1. Visual Sensors
According to Figure 1A, visual sensors can be divided into 2D image sensors and 3D image sensors based on whether they simultaneously acquire depth information. The information obtained by 2D image sensors includes color, shape, texture, and other morphological characteristics. On the other hand, 3D image sensors can extract the three-dimensional information of complex target fruits and vegetables, obtaining richer data, such as the spatial coordinates of the target fruits and vegetables. Common visual sensors include monocular cameras, stereo cameras, structured light cameras, and multispectral cameras. Based on the selection of 49 publications, structured light cameras are the most commonly used visual sensors, as shown in Figure 1B.
Figure 1. (
A) Classification of visual sensors. (
B) Number of publications selected for each type of visual sensor. (
C) Census algorithm flow
[4][1]. (
D) Three-dimensional information acquisition model
[4][1]. (
E) Basic principles of depth information acquisition by structured light cameras
[5][2]. (
F) FPGA implementation of stereo matching
[19][3].
Therefore, the selection of visual sensors for fruit and vegetable harvesting robots varies depending on the growing environment. Please refer to Table 1 for specific information. This table summarizes the application principles and advantages and disadvantages of commonly used visual sensing technologies for fruit and vegetable harvesting robots.
1.1. Monocular Camera
In the field of agriculture, monocular cameras have been widely used for target localization in harvesting robots. The main challenge in monocular 3D object detection is the lack of depth information to infer the distance of objects
[3][4]. Therefore, combining visual algorithms can address this weakness. The Beijing Institute of Intelligent Agriculture Equipment utilizes a monocular camera to calculate the accurate coordinates of peripheral fruits by measuring the deviation between the top fruit center and the image center
[20][5] (as shown in
Figure 2A). The University of KU Leuven in Belgium has designed an apple harvesting robot
[21][6] that uses a monocular camera mounted at the center of a flexible gripper, ensuring the alignment between the gripper and the camera, simplifying the image-to-robot-end-effector coordinate transformation and reducing image distortion (as shown in
Figure 2B). When approaching an apple, the remaining distance to the apple is calculated through triangulation. The Graduate School of Agricultural Science at the Iwate University
[22][7] uses a monocular camera to locate the center of apple fruit and the detachment layer of the stem under natural lighting conditions, establishing their geometric relationship with a success rate of over 90%. Zhao et al.
[23][8] identify apples using a monocular camera based on color and texture features, achieving an accuracy rate of 90%. The Industrial and Systems Engineering Department at the University of Florida employs a single-camera system to obtain the three-dimensional position of citrus fruits for localization
[24][9]. Its characteristics are shown in
Table 1. In the future, the application of monocular cameras in agriculture needs to be further improved by combining other technological means.
Table 1.
Common visual sensors used in picking robots and their application principles and advantages and disadvantages.
Types of Sensors |
Applications and Principles |
Advantages |
Disadvantages |
Images |
Monocular camera |
Color, shape, texture, and other features |
Simple system structure, low cost, can be combined with multiple monocular systems to form a multi-camera system |
It can only capture two-dimensional image information, has poor stability, and cannot be used in dark or low-light conditions [25][10] |
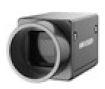 |
Stereo camera |
Texture, color, and other features; obtaining the spatial coordinates of the target through the principle of triangulation imaging |
By combining algorithms, the matching efficiency can be improved, and three-dimensional coordinate information can be obtained |
It requires high sensor calibration accuracy, and the stereo matching computation takes a long time. It is also challenging to determine the three-dimensional position of edge points |
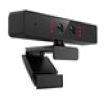 |
Structured camera |
Obtaining three-dimensional features through the reflection of structured light by the object being measured |
The three-dimensional features are not easily affected by background interference and have better positioning accuracy |
Sunlight can cancel out most of the infrared images, and the cost is high |
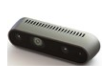 |
Multispectral camera |
Identifying targets based on the differences in radiation characteristics of different wavelength bands |
It is not easily affected by environmental interference |
It requires heavy computational processing, making it unsuitable for real-time picking operations |
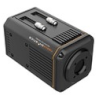 |
Monocular 3D object detection lacks depth information, and some researchers utilize the combination of monocular vision and LiDAR for recognition and localization tasks. Cao [26] utilizes two subsystems, LiDAR and monocular vision SLAM, for pose estimation. The visual subsystem overcomes the limitation of the LiDAR subsystem, which can only perform local calculations on geometric features, by detecting the entire line segments. By adjusting the direction of linear feature points, a more accurate odometry system is achieved. This system realizes more accurate pose estimation. Shu [27] proposes a recognition and localization method that includes LiDAR feature extraction, visual feature extraction, tracking, and visual feature depth recovery. By fusing inter-frame visual feature tracking and LiDAR feature matching, a frame-to-frame odometry module is established for rough pose estimation. Zhang [28] designs a visual system that trains using a database obtained by coupling LiDAR measurements with a complementary 360-degree camera. Cheng [29] based on distance, divides the depth map obtained from LiDAR and monocular images into various sub-images and treats each sub-image as an individual image for feature extraction. Each sub-image only contains pixels within a learning interval, thus obtaining rich pixel depth information. Ma [30] uses the original image to generate pseudo-LiDAR and bird’s-eye view, and then inputs the fused data of the original image and pseudo-LiDAR into a keypoint-based network for initial 3D box estimation.
1.2. Stereo Camera
Stereoscopic cameras capture depth information by simulating the parallax of human eyes. They utilize stereo matching algorithms to determine the spatial positions of picking points (as shown in
Figure 1C,D). The stereo matching algorithm calculates the disparity by subtracting the horizontal coordinates of matching points in the left and right images and then uses trigonometric measurement formulas to compute the baseline distance, the average distance between the two cameras, and the distance between the branch skeleton feature points and the cameras
[4][1].
Based on the stereo vision approach, the depth can be determined by utilizing the disparity between two monocular cameras
[13][16]. The University of Florida proposed the use of multiple monocular cameras for fruit localization in the context of robotic harvesting
[31][17]. Edan
[32][18] developed a stereo vision system using two monocular cameras for the detection and positioning of watermelons. The Queensland University of Technology in Australia
[33][19] captured images of dynamic clusters of lychee using two monocular cameras and employed stereo vision matching to calculate the spatial positions of interfered lychee clusters for picking (as shown in
Figure 2C,F). The depth accuracy range for the visual localization of lychee picking points was 0.4 cm to 5.8 cm. As shown in
Figure 2D, the Chinese Academy of Agricultural Mechanization Sciences
[34][20] used a mono-stereo vision sensor system to obtain the three-dimensional coordinates of apples. Mrovlje
[35][21] replaced two monocular cameras with a binocular stereo camera system to calculate the position of objects using stereo vision matching.
Pal
[36][22] uses the continuous triangulation method to generate a three-dimensional point cloud of the stereo camera. Wei
[37][23] obtains the three-dimensional information of apple tree obstacles through a binocular stereo camera. The binocular stereo system used by Wuhan University of Technology
[19][3] is implemented with the census stereo matching algorithm and FPGA, as shown in
Figure 1F. Guo
[38][24] captures images of lychee clusters using a binocular stereo vision system to obtain feature information such as the centroid and minimum bounding rectangle of lychee fruits, thereby determining the picking points. Jiang
[39][25] conducts recognition and localization research on tomatoes and oranges in greenhouse environments using a binocular stereo vision system. The Dutch Greenhouse Technology Company
[40][26] calculates the three-dimensional coordinates of cucumber stems using a stereo vision system. The Institute of Agricultural and Environmental Engineering
[41][27] utilizes a binocular stereo vision system to capture images of cucumber scenes and, thus, calculate the three-dimensional positions of cucumbers. Shown in
Figure 2G,H is a cucumber harvesting robot.
The advantages and disadvantages of stereo vision methods are shown in Table 1. When measuring targets, the depth of target edges may be lost, and this phenomenon worsens as the distance decreases. Therefore, it is difficult to accurately determine the three-dimensional positions of key points placed on target edges.
Figure 2. (
A) Tomato harvesting robot
[20][5]. (
B) Apple picking robot
[21][6]. (
C) Image processing of Lychee clusters in the orchard. (
a,
c) Lychee image, (
b,
d) calculation of picking points
[33][19]. (
D) Recognition and positioning system for harvesting robots
[34][20]. (
E) Components of harvesting robots
[42][28]. (
F) Lychee harvesting robot
[33][19]. (
G) Cucumber harvesting robot in the IMAG greenhouse
[41][27]. (
H) Cucumber harvesting robot in the IMAG greenhouse
[41][27]. (
I) Components of citrus harvesting robot
[43][29]. (
J) Hyperspectral imaging system
[44][30]. (
K) Region image acquisition
[45][31]. (
L) Tomato cluster harvesting robot system
[46][32]. (
M) NIR image
[47][33]. (
N) Visible spectrum image
[47][33]. (
O) Camera capturing pepper images with different levels of occlusion
[47][33]. (
P) Recognition and positioning in the orchard
[48][34]. (
Q) Illumination system layout with three lamps
[49][35]. (
R) Illumination system layout with three lamps
[49][35]. (
S) Strawberry end effector
[50][36].
1.3. Structured Camera
A structured light camera is a type of camera device that can capture surface details and geometric shapes of objects. It consists of one or more monocular cameras and a projector.
Figure 1E illustrates the basic principle of obtaining depth information using a structured light camera
[5][2]. It projects a series of known patterns onto the scene and performs correspondences between the projected frames and captured frames to obtain depth information based on the degree of deformation
[51,52][37][38]. Structured light camera systems are widely used in the field of robotics
[53][39]. They have the ability to measure depth with high precision and accuracy, as well as high speed
[54][40], and can obtain the three-dimensional coordinates of objects from the depth information in images
[55][41].
The National Center of Research and Development for Harvesting Robots in Japan
[42][28] utilizes a structured light camera to determine the three-dimensional positions of picking targets (as shown in
Figure 2E). The structured light camera is equipped with two infrared cameras, an infrared projector, and an RGB camera. It uses the two infrared cameras as a stereo camera to provide depth information and overlays color information with depth information using the RGB camera. The infrared projector projects image information to improve the accuracy of depth information. However, due to sunlight interference in outdoor environments during the daytime, most of the infrared images are offset.
Sa
[1][42] combined the detection results from color and near-infrared images to establish a deep fruit localization system. Shimane University
[56][43] constructed a cherry tomato harvesting robot equipped with a structured camera that uses three position-sensitive devices to detect the reflected beams of crops. By scanning laser beams, the shape and position of the crops are determined. Wang Z.
[57][44] used a structured camera to measure the size bounding box of mangoes. Rong
[58][45] employed a structured camera to detect the pedicels of tomatoes. South China University of Technology
[46][32] utilized a structured camera for tomato target recognition and localization, as shown in
Figure 2L. They obtained the optimal depth value of the harvesting point by comparing the difference between the depth average value and the original depth value of the picking point. The success rate of tomato harvesting point localization reached 93.83%. Nankai University
[59][46] used a structured camera to acquire depth information on vegetables. The Henan University of Science and Technology
[60][47] conducted edge recognition of leafy vegetables using a RealSense D415 depth camera. They extracted coordinate values in pixel coordinates and converted the pixel coordinates of the seedling edge to extremum coordinates. The researchers noticed that the RealSense D415 depth camera had calibration errors and the errors increased with the distance between the measured object and the depth camera. A ZED depth camera consists of left and right lenses and has a parallel optical axis
[61][48]. The Graduate School of Science and Technology
[62][49] at the University of Tsukuba used the ZED depth camera to obtain image information of pear orchards. The right lens produced depth images, while the left lens produced 4-channel RGB original images. When the operator’s distance to the pears was less than 50 cm, the ZED depth camera could obtain the distance and position of the pears through its depth function. Yang
[43][29] used the Kinect V2 depth camera to obtain the three-dimensional coordinates of citrus picking targets. As shown in
Figure 2I, they established the conversion relationship from the pixel coordinate system to the camera coordinate system by calibrating the camera and obtaining the intrinsic and extrinsic parameter matrices. By setting the top left and bottom right points of the position bounding box as the target points, they determined the three-dimensional coordinates of the target points. Their harvesting success rate reached 80.51%.
1.4. Multispectral Camera
With the development of spectral imaging technology, multispectral cameras have been used for fruit identification. By integrating spectral and imaging technologies in one system, multispectral cameras can obtain monochrome images at continuous wavelengths
[6][50]. Safren
[44][30] used a hyperspectral camera to detect green apples in the visible and near-infrared regions, as shown in
Figure 2J. Okamoto et al.
[45][31] proposed a method for green citrus identification using a hyperspectral camera with a spectral width between 369 and 1042 nm, as shown in
Figure 2K. They achieved the correct identification of 80–89% of citrus fruits in the foreground of the validation set. Queensland University of Technology in Australia
[47][33] proposed a multispectral detection system for field pepper detection. A set of LEDs was installed behind the multispectral camera to reduce interference from natural light. By using a threshold with near-infrared wavelengths (>900 nm) to segment the background from vegetation and a threshold with blue wavelength (447 nm) to remove non-vegetation objects in the scene, 69.2% of field peppers were detected at three locations, as shown in
Figure 2M–O. Bac
[63][51] developed a robotic harvesting system based on six-band multispectral data with a spectral width between 40 and 60 nm. The method produced fairly accurate segmentation results in a greenhouse environment, but the constructed obstacle map was not accurate. References
[64,65][52][53] designed a greenhouse cucumber harvesting robot based on spectral characteristics. Cucumber recognition was conducted by utilizing the spectral differences between cucumbers and leaves, achieving an accuracy rate of 83.3%.
2. Machine Vision Algorithms
Image segmentation, object detection, and 3D reconstruction are key technologies for the recognition and localization of fruit and vegetable harvesting robots. In Figure 3, different algorithms are shown, including branch and leaf segmentation, fruit and vegetable image segmentation, fruit and vegetable image detection, branch and leaf detection, and fruit and vegetable detection. These algorithms provide effective methods for image recognition and localization of fruit and vegetable harvesting robots.
Figure 3. Purposes of different image detection algorithms: (
a) branch and leaf segmentation
[66][54]; (
b) fruit and vegetable image segmentation
[7][55]; (
c) fruit and vegetable image detection
[67][56]; (
d) branch and leaf detection
[68][57]; (
e) fruit and vegetable detection
[9][58]; (
f) flowchart for obtaining the MFBB mask
[69][59]. (
g–
j) Results of stem and calyx recognition: (
g) results of stem and calyx recognition at gray level; (
h) 3D surface reconstruction image with a standard spherical model image; (
i) ratio image of 3D surface reconstruction image and the standard spherical model image; (
j) results of stem and calyx recognition
[70][60].
2.1. Image Segmentation Algorithms
In the process of fruit and vegetable harvesting robots, segmentation algorithms are used to distinguish fruits and vegetables from the background and separate them. Traditional feature-based algorithms for segmentation include the following methods: depth threshold segmentation, shape-based segmentation, similarity measurement, and image binarization segmentation. Machine learning algorithms can utilize a large amount of training data for model learning and training, thereby improving the accuracy and robustness of segmentation. As shown in Table 2, common machine learning segmentation algorithms include semantic segmentation algorithms and instance segmentation algorithms. Figure 4 shows the application proportion of image segmentation algorithms in the context of harvest recognition and localization. The selection of these segmentation algorithms depends on specific application scenarios and requirements and can be chosen and combined based on the actual situation of fruit and vegetable harvesting robots.
Figure 4.
The proportion of image segmentation algorithm applications in harvest recognition and localization.
Table 2.
Image segmentation algorithms for harvesting recognition and localization.
(1) Depth thresholding segmentation. Depth threshold segmentation is a method of dividing an image into different blocks by extracting key features. When the value of a specific pixel in the image is greater than the set threshold, it is assigned to the corresponding block for segmentation. The Beijing Intelligent Agriculture Equipment Center highlights the differences between fruit clusters and the background using the R-G color difference model and performs depth threshold segmentation on the fruit cluster region
[71][61], achieving an accuracy rate of 83.5%. Zhao successfully segmented tomato fruits using an adaptive threshold segmentation algorithm, achieving a recognition rate of 93%
[72][62]. Chongqing University of Technology used the HSV threshold segmentation algorithm to identify citrus targets
[73][63]. The combination of deep threshold segmentation algorithm and other algorithms can improve the results of recognition and localization. Suzhou University proposed an algorithm that combines Mask R-CNN and deep threshold segmentation for the recognition and localization of row–frame tomato picking points, and the results showed that the deep threshold algorithm helps in filtering the background, with a successful localization rate of 87.3% and an average detection time of 2.34 s
[74][64]. Zhejiang University of Technology performed initial segmentation on an image based on the deep threshold algorithm
[75][65].
(2) Similarity measure segmentation. Image segmentation methods based on similarity measurement involve the process of classifying or clustering data objects by computing the degree of similarity between two objects. As shown in
Figure 3a, Zhejiang University of Technology achieves cucumber image segmentation and detection by individually calculating the normalized cross-correlation (NCC) matrix with the target image
[66][54], with an accuracy rate of 98%. The Suzhou Industrial Park Sub-Bureau proposed a fruit target segmentation algorithm based on a color space reference table, which does not require complex operations on the background and can effectively remove the background, thereby improving the real-time operability of the picking robot
[76][66].
The clustering segmentation algorithm is a type of image segmentation method based on similarity measurement, which divides pixels in the image into different clusters, each representing a particular image region or object. When combined with thresholding and other algorithms, clustering segmentation algorithms can achieve better segmentation results. South China University of Technology combines K-means clustering segmentation algorithm, depth threshold segmentation algorithm, and morphological operations to obtain the image coordinates of tomato cluster picking points
[46][32]. The detection time is 0.054 s with an accuracy rate of 93.83%. The experimental results showed a significant reduction in noise within the region of the stem of interest after removing part of the background using the K-means clustering segmentation algorithm. In the literature, K-means clustering segmentation and Hough circle fitting were applied to achieve image segmentation of citrus fruits
[77][67]. The detection time is 7.58 s with an accuracy rate of 85%. South China Agricultural University used an improved fuzzy C-means clustering method to segment images of lychee fruits and their stems and calculated the picking points for lychee clusters to determine their spatial positions
[33,78][19][68]. The detection time is 0.46 s with an accuracy rate of 95.3%.
(3) Image binarization segmentation. The Otsu algorithm is a classic image binarization method that uses the grayscale histogram to determine the optimal threshold and convert the image from grayscale space to binary space. Shandong Agricultural University utilizes the Otsu algorithm and maximum connected domain to quickly identify and segment target grape images in two-dimensional space
[79][69]. This method achieves fast and effective separation of grapes and backgrounds based on Otsu, with a recognition success rate of 90.0% and a detection time of 0.061 s.
(4) Shape segmentation algorithm. Shape segmentation refers to the use of specific shapes to segment meaningful regions and extract features of the targets. South China Agricultural University successfully segmented the contour of banana stalks through edge detection algorithms
[80][70]. The detection time is 0.009 s with an accuracy rate of 93.2%. The Zhejiang University of Technology and Washington State University use the Hough circle transform method to identify apples, as it can better recognize all visible apples
[75][65]. The detection time ranges from 0.025 to 0.13 s, with an error rate of less than 4%. However, traditional image segmentation algorithms are sensitive to lighting changes in natural environments, making it difficult to accurately extract information about obstructed fruits and branches.
(5) Semantic segmentation algorithms. Semantic segmentation algorithms are machine learning algorithms that associate each pixel in the image with labels or categories to identify sets of pixels of different categories. Jilin University employs the PSP-Net semantic segmentation model to perform semantic segmentation of the main trunk ROI image, extract the centerline of the lychee trunk, and obtain the pixel coordinates of the picking points in the ROI image to determine the global coordinates of the picking points in the camera coordinate system
[81][71]. The accuracy rate of this method is 98%. Combining semantic segmentation algorithms with other algorithms can achieve better segmentation results. Nanjing Agricultural University proposes a mature cucumber automatic segmentation and recognition method combining YOLOv3 and U-Net semantic segmentation networks. This algorithm uses an improved U-Net model for pixel-level segmentation of cucumbers and performs secondary detection using YOLOv3, yielding accurate localization results
[82][72]. The accuracy rate of this method is 92.5%.
(6) Instance segmentation algorithms. Instance segmentation not only classifies pixels but also classifies each object, thus enabling the simultaneous recognition of multiple objects. In the identification and positioning of fruit and vegetable picking, the main instance segmentation algorithms used are Mask R-CNN and YOLACT. Northwestern A&F University proposed an improved Mask R-CNN algorithm for tomato recognition
[7][55], with a processing time of 0.04 s per image. The accuracy rate of this method is 95.67%. As shown in
Figure 3b, detection is performed using YOLACT, Mask R-CNN, and the improved Mask R-CNN, with the improved Mask R-CNN producing more accurate detection results.
Beijing Intelligent Agricultural Equipment Center proposed a tomato plant main stem segmentation model based on Mask R-CNN
[83,84][73][74]. The accuracy rate of this method is 93%. It locates the centerline of the main stem based on the recognized mask features and then calculates the image coordinates of the harvesting points by offsetting along the lateral branches from the intersection points of the centerline. The University of Lincoln in the UK proposed a Mask R-CNN segmentation detection algorithm with critical point detection capability, which is used to estimate the key harvesting points of strawberries
[85][75]. Yu Y achieved three-dimensional positioning of strawberry harvesting points by extracting the shape and edge features from the mask images generated by Mask R-CNN
[86][76]. The accuracy rate of this method is 95.78%. South China Agricultural University proposed a method for main fruit branch detection and harvesting point localization of lychees based on YOLACT
[69][59]. The detection time is 0.154 s with an accuracy rate of 89.7%. As shown in
Figure 3f, this method utilizes the instance segmentation model YOLACT to obtain clusters and masks of the main fruit branches in the lychee images and selects the midpoint as the harvesting point. At the same time, it uses skeleton extraction and least squares fitting to obtain the main axis of the lychee’s main fruit branch mask, thereby obtaining the angle of the lychee’s main fruit branch as a reference for the robot’s harvesting posture. The use of machine-learning-based image segmentation algorithms allows for the separation of targets and backgrounds, enabling robots to more accurately recognize and locate crops. By automatically learning rules from data, the crop recognition and localization capabilities of robots are improved.
2.2. Object Detection Algorithm
The object detection algorithm is a key algorithm in the recognition and localization work of fruit and vegetable harvesting robots. Researchers have conducted extensive research on the stability, real-time performance, and accuracy of object detection algorithms. Among them, the YOLO object detection model has the characteristics of fast detection speed, small model size, and easy deployment. Figure 5 shows the commonly used YOLOv7 model structure, while the proportion of YOLO model optimization methods applied in harvest recognition and localization is depicted in Figure 6. In order to improve the performance of the algorithm, researchers have taken some optimization measures, as shown in Table 3, such as adding residual modules (ResNet), changing or replacing the backbone feature extraction network, combining the K-means clustering algorithm, adding attention mechanism modules, improving activation functions, etc.
Figure 6.
The proportion of applied YOLO model optimization methods in harvest recognition and localization.
Table 3.
YOLO model optimization algorithm.
[87][88] have achieved fruit target detection by deepening the network model and processing the dataset to identify regions of interest.
2.3. A 3D Reconstruction Algorithm for Object Models
The goal of a 3D reconstruction algorithm is to convert 2D images or point cloud data into 3D object models. The target three-dimensional reconstruction algorithms in crop harvesting recognition and localization are shown in Table 4.
Table 4.
Object three-dimensional reconstruction algorithm in crop harvesting recognition and localization.
(1) Introducing residual modules ResNet. The Residual Network (ResNet) module allows the YOLO object detection model to perform deeper processing. The idea behind ResNet is to establish direct connections between preceding and subsequent layers to aid gradient backpropagation
[87][77]. Chongqing University of Posts and Telecommunications introduced residual modules based on YOLOv4 and constructed a new network that enhances small object detection in natural environments
[67][56]. The accuracy rate of this method is 94.44%, with a detection time of 0.093 s. South China Agricultural University incorporated the residual concept into the YOLOv3 model, addressing the issue of decreased detection accuracy due to increased network layer depth
[98][89]. The accuracy rate of this method is 97.07%, with a detection time of 0.017 s.
(2) Modifying or replacing the backbone feature extraction network. The role of the backbone feature extraction network is to extract more feature information from the image for subsequent network usage. Several studies have proposed improved backbone feature extraction network schemes. Hunan Agricultural University
[8][78] designed a new backbone feature extraction network based on YOLOv3 for fast detection of citrus fruits in natural environments. The accuracy rate of this method is 94.3%, with a detection time of 0.01 s. Qingdao Agricultural University
[68][57] combined the fast detection capability of YOLOv3 with the high-precision classification ability of DenseNet201, enabling precise detection of tea shoots. The accuracy rate of this method is 95.71%. DenseNet201 utilizes multiple convolutional kernels on smaller-sized feature maps to extract rich features and mitigate gradient vanishing problems. Shandong Agricultural University
[88][79] replaced the YOLOv5 model with multiple LC3, DWConv, and Conv modules, reducing network parameters while enhancing the fusion of shallow-level features, facilitating the extraction of features from small objects. DWConv divides standard convolution into depthwise convolution and pointwise convolution, promoting information flow between channels and improving operation speed. The accuracy rate of this method is 94.7%, with a detection time of 0.467 s. Dalian University
[89][80] replaced CSPDarknet53 in YOLOv4 with DenseNet to decrease gradient vanishing, strengthen feature transmission, reduce parameter count, and achieve the detection of cherry fruits in natural environments. Northwest A&F University
[91][82] replaced BottleneckCSP with BottleneckCSP-2 in YOLOv5s for accurate detection of apples in natural environments. The accuracy rate of this method is 86.57%, with a detection time of 0.015 s. The Shandong University of Science and Technology
[90][81] designed a lightweight backbone feature extraction detection network, LeanNet, that uses convolutional kernels with different receptive fields to extract distinct perceptual information from the feature map. It generates local saliency maps based on green peach features, effectively suppressing irrelevant regions in the background of branches and leaves for the detection of green peaches in natural environments. The accuracy rate of this method is 97.3%.
(3) Applying the K-means clustering algorithm for combining predicted candidate boxes. Based on YOLOv3, the feature extraction capability of the model can be enhanced by performing K-means clustering analysis on the predicted bounding boxes of leafy objects
[43,92][29][83]. South China Agricultural University
[93][84] proposed a small-scale lychee fruit detection method based on YOLOv4. This method utilizes the K-means++ algorithm to cluster the labeled frames to determine anchor sizes suitable for lychees. The K-means++ algorithm solves the problem of the K-means algorithm being greatly affected by initial values. The accuracy rate of this method is 79%. Guangxi University
[94][85] proposed an improved YOLOv3 algorithm for cherry tomato detection. This algorithm uses an improved K-means++ clustering algorithm to calculate the scale of the anchor box, thereby extracting more abundant semantic features of small targets and reducing the problems of information loss and insufficient semantic feature extraction of small targets during network transmission in YOLOv3. The accuracy rate of this method is 94.29%, with a detection time of 0.058 s.
(4) Incorporating attention mechanism modules. The attention mechanism module can focus more on the pixel regions in the image that have a decisive role in classification while suppressing irrelevant regions. Northwest A&F University
[91][82] added the SE module to YOLOv5s for fast apple recognition on trees. The accuracy rate of this method is 86.57%, with a detection time of 0.015 s. Jiangsu University
[95][86] integrated the CBAM module into YOLOX-Tiny to achieve fast apple detection in natural environments. The accuracy rate of this method is 96.76%, with a detection time of 0.015 s. Shandong Agricultural University
[92][83] proposed the SE-YOLOv3-MobileNetV1 algorithm for tomato ripeness detection, which achieved significant improvements in terms of speed and accuracy by introducing the SE module. The accuracy rate of this method is 97.5%, with a detection time of 0.227 s.
(5) Enhancing the activation function. Activation functions provide nonlinear functionality in the network structure, playing an important role in network performance and model convergence speed. Improved activation functions can enhance the detection performance of the model. For example, Dalian University
[89][80] replaced the activation function with ReLU in their research on the YOLOv4 object detection model. This improvement increased inter-layer density, improved feature propagation, and promoted feature reuse and fusion, thereby improving detection speed. The accuracy rate of this method is 94.7%, with a detection time of 0.467 s. In addition, other studies
[91,96,97][82]
The Norwegian University of Life Sciences
[9][58] identified harvestable strawberries by fitting the 3D point cloud to a plane. As shown in
Figure 3e, they used coordinate transformation, density-based clustering of base points, and position approximation methods to locate segmented strawberries. This algorithm can accurately determine whether the strawberries are within the safe harvest area, achieving a recognition accuracy of 74.1%.
Nanjing Agricultural University
[99][90] proposed a fast reconstruction method for greenhouse tomato plants. They used the nearest point iteration algorithm for point cloud registration from multiple viewpoints, which has high accuracy and stability. The accuracy rate of this method is 85.49%. Shanghai Jiao Tong University
[70][60] utilized a multispectral camera and a structured camera to reconstruct the upper surface of apple targets in 3D.
Figure 3g–j shows the schematic diagram of stem and calyx recognition results. The height information of each pixel can be calculated using triangulation, providing a reference for identifying the picking points of apple stems, with a recognition accuracy of 97.5%. Norwegian University of Science and Technology, Trondheim
[100][91], presented a more real-time and accurate 3D reconstruction algorithm based on ICP. This method can generate 3D point clouds with a resolution of 1 mm and achieve optimal results through 3D registration and reference trajectory optimization on a linear least squares optimizer. The GPU implementation of this method is 33 times faster than the CPU, providing a reference for the recognition and localization of fruit and vegetable harvesting robots.