Artificial intelligence (AI) is one innovation emerging from the digitalization trend, often being used for precision agriculture and to enhance smart farming techniques. The digitalization of agricultural systems is aimed at the technological optimization of production, value chains, and food systems, as well as minimizing the environmental impacts of agriculture.
1. Introduction
The global agricultural sector faces major challenges, such as a rapid, growing global population, climate change, resource depletion, soil degradation, water pollution, and biodiversity loss
[1][2][1,2]. There are increasing calls for sustainable agricultural intensification to increase productivity
[1], minimize environmental impacts, and provide social benefits
[3]. Finding solutions that are economically, politically, environmentally, socially, and culturally sustainable is a seemingly insurmountable challenge; yet, digital technologies are often positioned as the transformational solution for solving global agricultural challenges
[4]. The use of digital technologies to transform agri-food systems is often referred to as the fourth agricultural revolution and is characterized by “high-tech, radical, and potentially game-changing technologies”
[2].
AI refers to the development and implementation of intelligent machines or softwares that act by recognizing and responding to their environments, thereby allowing for the analysis of large amounts of data
[5][6][7,8]. Advancements in AI have already been applied to agricultural production contexts, with predictors expecting they will assist in ensuring global food security
[6][8], ushering in a wave of AI innovation in the agricultural sector
[7][8][9,10]. The benefits of integrating AI into agricultural production abound, ranging from improving the traceability of food-related outbreaks, improving hygiene on production sites, integrating supply chains, reducing waste related to production, reducing the agricultural sector’s carbon and ecological footprint, and increasing economic profitability
[7][9]. The motivation underlying AI adoption in agriculture is the idea of using innovation to optimize production systems to feed an increasing global population while simultaneously conserving per capita agricultural land area and preserving soil health and environmental quality
[9][10][6,11].
The role of AI in developing a sustainable agri-food system has been recognized
[7][9]; yet, the holistic social and political sustainability of technology adoption, specifically related to digital technologies, remains a relatively unexplored subject area
[3]. According to O’Connor
[11][12], there are four spheres of sustainability: the environmental, economic, political, and social spheres, each interacting and acting upon the others in a reciprocal manner. Research related to AI in agriculture has widely integrated the environmental
[12][13] and economic realm
[13][14], with research emerging targeting the political and social realms
[14][5]. Research to date has primarily focused on the technical aspects of applying technologies to improve agricultural practices or identifying the barriers to AI adoption
[14][15][16][5,15,16]. Less frequently mentioned in the discourse surrounding the digital agricultural revolution are the potential negative impacts, or unintended consequences, of digital transformation on economic, environmental, social, and institutional systems that comprise the agri-food sector and beyond
[4]. Research into the social sustainability of AI in agri-food systems
[3][14][17][3,5,17] foreshadows a need to avoid the consequences of the agricultural innovations of the past, such as those experienced with the introduction of genetically modified organisms (GMOs)
[18]. In addition, anticipating unintended public and policy-related challenges emerging from the disruptions ushered in by agricultural innovations
[19], especially those that reduce the labor market (which AI does), must be studied
[5][7]. The current research on AI, outside of the agricultural sector, delimits many of the potential pitfalls of AI, including impacts on the labor market, the political landscape, and the medical field
[20][21][22][20,21,22]. Limited research, however, examines the communication and outreach of AI as an emerging technology. Without examining the mechanisms of communication surrounding AI across sectors, scientists risk the efficacy and sustainability of an innovation within the global population. Effective communication and scientific outreach efforts should incorporate two-way communication between scientists and the public to not only improve the reputation of specific innovations but also to improve the development of innovations through listening to audiences and tailoring innovations to their needs
[23].
2. Exploring Twitter Discourse around the Use of Artificial Intelligence to Advance Agricultural Sustainability
The findings supported previous research that described the discourse around agricultural innovations as overwhelmingly positive
[2][3][2,3]. The general discourse within precision agriculture, and the AI conversation specifically, is operating under a pro-innovation bias
[24], supported by the findings presented in the current study. Maintaining a techno-optimistic stance in outreach alone does not ensure widespread adoption in the public sphere, which will ultimately impact policy for a specific innovation if it trends negatively, as evidenced by the pitfalls experienced related to GMOs
[18][25][18,28].
Using systems thinking to consider the consequences of an innovation within social, political, cultural, economic, and environmental contexts (
Figure 1) may be an effective tool in making improvements to the innovation itself prior to widespread dissemination
[26][30]. With the predominately positive outlook on AI in agriculture, few themes specifically addressed the potential drawbacks of the innovation, revealing a lack of systems thinking practice within innovation development and dissemination. Taking all aspects of a system adopting AI into account can also be used to improve outreach and science communication efforts related to AI as the industry considers widespread adoption as a solution to broad agricultural issues
[24]. Considering the results, the environmental implications of AI in agriculture are already embedded within Twitter discourse, specifically as identified in the themes and subthemes of climate change, higher yields and productivity, and AI and sustainability. The use of AI in agriculture was positioned as a necessary solution for combating climate change, enhancing the environmental sustainability of production agriculture, and reducing land use through higher yields. The sustainability frame of AI in agriculture may be an effective messaging strategy when attempting to influence policy among more environmentally focused decision-makers, as well as environmentally focused potential adopters of the innovations.
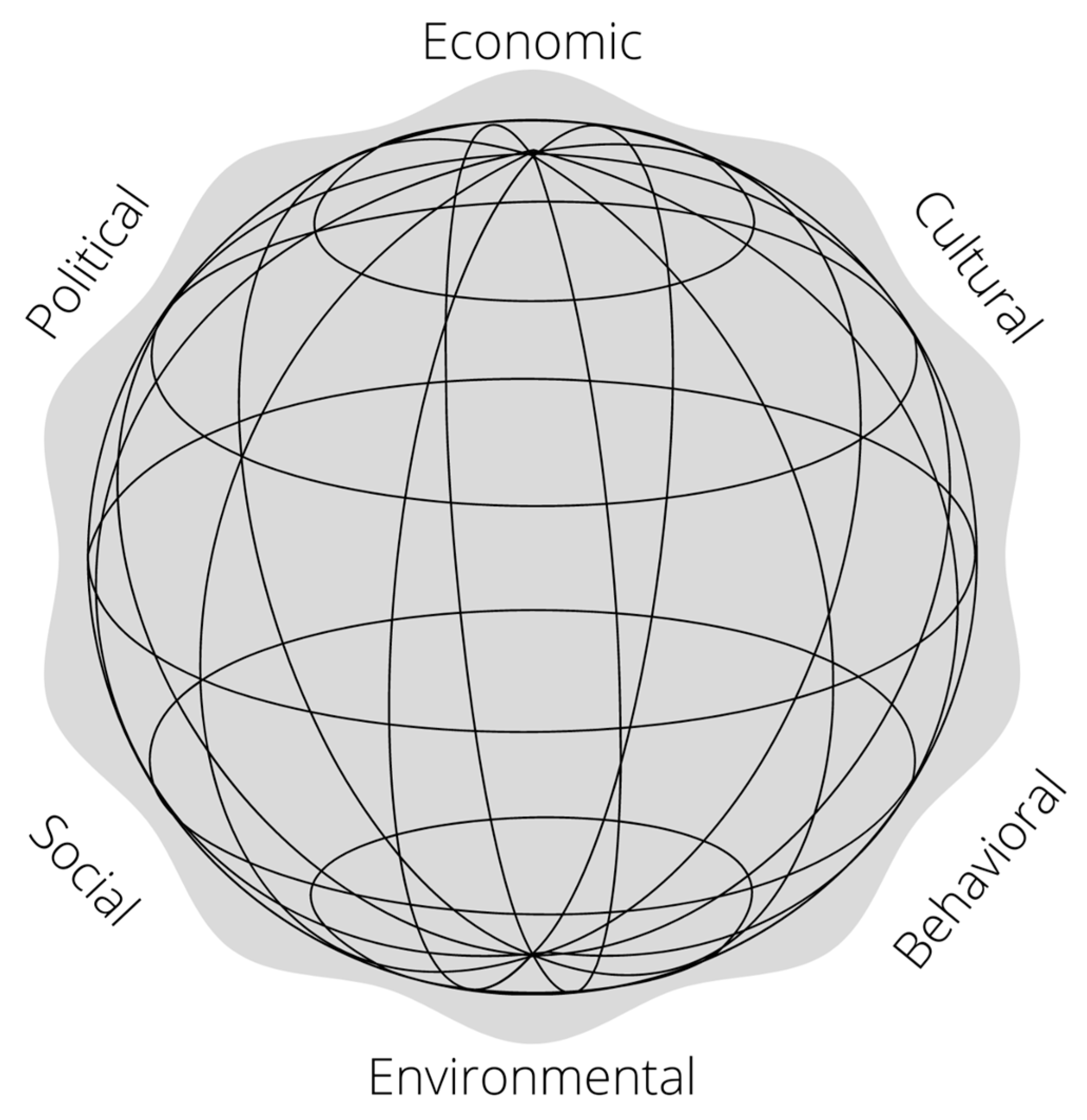
Figure 1. Elements of systems thinking.
The economic context was also present within Twitter discourse, depicted through the increasing profits and reducing production costs theme. The economic frames, however, were predominantly production-oriented, limiting the messaging effects for policy related to increasing the use of AI and precision agriculture techniques. Economic arguments, both on the producer and the national/global scale related to market value, can increase buy-in for policymakers intending to promote more environmentally sustainable agricultural practices. Combining the environmental and economic contexts in the messaging can broaden the potential scope and efficacy of the innovation as these messaging strategies can demonstrate an environmentally focused solution with potential economic benefits. An additional gap in the economic discourse on Twitter was accounting for the potential of job loss within the agricultural sector due to AI and the associated anxiety of this loss in the labor market
[17]. Without these discussions taking place within the public sphere (in this case, on social media), scientific innovations risk significant backlash and suspicion in the economic, social, and political contexts of the innovation both on the national and global scale
[17].
The frames oriented toward the social context of AI in agriculture primarily focused on social challenges, such as feeding a growing population and food security. The social frames were primarily discussed in a global context, relating to complex challenges at the nexus of climate change and pressures on the food system. However, AI has the potential to disrupt the social patterns in farming communities, relating not only to the social context but the political and cultural contexts as well. For example, the need for digital literacy and access could create unequal power structures within social systems where AI is implemented, especially in a global setting
[27][28][29][38,39,40], so strategies for outreach should be attuned to minimizing this risk considering these contexts simultaneously.
Within the behavioral context, communications surrounding the diffusion of AI in agriculture remain centered around prioritizing adoption, aligned with Rogers
[24] diffusion of innovation theory. However, limited messaging strategies incorporated other aspects of behavior change related to AI aside from the general benefits of adoption. In order to promote the social sustainability of AI in agriculture among not only producers, the messages should target policymakers and others with decision-making power to outline the needed actions for the sustainable integration of AI in agriculture across the diverse agri-food system. The contexts in which innovations exist continually overlap at the interstices of social, cultural, political, economic, environmental, and behavioral systems; thus, the potential unintended consequences of an innovation should not be discussed within an isolated context. Through the systems thinking approach, stakeholders are encouraged to anticipate the ripple effects of an innovation across these six contexts, increasing the sustainability of the innovation across the system.
Relative to the higher-level AI conversation, the discourse around the agricultural applications of AI is a small subset of the general AI discourse on Twitter. Remaining secluded from the broader AI communication spectrum, while potentially positive now due to the ability to avoid broader controversies attributed to AI, the agricultural sector is extremely susceptible to any backlash should a crisis occur or public opinion quickly turn. As evidenced in the results, the agricultural industry sits at a precipice of choosing to be proactive in obtaining public support for the use of AI to increase the sustainability of agriculture or remaining dependent upon blind techno-optimism that could limit the innovation’s resilience
[25][28].
The findings indicate that the practitioners working at the intersection of precision agriculture and sustainability have a unique opportunity to be proactive communicators and strive to build a relationship and connection to the public around AI’s use in agriculture. Connecting sustainability and precision agriculture in outreach efforts, by placing an emphasis on environmental sustainability, the public trust in production agriculture can be improved, especially with AI exhibiting the potential to have a massive global impact on the labor and food market
[2]. Specifically, social media communication campaigns should be developed that highlight the benefits of AI use in agriculture using visual imagery and video that the public can associate with solving environmental issues (e.g., reduced leaching into lakes and rivers, reduction in algae blooms in popular recreational areas, increased yields with less fertilizer and pesticide application). The communication campaigns could be collaborative, where scientists and agricultural companies and/or farmers are showcased working together to identify and evaluate the benefits of AI use. Once in place, the impact of the campaign should be measured, and compared to the baseline shared here, to determine if the public discourse is altered by the communication effort. Logically conducting public discourse analysis over time will ultimately help to predict backlash against any innovation that may be introduced, and, when combined with the systems thinking approach, improve the system-level sustainability of innovations prior to dissemination.
3. Conclusions
The overall discourse around AI and precision agriculture was generally positive, without widespread consideration of the potential drawbacks of the innovation, which supported previous research
[2][3][2,3]. The implications include emphasizing a systems thinking approach for both innovation development and dissemination to improve the system-level of an innovation. The framework depicted in
Figure 1 may assist as a reference point to ensure practitioners and scientists consider the various contexts in which the innovation will interact, emphasizing the need for considering the social, cultural, political, environmental, economic, and behavioral aspects of an innovation. With many livelihoods depending on the agricultural sector, combined with a broader need for increased sustainable practices, a systems thinking approach to innovation development and dissemination will combine critical thinking with evidence-based science to enhance sustainability across sectors.