Agriculture consumes an important ratio of the water reserve in irrigated areas. The improvement of irrigation is becoming essential to reduce this high water consumption by adapting supplies to the crop needs and avoiding losses. This global issue has prompted many scientists to reflect on sustainable solutions using innovative technologies, namely Unmanned Aerial Vehicles (UAV), Machine Learning (ML), and the Internet of Things (IoT). These new technologies can be used in the analysis of the water status of crops for better irrigation management, with an emphasis on arboriculture.
1. Introduction
The last 60 years have seen remarkable developments in the use of water resources in agriculture in response to population growth and the increased demand for food. The world’s population has grown from 2.5 billion in 1950 to 7 billion today, the area under irrigation has doubled, and water withdrawals have tripled due to the massive development of water infrastructure
[1]. In recent years, most countries in the world have suffered from water stress and drought. Due to the huge lack of precipitation and the high demand, water resources are decreasing dangerously, endangering the food security of many countries. The World Resources Institute has recognized Morocco as one of the countries most affected by water stress, such that by 2040 the level of water stress will reach 80%, which is too high for an agricultural country for which the agriculture consumes 73% of its water resources
[2]. Improved irrigation scheduling—which involves determining the appropriate time to irrigate, the amount of water to apply, and the duration of the irrigation process to ensure a balance between water use and crop response
[3] —is becoming essential to reduce the very high consumption by matching supplies to crop needs and avoiding losses. This problem at the global level has led many scientists to think about sustainable solutions to improve irrigation water consumption through innovative technologies, including Unmanned Aerial Vehicles (UAV), as well as two interesting concepts for more flexibility and automation, namely Artificial intelligence (AI) and Internet of Things (IoT)
[4][5][6][7][8][9][10][11] .
UAVs in agriculture are not only capable of filming to perform a visual field inspection to identify weeds or pest damage but also have the ability to be equipped with various sensors that facilitate the analysis of a complete dataset: nitrogen level, chlorophyll, biomass, and moisture, etc. Artificial intelligence, in this role, is a huge and constantly evolving field. The abilities to predict, estimate, and train decision-making systems make it a practical solution for complex phenomena such as irrigation scheduling. The focus is currently on Machine Learning (ML), which allows for the optimization of certain human activities through soil and crop monitoring, and predictive analysis. In order to feed these algorithms, the process consists in collecting data, thanks to soil mapping performed with UAV, or thanks to cameras and sensors planted in the ground or installed on tractors. Due to the development of wireless sensors (such as thermal sensors, moisture sensors, and light sensors) used in agriculture and the development of transmission technology, the concept of the Internet of Things has generated a lot of interest in data collection and irrigation automation.
2. UAV for Irrigation Management in Arboriculture
Irrigation management involves the determination of the proper time to irrigate and the amount of water to apply, as successful irrigation depends on a delicate balance between water application and crop response
[3]. The water supply must be accurately calculated as well, in an attempt to match the water demand with any amount.
The operation of defining the irrigation schedule and the amount of water to be applied is based mainly on the spatial and temporal monitoring of the water status of crops. Currently, the use of imagery acquired by UAV represents one of the most common methods used. The vast majority of methods based on UAV-acquired imagery and dealing with water status monitoring attempt to train models by transforming the information contained in the imagery into a variable that is highly correlated with well-established ground measurements. The main measurements discussed are evapotranspiration
[5], water potential, and stomatal conductance
[4][7][8][12][13][14]. In addition to these measurements, the contribution of other factors should be considered—such as the local weather patterns, soil types, estimated root depth, and irrigation method
[3]—in order to arrive at models with a correlation coefficient (R
2) close to 1.
-
Vegetation indices (NDVI, NDRE, SR, OSAVI …)
[4][5][7][14].
Generally, these are related to tree structural traits and vegetation characteristics. They can provide reliable spatial and temporal information about crops. These indices showed a clear correlation with variables such as crop factor, stomatal conductance, and water potential in most of the studied crops (see Table 1).
-
Photochemical reflectance index and the normalized photochemical reflectance index (PRI and PRI
norm)
[14][19][20
3. IoT Systems and Irrigation Management
The common definition of the IoT is defined as follows: the Internet of Things is a network of physical objects interconnected via the Internet. A unique identifier connects the objects so that the data can be transferred. The basic goal of an IoT system can be divided into four sections: the identification, detection, processing, and transmission of information. The key technologies in each section are, respectively, radio frequency identification (RFID), sensors, a smart chip, and a wireless telecommunications network. The design of IoT systems varies from system to system based on the intended application of the system, and the level of efficiency and security to be achieved. The most basic architecture is a three-layer architecture:
-
The perception layer: this layer gives each object a physical meaning. It consists of data sensors in various forms, infrared (IR) sensors, or other sensor networks (temperature, humidity, etc.). This layer collects useful information about objects on devices and converts them into digital signals that are then transmitted to the network layer for further action.
-
The network layer: the purpose of this layer is to receive useful information in the form of digital signals from the perception layer, and to transmit it to the processing systems through transmission technologies such as WiFi, Bluetooth, WiMaX, Zigbee, GSM, and 3G, etc., with protocols like MQTT, IPv4, IPv6, and DDS, etc.
[2731] |
Sensors/devices |
Humidity and temperature sensors/Water pump |
The sensor first reads the soil moisture level data. When the humidity level is below the desired level, the humidity sensor sends the signal to the raspberry pi and sends an alert message that notifies the water pump to turn on and provide water. |
Processing system |
Raspberry Pi |
Transmission supports |
Bluetooth |
Control interface |
Mobile app |
[2832] |
Sensors/devices |
Humidity and light sensors/Water pump |
The sensor first reads the soil moisture level data to identify the level of soil dryness. The node then sends the information using a radio transceiver to the base station. The base station then sends both data, moisture level, and exposed light, to the storage server, which is a cloud server. After the treatment, the water pump will turn on and provide water. |
Processing system |
Arduino + cloud server |
Transmission supports |
Radio waves |
Control interface |
Cloud web server + Mobile app |
[2933] |
Sensors/devices |
Humidity and light sensors/Water pump |
The Raspberry Pi computer makes the decision to supply water or not based on all the data received from the sensors. If the conditions are met, the raspberry Pi commands the relay module to turn on the water pump for a specified time, after which the computer commands the relay module to turn off the pump. |
Processing system |
Arduino + Raspberry Pi |
Transmission supports |
GSM and GPRS |
Control interface |
Mobile app |
[3034] |
Sensors/devices |
Humidity sensors, temperature/Water pump, Servomotor |
The humidity and temperature sensors are combined with the input pins of the controller. The water pump and actuator are coupled to the output pins. If the sensors deviate from the defined range, the controller switches the pump on. |
Processing system |
Arduino |
Transmission supports |
GSM |
Control interface |
Not mentioned |
[3135] |
Sensors/devices |
Humidity and temperature sensors/valves, water meter |
IRRIX receives sensor data once a day from the data logger. IRRIX in turn transmits to the data logger the irrigation rates for each sector, in mm, for the new day. The data logger starts the irrigation and ends it when it has measured the programmed rate. |
Processing system |
Data logger + IRRIX web platform |
Transmission supports |
3G |
Control interface |
Web platform |
[3236] |
Sensors/devices |
Humidity and temperature sensors/pumping system, main, branch and collector (feeder) pipes, Lateral booms, valves, water meters, pressure and flow regulators, automatic devices, backflow preventers, vacuum valves, air release valves, Filtering system, Chemical injection equipment, Drippers |
The smart humidity sensor monitors both the humidity and the temperature of the air. The ratio of the humidity of the air to the highest amount of humidity at a particular air temperature is known as relative humidity. This Relative humidity hence becomes an essential component in the operation of water pumping systems. |
Processing system |
A smart system built using the Field-Programmable Gate Array Technology (FPGAs) and HDL language |
Transmission supports |
Radio waves |
Control interface |
Not mentioned |
[3337] |
Sensors/devices |
weather station node/soil moisture and soil electrical conductivity sensors |
The remote server receives the environmental data through the ZigBee and GPRS network, and the weather data directly through the GPRS network. The remote server then allows using the deep learning algorithm long short-term memory (LSTM) to improve the prediction of soil moisture and electrical conductivity. |
Processing system |
Remote server |
Transmission supports |
ZigBee/GPRS |
Control interface |
Webserver |
-
Evapotranspiration.
The term “evapotranspiration” was coined to represent the combination of the two phenomena of evaporation and transpiration. The evaporation is the phenomenon of water changing from a liquid state to a vapor state. The evaporation of a water surface, a pond or a lake, or the surface of soil are examples. Transpiration is the phenomenon of evaporation of water by trees through stomata
[15].
The water requirement of a tree is the amount of water needed to make a tree mature. A tree requires between 400 and 1000 kg of water to produce one kg of dry matter
[15]. These water requirements and the actual evapotranspiration are identical under standard conditions
[3]. The actual evapotranspiration of a crop is calculated as follows
[16]:

(1)
where k
c is the single-crop coefficient, ETc is the actual crop evapotranspiration, and ET
0 is the reference evapotranspiration, i.e., the evapotranspiration of an ideal plant stand with water available at will.
The indices derived from the UAV images represent the second input of the models (see
Table 1). These indices are classified into two categories: the reflectance indices and the indices based on the leaf/canopy temperature.
2.1. Reflectance Indices
The reflectance indices processed are in the form of two categories:
PRI is an index that takes into account changes in xanthophyll concentration due to water stress. The PRI
norm is an improvement of the PRI index over water potential and stomatal conductance; it not only takes into account changes in xanthophyll concentration due to water stress (PRI) but also generates a normalization considering the chlorophyll content, sensitivity to chlorophyll, and stress-induced reduction in the canopy leaf area
[21]. The PRI
norm showed an improved ability to detect water stress compared to other greenness and structure indices
[21].
2.2. Indices Based on Leaf/Canopy Temperature
The extraction of the temperature of the leaves/canopy, through thermal sensors, can have an observable effect on the estimation of the water status of crops, especially in arboriculture. This effect has been observed through several studies that have collected thermal indices for different crops, such as peach, vineyard, olive, and almond, etc. On the other hand, the Crop Water Stress Index (CWSI) represents the most widely used index. Following Idso et al., 1981
[22], the CWSI ranges from 0 to 1 (values close to 1 are related to high stress levels). This index showed better agreement with Ψ and g on most of the studied fruit trees (see
Table 1)
[8][23][24].

(2)
where Tcanopy is the surface temperature of the canopy and reference surfaces, and Twet and Tdry are completely wet or dry in order to simulate the maximum and minimum leaf transpiration under the exposed environmental conditions.
Table 1. Examples of studies addressing water status monitoring using UAV imagery in arboriculture.
Analyzing the different examples cited in Table 1, generally, the architecture of irrigation systems based on IoT technology takes the structure detailed in Figure 1.
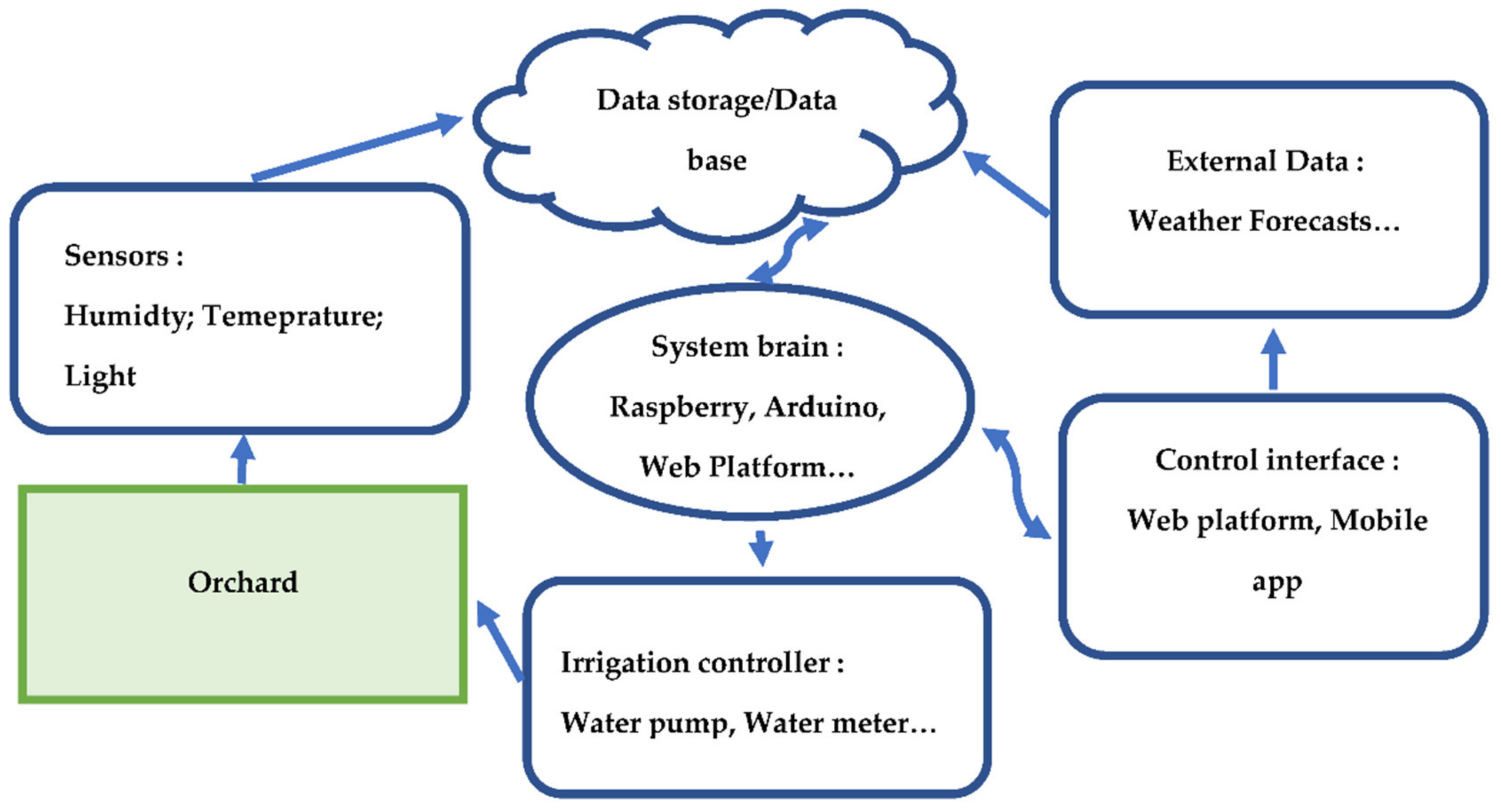
Figure 1. Generalized structure of the architecture of IoT-based irrigation management systems.
An irrigation management IoT solution is based on three levels of components that include the sensors/actuators, the gateway, and Cloud computing:
-
Sensors and actuators—all of the systems mentioned include sensors to collect data on physical quantities such as luminosity, temperature, and soil moisture. The actuators remain a complementary technology to the sensors; they convert electrical energy into motion or mechanical energy, which is used to control the water pump. A microcontroller (Arduino, in most systems) includes a processor, memory, input, and output devices on a single chip. The role of a microcontroller is to process the raw data captured by the sensors and extract useful information.
-
The gateway—gateways have the role of connecting sensors or sensor nodes with the outside world, and have the ability to perform local processing on the data before transmitting it to the Cloud. The data can be transmitted between all of the processing system counterparts via transmission media such as WiFi, Bluetooth
[2630], WiMaX, Zigbee
[3337], GSM
[3034], and 3G
[3135], with protocols such as IPv4, IPv6, MQTT, or DDS, etc.
-
Cloud computing—three forms of IoT cloud are available: the cloud infrastructure, cloud platform, and software cloud. Some systems have used a cloud
[2832][3135], while other systems are based totally on local processing
[2731][2933][3034]. Regarding cloud computing, it is an optional choice that may be used to lighten the load of work for the cloud. This processing can be performed at the local nodes before relaying the information to the cloud (Fog Computing); at the network edge, at gateways, or at intermediate nodes (Edge Computing); or can be performed locally in the sensor node (Mist Computing).
Reference |
Type of Crop |
Model Inputs |
Results |
[5] |
Pomegranate |
NDVI |
Kc |
R2 = 0.999 |
[7] |
Vineyard |
IVs combinés |
Ψ |
R2 = 0.83 |
[4] |
Sweet cherry |
NDVI |
Ψ |
R2 = 0.60 |
[25] |
Almond |
NDVI |
Ψ |
R2 = 0.7 |
[1426] |
Peach |
NDVI |
Ψ |
R2 = 0.72 |
Peach |
R700/R670 |
Ψ |
R2 = 0.88 |
Apricot |
TCARI/OSAVI |
Ψ |
R2 = 0.88 |
TCARI/OSAVI |
G |
R2 = 0.77 |
Almond |
TCARI/OSAVI |
G |
R2 = 0.65 |
PRI |
Ψ |
R2 = 0.53 |
Orange |
R700/R670 |
G |
R2 = 0.62 |
Peach |
R700/R670 |
G |
R2 = 0.93 |
PRInorm |
Ψ |
R2 = 0.72 to 0.88 |
[1927] |
Olive |
PRI |
Ψ |
R2 = 0.84 |
[8] |
Peach |
CWSI |
Ψ |
R2 = 0.72 |
Peach |
CWSI |
G |
R2 = 0.82 |
[2328] |
Vineyard |
CWSI |
Ψ |
R2 = 0.69 |
Vineyard |
CWSI |
G |
R2 = 0.70 |
[2429] |
Olive |
CWSI |
Ψ |
R2 = 0.60 |
Olive |
CWSI |
G |
R2 = 0.91 |
3.1. Architecture of IoT-Based Irrigation Systems
Presently, IoT is becoming established as a new industrial revolution in all sectors of agricultural activity, especially in irrigation management, while remote monitoring systems have been proposed to manage irrigation in an optimized way by applying the right dose at the right place at the right time. Herein present examples of systems with the proposed composition
[2731][2832][2933][3034][3135][3236] (see
Table 2) that will provide a comprehensive view of smart irrigation management systems based on IoT technology, and will arrive at a generalized structure of its systems.
Table 2. Examples of irrigation management systems based on Internet of Things (IoT) technology.
Reference |
Composition |
Operation |
3.2. Combination UAV and IoT in Irrigation
UAVs have the potential to be part of an IoT solution for irrigation management by performing several tasks
[9][3438][3539][3640][3741]. The tasks in which these aircraft can intervene are classified into two categories according to the communication between the UAV and other system parts.
Tasks with direct communication with the system include real-time communication between UAV and sensors, which is now available with the development of federated wireless smart sensors (WSN) which communicate with the UAV. The use of UAVs with intelligent ground-based WSNs is proven to be a robust and efficient solution for data collection, monitoring, and analysis. The advantage lies in online data collection and relaying to a central monitoring point, while effectively managing the network load and latency through optimized UAV trajectories
[3438]. The GS-UAV-SC (GS for ‘Ground Sensors’, and UAV-SC for ‘Unmanned aerial vehicle small cells’) model, proposed by Duangsuwane et al. 2020
[9], represents a perfect example. The GS-UAV-SC (
Figure 2) is a soil moisture-monitoring sensor, which is connected to the UAV to stream data over the Internet in order for the data to be stored and calculated on the cloud platform. The farmer/user can further control the UAV in its movements around the field to collect all of the data from each sensor.
Figure 2. Illustration of UAV and WSN
[9].
On the other hand, a UAV can function as an energy controller. Especially with the fact that most wireless sensors in the IoT environment are facing the limited energy problem, the way in which to provide sustainable energy to these sensors has become a challenge. In this background, Liu et al. 2019
[3539] presented a UAV to swap energy among wireless sensors by adapting the optimal resource allocation approach based on dynamic game theory (
Figure 3).
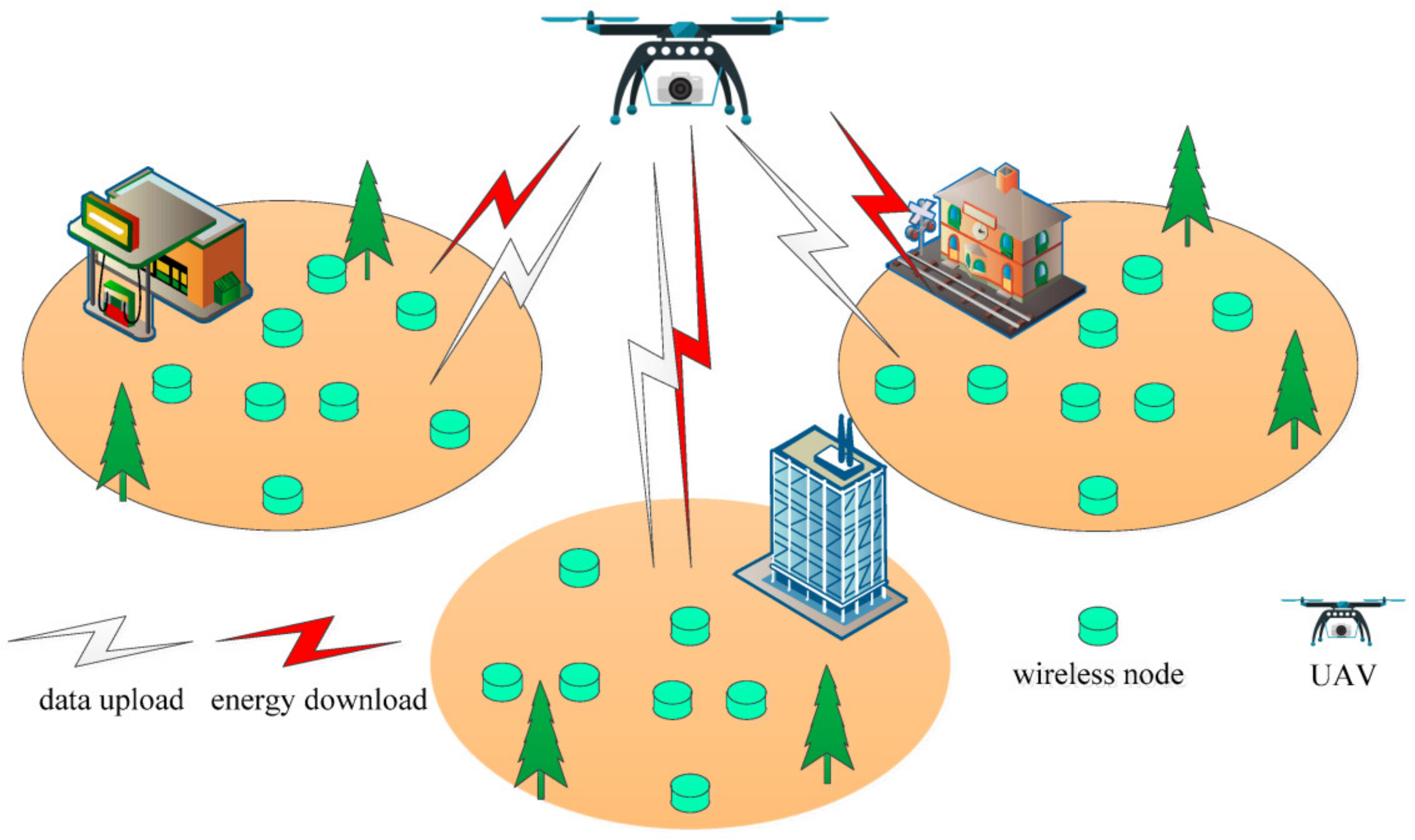
Figure 3. Illustration of the system proposed by
[3539].
In tasks with non-direct communication with the system, a UAV can play the role of a versatile sensor for an IoT system by providing it with a set of external data extracted from UAV images after treatments. Data such as the plant height
[3640] can be used by the system to estimate the growth phase of the fields, and to provide them with the doses that suit with this phase.
The advantage lies in the use on large farms. The acquisition of several interesting data in the monitoring of the water status of crops (temperatures, humidity, ETc, water potential, etc.) requires the use of several sensors to cover the whole area, while a UAV has the potential to replace some sensors, and to provide the IoT system with these data after extracting them from the processed images.
4. Conclusions
The water stress that faces global agriculture requires a scientific intervention to develop solutions to this problem in order to ensure food security and sustainable development by making irrigation management one of the priorities. The papers treated have shown a significant contribution of UAV-ML-IoT technologies in irrigation management and the analysis of the water status of crops. This contribution is due to the relevant information that can be extracted from UAV images through the different sensors (RGB, multispectral, heperspectral, and thermal), and due to the ability of ML algorithms to define models for the estimation of water and other requirements by linking ground and aerial measurements, as shown by the medium-to-high correlation coefficients and the important role of wirelessly connected sensors in the implementation of smart systems.
The challenge of water scarcity, combined with the utility of these technologies, has made the development of an integrated Drone-IoT-ML approach to irrigation management an absolute necessity. Focusing on Morocco and developing countries in general, the usefulness of these technologies can be emphasised in the face of a set of challenges such as the difficult financial situation of many farms and the inability of the farmer to use the information if human interventions are not available. Those challenges are likely to hinder the widespread adoption of these new technologies by farmers in order to adapt and modernize their production practices to obtain the best returns on these investments.
Those facts suggest two perspectives for next works, which are the estimation of water needs in the case of arboriculture, and the implementation of a system of which the role will be to balance the estimated water needs and the water provisioning, based on the Drone-IoT-ML approach.
Abbreviations
CWSI |
Crop Water Stress Index |
DDS |
Data Distribution Service |
FPGA |
Field-Programmable Gate Array Technology |
GRVI |
Green Red Vegetation Index |
IoT |
Internet of Things |
ML |
Machine Learning |
MQTT |
Message Queuing Telemetry Transport |
NDRE |
Normalized Difference Red-edge Index |
NDVI |
Normalized Difference Vegetation Index |
RFID |
Radio Frequency Identification |
RGB |
Red, Green, Blue |
RNN |
Recurrent Neural Networks |
SVD |
Singular Value Decomposition |