Battery Swapping Stations (BSS), refers to the rapid recovery of electric vehicle energy by replacing batteries when the electric vehicle energy is about to run out. Figure 1 shows the structure of BSS. The Electric vehicle users only own cars and rent batteries from service operators, thus greatly reducing the purchase cost of electric vehicles and solving the problem of long charging times.
1. Advantages and Problems of Battery Swapping Stations
Battery Swapping Station (BSS), refers to the rapid recovery of electric vehicle energy by replacing batteries when the electric vehicle energy is about to run out.
Figure 1 shows the structure of BSS. It Electric vehicle users only own cars and rent batteries from service operators, thus greatly reducing the purchase cost of electric vehicles and solving the problem of long charging times. In 2011, Israel’s Better Place first promoted battery swapping in Denmark, then signed agreements on the development of electric vehicles in Australia, Tokyo and Canada, reaching the intention of building battery swapping stations. Tesla, an American electric vehicle giant, also launched a pilot project for replacing electric vehicles
[1][2][19,21], and Gogoro, an electric motorcycle from Taiwan Province, also completed the layout of the electric motorcycle replacement network in Taipei. Although Better Place was finally declared bankrupt due to the break of capital chain, and Tesla’s pilot project of power conversion did not continue to be implemented, the concept of battery swapping has attracted attention in China. In 2014, BAIC Group indicated that it would vigorously promote the construction of battery swapping stations in the Yangtze River Delta and Pearl River Delta regions; and in 2015, it will jointly build battery swapping stations with Sinopec. In 2018, Weilai Company’s battery swapping station for private users was built in Shenzhen. With the continuous expansion of electric vehicle market, many enterprises such as Aodong New Energy, Sinopec, and Weilai accelerated the layout of power stations, which shows that the technological path of battery swapping is an important direction for the development of the new energy automobile industry.
Table 12 compares the techniques for battery swapping and battery charging.
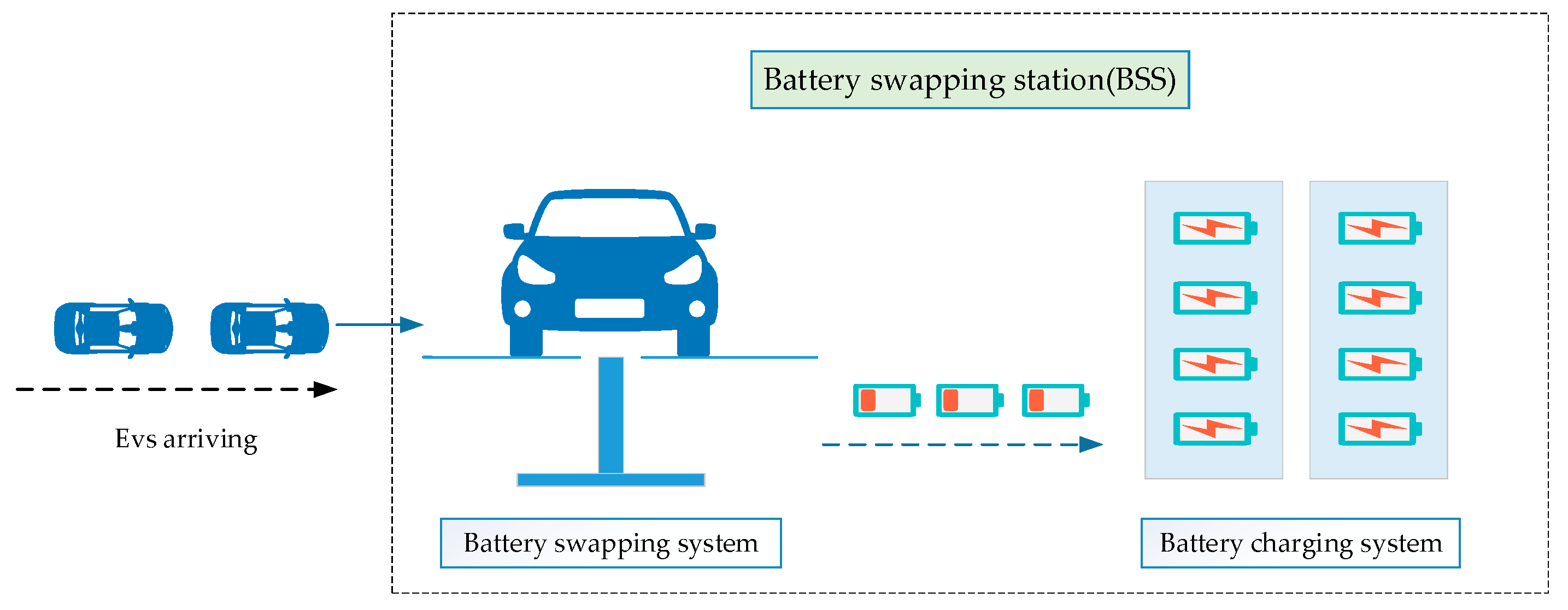
Figure 1. BSS architecture.
Table 12. EVs battery swapping technology vs. charging technology.
|
Battery Swapping |
Charging |
Charging efficiency |
3–5 min |
≥30 min |
Figure 2 shows the operating framework of a battery swapping station. It can be seen that it is a system in which users, battery swapping stations and power grids interact with each other. When the EV battery reaches the threshold of changing power, a BSS will be selected to change power and generate depleted batteries (DB) to be recharged, then put the battery to be charged into chargers. EVs must wait if there are no fully charged batteries (FB) in the station. It can be seen that the battery swapping station is not a separate operation system. Due to the operation of battery charging or discharging, the battery, the distribution network and the battery swapping station are all under centralized management and constitute an integrated system. Compared with the charging station, the battery swapping station (BSS) has three main advantages:
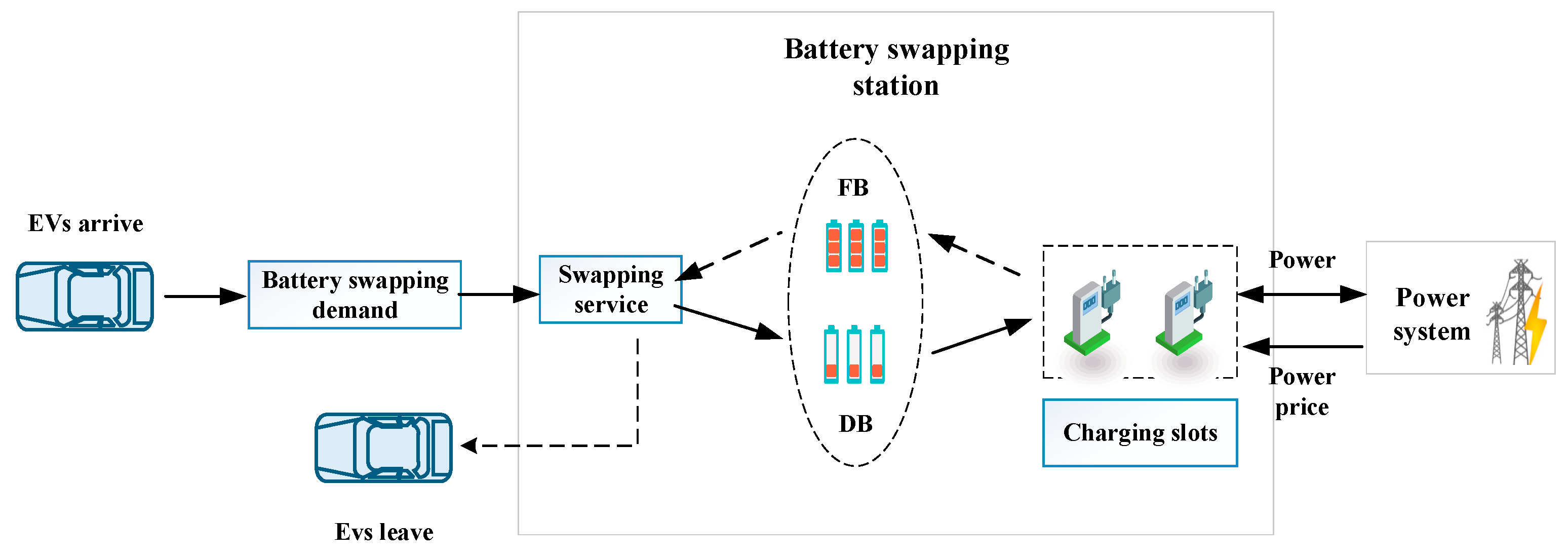
Figure 2. The operating framework of BSS.
Battery recycling |
easy battery recovery |
difficult battery recovery |
Battery maintenance |
managed by professionals, extending the battery life by 30–60% |
managed by consumer, short battery life |
Operability |
However, it also faces some challenges:
-
High construction investment cost. Battery cost is very expensive—too many batteries increase costs, and too few batteries cannot meet customer demand.
-
Heavy power grid pressure caused by disordered charging of batteries. Both fast charging and slow charging will cause load pressure on the power grid with the popularity of electric vehicles.
Intelligent, fully automated |
2. Construction and Planning of Battery Swapping Infrastructure
2.1. Deployment of Battery Swapping Stations
In order to ensure the availability of charging capacity and promote the development of electric vehicles, extensive basic charging facilities must be established. Based on the classical location theory, many studies have incorporated the charging characteristics of electric vehicles into the optimization model to locate the charging infrastructure. The location model of electric vehicles is greatly inspired by the early gas station location problem. Based on the assumption of refueling demand, the proposed models can be divided into node-based, arc-based and flow-based models
[3][22]. Different from the node-based model, the flow-based model assumes that the refueling demand exists in the form of traffic flow passing through refueling facilities, which is more in line with the reality
[4][23]. The model is derived from the concept of maximum coverage, and its goal is to capture traffic flow as much as possible on the basis of locating P facilities. On this basis, many scholars have extended this to the problem of charging stations and site selection of power exchange stations. This paper mainly summarizes the site selection of power exchange stations.
On the basis of the flow-based location model, the location problem of a battery swapping station based on two objectives is proposed, then two distributed robust optimization models are established and approximated as mixed-integer second-order cone programming to determine the boundary which was solved by CPLEX
[5][6]. When electric vehicles drive on expressways, it is easy to run out of electric power due to the long distance. Then, the integer programming model is constructed under the traffic flow determination scenario and the genetic algorithm is used to solve the problem. Secondly, under random conditions, an opportunity constraint model considering the service level is constructed, and the genetic algorithm and simulation method are used to solve the problem. The results show that the location of the BSS can be arranged in high-profile areas, highway intersections and high-traffic areas
[6][24]. Considering traffic and weather conditions, a two-stage model was proposed to solve the BSS location problem and minimize the sum of construction and operation costs
[7][25]. The network equilibrium framework is another solution to address the location problem
[8][26]. The authors propose mixed-integer non-linear programming, which combined the driver’s route selection with the charging decision. In addition to flow-based location and network equilibrium, some scholars have proposed data-driven methods to solve the location problem. For instance, a data-driven framework is proposed to solve the PROBLEM of BSS positioning based on GPS data of taxi in large cities
[9][10][27,28]. Through region matching and trajectory extraction using the Hidden Markov Model (HMM), the power consumption process of electric vehicles is inferred, and then the space-time distribution of power exchange demand is obtained by clustering calculation. Finally, places with dense battery demand are selected as BSS location candidates. Research shows that the average distance error between the power demand position and the nearest BSS calculated by this method is reduced by 52.5% and 62.7%, respectively. In addition, the land occupation cost of BSS, the comfort of drivers and the impact on the load level of the power grid may all be factors affecting the location of BSS, and the comprehensive evaluation model for the location of BSS from three angles of economy, society and technology was put forward for the first time
[11][29].
2.2. Location-Routing Problem
In addition to the basic location problem, another research branch is to consider the vehicle route when determining the optimal location of the substation, that is, the popular location-routing problem. Location problems are usually aimed at minimizing the construction cost and transportation cost of the power station, for which the tabu search algorithm and the mileage-saving algorithm can be used. However, electric vehicles are only allowed to visit customers once
[12][30]. On this basis, there are other authors who assume that customers can be visited many times and adopt the adaptive large neighborhood search algorithm to determine the location of BSS
[13][31]. Then, the impact of BSS on the distribution network is also considered
[14][32]. With the development of research, the two-stage vehicle routing problem of electric vehicles with time windows and battery swapping stations was put forward
[15][16][33,34]. On the first floor, large-capacity electric vehicles are used to transport goods from storage to the distribution center; and on the second floor, small-capacity electric vehicles are used to provide services for customers from the distribution center who visit the battery swapping station many times. In this paper, an integer programming model is built with the goal of minimizing transportation cost, the loading and unloading cost of the distribution center and battery exchange cost, which is solved by the gurobi exact solution, the column generation algorithm and the adaptive large neighborhood search algorithm. Wind energy also be used in power supply. In
[17][35], the battery distribution path is considered under the centralized charging and unified distribution mode. A joint optimal scheduling model of the wind power generation plan, battery exchange demand, battery charge and discharge and VRP is established, and the model is solved by the genetic algorithm. The dynamic programming algorithm
[18][36], the mixed-variable neighborhood search (HVNS)
[19][37], the adaptive genetic algorithm
[20][38] and the colony algorithm
[21][39] are also effective algorithms to solve mathematical models. These studies are summarized in
Table 23, which includes the research objective, the optimization model, and the solution method for the optimization step.
Table 23.
Collation of key documents of BSS planning.
(1) Deployment of Battery Swapping Stations |
References |
Target |
Model |
Solution Method |
Mak et al. (2013) |
Minimize the cost in the worst case; maximize the probability of the worst case |
Distributed robust optimization |
CPLEX |
Yang and Sun (2015) |
Minimize construction cost and inventory cost |
Mixed-integer linear programming |
Genetic algorithm |
An et al. (2020) |
Minimize construction cost |
Two-stage stochastic programming model |
Gradient descent algorithm |
He et al. (2015) |
Minimize the total social cost |
Mixed-integer non-linear programming |
Genetic algorithm |
Zeng et al. (2019) |
Reduce the average distance error between power demand point and BSS |
Hidden Markov Model |
Map matching algorithm based on hidden Markov: trajectory reduction algorithm (TTR); K-means clustering; hierarchical clustering |
Wang et al. (2019) |
Minimize cost |
System dynamics model; space-time distribution model of electricity demand |
Improve the difference algorithm; Monte Carlo search |
(2) Location-Routing Problem |
Yang and Sun (2015) |
Minimize construction and transportation costs |
Mixed-integer linear programming |
Taboo search; improve mileage saving; four-stage heuristic algorithm: scanning algorithm, iterative greed, adaptive large neighborhood, CPLEX |
Hof et al. (2017) |
Minimize construction and transportation costs |
Mixed-integer linear programming |
Adaptive large neighborhood search algorithm |
Jie et al. (2019) |
Minimize transportation costs, distribution center handling costs, and battery exchange costs |
Bilevel integer programming |
Gurobi; column generation algorithm; adaptive large neighborhood search algorithm |
Li et al. (2020) |
Minimize the total cost associated with energy consumption and travel time of electric vehicles |
Mixed-integer programming |
Adaptive genetic algorithm based on climbing optimization and neighborhood search |
2.3. Inventory Problem of Battery
How many batteries should be stored in the battery swapping station is a very important decision in the early stage. It is of practical significance to determine the number of batteries and chargers under the background of the random arrival of automobiles, random battery status and random charging time
[22][23][40,41]. It is ideal to improve battery availability by replacing exhausted batteries immediately upon arrival at the battery swapping station and charging them at the same time, but the charger and battery costs are the main factors affecting the battery inventory
[24][42]. Therefore, a mathematical model aiming at the annual average cost of facilities and taking the scale of power stations and daily battery exchange demand as constraints is established, which is solved by the differential evolution method
[25][43]. This method ignores the battery charging cost. The BSS optimization model with the largest battery inventory can be built by optimizing the battery charging mode, and then different charging schemes are designed to minimize the battery cost, such as the battery optimization for Hong Kong airport bus station
[26][44]. The scale and capacity mentioned above are hard constraints, and customer waiting time can also be used as a soft constraint that affect the capacity of electrical changing stations. In
[27][45], the authors constructed an optimization model of the power station aiming at minimum investment, minimum maintenance and minimum charging cost, and restricted extra waiting time (EWT) of electric vehicles as the service quality. By simulating the states of electric vehicles and batteries under different operation strategies, the EWT of each electric vehicle is calculated, and the quantitative relationship between electric vehicles, batteries and chargers under different EWT levels is obtained, which provides a reference for the configuration number of batteries and chargers in the substation. The process of vehicles arriving at stations to receive services is actually a hybrid queuing network, and the queuing theory can also be used to solve the problem of capacity optimization
[28][46].
3. Conclusions
- (1) The existing research on the battery swapping station focuses on the location problem and charging strategy optimization, while the research on the number of batteries in the early stage of the BSS is scant. Some articles regard the initial battery reserve quantity as a fixed value that must be met while other articles utilize sensitivity analysis in determining the the battery reserve, without related research modelling the battery reserve.
- (2) Optimization of the electric vehicle charging strategy has become a hot topic in recent years, and has also achieved rich research results. However, under the current development mode of BSS, there are some limitations for practical engineering applications. First, most studies directly control the load on the power grid from the perspective of operators, power grids and electric vehicle users, without considering the operational difficulties caused by actual user needs and user experience. Second, the current research results fully consider the peak shaving and valley filling effect brought by orderly charging under the time-of-use power price, while ignoring the restriction of the number of chargers in the BSS and the constraints of user service ability. Moreover, the research results are suitable for centralized scheduling of electric vehicles, and are not suitable for the orderly scheduling of a single vehicle or a small number of vehicles in the battery swapping mode. Third, most studies model the probability distribution of power price and battery demand by fitting statistical laws to historical data. The difference between artificial assumptions and actual uncertainties will affect the reliability of the results.
- (3) At present, there are few studies on the assignment of BSS, and the existing research focuses on how to provide the best scheme for users and reduce the waiting time for power exchange in the future; how to provide users with the shortest waiting time for power exchange; how to effectively improve the utilization rate of power station. Although cooperative scheduling between moving electric vehicles and the power exchange station is considered, the following problems are not considered: when the power exchange demand increases to a certain threshold, the waiting time for power exchange is too long due to an insufficient battery reserve and the single battery swapping stations accepting excessive demand. There is usually only one optimization index, which does not have the ability for self-learning and self-improvement of decision accuracy and reliability.
First, it is possible to build a battery reserve planning model based on a queueing network in combination with battery swapping requirements. Second, instead of harmonizing past data, the reinforcement learning algorithm can be directly used for data training to control the battery charging strategy. Thirdly, from the perspective of BSS operators, optimal decisions can be made using the reinforcement learning method with the goal of balancing the resource allocation of multiple swapping stations.