Artificial intelligence (AI) is a game changer in many fields, including cultural heritage. It supports the planning and preservation of heritage sites and cities, enables the creation of virtual experiences to enrich cultural tourism and engagement, supports research, and increases access and understanding of heritage objects. Despite some impressive examples, the full potential of AI for economic, social, and cultural change is not yet fully visible.
1. Introduction
Digitization is key for protecting, preserving, documenting and opening up European and global cultural heritage (CH) to meet pressing sustainability threats, including environmental ones and increasing social inclusivity. Within the CH sector, economic activities related to digital collections in cultural institutions are a market worth ten bn EUR in 2015
[1]. These developments have been accelerated by the COVID-19 pandemic
[2]. Digital technologies can transform the entire value chain model in CH institutions—from capturing and digitizing tangible and intangible heritage and long-term preservation over innovative digital research methods to digital channels allowing people across the globe to interact with digital objects. These channels enable connections to other collections published on the web and accelerate the creation of new artistic works, unearthing new narratives in collections. While all these areas of work could be improved by applying the latest digital technologies, a significant increase is expected during the next few years.
The Strategic Topic Group (STG) Cultural Heritage in Green and Digital Transitions for Inclusive Societies was formed in 2022 within the European Institute of Innovation and Technology’s (EIT) Knowledge and Innovation Community for Culture & Creativity and seeks to unlock the potential of CH for the green and digital transitioning of Europe encompassing societal challenges on this key policy topic. The group includes 32 partner organizations in mid-2023 and focuses on four closely connected areas, including (i) upskilling and capacity building; (ii) environmental impact of operations of CH institutions; (iii) increasing outreach and community engagement; and (iv) creation of new business models. This article investigates the state of the art and proposes future steps to leverage artificial intelligence (AI), particularly machine learning (ML), for CH innovation.
2. Application Fields of AI in CH
In CH, AI is being used in a variety of research areas. These include:
-
Image analysis and restoration: AI algorithms can analyze and restore old, damaged, or degraded (moving) images, sounds, paintings, and photographs. These algorithms can enhance image quality, remove noise, and even reconstruct missing parts of the artwork, aiding in preserving and restoring cultural artifacts. Examples listed in
[3][27] are the prediction of the painting’s style, genre, and artist, the detection of fake artworks by stroke analysis, and the artistic style transfer using adversarial networks to regularize the generation of stylized images.” Further research deals with the automatic colorization of images
[4][31] and the restoration of ancient mosaics
[5][32].
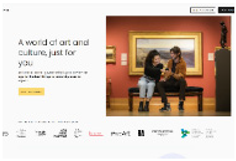 |
Smartify App by Smartify |
|
Smartify utilizes AI to provide interactive experiences with artworks in museums and galleries. The mobile app uses image recognition to identify artworks, delivering detailed information, audio guides, and curated tours. It is compatible with numerous cultural institutions across Europe and beyond. |
|
Link: |
https://smartify.org/ |
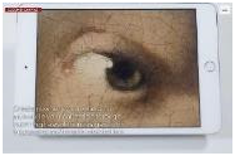 |
Second Canvas App by Madpixel and the Prado Museum |
|
The app uses AI technology to enhance the visitor experience. It provides high-resolution images of artworks, along with interactive features that allow users to explore the details and stories behind the paintings. |
|
Link: | https://www.secondcanvas.net/ |
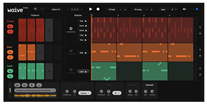 |
WAIVE WAIVE is a smart DJ system utilizing AI to create unique music samples, beats, and loops from the digitized audio archives of the Netherlands Institute for Sound & Vision. Link: https://www.thunderboomrecords.com/waive |
4. AI Technologies for CH State of the Art
4.1. AI and Images
Historical images hold immense value in documenting our collective heritage. However, analyzing and extracting information from these images manually can be limited, e.g., due to the required effort. Current evolvements in computer visualization are closely coupled to the massive renaissance in ML
[35][68] with the use of convolutional neural networks (CNNs, cf.
[36][69]). There is a large number of computer vision techniques employed in historical image analysis
[37][38][70,71], including:
-
Content-based image retrieval: Efficient retrieval and exploration of historical images based on visual similarity and content-based features. However, traditional ML technologies currently require large-scale training data
[3][39][40][41][27,72,73,74], which are only capable of recognizing well-documented and visually distinctive landmark buildings
[33][62] but fail to deal with less distinctive architecture, such as houses of similar style. Even using more advanced ML approaches or combining different algorithms
[42][75] only allows the realization of prototypic scenarios
[43][44][76,77].
-
59][90,91,92]. Approaches include these ML approaches commonly used in historical text analysis:
-
NLP techniques: Named entity recognition, part-of-speech tagging, sentiment analysis, and topic modeling. The most recent applications of CNNs and Transformer [
-
Preprocessing:60][93] are consistently successful in accurately extracting and reducing the number of errors even with unsupervised pre-training.
Includes character recognition (e.g., OCR), unification, processing of spelling variations and alignment to controlled vocabularies (e.g.,
[61][94]).
40][41][63][72,73,74,96], and to preselect imagery
[64][65][97,98]. Other tasks include AI-based semantic segmentation techniques to partition 3D models into meaningful regions or components
[66][99].
Object recognition and classification: AI-powered computer vision techniques enable automatic recognition and classification of cultural objects. By analyzing visual features and patterns, AI algorithms can identify and categorize artifacts, sculptures, and architectural elements
[6][33], facilitating the organization and cataloging of museum collections. Examples are the prediction of color metadata, e.g., for textile objects
[7][34], of technique, timespan, material, and place metadata for European silk fabrics
[8][35], and the recognition and classification of symbols in ancient papyri
Image-based localization[9][36].
: Connecting images with the 3D world relevant for AR/VR applications requires estimating the original six-degree-of-freedom (6DOF) camera pose. While several methods exist for homogeneous image blocks
[45][46][78,79], the problem becomes increasingly complex for varying radiometric and geometric conditions, especially relevant for historical photographs
[47][
Text classification algorithms80].
Translation and transcription: AI language models are capable of translating. e.g., ancient texts, inscriptions, and manuscripts into modern languages. They can also be used for modern languages by translating metadata or full-text content of heritage objects and related information, making sharing cultural heritage across languages easier. Other models can transcribe handwritten texts, allowing researchers and historians to access and understand historical documents and perform automated analysis (e.g.,
[10][37]).
-
Automatic text analysis: This comprises various approaches
[11
: Naive Bayes, Support Vector Machines, and Random Forests.
-
3D model creation: Research has focused on developing AI-based algorithms for efficient and accurate 3D reconstruction of CH objects, buildings, and sites. Traditional algebraic approaches, as in photogrammetry, employ algorithms within equations, e.g., to detect, describe, and match geometric features in images
[67][100] and to create 3D models. ML approaches are currently heavily researched and used for image and 3D point cloud analytics in CH (recent overview:
[3][27]), but increasingly for 3D modeling tasks. Generative adversarial networks (GAN), a combination of the proposal and assessment components of ML, are frequently employed as approximative techniques in 3D modeling, e.g., for single photo digitization
[68][101], completion of incomplete 3D digitized models
[69][70][102,103] or photo-based reconstructions
[71][104]. Recent approaches include neural radiance fields (NeRF)
[72][73][74][75][105,106,107,108], which have shown strength in creating 3D geometries from sparse and heterogeneous imagery and short processing time
[76][77][109,110].
Image recognition and classification: Identifying objects, scenes, or people depicted in historical images using deep learning models, such as CNNs. This field ranges from the detection of WW2 bomb craters in historical aerial images
[48][81], via historical photo content analysis
[49][82] to historical map segmentation
[50][51][52][83,[14184
-
Sequence models: Hidden Markov models, conditional random fields, and recurrent neural networks.
In addition, various preprocessing techniques are used for historical texts to enable their digital processing and respond to challenges such as linguistic variations, archaic vocabulary, and textual degradation:
-
Postprocessing: Used to check and correct any OCR reading errors via neural network approaches
[62][95].
-
-
Image to visualization approaches: Approaches bypass the modeling stage to generate visualizations directly from imagery
[39][78][79]].
4.6. AI and Audiovisual Material
Audiovisual heritage includes various materials such as films, videos, and multimedia content. AI for audiovisual heritage supports various aspects of preserving, analyzing, enhancing, and making accessible audiovisual content of historical and cultural significance. Key areas of application for AI in audiovisual heritage include:
-
Digitization and restoration: AI assists in digitizing and restoring deteriorating audiovisual materials, improving their quality and preserving their historical significance.
-
Video summaries: Can speed up the process of finding content in audiovisual archives
[99][142].
-
Content analysis and knowledge extraction: AI algorithms analyze audio and visual elements within content to identify patterns, objects, scenes, speakers, and other relevant information. It can also help to spot biases and contentious terms and track semantic drift in metadata, supporting curators, cataloguers, and others in deciding on potentially updating catalog records
[100][143112], e.g., by transforming or assembling image content (recent image generators like DALL-E
[80][113], Stable Diffusion or Midjourney). Other approaches based on NeRF to predict shifting spatial perspectives even from single images
[81][114] can predict 3D geometries.
].
][38]. An example is the automatic semantic indexing of pre-structured historical texts, which enables historians to mine large amounts of text and data to gain a deeper understanding of the sources (e.g.,
[12][39]); for example, tax lists or registers of letters sent to a historical entity
[
Semantic segmentation and object detection: Locating and recognizing specific objects or regions of interest within historical images using techniques like Faster R-CNN and YOLO. In semantic segmentation, to classify parts of images
[41][53]13][40].
-
-
Use of ML algorithms to detect patterns, anomalies, or changes over time within 3D models (e.g.,
[27]
-
Metadata enhancement: AI enriches metadata for better content organization, search, and context by extracting keywords or using LLMs to organize and enrich metadata records at scale.
Virtual Reality (VR) and Augmented Reality (AR): AI technology supports the creation of immersive VR and AR experiences for CH sites and museums. Visitors can virtually explore ancient ruins, historical sites, or museum exhibitions, interacting with AI-generated virtual characters or objects to enhance their understanding and engagement with the cultural context
[14
Image restoration and enhancement:] Repairing and enhancing degraded or damaged historical images through techniques like denoising, inpainting, and super-resolution
[55][15][41,[56][8842].
,
-
Transcription and translation:89].
AI-powered speech-to-text transcription and translation services make audiovisual content more accessible and understandable to a wider audience [
Recommender systems for personalized experiences: AI algorithms can analyze user preferences, historical data, and contextual information to provide personalized recommendations for CH experiences. Despite the risks of information filtering (e.g.,
[16][43]), use is to suggest relevant exhibits, customized tours, or tailored content, AI-powered recommender systems enhance visitor engagement and satisfaction, or—triggered by the advent of large language models (LLMs) such as GPT—dialogue and chatbot systems. Examples are the use of chatbots in museums
[17][18][44,
4.2. AI and Text
Historical texts provide a rich source of information for understanding the past. However, the sheer volume and complexity of historical archives make manual analysis laborious and time-consuming
[57][90]. ML algorithms supported these processes in various ways—from optical character recognition (OCR) to automating the extraction of knowledge and patterns from historical texts
[57][58][
4.3. AI and Virtual 3D Objects
The application of AI in 3D for CH has gained significant attention in the research community to enhance the analysis, interpretation, and preservation of CH in 3D environments. Here are some key areas of scientific analysis:
-
Object recognition and classification and semantic segmentation: In 3D/4D reconstruction of CH, ML-based technologies are currently used primarily for specific tasks. This involves AI models to identify specific architectural elements, artifacts, or decorative motifs, to recognize specific objects
[39][ [
]). The analysis involves assessing the effectiveness of AI in extracting meaningful information from large-scale 3D datasets, supporting archaeological research, conservation efforts, or architectural analysis.
45] or recommender systems for CH collections (e.g.,
[19][20][46,47]).
].
-
Partial audio matching: Supports framing analysis in identifying segments in one source audio file that are identical to segments in another target audio file. Framing analysis can reveal patterns and biases in the way content is being recontextualized in the media to shape public discourse
[102][145].
Cultural content analysis and interpretation: AI techniques, such as natural language processing (NLP), are used to analyze large volumes of cultural content, including literature, music, and artwork. This analysis can reveal patterns, themes, and cultural influences, providing valuable insights into historical contexts and artistic movements. Examples are metadata enrichment (e.g.,
[21][22][23][48,49,50]) and linking to open data sources (e.g.,
[6][33]).
-
Cross-modal analysis: AI techniques analyze both audio and visual components of content, facilitating holistic interpretation and understanding.
Heritage digitization and preservation: AI can be crucial in digitizing cultural artifacts and archives. By automating digitization processes and extracting knowledge, AI speeds up the preservation of CH, allowing researchers and the public to explore and study rare artifacts remotely. Several articles provide an overview of particular technologies, e.g., for 3D acquisition, such as laser scanning
[24][51] or photogrammetry
[25][52], and quantify their use
[26][53]. AI-powered systems can monitor and analyze CH site environmental conditions, helping with early detection of potential threats such as humidity, temperature fluctuations, and structural damage. This real-time monitoring aids in the proactive conservation and protection of cultural landmarks (e.g.,
[27][28][54,55]).
-
Interactive storytelling and content-generation interfaces: AI-powered interactive narratives and documentaries engage users with historical events and cultural context. AI can further enhance access by using fine-grained and time-based data extracted by AI systems as a basis for creating “generous interfaces” that allow for the rich exploration of CH collections
[103]
Multimodal analysis: AI is capable of bringing together different sources and types of data. Approaches include text, images
[8][35], 3D models
[29][56], audio
[30][57], and video
[31][58].
-
AI supports or creates artistic expressions: Applying algorithms that analyze heritage objects (or entire collections) and extract information that either artists and other creators can use to create new works
[32][59] or AI creating “artistic” expressions.
3. Project Examples
To date, there are some impressive examples of the utilization of AI technologies in the field of CH (Table 1).
Table 1.
Project examples of AI application in CH (all links accessed on 1 December 2023).
[
]
[
,
] and using conversational speech to provide new ways of interacting with audiovisual collections
[105][148].
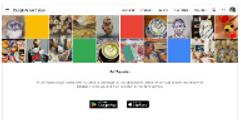 |
Art Transfer by Google Arts & Culture Using AI algorithms, Art Transfer allows users to transform their photos into the style of famous artists such as Van Gogh or Picasso. Link: https://artsandculture.google.com/camera/art-transfer |
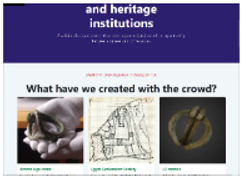 |
MicroPasts by the British Museum MicroPasts is a project that combines crowd-sourced data with AI technology. Volunteers contribute by digitizing and tagging images while AI algorithms analyze the data. Link: https://micropasts.org/ |
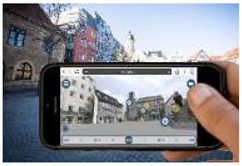 |
4Dcity by the University of Jena This application uses AI to automatically 4D reconstruct past cityscapes from historical cadastre plans and photographs. This 4D model is world-scale and enriched by links to texts and information, e.g., from Wikipedia, and accessible as mobile 4D websites [62]. Link: https://4dcity.org/ |
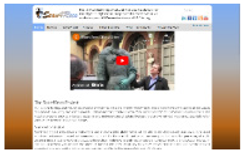 |
SCAN4RECO This EU-funded project combines 3D scanning, robotics, and AI to create digital reconstructions of damaged or destroyed CH objects. Link: https://scan4reco.iti.gr/ |
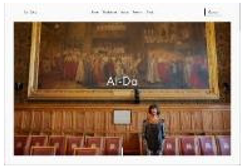 |
AI-DA by Aidan Meller Gallery AI-DA is an AI-powered robot artist developed by Aidan Meller Gallery in the United Kingdom. The robot uses AI algorithms to analyze and interpret human facial expressions, creating drawings and paintings inspired by the emotions it perceives. AI-DA’s artworks have been exhibited in galleries across Europe. Link: https://www.ai-darobot.com/ |
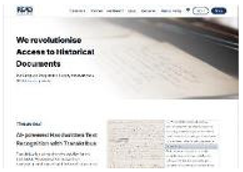 |
Transkribus by Read Coop SCE Transkribus is a comprehensive solution for digitization, AI-powered text recognition, transcription, and searching historical documents. A specific emphasis is on handwritten text recognition. https://readcoop.eu/transkribus/ |
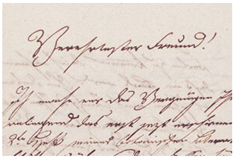 |
Transcribathon The Transcribathon platform is an online crowd-sourcing platform for enriching digitized material from Europeana. It applies the Transkribus handwriting recognition technology to input documents, performs some automatic enrichments (including translation) on the obtained text and metadata, and lets volunteers validate the results. https://transcribathon.eu/ |
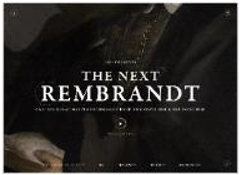 |
The Next Rembrandt by ING Bank and Microsoft This project employed AI algorithms to analyze Rembrandt’s works and create a new painting in his style. https://www.nextrembrandt.com/ |
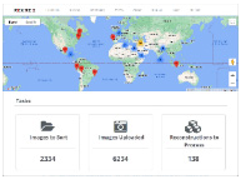 |
Rekrei (formerly Project Mosul) Rekrei is a crowd-sourcing and AI project aimed at reconstructing CH sites that have been destroyed or damaged. Users can contribute photographs and other data, and AI algorithms help in reconstructing the lost heritage digitally. https://rekrei.org/ |
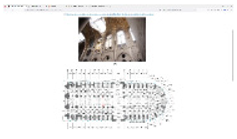 |
Notre Dame reconstruction After a fire destroyed parts of the Notre Dame Cathedral in Paris in 2019, a digital twin model was created to experiment—physical anastylosis, reverse engineering, spatiotemporal tracking assets, and operational research—and create a reconstruction hypothesis. The results demonstrate that the proposed modeling method facilitates the formalization and validation of the reconstruction problem and increases solution performance [63]. https://news.cnrs.fr/articles/a-digital-twin-for-notre-dame |
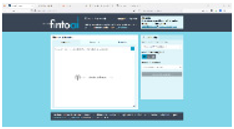 |
Finto AI by the National Library of Finland Finto AI is a service for automated subject indexing. It can be used to suggest subjects for text in Finnish, Swedish, and English. It currently gives suggestions based on concepts of the General Finnish Ontology, YSO. Link: https://ai.finto.fi |
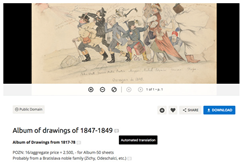 |
Europeana Translate This project has trained translation engines on metadata from the common European data space on cultural heritage in order to obtain a service that can translate CH metadata from 22 official EU languages to English, improving the multilingual experience provided to its users. It has been applied to 29 million metadata records so far. Link: https://pro.europeana.eu/post/europeana-translate-project-brings-together-multilingualism-and-cultural-heritage |
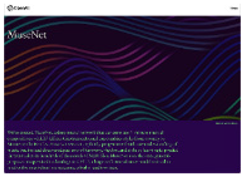 |
MuseNet by OpenAI MuseNet composes original music in a wide range of styles and genres. It can create music inspired by different cultural traditions and historical periods, demonstrating the potential of AI in generating new compositions that reflect CH. Link: https://openai.com/research/musenet |
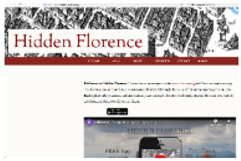 |
The Hidden Florence by the University of Exeter The Hidden Florence is an AI-enhanced mobile app that guides visitors through the streets of Florence, Italy, offering insights into the city’s rich CH in an engaging way. The app utilizes AI algorithms to provide location-based narratives, AR experiences, and interactive storytelling. Link: https://hiddenflorence.org/ |
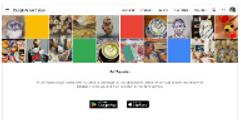 |
Art Transfer by Google Arts & Culture Using AI algorithms, Art Transfer allows users to transform their photos into the style of famous artists such as Van Gogh or Picasso. Link: https://artsandculture.google.com/camera/art-transfer |
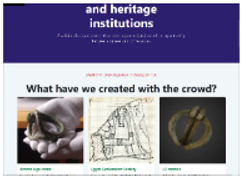 |
MicroPasts by the British Museum MicroPasts is a project that combines crowd-sourced data with AI technology. Volunteers contribute by digitizing and tagging images while AI algorithms analyze the data. Link: https://micropasts.org/ |
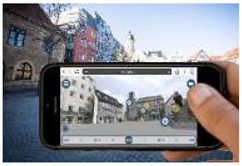 |
4Dcity by the University of Jena This application uses AI to automatically 4D reconstruct past cityscapes from historical cadastre plans and photographs. This 4D model is world-scale and enriched by links to texts and information, e.g., from Wikipedia, and accessible as mobile 4D websites [33]. Link: https://4dcity.org/ |
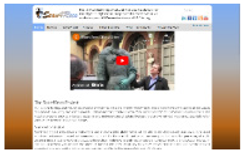 |
SCAN4RECO This EU-funded project combines 3D scanning, robotics, and AI to create digital reconstructions of damaged or destroyed CH objects. Link: https://scan4reco.iti.gr/ |
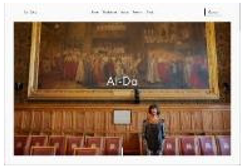 |
AI-DA by Aidan Meller Gallery AI-DA is an AI-powered robot artist developed by Aidan Meller Gallery in the United Kingdom. The robot uses AI algorithms to analyze and interpret human facial expressions, creating drawings and paintings inspired by the emotions it perceives. AI-DA’s artworks have been exhibited in galleries across Europe. Link: https://www.ai-darobot.com/ |
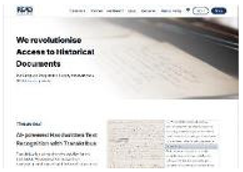 |
Transkribus by Read Coop SCE Transkribus is a comprehensive solution for digitization, AI-powered text recognition, transcription, and searching historical documents. A specific emphasis is on handwritten text recognition. https://readcoop.eu/transkribus/ |
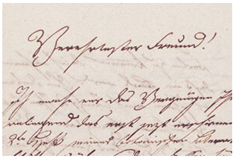 |
Transcribathon The Transcribathon platform is an online crowd-sourcing platform for enriching digitized material from Europeana. It applies the Transkribus handwriting recognition technology to input documents, performs some automatic enrichments (including translation) on the obtained text and metadata, and lets volunteers validate the results. https://transcribathon.eu/ |
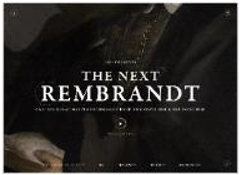 |
The Next Rembrandt by ING Bank and Microsoft This project employed AI algorithms to analyze Rembrandt’s works and create a new painting in his style. https://www.nextrembrandt.com/ |
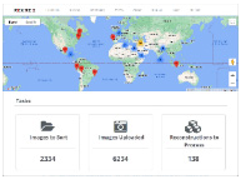 |
Rekrei (formerly Project Mosul) Rekrei is a crowd-sourcing and AI project aimed at reconstructing CH sites that have been destroyed or damaged. Users can contribute photographs and other data, and AI algorithms help in reconstructing the lost heritage digitally. https://rekrei.org/ |
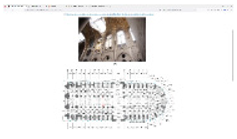 |
Notre Dame reconstruction After a fire destroyed parts of the Notre Dame Cathedral in Paris in 2019, a digital twin model was created to experiment—physical anastylosis, reverse engineering, spatiotemporal tracking assets, and operational research—and create a reconstruction hypothesis. The results demonstrate that the proposed modeling method facilitates the formalization and validation of the reconstruction problem and increases solution performance [34]. https://news.cnrs.fr/articles/a-digital-twin-for-notre-dame |
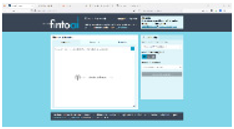 |
Finto AI by the National Library of Finland Finto AI is a service for automated subject indexing. It can be used to suggest subjects for text in Finnish, Swedish, and English. It currently gives suggestions based on concepts of the General Finnish Ontology, YSO. Link: https://ai.finto.fi |
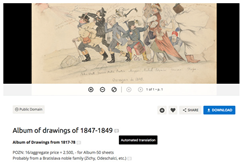 |
Europeana Translate This project has trained translation engines on metadata from the common European data space on cultural heritage in order to obtain a service that can translate CH metadata from 22 official EU languages to English, improving the multilingual experience provided to its users. It has been applied to 29 million metadata records so far. Link: https://pro.europeana.eu/post/europeana-translate-project-brings-together-multilingualism-and-cultural-heritage |
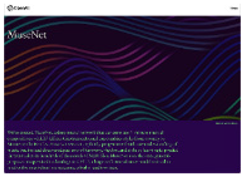 |
MuseNet by OpenAI MuseNet composes original music in a wide range of styles and genres. It can create music inspired by different cultural traditions and historical periods, demonstrating the potential of AI in generating new compositions that reflect CH. Link: https://openai.com/research/musenet |
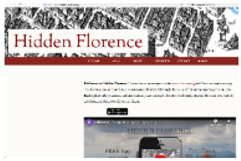 |
The Hidden Florence by the University of Exeter The Hidden Florence is an AI-enhanced mobile app that guides visitors through the streets of Florence, Italy, offering insights into the city’s rich CH in an engaging way. The app utilizes AI algorithms to provide location-based narratives, AR experiences, and interactive storytelling. Link: https://hiddenflorence.org/ |
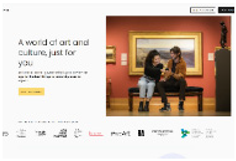 |
Smartify App by Smartify Smartify utilizes AI to provide interactive experiences with artworks in museums and galleries. The mobile app uses image recognition to identify artworks, delivering detailed information, audio guides, and curated tours. It is compatible with numerous cultural institutions across Europe and beyond. Link: https://smartify.org/ |
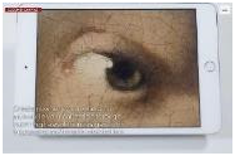 |
Second Canvas App by Madpixel and the Prado Museum The app uses AI technology to enhance the visitor experience. It provides high-resolution images of artworks, along with interactive features that allow users to explore the details and stories behind the paintings. Link: https://www.secondcanvas.net/ |
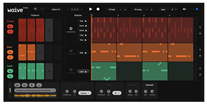 |
WAIVE WAIVE is a smart DJ system utilizing AI to create unique music samples, beats, and loops from the digitized audio archives of the Netherlands Institute for Sound & Vision. Link: https://www.thunderboomrecords.com/waive |
4.4. AI and Maps
The application of AI to cartographic corpora is relatively new and for now primarily addresses the need to segment historical cartography to extract graphs and assign semantic classes to them. To date, these approaches are still entirely manual in many cultural institutions, making it possible to extract useful information on the stylistic-graphic evolution of cartography or graphical elements of the past, such as the road network
[82][115] or the footprints of buildings on a large scale. Recently, the CNN approach has inaugurated some promising lines of study on segmentation
[83][84][85][116,117,118]. Historical cadastres provide a stable geometric medium to infer procedural 3D reconstructions
[86][119]. Because of their visual homogeneity, they can be segmented and annotated using CNN and Transformer approaches
[87][88][120,121].
4.5. AI and Music
The International Society for Music Information Retrieval defines Music Information Retrieval (MIR) as “a field that aims at developing computational tools for processing, searching, organizing, and accessing music-related data”
[89][132]. MIR utilizes various computational methods such as signal processing, ML, and data mining (i.e.,
[90][133]). MIR may use various forms of music data such as audio recordings, sheet music, lyrics, and metadata. Supervised ML relies on the accessibility of large datasets of annotated data. However, the dataset size can be increased by data augmentation. For sound, two data augmentation methods may be used: transformation and segmentation. Sound transformation transforms a music track into a set of new music tracks by applying pitch-shifting, time-stretching, or filtering. For sound segmentation, one splits a long sound signal into a set of shorter time segments
[91][134].
In terms of digital CH and its research, the following areas of MIR are relevant:
-
Automated music classification utilizes computer algorithms and ML techniques to automatically categorize music into classes or genres based on features extracted from the music data. Automated music classification has various applications, such as organizing music libraries and archives, and assisting in music research. Music-related classification tasks include mood classification, artist identification, instrument recognition, music annotation, and genre classification. For instance, one study investigates automatic music genre classification model creation using ML
[92][135].
-
Optical Music Recognition (OMR) research investigates how to computationally read music notation in documents
[93][136]. OMR is a challenging process that differs in difficulty from OCR and handwritten text recognition because of the properties of music notation as a contextual writing system. First, the visual expression of music is very diverse. For instance, the Standard Music Font Layout
[94][137] lists over 2440 recommended characters and several hundred optional glyphs. Second, it is only their configuration—how they are placed and arranged on the staves and with respect to each other—that specifies what notes should be played. The two main goals of OMR are:
- 1.
-
Recovering music notation and information from the engraving process, i.e., what elements were selected to express the given piece of music and how they were laid out. The output format must be capable of storing music notation, e.g., MusicXML
[95][138] or MEI
[96][139].
- 2.
-
Recovering musical semantics (i.e., the notes, represented by their pitches, velocities, onsets, and durations). MIDI
[97][140] would be an appropriate output representation for this goal.
-
Automatic Music Transcription (AMT) is the process of automatically converting audio recordings of music into symbolic representations, such as sheet music (e.g., MusicXML or MEI) or MIDI files. AMT is a very useful tool for music analysis. AMT comprises several subtasks: (multi-)pitch estimation, onset and offset detection, instrument recognition, beat and rhythm tracking, interpretation of expressive timing and dynamics, and score typesetting. Due to the very nature of music signals, which often contain several sound sources that produce one or more concurrent sound events that are meant to be highly correlated over both time and frequency, AMT is still considered a challenging and open problem
[98]