resonance
Surface Plasmon Resonance (SPR) has been extensively used in the past decade for optical biosensors
[116,117][76][77]. Its principle works as follows: when a beam of light illuminates an interface between two media, a portion of the incident light is reflected on the interface and the other portion of the light is refracted through the surface. If a thin layer of metal, rich in free electrons, is deposited at the interface, they come into resonance with the photons of the incident beam. This phenomenon is called Surface Plasmon Resonance. The resonance is visible through a loss of intensity in the reflected beam at a given angle. Any deposit on the surface can be detected because it modifies the angle at which the plasmon resonance occurs. In biosensors, this sensitive detection is used to detect the binding of species present in solutions to ligands grafted to the surface (
Figure 98)
[118,119][78][79]. By grafting ligands specific to different types of pathogens on different positions on the surface, it is possible to perform a multiplex assay. This method is called SPR imaging (SPRi) as the surface is imaged to cover all ligand positions. The identification of the pathogens can be performed simultaneously to detection, depending on the specificity of the ligands used.
Figure 98. Schematic representation of SPRi. Analytes flowing in the channel bind to ligands grafted on a gold surface. This interaction is detected by a change in the intensity of the light reflected on the other side of the gold surface.
The technology of an SPR biosensor has been successfully used for the detection of various analytes such as biomolecules
[120][80] and proteins, bacterial toxins
[121][81], or to monitor the formation of a bacterial biofilm and its degradation
[122][82]. In the case of microorganisms, SPRi have been used for their detection in various media. Nair el al. detected
E. coli in PBS and human urine in under 35 min for concentrations ranging from 10
3 to 10
9 CFU/mL and an LOD of 10
2 CFU/mL
[123][83]. In food samples, Chen et al. demonstrated the detection of
Salmonella, E. coli and
Listeria with a SPRi-based immunoassay. Their system had an LOD around 10
6 CFU/mL for direct measurement on a chicken carcass rinse or 1 CFU/mL with overnight enrichment
[124][84].
Pardoux et al.
[41,68,125,126,127][2][30][85][86][87] proposed a method to make the detection of bacteria by SPRi as fast as possible where they no longer analyze a bacterial suspension after a growth step. The suspension to be tested is injected directly in contact with the microarray. The possible growth of the bacteria and their detection is thus performed at the same time (
Figure 109). The detection limits claimed for standard SPRi in sample-based detection are often less relevant. Thus, by combining the growth of microorganisms and their detection, the time saving is real. This technique has similarities with blood cultures with comparable analysis times. The advantage here is real-time monitoring, whereas blood culture bottles provide a positive response without allowing quantification.
Figure 109. Principle of the method “Culture-Capture-Measurement” for the detection of bacteria by SPRi. The bacteria initially present in small numbers in the sample multiply and end up interacting with the AMPs placed on the surface provided that some have an affinity for them. These interactions are visualized either on the differential images where a difference of color intensity with respect to the initial levels is carried out, or on the kinetics of variation in reflectivity (ΔR) as a function of time.
In this method, the analysis time is governed by the growth rate of the microorganism; the faster the growth, the sooner the detection threshold of the sensor can be reached. (Figure 109) The method has been validated on various strains of Salmonella, Listeria, Staphylococcus, and Escherichia.
SPRi is suitable for bacteria detection in whole blood or cellular products. With few probes, it is possible to detect a wide range of species within less than 18 h from a low concentration sample. The method can be used in real-time monitoring without the need of sampling and the associated contamination risks.
SPRi has a multitude of advantages in bacterial detection. There is no need for multiple sampling and no breach of containment. Each breach of containment can potentially lead to a risk of contamination of the sample, whether in the production of advanced therapy drugs or industrial production of complex media. The analysis is performed in real-time and is extremely interesting in the quality control of health products.
Despite this progress, the conditions required for applications in the health and pharmaceutical industries are not yet in place. Advances in sensor and device design, as well as improvements in ligands, are future prospects in this area. Future research in SPRi can provide this technique a promising role in the pharmaceutical and food industries.
Other techniques are under development, in particular the Localized Surface Plasmon Resonance (LSPR) technique
[128][88] with very promising results in the detection of viruses
[129][89] or in the detection of biomarkers in cancer
[130][90].
If broad-spectrum probes are validated, they can be combined with more specific probes within SPRi sensors. This provides broad-spectrum detection on the one hand, and specific identification of selected relevant strains on the other. A significant advantage of SPRI is that it makes it possible to probe the sample without altering it and then inject the patient with the cell therapy. Another possibility would be to copy the automatic detection model of blood culture bottles and apply it with an SPRi chip. One could proceed to an automated analysis of the final product of ATMP while leaving the possibility of complementary analysis with other conventional techniques from the same sample. Originally the main limitation of SPRi was the limited recognition spectrum due to the ligands used, the antibodies. The use of antimicrobial peptides in complex biological media provides future perspectives in research and development of microorganism detection methods.